Dear friends,
I spent my birthday last week thinking about how AI can be used to address one of humanity's most pressing problems: climate change. The tech community can help with:
- Improved climate modeling
- Mitigation (such as a smart grid to reduce emissions)
- Adaptation (prediction of fires, floods, and storms)
- Restoration and geo-engineering
My Stanford group has launched an AI for Climate Change bootcamp. Stay tuned!
If you want to learn TensorFlow, check out the brand-new Course 2 of TensorFlow: From Basics to Mastery from deeplearning.ai.
Keep learning,
Andrew
News
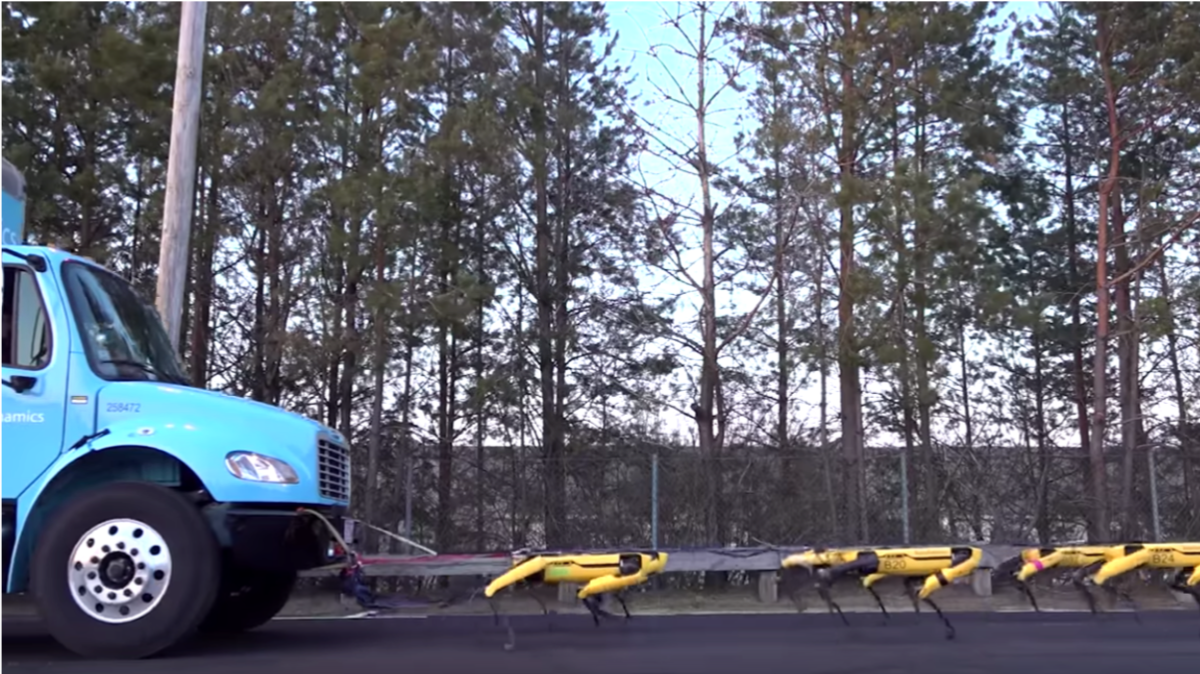
Attack of the Robot Dogs
Boston Dynamics' robot dog is straining at the leash. In a new promotional video, a pack of the mechanical canines pull a truck down a road like huskies mushing across the tundra. It's another jaw-dropping demo from one of the world's most ambitious robotics companies.
What’s new: SpotMini, previously seen climbing stairs, opening doors, and twerking to "Uptown Funk," is due to hit the market this year. No price has been announced.
