Dear friends,
In March, I announced our Pie & AI series of meetups. Since then, we've held events in Seattle and London, two growing centers of AI talent.
It’s inspiring to see AI develop around the world and not just in Silicon Valley and Beijing. At both Pie & AI events, I met many people studying machine learning and deep learning, and working to apply them to all sorts of startups and big companies.
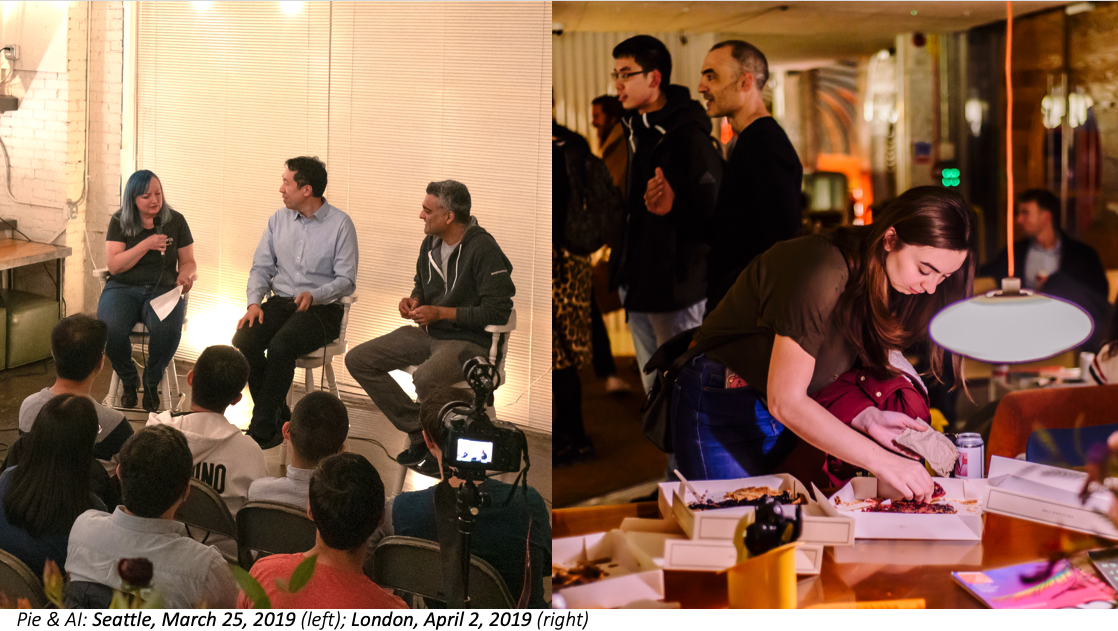
AI is still immature enough that many cities still have a shot at being an AI hub. Many of the most important uses of AI will happen outside the software industry, and they will need to be built for businesses in different cities. We need lots of hubs for AI to reach its full potential!
