Dear friends,
If you wonder how often I take online courses myself, the answer is: Quite often. I have a longstanding interest in AI for healthcare. I had some time off during the Fourth of July holiday in the U.S., and finished the Johns Hopkins course on clinical trial design taught by Professors Janet Holbook and Lea Drye.
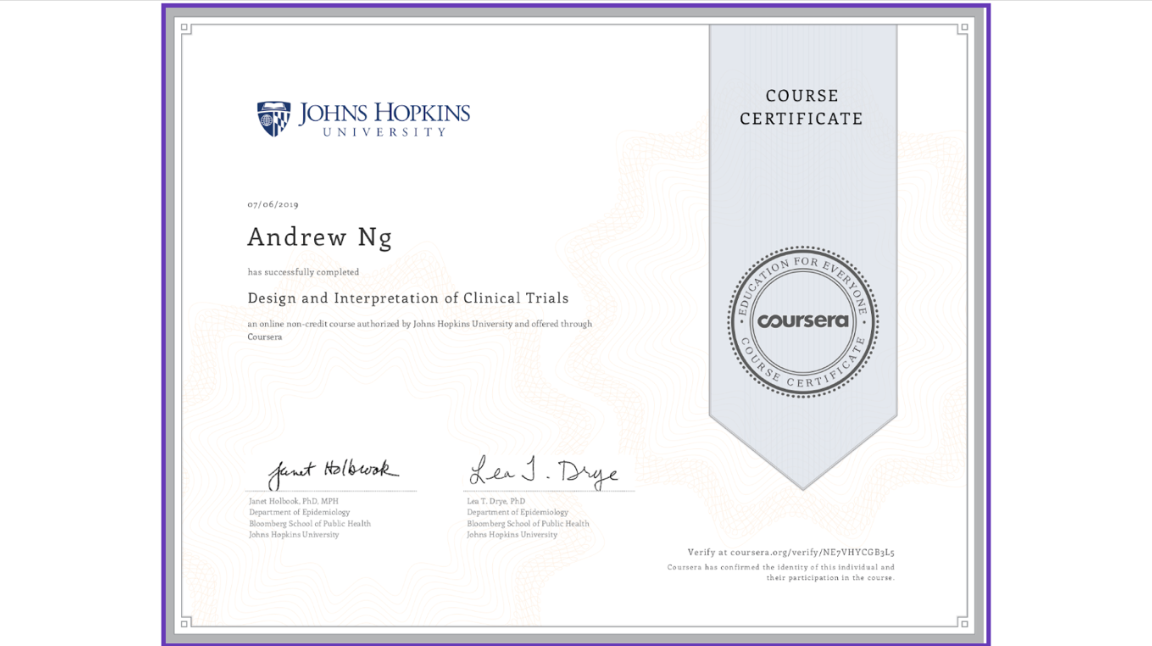
As AI practitioners, to work effectively on AI+X, we have to learn a bit about X as well. Whether you want to work on AI for healthcare, climate change, manufacturing, agriculture, logistics, or something else, opportunities abound and I encourage you to both find collaborators in application area X as well as learn about it yourself.
Keep learning!
Andrew
News
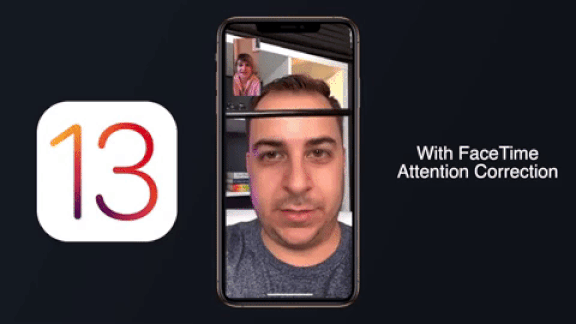
Then Their Eyes Locked — Not!
Eye contact is such an essential element in interpersonal communication that it’s considered rude in face-to-face conversation to avoid another person’s eyes. But a lowered gaze is standard in video chat, when the face on the screen is often several inches lower than the camera’s lens. Guess what? There’s an app for that!
What’s new: Apple added a feature to its FaceTime video-chat app that warps the image of your face, so people you chat with will think you’re looking them in the eye.
