Dear friends,
How much data do you need to collect for a new machine learning project? If you’re working in a domain you’re familiar with, you may have a sense based on experience or from the literature. But when you’re working on a novel application, it’s hard to tell. In this circumstance, I find it useful to ask not how much data to collect but how much time to spend collecting data.
For instance, I’ve worked on automatic speech recognition, so I have a sense of how much data is needed to build this kind of system: 100 hours for a rudimentary one, 1,000 hours for a basic one, 10,000 hours for a very good one, and perhaps 100,000-plus hours for an absolutely cutting-edge system. But if you were to give me a new application to work on, I might find it difficult to guess whether we need 10 or 10,000 examples.
When starting a project, it’s useful to flip the question around. Instead of asking,
How many days do we need to collect m training examples?
I ask,
How many training examples can we collect in d days?
Taking a data-centric approach to model development, let’s say it takes about two days to train a model and two days to perform error analysis and decide what additional data to collect (or how to tweak the model). How many days should you spend collecting data before training and error analysis? Allocating comparable amounts of time to each step seems reasonable, so I would advocate budgeting a couple of days — a week at most — for data collection. Then iterate through the loop.
![]() |
I’ve seen many teams spend far too much data collecting data before jumping into the model development loop. I’ve rarely seen a team spend too little time. If you don’t collect enough data the first time around, usually there’s time to collect more, and your efforts will be more focused because they’ll be guided by error analysis.
When I tell a team, “Let’s spend two days collecting data,” the time limit often spurs creativity and invention of scrappy ways to acquire or synthesize data. This is much better than spending two months collecting data only to realize that we weren’t correcting the right data (say, the microphone we used was too noisy, leading to high Bayes/irreducible error).
So, next time you face an unfamiliar machine learning problem, get into the model iteration loop as quickly as possible, and set a limited period of time for collecting data the first time around, at least. You’re likely to build a better model in less time.
Keep learning!
Andrew
P.S. Once I created an unnecessarily scramble when asked a team to make sure that data collection took no longer than two days. Because of a bad Zoom connection, they thought I said “today.” Now I've learned to hold up two fingers whenever I say “two days” on a video call.
News
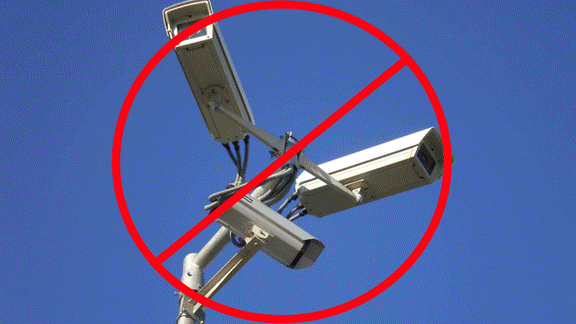
The Coming Crackdown
The European Union proposed sweeping restrictions on AI technologies and applications.
What’s new: The executive arm of the 27-nation EU published draft rules that aim to regulate, and in some cases ban, a range of AI systems. The proposal is the first to advance broad controls on the technology by a major international body.
What it says: The 100-plus page document divides AI systems into three tiers based on their level of risk. The definition of AI includes machine learning approaches, logic-based approaches including expert systems, and statistical methods.
- The rules would forbid systems deemed to pose an “unacceptable” risk. These include real-time face recognition, algorithms that manipulate people via subliminal cues, and those that evaluate a person’s trustworthiness based on behavior or identity.
- The “high risk” category includes systems that identify people; control traffic, water supplies and other infrastructure; govern hiring, firing, or doling out essential services; and support law enforcement. Such systems would have to demonstrate proof of safety, be trained using high-quality data, and come with detailed documentation. Chatbots and other generative systems would have to let users know they were interacting with a machine.
- For lower-risk applications, the proposal calls for voluntary codes of conduct around issues like environmental sustainability, accessibility for the disabled, and diversity among technology developers.