Keep learning,
Andrew
DeepLearning.ai Exclusive
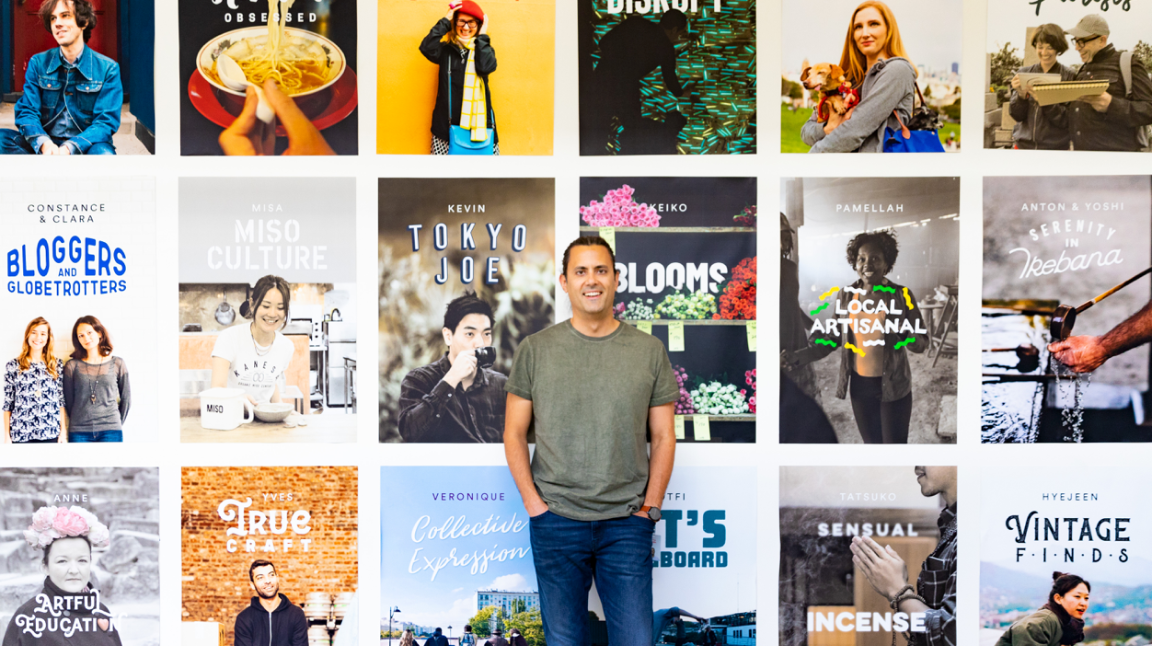
Working AI: Airbnb's Recommender
Data scientist Mihajlo Grbovic hammers out the algorithms that power Airbnb's recommendation engines. Learn what his day-to-day looks like and his advice for up-and-coming machine learning engineers. Read more
News
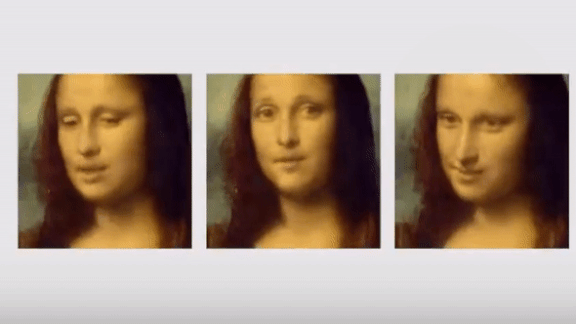
If Mona Lisa Could Talk
The art of video fakery leaped forward with a system that produces lifelike talking-head videos based on a single still portrait.
What’s new: Deepfake videos typically are created by feeding thousands of images of a person to a neural network. Egor Zakharov and his colleagues at Samsung and Skolkovo Institute of Science and Technology devised a method that needs only a handful — even just one — to generate good results.
How it works: The system consists of three networks, all of them pre-trained on thousands of talking-head videos of diverse speakers:
- The embedder maps video frames and facial landmarks to vectors that capture characteristics of a variety of faces.
- The generator maps input face landmarks from different portions of the same data set onto output frames. It uses both trained weights and drop-in weights derived from the embedder outputs.
- The discriminator and generator engage in an adversarial process to learn how to produce images of greater realism. In addition, the system minimizes the differences between original and synthesized images to preserve the speaker's identity.
- The resulting meta-learning setup learns to combine source and target landmarks in single image.
- The generator and discriminator are fine-tuned on source and target images to create output that's more true to the source.
Results: Test subjects were asked to rate the system’s output as fake or real. When the system had been fine-tuned on just one image, they were fooled a considerable amount of the time. When it had been fine-tuned on 32 photos, their guesses were random. See for yourself in this video.
Why it matters: The new technique drastically cuts the time, computation, and data needed to produce lifelike video avatars. The researchers suggest using it to create doppelgängers for gaming and videoconferencing — that is, using imagery of a single person both to generate the talking head and drive its behavior.