How it works: FaceTime Attention Correction works like a Snapchat filter, continually adjusting a map of the user’s face so the eyes appear to look at the camera. A MacRumors video highlights the warping effect: A drinking straw — the dark horizontal line in the clip above — curves slightly as it passes over the commentator’s eyes. The feature reportedly works only on Apple’s newest phones, the iPhone XS and XS Max. It can be switched off in the settings.
Behind the news: Mike Rundle, a product designer at Intuit, noticed the gaze-fixing feature and pointed it out in a tweet. In fact, he had predicted the feature in a 2017 blog on the future of the iPhone. He analyzed Apple’s recent acquisitions and told readers to look out for “advanced image-manipulation algorithms that make sure FaceTime calls always show your eyes looking at the other person.”
Why it matters: Anything that might improve interpersonal communication is worth exploring. Yet our perception of reality is increasingly subject to automated tampering. For instance, Zoom's videoconferencing system offers a "touch up my appearance" switch that subtly smoothes facial wrinkles. Apple added gaze correction without notice, but it did provide a way to turn it off. Companies with a lower standard of accountability to users could seed communication tools with features that mediate communications without your knowledge or control.
Takeaway: Will this new feature make video chat more intimate? Or will it lead to less-present telepresence as people who seem fully engaged are actually scanning Reddit? While we mull the answer, we’ll be on the lookout for software that flags manipulated facial expressions during video chats.
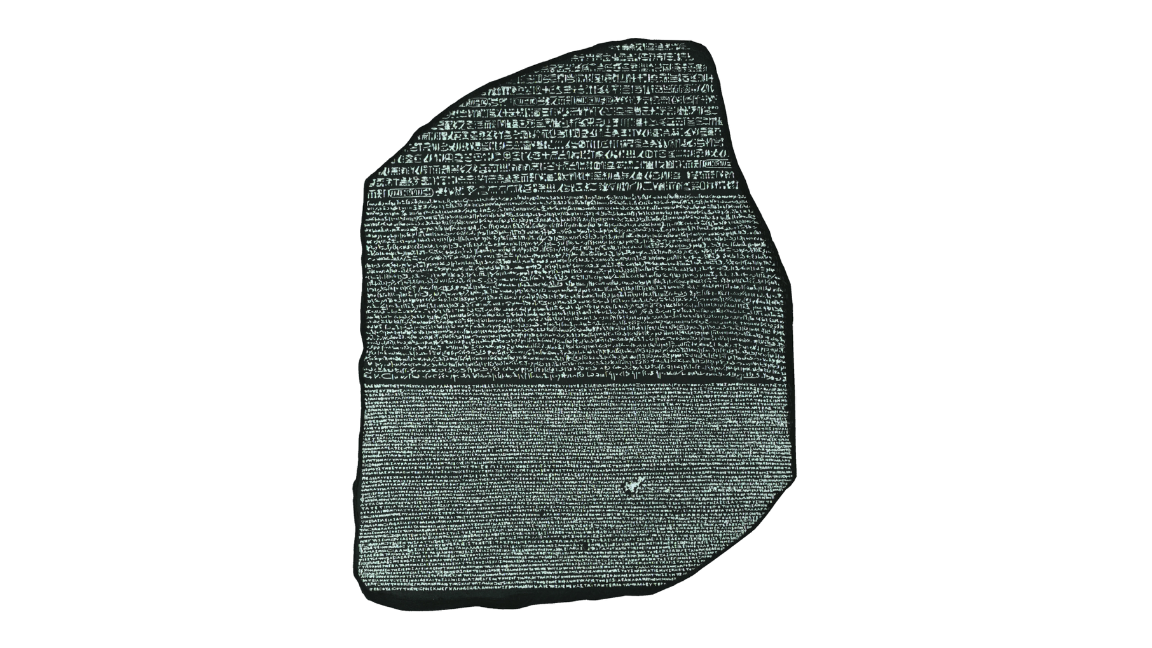
Digital Rosetta Stone
Translating languages that haven't been understood since ancient times typically requires intensive linguistic research. It turns out that neural networks can do the job.
What’s new: Researchers at MIT CSAIL and Google Brain devised an algorithm that deciphers lost languages. It’s not the first, but it achieves state-of-the-art results across a variety of tongues.
