Dear friends,
I’ve been thinking about AI and ethics. With the techlash and an erosion of trust in technology as a positive force, it’s more important than ever that we make sure the AI community acts ethically.
There has been a proliferation of AI ethical principles. This paper surveys 84 such statements. These statements are a great start, but we still need to do better.
Take, for example, the OECD’s statement: AI should benefit people and the planet by driving inclusive growth, sustainable development, and well-being. When I ask engineers what effect such statements have on their day-to-day actions, they say, “pretty much none.” It is wonderful that the OECD is thinking about this. But we need more actionable codes of ethics that give more concrete and actionable suggestions.
I described earlier struggling with an ethical decision of whether to publicize an AI threat. It’s in situations like that we need better guidelines and processes for decision making.
Many existing AI ethics codes come from large corporations and governments. But if we hope that the global AI community will follow a set of guidelines, then this community — including you — needs to have a bigger voice in its development. We need an ethical code written by the AI community, for the AI community. That will also be the best way to make sure it truly reflects our community’s values, and that all of us buy into it and will follow it.

Last Friday, deeplearning.ai hosted our first Pie & AI on AI and ethics. Four cities joined us: Hong Kong, Manila, Singapore, and Tokyo. We started with an interactive discussion, and each city came up with three actionable ethics statements, preferably starting with, “An AI engineer should …” The ideas they presented ranged from seeking diverse perspectives when creating data to staying vigilant about malicious coding. I was heartened to see so many people motivated to debate ethical AI in a thoughtful way.
I hope to do more events like this to encourage people to start the conversation within their own communities. This is important, and we need to figure this out.
I would love to hear your suggestions. You can email us at hello@deeplearning.ai.
Keep learning!
Andrew
News
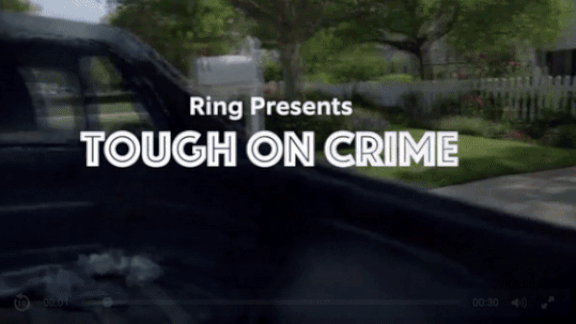
Neighborhood Watchers
Smart doorbell maker Ring has built its business by turning neighborhoods into surveillance networks. Now the company is drawing fire for using private data without informing customers and sharing data with police.
What’s new: A three-part exposé in Vice is the latest in a series of media reports charging that Ring, an Amazon subsidiary, mishandles private data in its efforts to promote AI-powered “smart policing.”
How it works: Ring’s flagship products are doorbells with integrated camera, microphone, and speaker. The camera’s face and object recognition software alerts users, via a smartphone or connected-home device, whenever someone or something enters its field of view. Users can talk with people at the door remotely through the microphone and speaker.
- Ring has found success promoting its devices as crime-prevention tools. It bolsters this reputation by advertising its partnerships with more than 600 U.S. police departments and 90 city governments. In return for the implicit endorsement, Ring provides police departments with free devices and access to user data.
- The company exports user data to its R&D office in Kiev, Ukraine, to train its models. A December 2018 report by The Information documented a lax security culture in Kiev, where employees routinely shared customer video, audio, and personal data. Ring says it uses only videos that users have shared publicly (generally with neighbors and/or police) through an app, and that it has a zero-tolerance policy for privacy violations.
- Google Nest, Arlo, Wyze, and ADP also offer smart doorbells. Ring, however, is the only one sharing data with police departments.
Behind the news: When it was founded in 2012, Ring was called DoorBot and marketed its system as a hassle-free way to see who’s at the door. The following year, founder Jamie Siminoff appeared on the TV game show Shark Tank. While he didn’t sway the show’s celebrity investors, he raised several million dollars soon after, and changed the name to Ring. In recent years, he has pivoted from promoting the doorbell as a way to enhance social life to positioning it as a crime-prevention tool. In 2018, Amazon purchased Ring for $839 million and now markets the product as part of its Alexa smart-home services.
Pushback: Troubled by the privacy implications of sharing customer data with police, 36 civil rights groups published an open letter calling for a moratorium on partnerships between Ring and public agencies. The letter also asked Congress to investigate the company.
We’re thinking:
Knock knock.