The fun stuff: The most dramatic results arose from animating faces from iconic paintings, including the Mona Lisa. How wonderful it would be to see her come to life and tell her story! Applications in entertainment and communications are as intriguing as the potential for abuse is worrisome.
We’re thinking: The ability to make convincing talking-head videos from a few snapshots, combined with voice cloning tech that generates realistic voices from short audio snippets, portends much mischief. We're counting on the AI community to redouble its effort to build countermeasures that spot fakes.
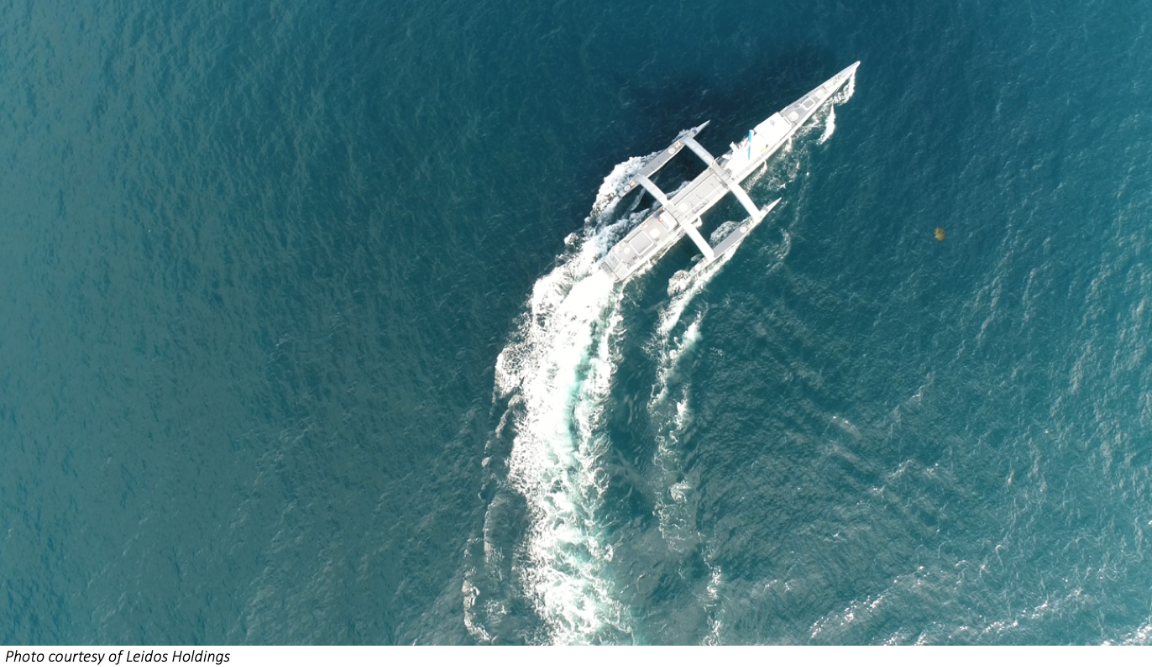
Robots on the High Seas
An autonomous U.S. Navy warship prototype is the first crew-less vessel to make an ocean crossing.
What’s happening: Sea Hunter last fall completed a round trip between its San Diego port and Pearl Harbor, Hawaii, as reported by Fortune. On the return voyage, the craft spent ten days at sea with no input from human navigators or mechanics.
How it works: Sea Hunter's main functions are to clear mines, track submarines, and securely relay communications. The vessel is 132 feet long and moves at around 37 miles per hour. Its software allows it not only to navigate across featureless expanses of water, but also to assess other craft and observe conventional protocols for ship-to-ship encounters.
The challenge: Navigating the open ocean autonomously involves a number of difficult, high-stakes tasks:
- Object recognition: Large ships are less maneuverable than cars. They need to know what’s coming from a distance, despite dark or stormy conditions.