Key insight: The new approach identifies cognates, words in different languages that have the same meaning and similar roots. Cognates follow consistent rules, such as:
- Related characters appear in similar places in matching cognates.
- The vocabulary surrounding cognates is often similar, since they have the same meaning.
How it works: The new method is based on a mapping between cognates in an unknown language and a known language.
- The mapping begins at random.
- A sequence-to-sequence LSTM network draws its ground truth from the map.
- Given a word in a lost language, the LSTM tries to predict the spelling of that word in the known language.
- The map is updated (using a mathematical structure, commonly used in operations research, known as a flow network) to minimize the distance between predicted spellings and actual words in the known language.
- The network and map bootstrap one another as they converge on a consistent mapping.
Results: The new approach outperforms previous methods on Ugartic, a relative of Hebrew, translating cognates with up to 93.5 percent accuracy. It also sets a new state of the art in translating the proto-Greek script Linear B into Greek, spotting cognates with 84.7 percent accuracy.
Why it matters: Previous translation algorithms for lost languages were designed specifically for a particular language. The new method generalizes to wildly dissimilar tongues and achieves stunningly high accuracy.
Takeaway: Historically, specialists labored for decades to decipher the thoughts encoded in lost languages. Now they have a general-purpose power tool. We look forward to ancient secrets revealed.
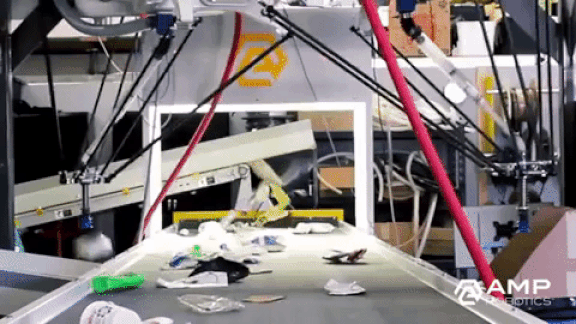
Picking Up the Pieces
Sorting data into the right categories is AI's bread-and-butter task. Now the technology is being used to sort recyclables into the right bins.
What’s new: Single Stream Recyclers of Sarasota, Florida, recently increased its fleet of AI-equipped sorters from six to 14. That's the largest deployment of recycling robots in the U.S. and possibly the world, according to The Robot Report.
Bot master: Single Stream uses sorters built by AMP Robotics, which combines proprietary deep learning software with off-the-shelf robotics. Its machines use suction-tipped appendages to sort 80 pieces of waste a minute — twice as fast as the average for humans, the company claims — and work much longer hours. The robots are in use in the U.S., Canada, and Japan.
How it works: AMP trained its model on photos of waste material. It learned to distinguish not only newspapers from milk cartons, but also various classes of plastics and metals. The system reportedly achieves high accuracy in spite of discards that are often battered, dented, crumpled, and dirty.
Behind the news: Recycling makes economic sense if producing goods from old materials is less expensive than making them afresh. Using humans to sort recyclables is expensive in Western countries, and robots can reduce the cost. An alternative is to ship waste to places like China, but such relationships are susceptible to geopolitical turmoil — not to mention the greenhouse gases emitted shipping waste across the ocean.
Why it matters: The average American produces nearly four and a half pounds of trash daily. Globally, daily waste tops 3.5 billion tons. Much of this doesn’t degrade and winds up in oceans or seeping into the food supply. More efficient recycling keeps more waste out of the environment and conserves resources for other uses.
We’re thinking: The cost-benefit ratio of recycling is hotly debated and difficult to calculate. Of course, robots aren’t cheap — AMP, a private company, doesn’t publicize its prices or sales — but they clearly have potential to cut immediate costs. Meanwhile, recycling itself saves the external costs of environmental degradation. AMP’s success suggests that recycling plants, at least, are finding the tradeoff worthwhile.