How it works: Although SpotMini can operate autonomously, there's usually a human in the loop. The all-electric robot:
- Weighs 66 pounds
- Carries 31 pounds
- Features a grasping arm, 3D cameras, proprioceptive sensors, and a solid-state gyro
- Works up to 90 minutes per battery charge
Behind the news: Boston Dynamics’ previous owner, Alphabet, sold the company to SoftBank reportedly because it lacked commercial products. SpotMini will be its first.
Smart take: Robotic control is advancing by leaps and bounds, but there's still a long road ahead. Machines this cool could inspire machine learning engineers to take it to the next level.

Un-Redacting Mueller
Last week’s release of the redacted Mueller Report prompted calls to fill in the blanks using the latest models for language generation. A fun test case for state-of-the-art natural language processing—or irresponsible deepfakery tailor-made for an era of disinformation and paranoia?
A really, really bad idea: University of Washington computational linguistics professor Emily M. Bender unleashed a tweet storm explaining why machine learning engineers should resist the temptation. Using AI to “unredact” the report would:
- encourage unrealistic notions of what AI can achieve
- create confusion about the report's actual contents
- unduly influence discussion about the unredacted document, should it become available
- create controversy around any names inserted by the AI
What to do instead: For people interested in applying language generation in ways relevant to politics, Bender suggests working on rumor detection or “tools that might help users think twice before retweeting."
Takeaway: A language model knows only what’s in the data it was trained on. It can’t possibly know what the report's redactors hid from view, and it can't reason about it. Given the state of today's machine learning tech, a well informed human would make far better guesses about what’s missing.
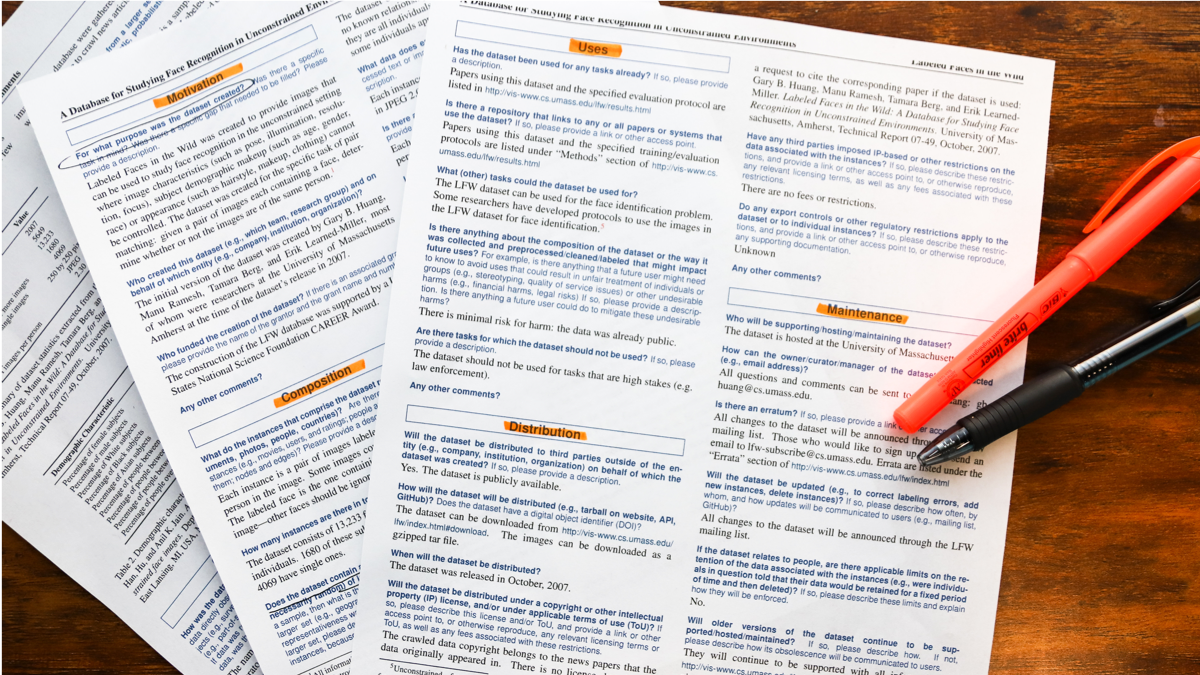
Transparency for Training Data
AI is only as good as the data it trains on, but there’s no easy way to assess training data’s quality and character. Researchers want to put that information into a standardized form.