Who’s there?
Stopwatch.
Stopwatch who?
Stopwatching me and respect my privacy!
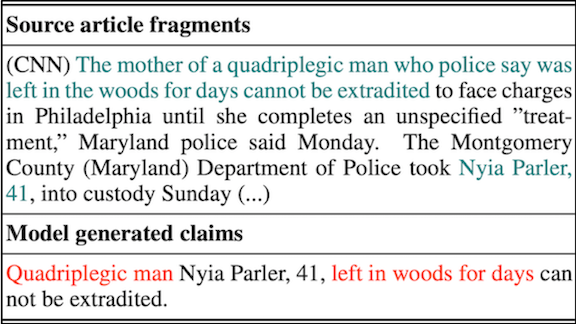
Keeping the Facts Straight
Automatically generated text summaries are becoming common in search engines and news websites. But existing summarizers often mix up facts. For instance, a victim’s name might get switched for the perpetrator’s. New research offers a way to evaluate factual consistency between source documents along with a measure to evaluate it.
What’s new: Wojciech Kryściński and colleagues at Salesforce Research introduce FactCC, a network that classifies such inconsistencies. They also propose a variant called FactCCX that justifies the classifications by pointing out specific inconsistencies.
Key insight: Earlier approaches to checking factual consistency determine whether a single source sentence implies a single generated sentence. But summaries typically draw information from many sentences. FactCC evaluates whether a source document as a whole implies a generated sentence.
How it works: The authors identified major causes of factual inconsistency in automated abstractive summaries (that is, summaries that don’t copy phrases directly from the source document). Then they developed programmatic methods to introduce such errors into existing summaries to generate a large training dataset. FactCC is based on a BERT language model fine-tuned on the custom dataset.
- The researchers created a training dataset by altering sentences from CNN news articles. Transformations included swapping entities, numbers, or pronouns; repeating or removing random words, and negating phrases (“snow is in the forecast” versus “snow is not in the forecast”).
- Some transformations resulted in sentences whose meaning was consistent with the source, while others resulted in sentences with altered meaning. The authors labeled them accordingly.
- The development and test sets consisted of sentences from abstractive summaries generated by existing models. Each sentence was labeled depending on whether it was factually consistent with the source.
- BERT received the source document and a sentence from the generated summary. It predicted a binary classification of consistent or inconsistent.
Results: FactCC classified summary sentences with an F1 score of 0.51. By contrast, a BERT model trained on MNLI, a dataset of roughly 400,000 sentence pairs labeled as either concordant or contradictory, achieved an F1 score of 0.08. In a separate task, FactCC ranked pairs of new sentences (one consistent, one not) for consistency with a source. It awarded consistent sentences a higher rank 70 percent of the time, better by 2.2 percent than the best previous model ranking the same dataset.
Why it matters: A tide of automatically generated text is surging into mainstream communications. Measuring factual consistency is a first step towards establishing further standards for generated text—indeed, an urgent step as worries intensify over online disinformation.
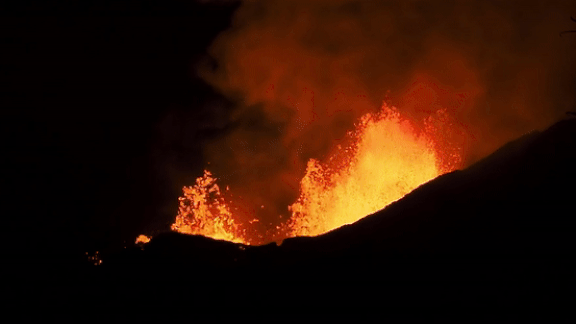
Predicting the Next Eruption
AI is providing an early warning system for volcanoes on the verge of blowing their top.
What happened: Researchers at the University of Leeds developed a neural net that scans satellite data for indications that the ground near a volcano is swelling—a sign it may be close to erupting.
How it works: Satellites carrying certain sensors can track centimeter-scale deformations of Earth’s crust. Seismologists in the 1990s figured out how to manually read this data for signs of underground magma buildups. However, human eyeballs are neither numerous nor sharp enough to monitor data for all of Earth’s 1,400 active volcanoes.
- Matthew Gaddes, Andy Hooper, and Marco Bagnardi trained their model using a year’s worth of satellite imagery leading up to the 2018 eruption of a volcano in the Galapagos Islands.
- Data came from a pair of European satellites that passed over the volcano every 12 days.