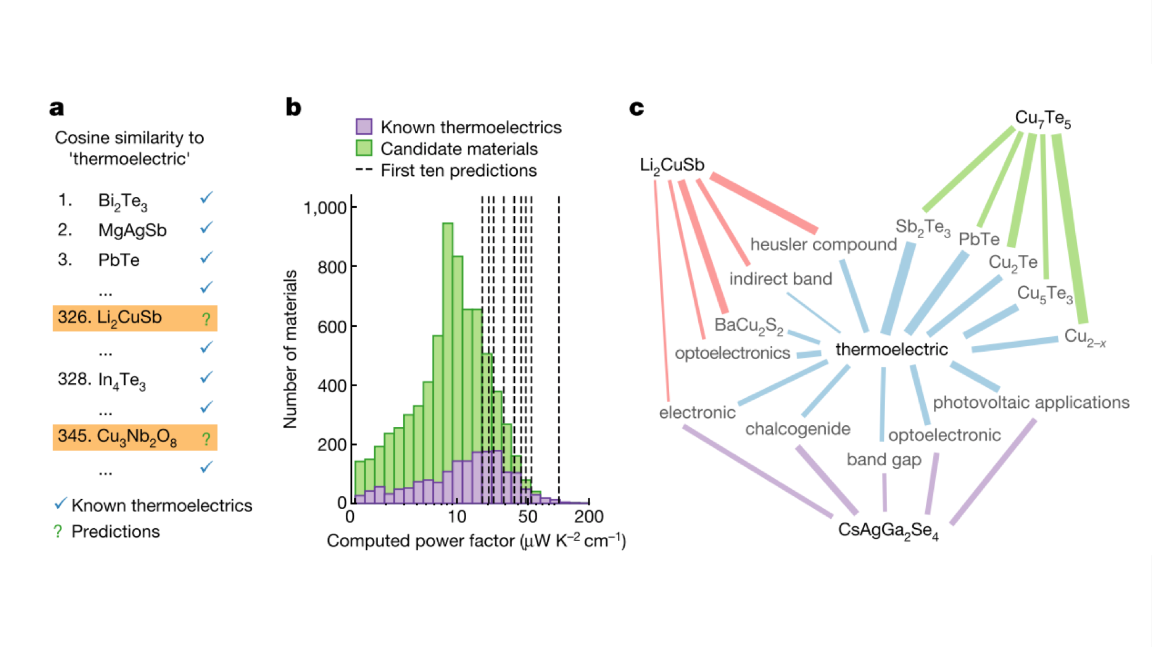
Science on Steroids
Science is drowning in data. Hard-won findings can sink into obscurity amid the rising tide of research. But new research shows that deep learning can serve as a net for catching knowledge.
What’s new: A neural network trained by researchers at the Department of Energy’s Berkeley Laboratory found materials with special properties by parsing abstracts published between 1922 and 2018.
How it works: Berkeley’s library of 3.3 million abstracts contains roughly 500,000 distinct words.
- The researchers assigned each word a vector value in 200 dimensions, numerically describing its relatedness to every other word.
- Their model manipulated the vectors to discover fundamental terms, concepts, and principles of materials science.
- In the course of educating itself, the model produced a ranked list of materials that were strong candidates for having thermoelectric properties, meaning they convert heat to energy.
- The researchers checked the predicted materials against the historical record and found that their method could shave years off the process of discovering new materials. For instance, of the neural network's top five predictions based on research published prior to 2009, one wasn’t discovered until 2012, and two others had to wait until 2018.
Why it matters: Pick a field of science, and you’ll find loads of research that haven’t yet been wrung for insight. Untold insights hide therein. This work “suggests that latent knowledge regarding future discoveries is to a large extent embedded in past publications,” the researchers write. Now scientists may be able to take advantage of that knowledge to make more rapid progress.
Takeaway: Artificial intelligence has been touted as a way to extrapolate new discoveries from extant research. Now it’s beginning to make good on that promise. Recent experiments have shown success in physics, chemistry, astronomy, and genomics. Other computationally intensive fields — for instance medicine, economics, and climatology — are in line for breakthroughs.