What’s new: Timnit Gebru, Jamie Morgenstern, Briana Vecchione, and others propose a spec sheet to accompany AI resources. They call it "datasheets for datasets."
How it works: Anyone offering a data set, pre-trained model, or AI platform could fill out the proposed form describing:
- motivation for generating the data
- composition of the data set
- maintenance issues
- legal and ethical issues
- demographics and consent of any people involved
Why It matters: Data collected from the real world tends to embody real-world biases, leading AI to make biased predictions. And data sets that don’t represent real-world variety can lead to overfitting. A reliable description of what’s in the training data could help engineers avoid problems like these.
Bottom line: We live in a world of open APIs, pre-trained models, and off-the-shelf data sets. Users need to know what’s in them. Standardized spec sheets would give them a clearer view.
A MESSAGE FROM DEEPLEARNING.AI
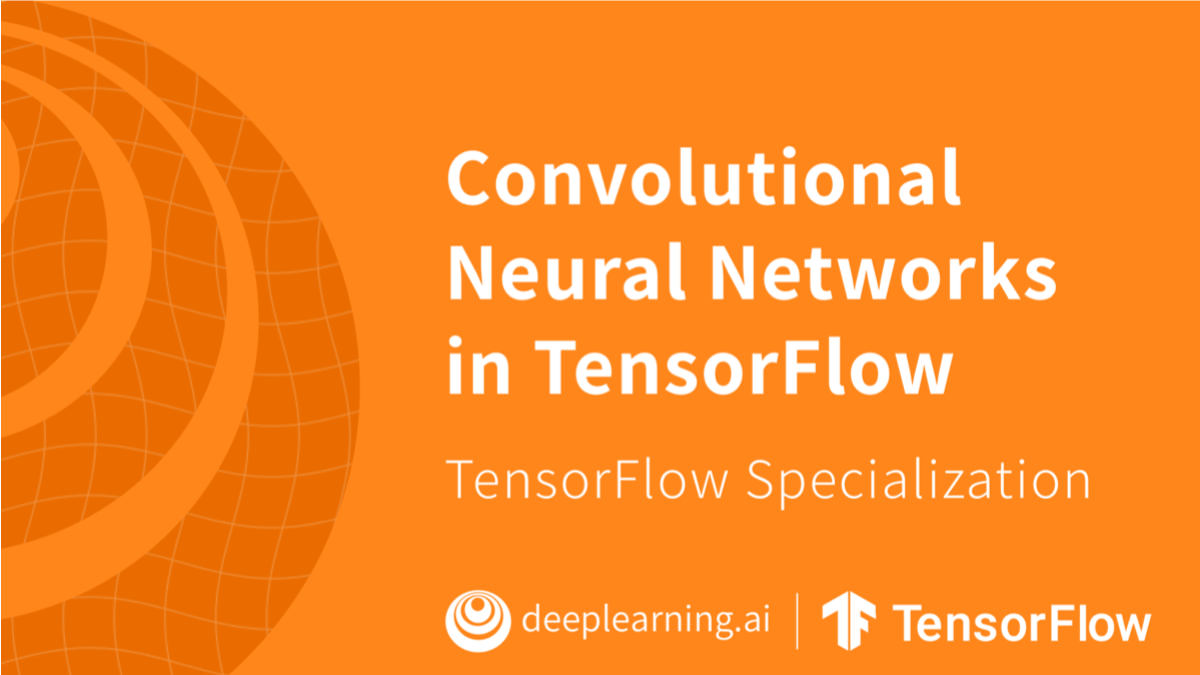
Get convolutional with TensorFlow! Check out Course 2 of deeplearning.ai's TensorFlow: From Basics to Mastery, newly available on Coursera.