- Companies that violate the rules could pay fines of up to 6 percent of their annual revenue.
Yes, but: Some business-minded critics said these rules would hinder innovation. Meanwhile, human rights advocates said the draft leaves loopholes for applications that are nominally prohibited. For example, face recognition is prohibited only if it’s conducted in real time; it could still be used on video captured in the past.
Behind the news: Governments worldwide are moving to regulate AI. The U.S. Federal Trade Commission last week signaled its intent to take legal action against companies that make biased systems. A number of other countries including Australia, China, Great Britain, and India have enacted laws aimed at reining in big tech companies.
Why it matters: The EU’s AI proposal is the spiritual successor to its 2018 privacy law, the General Data Protection Regulation (GDPR). That law sparked a global trend as Brazil, China, India, and other countries proposed or enacted laws to protect user data. The new plan could have a similar impact.
We’re thinking: Despite its flaws, the GDPR drew a line in the sand and advanced the conversation about uses of personal data. While this new set of rules is bound to provoke criticism —some it valid, no doubt — we welcome moves to promote regulation around AI and look forward to a spirited, global discussion.
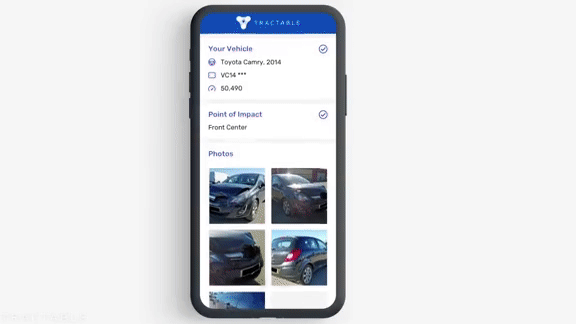
Wreck Recognition
Automobile insurers are increasingly turning to machine learning models to calculate the cost of car repairs.
What’s new: The pandemic has made it difficult for human assessors to visit vehicles damaged in crashes, so the insurance industry is embracing automation, Wired reported.
How it works: When drivers get into an accident, insurance companies direct them to download an app that guides them through documenting the effects. These systems are particularly good at assessing damage from minor collisions and determining when a car has been totaled.
- Such apps classify damage using a model trained on crash photos of a variety of makes and models. The app determines whether the damaged part needs to be inspected by a human. If not, it analyzes what needs to be fixed and estimates a repair cost using data from local mechanics and parts suppliers. Then a human adjustor reviews the model’s work.
- Tractable, which makes such software, says its system correctly estimates 25 percent of cases without human intervention.
- CCC Information Services, which makes an app called Smart Estimate, claims that adjusters who use its system are 30 percent more productive.
- Such models are particularly good at assessing minor damage and determining when a car has been totaled.
Yes, but: Several body shop owners said that automated estimates weren’t accurate and often failed to spot hard-to-see damage such as a misaligned frame. Bad estimates resulted in substandard repairs and delays as mechanics haggled with insurance companies for more money.
Why it matters: Smart damage-assessment apps can inspect vehicles far more quickly than a human who examines the damage first-hand. Accurate output helps insurance companies save money and drivers settle claims more quickly.
We’re thinking: Will self-driving cars that get into a fender bender use an app to assess the damage?