A MESSAGE FROM DEEPLEARNING.AI
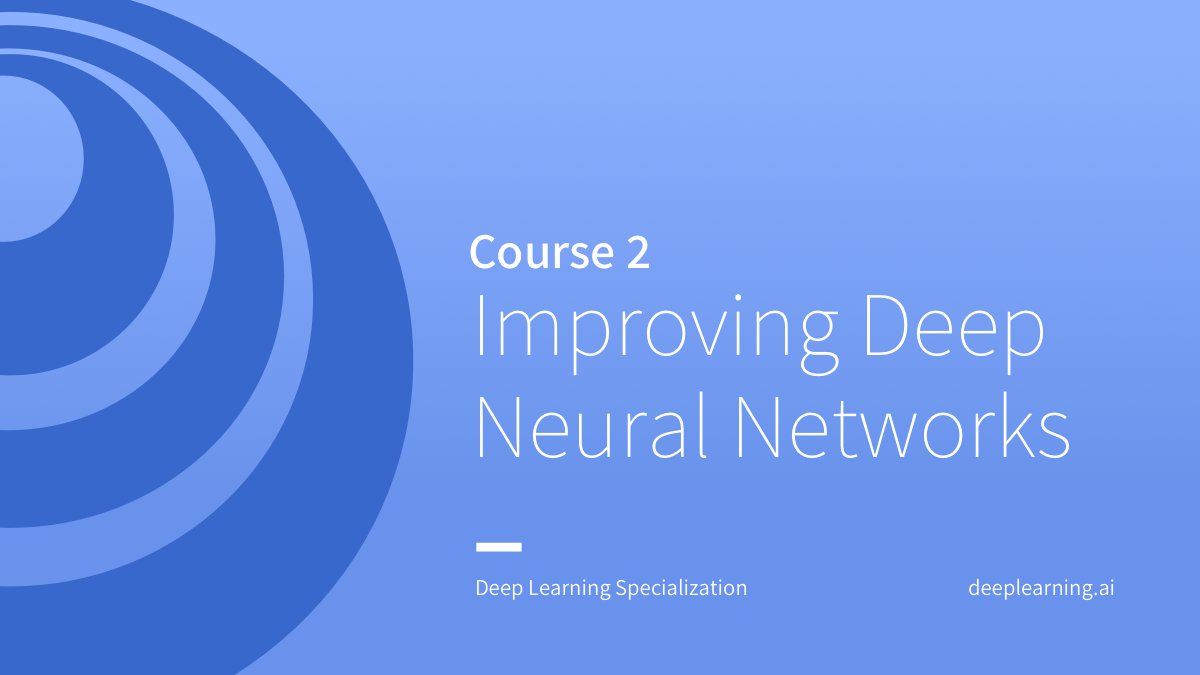
Learn essential neural network tricks including initialization, L2 and dropout regularization, batch normalization, and gradient checking. Enroll now in the Deep Learning Specialization.
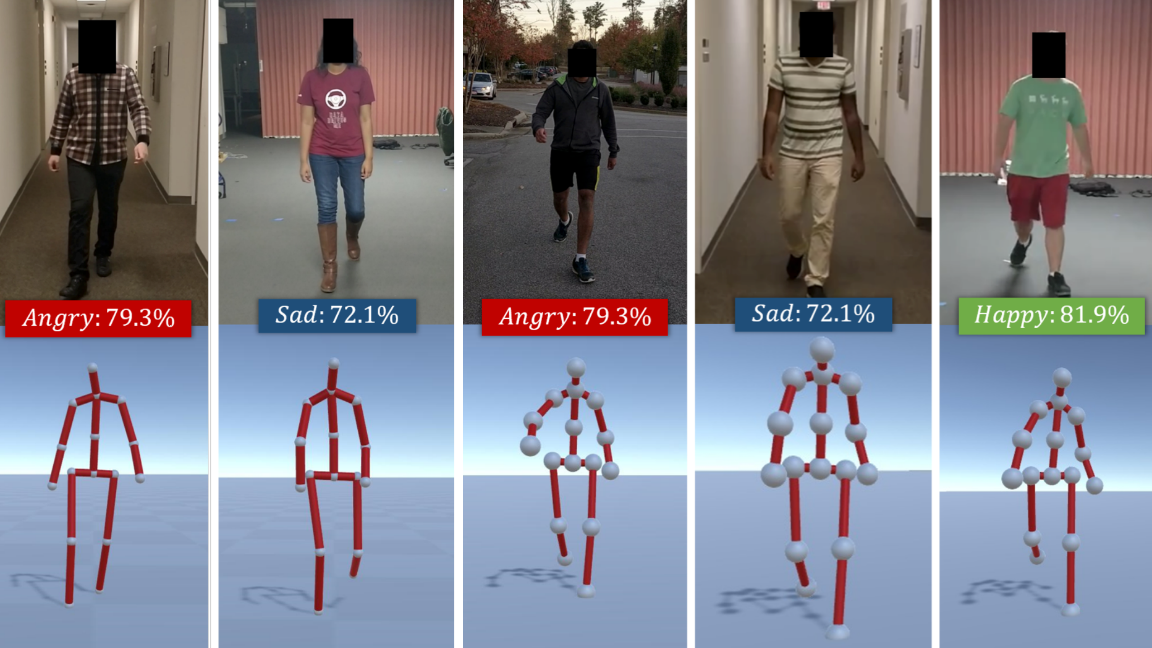
Emotions in Motion
Automated systems for interpreting human emotions have analyzed speech, text, and facial expressions. New research shows progress on the subtle art of reading body language.