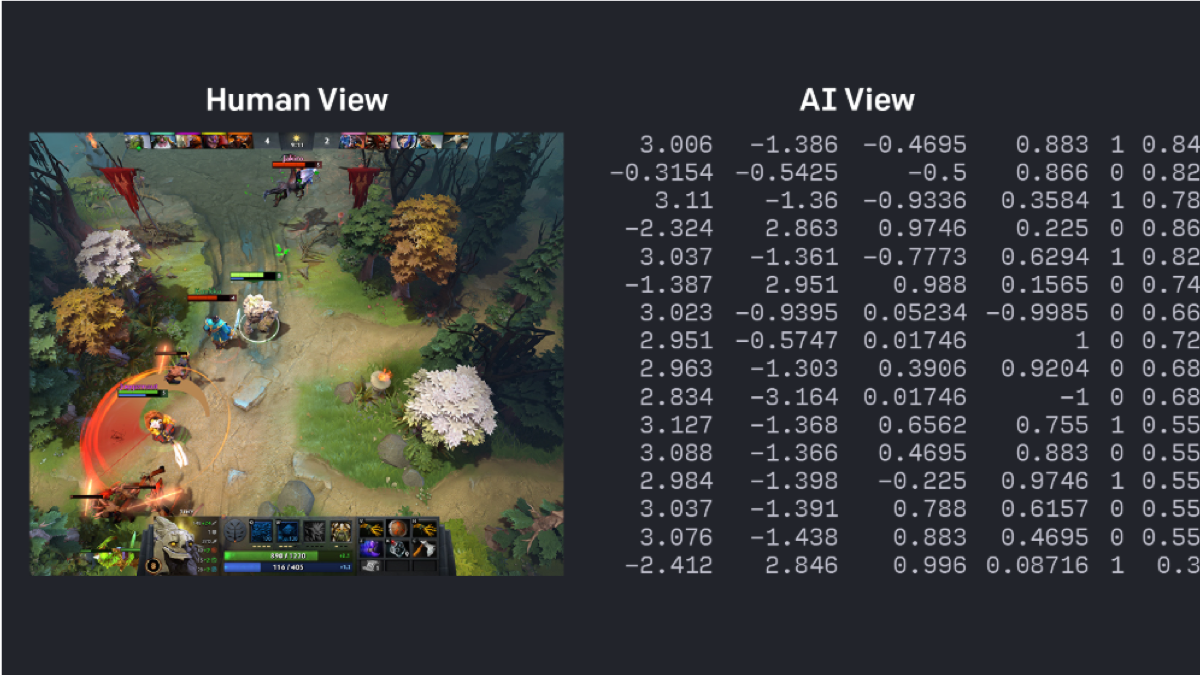
Our New DoTA-Playing Overlords
A software agent from OpenAI crushed human players of Defense of The Ancients 2, a multiplayer online game, in an Internet-wide free-for-all.
What’s new: More than 15,000 humans took on OpenAI Five over four days last week. The bot won 99.4 percent of 7,215 games.
How it works: OpenAI Five is a team of five neural networks. Each contains a single 1,024-unit LSTM layer that tracks the game and transmits actions through several independent action heads.
The challenge: DoTA2 is enormously complex. Games last around 45 minutes, requiring long-term strategy. The landscape is not entirely visible at all times, so players must infer the missing information. Roughly 1,000 possible actions are available at any moment. And it’s played by teams, so the five neural nets must employ teamwork.
What they’re saying: “No one was able to find the kinds of easy-to-execute exploits that human programmed game bots suffer from,” OpenAI CTO Greg Brockman told VentureBeat.