A MESSAGE FROM DEEPLEARNING.AI
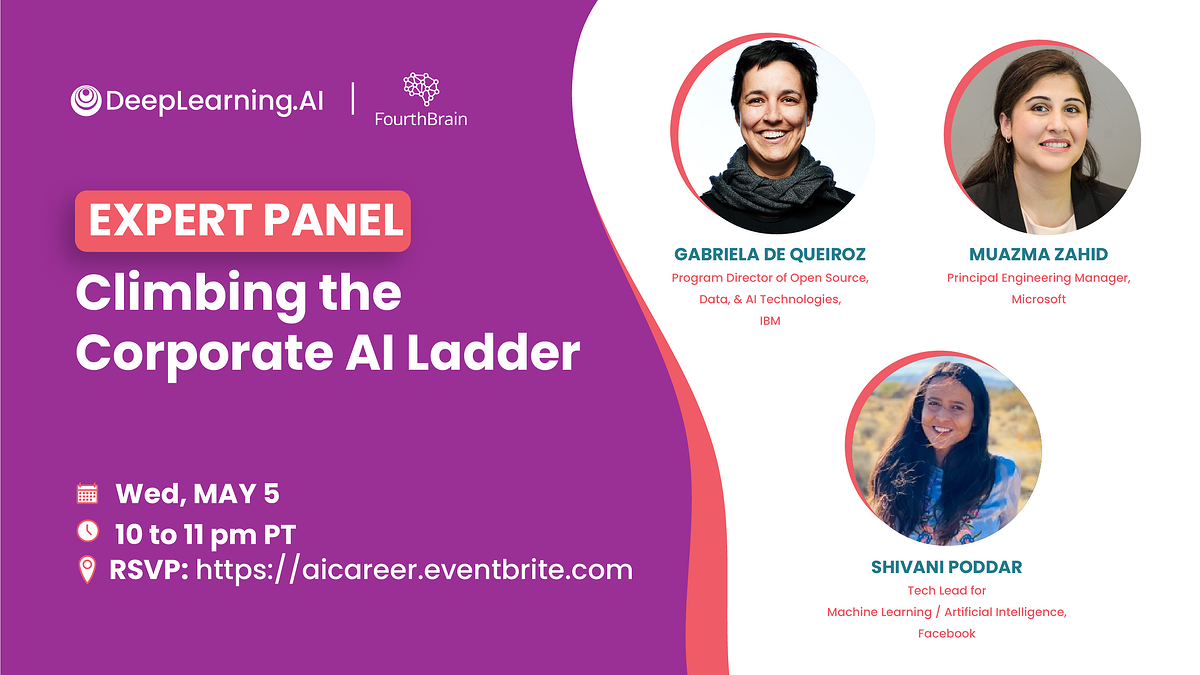
Join us for “Climbing the Corporate AI Ladder,” a live virtual event, on May 5, 2021. Speakers from Facebook, IBM, and Microsoft will show you how to level up your career. Co-hosted by DeepLearning.AI and FourthBrain.
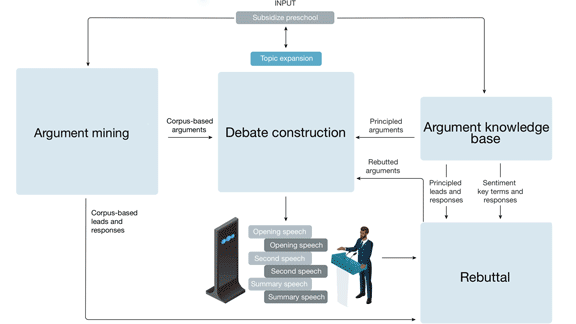
Up for Debate
IBM’s Watson question-answering system stunned the world in 2011 when it bested human champions of the TV trivia game show Jeopardy! Although the Watson brand has fallen on hard times, the company’s language-processing prowess continues to develop.
What’s new: Noam Slonim led a team at IBM to develop Project Debater, which is designed to compete in formal debates.
Key insight: A debate opens with four-minute opening statements by both sides followed by rounds of rebuttals and finally closing statements. To perform well, a debater must quickly prepare arguments supported by evidence, address competing arguments, and organize statements logically — a set of tasks too diverse for an end-to-end system. Instead, the team built a pipeline of independent components, each a complex system in its own right.
How it works: Project Debater receives a motion to argue in favor of or against. Then it’s off to the races finding facts, arguments, and counterarguments and stitching them together into speeches.
- The argument mining component searches the 400 million articles in LexisNexis for relevant opinions and extracts evidence that backs or refutes them. A model based on a gated recurrent unit (a type of recurrent neural network) in conjunction with an SVM classifies whether an opinion supports or opposes the motion.