What’s new: Researchers from the Universities of North Carolina and Maryland built EWalk, a collection of 1,348 videos of people walking, manually labeled according to perceived emotions: happy, sad, angry, and neutral. For instance, gaits labeled happy tend to have a quick pace and widely swinging arms, while sad gaits are slower with a slumped posture. A model trained on EWalk achieved state-of-the-art results matching gaits with emotional states.
Key insights: The model uses a random forest to classify the emotion expressed by a given gait using a combination of features extracted using hand-crafted rules and a neural network.
- Decision trees in this case are less data-hungry than neural networks, but they're prone to overfitting large-dimensional inputs.
- The researchers pre-processed the data using an LSTM to reduce the number of dimensions describing the input.
How it works: TimePoseNet, a neural network detailed in previous research, extracts from videos a 3D skeletal representation of the gait in each frame. The researchers compute pose and movement features from the skeleton and feed them to the random forest. They also feed the skeleton to an LSTM network, which supplements the random forest’s input.
- Pose measurements include the volume of the bounding box surrounding a skeleton, area of bounding boxes for upper and lower body, distance between feet and hands, and angles between head and shoulders as well as head and torso.
- Each pose measurement is averaged across all frames to produce the pose feature. Maximum stride length is also included in the pose feature, but not averaged over frames.
- Movement measurements include the average of the velocity, acceleration, and jerk (rate of change in acceleration) of each skeletal joint in each frame. The time of a single walk cycle, from the moment a foot lifts to the time it hits the ground, is also a movement measurement.
- Each movement measurement is averaged over all frames to produce the movement feature.
- The random forest takes pose and movement features as inputs to predict emotion.
- LSTM is also trained to predict emotion from the pose and movement features. The random forest receives the values of the LSTM’s hidden units, but not its prediction.
Results: The previous best method achieved 68 percent accuracy on EWalk. The random forest, given the pose and movement features plus the LSTM’s hidden units, achieves 80.1 percent. The random forest also outperforms the LSTM alone.
Why it matters: The better computers understand human emotion, the more effectively we’ll be able to work with them. Beyond that, this work has clear applications in security, where early warning of potential aggression could help stop violent incidents before they escalate. It could also be handy in a retail environment, helping salespeople choose the most productive approach to prospective customers, and possibly in other face-to-face customer service situations.
We’re thinking: Psychology demonstrates that emotion affects human planning and actions. While models exist that are startlingly accurate at predicting future human actions — see this paper — they don’t explicitly take emotion into account. Factoring in emotions could enable such systems to make even better predictions.