We’re thinking: OpenAI is one of several teams doing brilliant work in playing video games. We're thrilled by their accomplishment and momentum. But when and how will these algorithms translate to more practical applications?
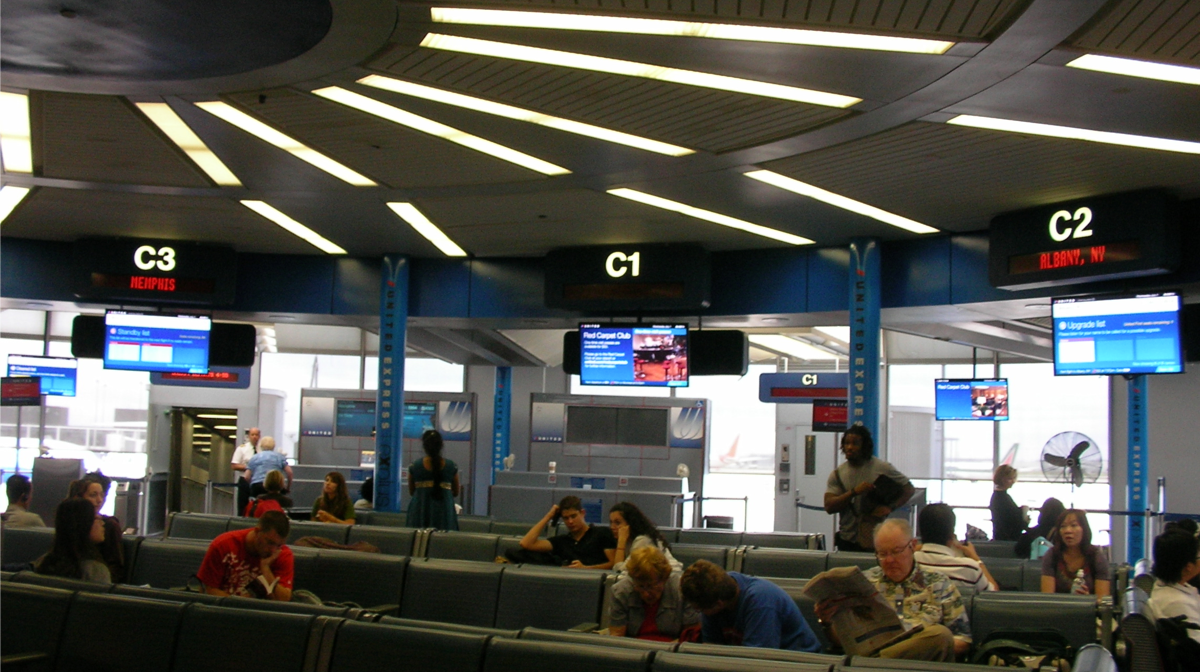
Smile as You Board
U.S. authorities, in a bid to stop aliens from overstaying their visas, aim to apply face recognition to nearly all travelers leaving the U.S.
What’s new: Within four years, U.S. Customs and Border Protection expects to scan the faces of 97 percent of air travelers leaving the U.S., according to a new report from the Dept. of Homeland Security.
How it works: Passengers approaching airport gates will be photographed and their faces will be compared to collected photos from passports, visas, and earlier border crossings. If the system finds a match, it creates an exit record.
Behind the news: The CBP plan is already well underway:
- The agency has been scanning faces in 15 airports since late last year.
- JetBlue rolled out face recognition at New York’s JFK International Airport in November.
- Other airports have committed to implementing the technology, the DHS says.
Why it matters: Face recognition is a flashpoint for discussions of ethics in AI. Microsoft refused to supply it to a California law enforcement agency over concern that built-in bias would work against women and minorities. Amazon employees have petitioned the company to stop selling similar technology to law enforcement agencies.
Bottom line: U.S. companies are wrestling with self-regulation in lieu of legal limits on how AI can be used. Their choices will have a huge impact on the industry and society at large.