Dear friends,
The United States Federal Reserve Bank has signaled that it will continue to raise interest rates. As one consequence, the stock market is significantly down, particularly tech stocks, relative to the beginning of the year. What does this mean for AI? In this two-part series, I’d like to discuss what I think will happen — which may have implications on your AI projects — and what I think should happen. Unfortunately, these are different things.
The U.S. has enjoyed low interest rates over the past decade. Simplifying a bit, if r is the interest rate (if the interest rate is 2%, then r = 0.02), then one dollar T years in the future is worth 1/(1+r)^T as much as one dollar today. The larger r is, the less that future dollar is worth relative to its value today. If you’re familiar with the discount factor ɣ (Greek alphabet gamma) in reinforcement learning, you may notice that ɣ plays a similar role to 1/(1+r) and weights rewards T steps in the future by ɣ^T.
If interest rates were near zero, then one dollar in 10 years would be worth about the same as it is today. But if the interest rate were 5%, then a guaranteed promise of one dollar in 10 years would be worth only 61 cents today. What this means is that investors in the stock market are shifting to place a higher premium on cash today rather than cash in the future. This, in turn, will drive many CFOs, CEOs, and venture capital investors to discount investments that they deem likely to pay off only many years into the future.
This has important implications for AI. Over the past decade, many ambitious AI efforts sought to build fundamental technology that might pay off over many years. A few years ago, highly speculative bets on an experimental technology — from bold initiatives such as self-driving to more measured ones in which a team sought to execute a clear roadmap for a particular company — seemed like reasonable risks. Amid rising interest rates, such long-term bets look less attractive.
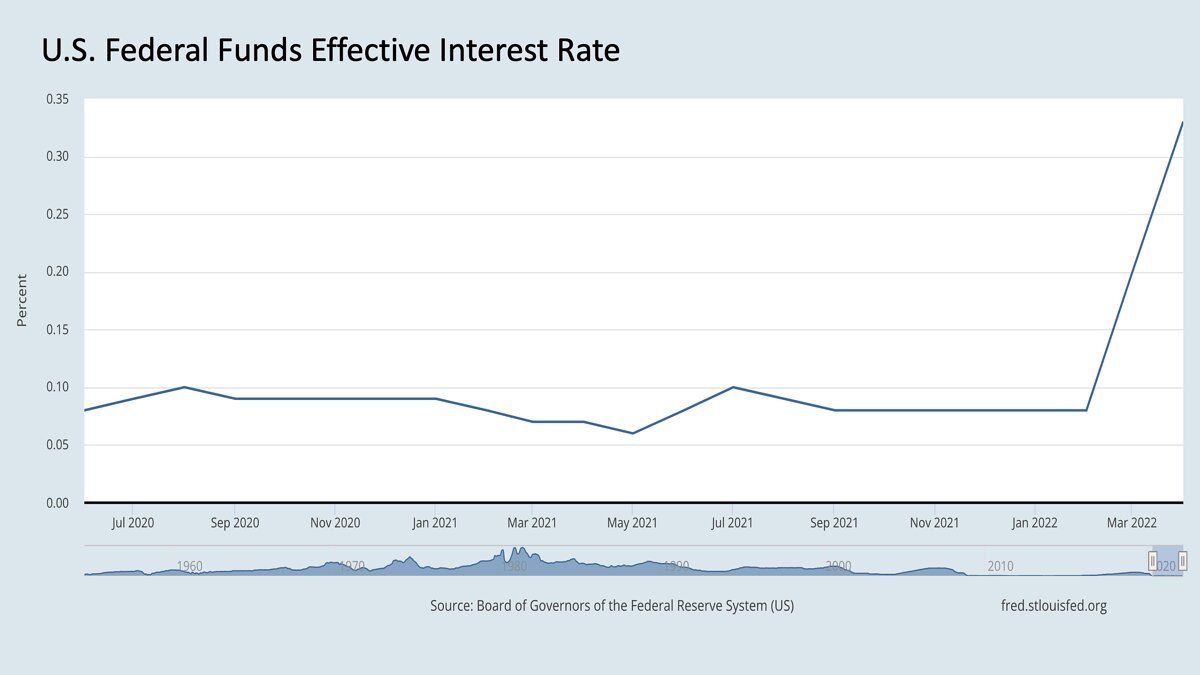
Many investors are wondering if the stock market’s 13-year bull run has come to an end, and if the next era will be very different. If interest rates continue to rise, then:
- Highly speculative, long-term technology development will have a harder time getting funded. I think this is unfortunate, since we will forgo many innovations. It's true that a tighter investment environment will reduce irresponsibly speculative, overhyped bets, but I believe that society will suffer a net loss.