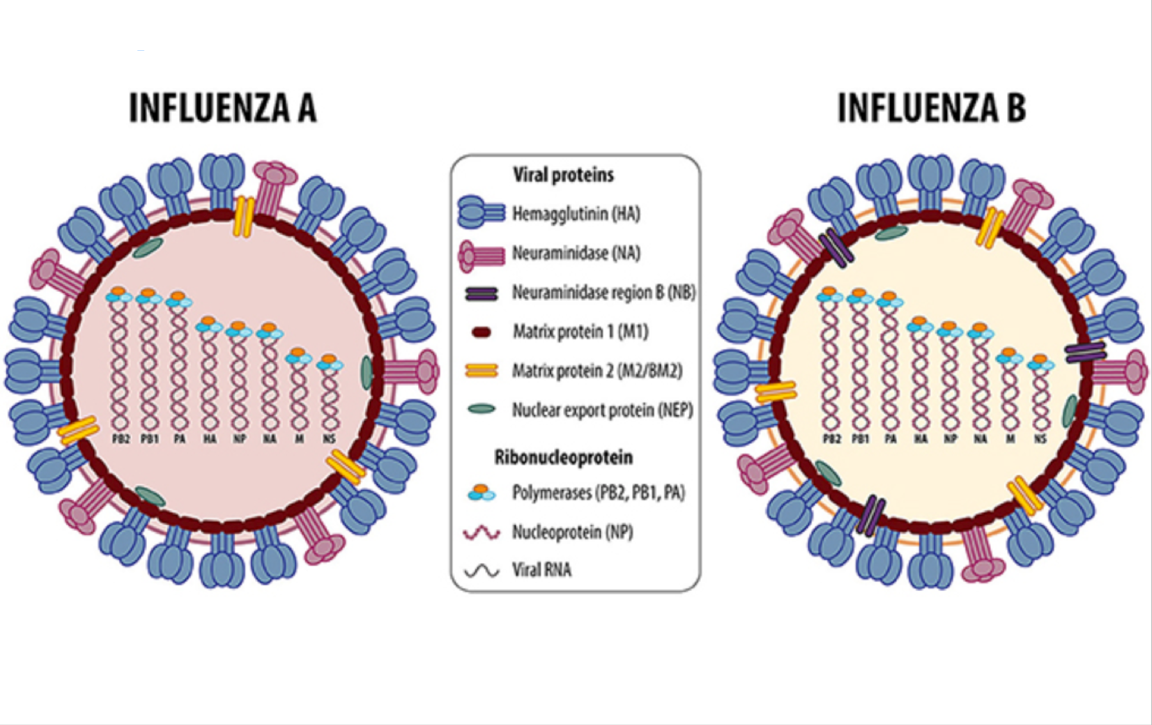
Automating Immunity
Viruses evolve at a breakneck clip. That keeps medical researchers working overtime to develop vaccines. Now AI is designing inoculations that could help humans stay one step ahead of the bugs.
What’s new: The first AI-generated vaccine has entered clinical trials in the U.S. Nikolai Petrovsky, a professor at Flinders University in South Australia who led the fight against swine flu in 2009, is heading up the project.
How it works: Petrovsky and his colleagues trained a neural network to discover new substances that boost the body’s immune response to a specific trigger. Called the Search Algorithm for Ligands, the model sifted through the medical literature on vaccine efficacy. Then it invented trillions of compounds and predicted their effectiveness. The team synthesized and tested the model’s top suggestions, and the results were promising enough that the U.S. National Institute of Allergy and Infectious Diseases sponsored a year-long trial.
Behind the news: In the war of immunization, the battle line can shift within a single season. This year’s flu vaccine was no match for a late-season mutation, and its effectiveness dropped from 47 percent to 9 percent.
Why it matters: Flu killed an estimated 79,000 people during the 2017-2018 season. AI that fast-tracks effective vaccines could save countless lives.
Takeaway: The number of recent flu victims is a small fraction of the historic toll, and evolution could enable it to come roaring back. AI could allow for rapid response to new strains before they show a glimmer of pandemic potential.