- Motion planning: If it recognizes an object, the vessel must infer its intent to respond appropriately. The standard rules for avoiding collisions leave plenty of opportunity for potentially devastating errors.
- Localization: To plan a trans-ocean route, the craft must integrate data from GPS, weather forecasts, and depth maps to avoid storms and shallows.
- Control: Responding to motion algorithms involves integrating diverse mechanical systems while accounting for physics.
Why it matters: Built by defense contractor Leidos Holdings, the $59 million craft is an early step in the Navy’s plan to counter foreign sea power with autonomous ships. It’s also a bid to save money: Sea Hunter costs $20,000 a day to operate, compared to $700,000 a day for a destroyer deployed for similar tasks.
What’s next: The Navy plans to build a dozen or more autonomous ships, though it hasn’t settled on Leidos’ design. In April, the agency put out a call for combat-ready unmanned ships up to 300 feet long. Leidos plans to compete for the contract.
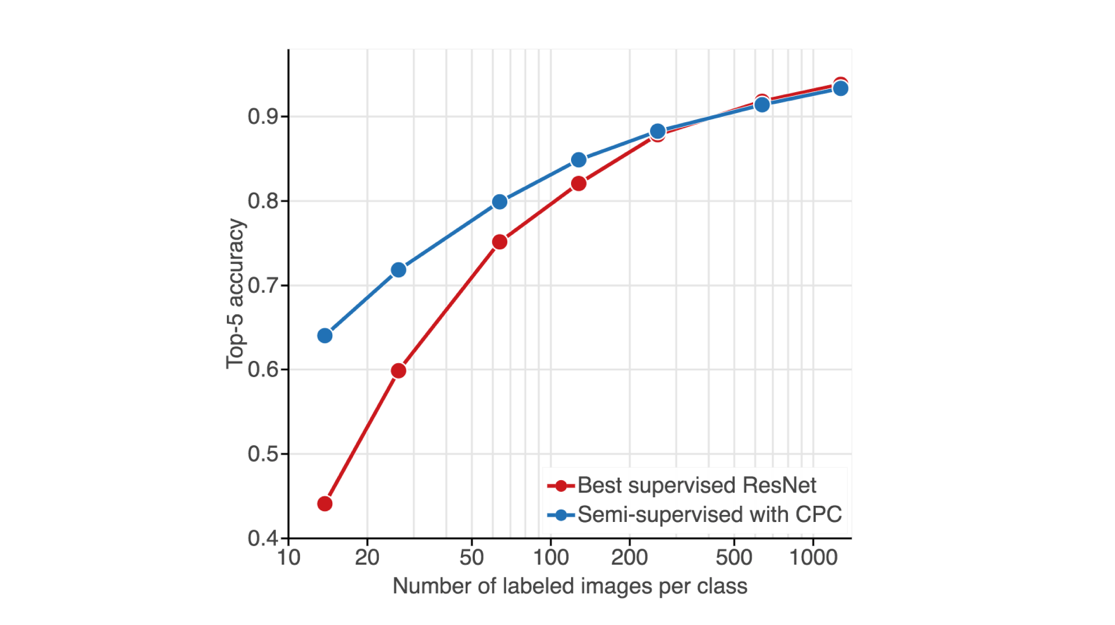
Small Data, Big Results
The power of deep learning is blunted in domains where labeled training data is scarce. But that may be changing, thanks to a new architecture that recognizes images with high accuracy based on few labeled samples.
What’s new: Researchers devised a network that, given a small number of labeled images, can learn enough from unlabeled images to outperform a fully trained AlexNet model. Their architecture achieves strong performance with as few as 13 images per class. And performance improves with additional labeled examples, unlike other semi-supervised approaches.