- The argument knowledge base is a compendium of arguments, quotes, and analogies grouped into thematic classes. The system classifies the theme of the motion it’s arguing to find relevant arguments, both supporting and opposing. Claims are linked to counterclaims, so the system can rebut common opposing arguments and avoid concurring accidentally.
- A rebuttal module turns an opponent’s speech into text using Watson Speech to Text. It compares the opponent’s arguments with those discovered by the earlier components using a combination of models including LSTMs, hand-written rules, and logistic regression. It uses the most relevant argument to form a rebuttal.
- The debate construction component clusters arguments based on their theme. A rule-based system filters out similar arguments, picks the best paragraphs, and organizes them into a speech. Finally, a text-to-speech service synthesizes audio output.
Results: Project Debater is the first system of its kind, and no established benchmark exists to evaluate it. The researchers compared the quality (judged by humans on a scale of one to five) of the system’s opening statement with a speech on the same topic generated by a GPT-2 pretrained on a large text corpus and fine-tuned on speeches. Project Debater achieved an average score of 4.1, far outperforming the fine-tuned GPT-2’s score of 3.2.
Yes, but: Project Debater lost a 2019 competition with debate champion Harish Natarajan — albeit narrowly.
Why it matters: Building a system that can beat humans at competitive debate isn’t a multi-decade, multi-team project like winning at chess or Go, but it’s a substantial endeavor. So far, Project Debater has generated over 50 papers and spawned the subfields in claim detection and evidence detection.
We’re thinking: The AI community is embroiled in its own debates, including an annual event in Montreal. Maybe this system can participate next time around?
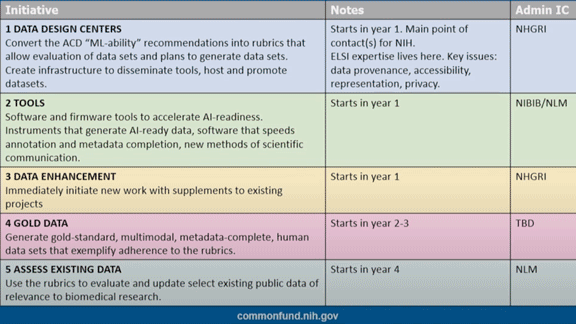
Boosting Biomedicine
The U.S. government aims to turbocharge biomedical AI research.
What’s new: The National Institutes of Health, which invests $41.7 billion annually in medical research, announced a program called Bridge to Artificial Intelligence (Bridge2AI) to promote machine learning in human biology and medicine.
Take it to the bridge: The program’s primary goal is to develop new datasets. It also aims to standardize data from different sources and develop automated tools to help create datasets and ensure that they adhere to FAIR principles, which aim to enable machines to use data with little human intervention. Bridge2AI will fund research into two areas:
- Creating datasets geared toward solving research “grand challenges” such as understanding how genes respond to disease, modeling physiological movement, and monitoring biological processes that lead from illness to health.
- Establishing an administration center for Bridge2AI projects. The center will focus on developing best practices to meet grand-challenge goals in areas like teamwork, ethics, standards, tool optimization, and workforce development.
- The NIH will begin accepting applications in June and will award funds the following spring.
Behind the news: U.S. government agencies bringing AI into mainstream healthcare.
- A recent study estimated that U.S. regulators have approved 222 AI-powered medical devices.
- The Food and Drug Administration released a plan to update its medical-device regulations for machine learning.
- The Centers for Disease Control and Prevention uses machine learning to forecast annual flu outbreaks. Last year it deployed a chatbot to help screen people for Covid-19 infections.
Why it matters: Bigger, better datasets specially designed for machine learning could help illuminate human biological processes and the diseases that disrupt them.
We’re thinking: AI for medicine has tremendous potential. Datasets designed specifically to help us realize that potential may be just what the doctor ordered.