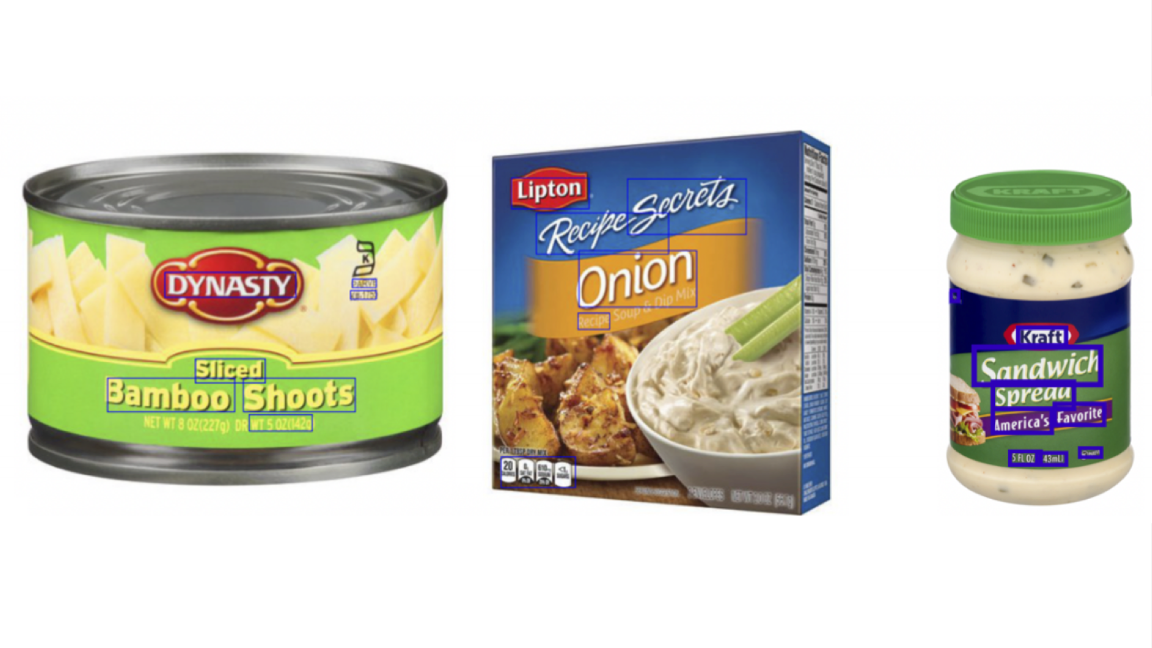
New! Improved! Text Recognition
The ability to recognize text is useful in contexts from ecommerce to augmented reality. But existing computer vision systems fare poorly when a single image contains characters of diverse sizes, styles, angles, and backgrounds along with other objects — like, say, loudly designed commercial packaging. Researchers at Walmart’s Bangalore lab devised an algorithm to tackle this problem.
What’s new: A team put together an end-to-end architecture that segments images into regions and feeds them into existing text extraction networks. The system outputs boxes containing the extracted text.
Key insights: The approach is twofold: Segment, then extract. The team, led by Pranay Dugar, found that:
- Segmenting an image into regions before extracting text simplifies the task by minimizing background noise and handling varied text styles separately.
- Using an ensemble of text extraction networks improves the performance of an end-to-end system. And the ensemble can work in parallel, so it’s exceptionally fast.
How it works: The system segments images by creating and classifying superpixels: groups of adjacent pixels of similar color and intensity. It feeds them into pretrained text extraction networks and merges the outputs.
- To generate superpixels, the authors dilate homogeneous regions by convolving the image in the following way: As the kernel slides over the image, the pixel value at the center is replaced by the maximum value of the region overlapping the kernel. Higher pixel values fill in neighboring lower pixel values, which expands foreground regions (i.e., text) and shrinks background regions (gaps between objects).
- To classify superpixels, the authors create vectors composed of the mean, standard deviation, and energy of filters at various scales and orientations. Vectors close in Euclidean distance correspond to the same text region. Superpixels are grouped by maximizing the probability that they belong together.
- To extract text, the authors run TextBoxes and TextBoxes++ models pretrained on the ICDAR 2013 data set of photos depicting commercial packaging, business signs, and the like. They prune redundant text boxes from the two models by keeping the one with the highest confidence score.
Results: The system is competitive with earlier methods on ICDAR 2013. But it excels with so-called high-entropy images that are unusually complex. It improves both precision (the proportion of predictions that are correct) and recall (the proportion of correct labels predicted) by 10 percent on the authors' own Walmart High Entropy Images data set.
Why it matters: Extracting words from images containing multiple text-bearing objects is difficult. The letters may be poorly lit, slanted at an angle, or only partially visible. Jumbled together, they can give a computer vision system fits. Segmenting text regions and then using the ensemble of text-extraction models makes the problem more tractable.
Takeaway: In a world increasingly crowded with signs, symbols, and messages, applications of such technology are numerous. It could mean efficient creation of digital restaurant menus from physical copies, or an agent that lets you know when an ice cream truck passes your house. It gives machines a way to read words in chaotic environments — and possibly new ways to communicate with us.