- The model differentiates rapid ground-level changes associated with catastrophic eruptions from slower, more routine deformations.
Behind the news: Researchers at the University of Bristol developed a similar method to measure deformations in the Earth’s crust using satellite data. However, their model can be fooled by atmospheric distortion that produces similar signals in the data. The Leeds and Bristol groups plan to collaborate in side-by-side tests of their models on a global dataset in the near future. Another group based at Cornell University is attempting to make similar predictions through satellite data of surface temperature anomalies, ash, and gaseous emissions.
Why it matters: Approximately 800 million people live within the blast zones of active volcanoes, and millions of sightseers visit their slopes each year. On Monday, New Zealand’s White Island volcano erupted, killing at least five tourists.
We’re thinking: If researchers can scale their model up to cover the entire globe, they’ll deserve applause that thunders as loudly as Krakatoa.
A MESSAGE FROM DEEPLEARNING.AI
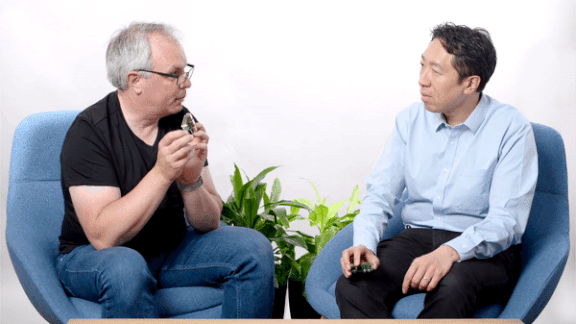
Bringing an AI system into the real world involves a lot more than just modeling. Learn to deploy on various devices in the new deeplearning.ai TensorFlow: Data and Deployment Specialization! Enroll now
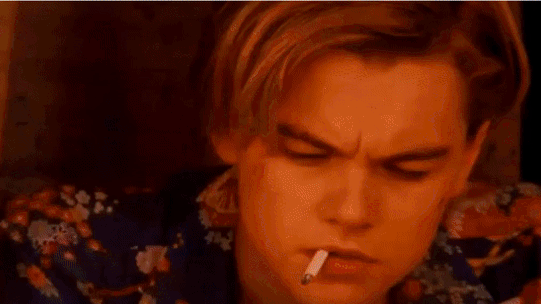
Fighting Fakes
China announced a ban on fake news, targeting deepfakes in particular.
What happened: The Cyberspace Administration of China issued new rules restricting online audio and video, especially content created using AI. Deepfakes and other such tech, the administration warned, may “disrupt social order and violate people’s interests, creating political risks and bringing a negative impact to national security and social stability.”
What could get you in trouble: The rules take effect January 1, 2020. They target both the creators of fake news and websites that host their content.
- The rules extend beyond explicitly malicious content. Any videos containing AI-generated characters, objects, or scenes must come with an easy-to-see disclaimer, including relatively benign fakes like those above, created by game developer Allan Xia using the Zao face-swapping app.
- The new restrictions extend China’s earlier efforts to nip digital fakery in the bud. In 2015, the National People’s Congress passed a law mandating three to seven years in prison for anyone caught spreading fake news.
- China’s laws also make it easy for the country to track deepfake creators. News and social media platforms operating in the country require users to register with their real names.
Behind the news: Computer-generated media are facing government scrutiny in the U.S. as well. California issued its own law barring doctored audio and video earlier this year. The law, however, does not name deepfakes or any specific technology. It targets only political content released within 60 days of an election, and it sunsets in 2023. U.S. legislators are considering federal restrictions on deepfakes, according to The Verge.
Why it matters: There’s a legitimate fear that deepfakes will be used for political purposes. To date, however, the technology’s biggest victims have been women whose bodies have been visualized without clothes or whose faces have been pasted onto pornographic scenes.
We’re thinking: It’s a good idea for governments to think ahead to potential problems caused by deepfakes in advance of broader calamities. However, efforts to crack down on them may turn into a high-tech game of whack-a-mole as falsified media becomes ever harder to spot.