- There will be more pressure for teams to demonstrate short-term business impact. For example, projects that are likely to generate financial returns on investment within a few years will look more attractive than long-term bets.
What this means for our community is that we should be ready for increased pressure to develop projects that demonstrate near-term, tangible value. For example, if you can explain how your AI system — for reading hospital records, inspecting parts, ensuring worker safety, or what have you — can save $1 million in two years, it will be easier to justify the $300,000 annual budget that you might be asking for. So if you’re looking for funding for a company or project, consider near-term impacts or financial justifications you can develop.
So far, I’ve laid out my prediction about what will happen, but what I think should happen is different. I believe this is still a good time to invest in long-term bets, because (i) the real interest rate (that is, the rate adjusted for inflation) is still very low, and (ii) the transformative value of AI is more financially powerful than interest rates, even as they compound at the moderate pace of 1/(1+r)^T. More about this in my next letter.
Keep learning!
Andrew
P.S. I’m grateful to Erik Brynjolfsson, a brilliant economist who has done seminal work on tech’s impact on the economy, for helping me think through the contents of this letter. Responsibility for any errors lies with me.
News
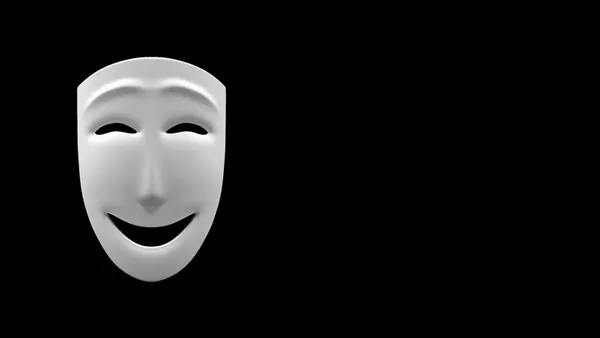
Actors Act Against AI
Performing artists are taking action to protect their earning power against scene-stealing avatars.
What’s new: Equity, a union of UK performing artists, launched a campaign to pressure the government to prohibit unauthorized use of a performer’s AI-generated likeness. The union published tips to help artists who work on AI projects exercise control over their performances and likenesses.
Protections for performers: Equity demands that the UK revise existing copyright laws and adopt guidelines enacted by other jurisdictions.
- The union is pressing lawmakers to revise the UK Copyright, Designs, and Patents Act, which gives performers rights with respect to their performances, to give them rights to computer-generated likenesses as well.
- Equity wants to give performers greater control over AI-generated representations they believe are negative or harmful, such as deepfakes that expound hateful rhetoric. Under existing law, such rights cover only audio.
- The union has called for lawmakers to implement the 2012 Beijing Treaty, which ensures that artists control reproduction and distribution of audiovisual performances; image rights provided by the British dependency of Guernsey that empower them to control their voice, mannerisms, and other distinctive attributes; and elements of the 2019 EU Copyright Directive that grant copyright protection to artists whose work is used to train or inspire replicas.
What performers think of AI: Equity conducted a survey of its members between November 2021 and January 2022. Among the 430 people who responded:
- 65 percent believed that AI poses a threat to employment opportunities. This figure jumped to 93 percent among audio artists.
- 24 percent had worked on projects that involved synthesizing a voice or avatar.
- 29 percent had recorded audio for a text-to-speech system.
- 93 percent supported prohibiting AI-generated replication of an artist’s performance without consent.
Why it matters: While synthetic images, video, and audio contribute to countless exciting works, they’re an obvious source of concern for artists who wish to preserve — never mind increase — their earning power. These developments also affect members of the audience, who may find that their favorite performers have less and less to do with the productions they nominally appear in.
We’re thinking: Using autotune to fix a wayward vocal performance doesn’t require the performer’s permission (though perhaps it should). The emerging generation of media production tools can generate performances entirely without the artist’s participation, further concentrating power in the hands of studios that own the technology. Defining the legal and ethical boundaries of generated media should help tip the balance toward performers, and it might lead to more fruitful creative collaborations between artists and machines.
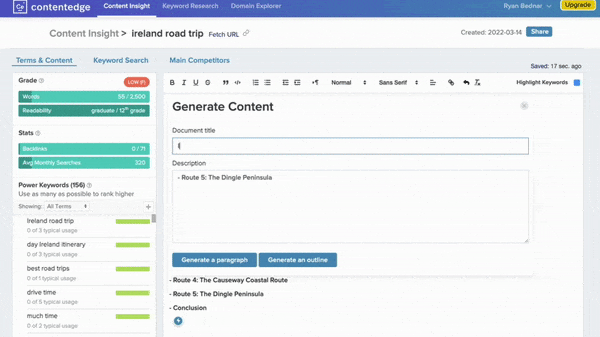
Winning The Google Game
AI startups are helping writers tailor articles that appear near the top of Google’s search results.