How it works: The trick is a technique called contrastive predictive coding, which learns to predict high-level features of an image from lower-level ones. Olivier J. Hénaff and his colleagues at DeepMind adapted earlier work with CPCs by using an unusually deep and wide residual network, using layer rather than batch normalization, and predicting both lower- and higher-level features. They also messed with image patches to remove low-level cues, forcing the network to focus on high-level structure. The resulting network learns to capture image features at various levels of detail.
Results: With a small number of labeled images, the CPC network beat the state-of-the-art performance of supervised models in ImageNet classification.
Why it matters: Small data is a frontier for deep learning. The new approach opens new territory in tasks like diagnosing rare diseases, spotting defects on a production line, and controlling robots, where few labeled examples may be available.
Takeaway: The Deep Mind team’s accomplishment reduces a barrier to applying deep learning in low-data domains. Watch for evolving small-data techniques to open up exciting new applications in the next few years.
A MESSAGE FROM DEEPLEARNING.AI
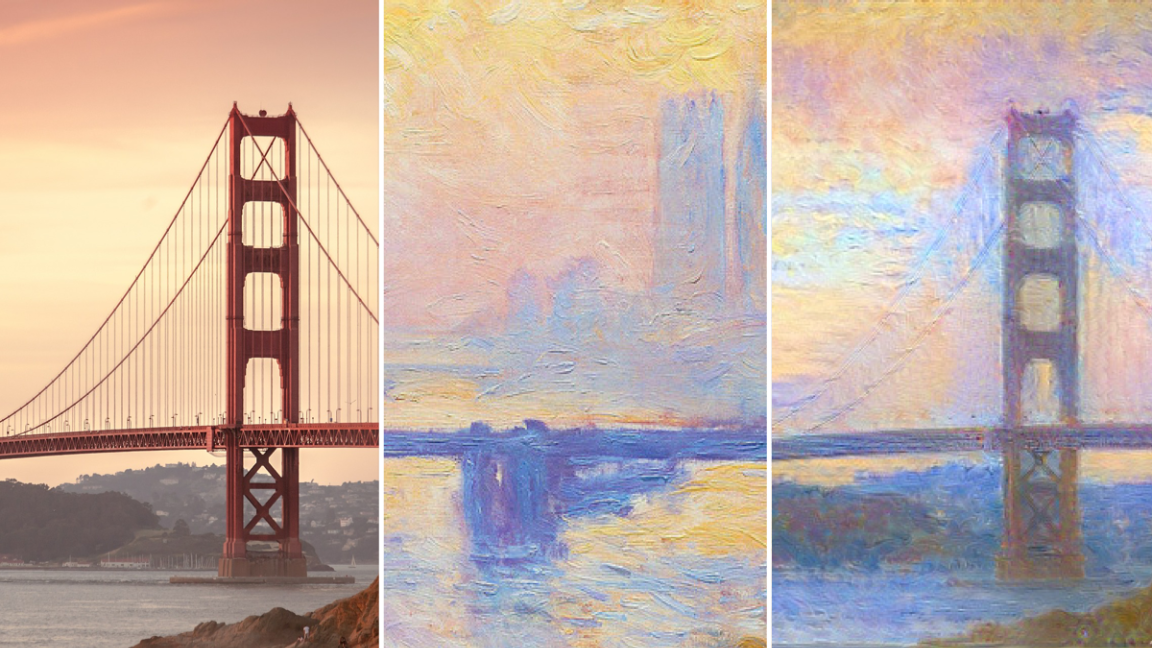
Be the next Picasso. Learn how to generate art pieces using neural style transfer in the Deep Learning Specialization. Enroll now
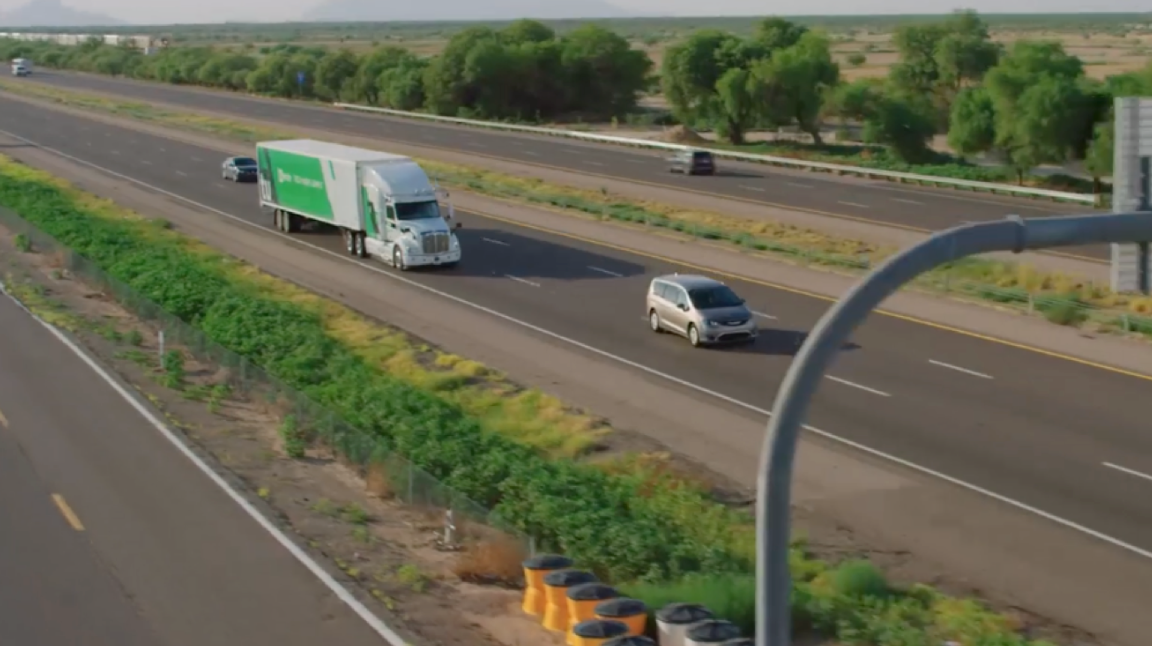
Autonomous Trucks Hit the Gas
An autonomous big rig is barreling down U.S. interstates in a high-profile trial, raising the prospect that driverless trucking will leapfrog self-driving cars.