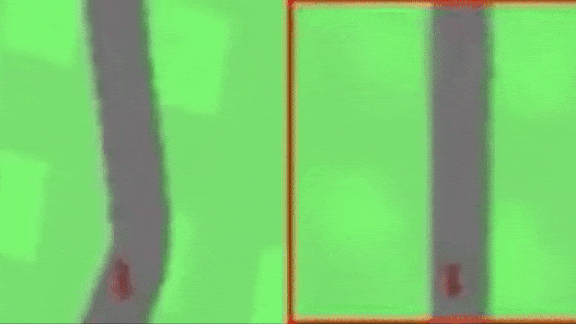
Seeing the World Blindfolded
In reinforcement learning, if researchers want an agent to have an internal representation of its environment, they’ll build and train a world model that it can refer to. New research shows that world models can emerge from standard training, rather than needing to be built separately.
What’s new: Google Brain researchers C. Daniel Freeman, Luke Metz, and David Ha enabled an agent to build a world model by blindfolding it as it learned to accomplish tasks. They call their approach observational dropout.
Key insight: Blocking an agent’s observations of the world at random moments forces it to generate its own internal representation to fill in the gaps. The agent learns this representation without being instructed to predict how the environment will change in response to its actions.
How it works: At every timestep, the agent acts on either its observation (framed in red in the video above) or its prediction of what it wasn’t able to observe (imagery not framed in red). The agent contains a controller that decides on the most rewarding action. To compute the potential reward of a given action, the agent includes an additional deep net trained using the RL algorithm REINFORCE.
- Observational dropout blocks the agent from observing the environment according to a user-defined probability. When this happens, the agent predicts an observation.
- If random blindfolding blocks several observations in a row, the agent uses its most recent prediction to generate the next one.
- This procedure over many iterations produces a sequence of observations and predictions. The agent learns from this sequence, and its ability to predict blocked observations is tantamount to a world model.
Results: Observational dropout solved the task known as Cartpole, in which the model must balance a pole upright on a rolling cart, even when its view of the world was blocked 90 percent of the time. In a more complex Car Racing task, in which a model must navigate a car around a track as fast as possible, the model performed almost equally well whether it was allowed to see its surroundings or blindfolded up to 60 percent of the time.
Why it matters: Modeling reality is often part art and part science. World models generated by observational dropout aren’t perfect representations, but they’re sufficient for some tasks. This work could lead to simple-but-effective world models of complex environments that are impractical to model completely.
We’re thinking: Technology being imperfect, observational dropout is a fact of life, not just a research technique. A self-driving car or auto-piloted airplane reliant on sensors that drop data points could create a catastrophe. This technique could make high-stakes RL models more robust.
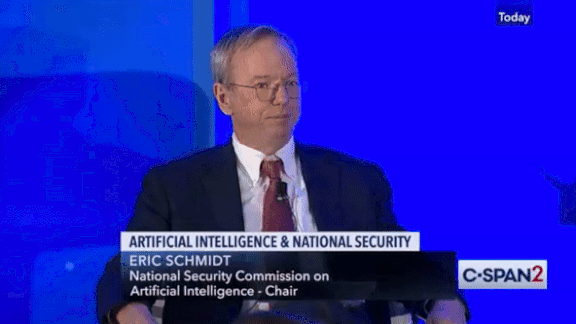
Transparency for Military AI
A U.S. federal judge ruled that the public must be able to see records from a government-chartered AI advisory group.
What’s new: The court decided that the National Security Commission on AI, which guides defense research into AI-powered warfighting technology, must respond to freedom-of-information requests.
What the suit says: The National Security Commission on AI was set up by Congress in late 2018, and the Electronic Privacy Information Center has been requesting its records under the Freedom of Information Act for almost as long. Finding its requests ignored repeatedly, EPIC took the commission to court in late September.
- Lawyers for the AI commission argued that their client was protected from freedom of information laws by exemptions for members of the president’s staff, some of whom sit on the commission.
- EPIC’s attorneys countered by citing a 1990 ruling that congressionally funded groups, such as the Defense Nuclear Facilities Safety Board, are considered federal agencies, and therefore obligated to share information with the public.
- The judge sided with EPIC, so the AI commission will have to respond to the nonprofit’s requests for copies of its meeting transcripts, working papers, studies, and agendas. EPIC also asked that the AI commission publish advance notice of its meetings in the federal register.
Behind the news: Former Google CEO Eric Schmidt (shown above) chairs the commission. Other members include a mix of private sector and government experts. It released an interim report of its recommendations on November 4.
Why it matters: EPIC contends that, without public oversight, the commission could steer the Defense Department towards invasive, biased, or unethical uses of AI.
We’re thinking: We look forward to learning more about what this group has been up to. Decisions about deploying AI as a weapon of war should not be made in a back room.