What’s new: At least 14 companies sell access to software that uses GPT-3, the language model from OpenAI, to generate headlines, product descriptions, blog posts, and video scripts, Wired reported.
How it works: The services enable people who have little experience or skill in writing to make content that’s optimized for web search engines.
- ContentEdge allows users to type or paste text into an editing window outfitted with GPT-3-powered tools for improving it. One tool suggests frequently searched-for keywords. Another generates paragraphs sprinkled with words found on web pages that are highly ranked by Google.
- Jasper provides templates for 50 common types of marketing posts including YouTube video scripts, LinkedIn bios, and Amazon product descriptions. It creates tailor-made prose given a company name, product description and selected tone of voice (such as “professional” or “Hulk Hogan”). A plagiarism checker flags instances when GPT-3 reproduces its training data verbatim.
- Copysmith focuses on generating cohesive language across marketing campaigns. Users can enter an outline or keywords into a template, and Copysmith will generate text and check it for plagiarism.
Machine privilege: Google’s guidelines state that it may take action against automatically generated content. However, a Google spokesperson told Wired that the company may take a more lenient approach toward generated text that has been designed to serve readers rather than manipulate search results.
Behind the news: Neural networks are reaching into video production, too. Given a script, Synthesia produces customized videos, rendered by a generative adversarial network, aimed at corporate customers. Given a finished video, Mumbai-based Videoverse tags key highlights and renders them into clips optimized for sharing on social media.
Why it matters: Producing text for online marketers is an early commercial use case for text-generation models. The tech gives people who don’t specialize in marketing a leg up and raises the bar for professional writers — assuming it produces consistently high-quality output. In any case, AI has found a lucrative place in advertising and marketing, helping to drive $370 billion in ad sales this year, according to the marketing agency GroupM.
We’re thinking: AI may write compelling marketing copy, but it’s still a long way from producing a great newsletter. Right?!
A MESSAGE FROM DEEPLEARNING.AI
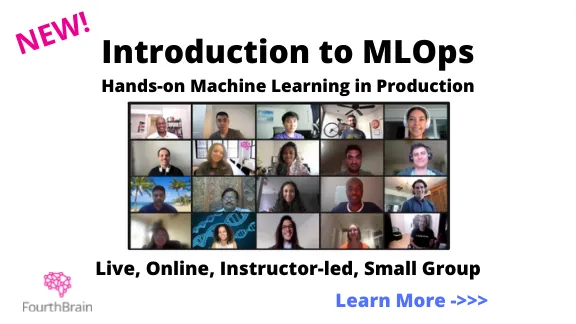
In FourthBrain’s new Introduction to MLOps course, you’ll walk through the AI product life cycle by building a minimum viable product using the latest tools. This live course meets on Tuesdays from July 5 to July 26, 2022, 5 p.m. to 8 p.m. Central European Summer Time. Join us! Learn more
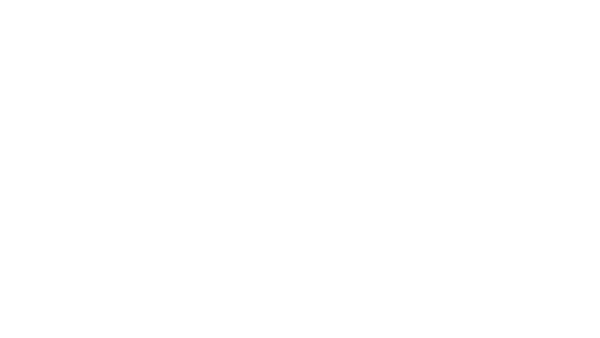
Deep Learning for Deep Discounts
With prices on the rise, an app analyzes user data to deliver cash back on retail purchases.
What’s new: Upside, a startup based in Washington, D.C., works with gas stations, grocery stores, and restaurants to offer personalized discounts to consumers, The Markup reported.
How it works: The app displays a map studded with offers, customized for each user, from 30,000 partners, most of them U.S. retail chains. A user who patronizes a partner pays full price, then uploads an image of the receipt. The app applies a discount to the user’s in-app balance, which can be transferred to a bank account — for a fee — or traded for digital gift cards.
- A machine learning system calculates discounts based on anonymized data including the user’s location, credit card number, and past purchases. External factors such as prices offered by competing establishments nearby also affect the discount.
- To pre-empt price wars among, say, gas stations clustered around a single intersection, Upside partners with only a single station in the cluster.