What’s new: The U.S. Postal Service is using an autonomous truck to ferry mail between distribution centers in Phoenix and Dallas. A Peterbilt rig equipped with self-driving technology by TuSimple will make the 2,100-mile round trip through Arizona, New Mexico, and Texas five times over two weeks, moving for 22 hours at a stretch. Although the rig will drive itself, humans will sit behind the wheel and in the passenger seat.
Why it matters: The post office lost $3.9 billion in 2018, making 12 years of consecutive annual loss. It spends $4 billion a year on independent highway trucking, and a dearth of drivers is adding to the expense as truckers age out of the industry. Autonomous rigs could pick up the slack, saving money while keeping trucks on the road.
What they’re saying: Self-driving tech “could save hundreds of millions by eliminating human drivers and hours-of-service rules that keep them from driving around the clock.” — Bloomberg
Behind the news: The test comes as several companies developing self-driving taxis are reassessing their plans, including Cruise and Uber. Meanwhile, their trucking counterparts are stepping on the gas. TuSimple is carrying cargo discreetly for 12 customers in the U.S. and China. Swedish rival Einride recently began making driverless freight deliveries. And the Post Office is making its own moves.
Takeaway: Highway driving is simpler to automate than urban driving, where roads are denser and less predictable. On the other hand, autonomous trucks must comply with diverse regulations as they cross various jurisdictions in a single journey. Compliance issues are bound to tap the brakes until a national regulatory framework is in place.