Behind the news: Founded in 2015, Upside says its services reach 30 million U.S. users. Lyft and Uber integrate it with their driving app to offset inflation-driven spikes in gas prices. Fuel-saving apps GasBuddy and Checkout51 offer Upside-powered promotions, and DoorDash and Instacart have offered Upside to their drivers.
Yes, but: Upside’s algorithmic approach to calculating discounts may leave some customers feeling left out.
- It’s more profitable for partners to offer bigger discounts to newer or less-frequent customers, Upside’s CEO wrote in a white paper. He advocated cutting discounts for users who are part of a partner’s loyalty program.
- A driver for a ride-sharing service told The Markup that an offer he had received from his employer through Upside — up to 25 cents cash back per gallon of gasoline — was misleading, and that he often received far less in cash back.
Why it matters: Many families, individuals, and employees are on the lookout for ways to cut their expenses, and they may consider surrendering personal information a fair trade. However, the terms of the deal should be transparent and easy to understand. It’s deceptive to offer discounts that don’t pan out or diminish without warning as a casual shopper becomes a steady customer.
We’re thinking: Offering discounts to attract users is an old tactic; think of Groupon and its countless competitors. But AI can tailor a deal to each individual user — a new approach that could make this strategy more effective, scalable, and sticky.
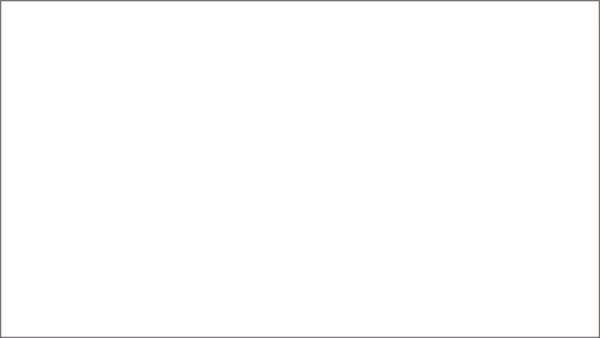
Right-Sizing Confidence
An object detector trained exclusively on urban images might mistake a moose for a pedestrian and express high confidence in its poor judgment. New work enables object detectors, and potentially other neural networks, to lower their confidence when they encounter unfamiliar inputs.
What’s new: Xuefeng Du and colleagues at University of Wisconsin-Madison proposed Virtual Outlier Synthesis (VOS), a training method that synthesizes representations of outliers to make an object detector more robust to unusual examples.
Key insight: Neural networks that perform classification (including object detectors) learn to divide high-dimensional space into regions that contain different classes of examples. Having populated a region with examples of a given class, they can include nearby empty areas in that region. Then, given an outlier, they’re likely to confidently label it with a class even if all familiar examples are far away. But a model can learn to recognize when low confidence is warranted by giving it synthetic points that fall into those empty areas and training it to distinguish between synthetic and actual points.
How it works: Given an image, an object detector generates two types of outputs: bounding boxes and classifications for those boxes. VOS adds a third: the model’s degree of certainty that the image is an outlier.
- For a batch of training images, the model proposed bounding boxes around regions that should contain objects.
- To synthesize an outlier, VOS looked at the representations at the network’s penultimate layer, then fit a Gaussian distribution to the representations of each class and sampled a representation with low probability. Conceptually, this is like drawing an ellipse around each class, then sampling a point close to the boundary of the ellipse. (They synthesized representations rather than images because it's easier to learn to generate relatively compact vectors than data-rich images.)
- To detect outliers, the authors added a logistic regression layer after the penultimate layer of the network. Given a representation of an image or a synthetic outlier, this layer learned to compute its likelihood of an outlier.
- The loss function consisted of a bounding box regression loss that taught the model to locate objects in an image, a bounding box classification loss that taught it to recognize the objects in the boxes, and an “uncertainty” loss that taught it to recognize certain objects (actually representations) as outliers.
Results: VOS maintained object detectors’ classification performance while reducing its false-positive rate. For instance, a ResNet-50 trained using VOS on a dataset that depicts persons, animals, vehicles, and indoor objects achieved object-detection performance of 88.66 percent AUC with a false-positive rate (FPR95) of 49.02 percent. By comparison, a ResNet-50 trained via a method that used a GAN to generate outlier images achieved slightly lower object-detection performance (83.67 percent AUC) and a much higher false-positive rate (60.93 percent FPR95).
Why it matters: It’s difficult to teach a neural network that the training dataset is just a subset of a diverse world. Moreover, the data distribution can drift between training and inference. VOS tackles the hard problem of encouraging object detectors to exercise doubt about unfamiliar objects without reducing their certainty with respect to familiar ones.
We’re thinking: The typical machine learning model learns about known knowns so it can recognize unknown knowns. While it’s a relief to have a neural network that identifies known unknowns, we look forward to one that can handle unknown unknowns.