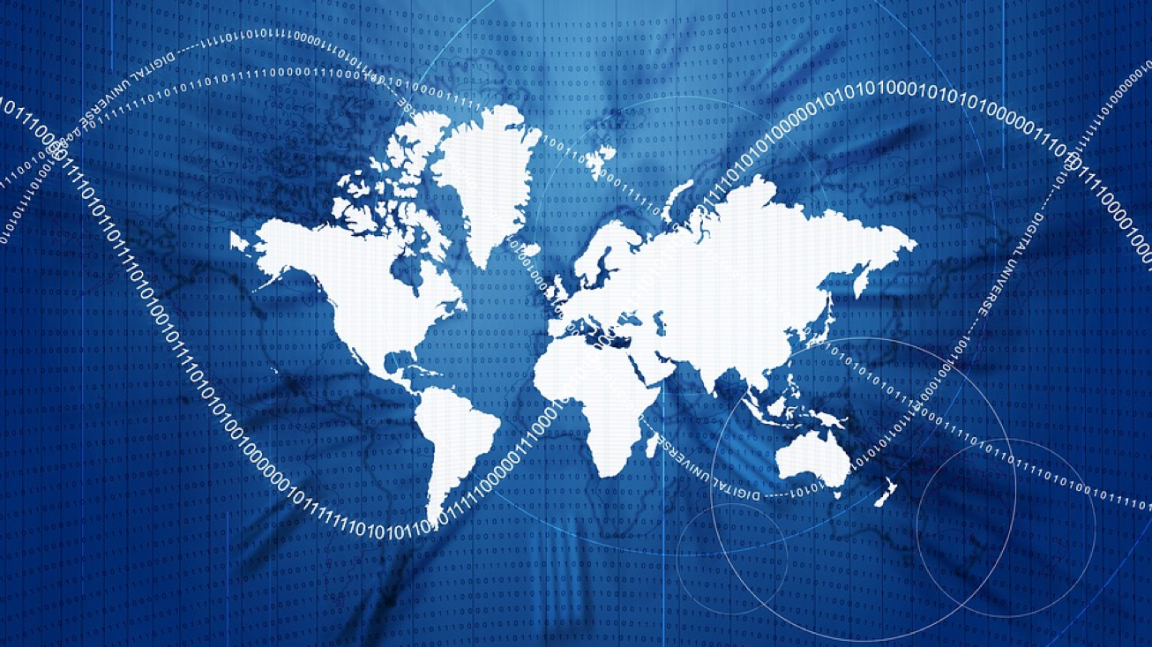
Toward A Smarter Globe
A leading international policy organization issued recommendations for artificial intelligence, adding momentum to international efforts to guide the technology in broadly beneficial directions.
What’s new: The Organization for Economic Cooperation and Development published guidelines to promote AI’s benefits while protecting human rights and democratic values. This is not just another think tank: The group represents 36 wealthy countries including the U.S. Six more countries, mostly in South America, signed on as well.
The recommendations: The OECD document offers five main principles. AI should:
- Benefit people and the planet.
- Respect the rule of law and human rights.
- Make its decisions understandable.
- Be secure and safe.
- Be developed and deployed in an accountable way.
It also outlines a set of governance policies. Countries developing AI should:
- Fund research and public data sets.
- Foster shared knowledge.
- Manage the transition from research to deployment.
- Prepare for shifts in the labor market.
- Cooperate with other nations on technical standards.
The hitch: The recommendations are non-binding. There’s no agreement about enforcement or mechanism for doing so. Moreover, there’s no plan for putting them into practice. The OECD is forming an AI Policy Observatory to focus on implementation.
Takeaway: The OECD’s work may look redundant, but it's an important step toward harnessing AI on a global scale. It signals widespread acknowledgement — by the world’s most developed countries, at least — that AI is on track to change every sector of the global economy. And it shows a will to grapple with that change sooner than later, and to make sure that fostering prosperous, equitable, and sustainable societies is part of the discussion. Whether and how governments will heed the call remains to be seen.