Dear friends,
I have a two-year-old daughter, and am expecting my son to be born later this week. When I think about what we can do to build a brighter future for our children, the most important thing is to create a foundation for education. Because education is knowledge, and knowledge is human progress.
Today Coursera, which I co-founded almost nine years ago to transform lives through learning, became a publicly listed company.
I remember building the machine learning course that wound up being the first course on Coursera. There were many Friday nights when I met friends for dinner and then headed back to the office to record videos until 3 a.m. I felt privileged and humbled sitting in a room by myself speaking to a webcam, knowing I was playing a small role in helping thousands of learners.
Of course, Coursera quickly became much bigger than a professor and a webcam. I’m grateful to my cofounder Daphne Koller, my early team members, our university partners, instructors, investors, advisors, executives, board members, and 1,000-plus employees over the years. Special shout-out to the company’s CEO Jeff Maggioncalda, who treasures the education mission as much as I do.
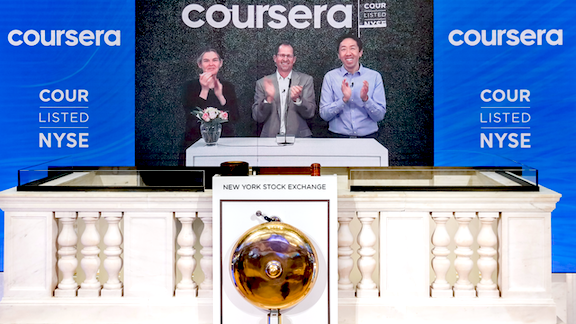
Most of all, I want to thank all the learners. Let's face it — learning is fun, but it can also be hard work. I remember once reading an article about the percentage of programmers who were self-taught. I couldn’t understand anything less than 100 percent, because I think all learners are self taught. Teachers can play a role, but ultimately it's up to learners to learn. So thank you for watching the online videos, doing homework, and spending your spare time to master these materials.
Coursera was launched on April 18, 2012 (the company and I share a birthday!). I hope we’ll continue to reach more learners, because everyone should be a lifelong learner, and everyone should have the opportunity to transform their life through learning.
The education mission is bigger than any person or single institution. If we can unlock the full potential in every person, we will move humanity forward.
(This letter is excerpted from a speech I made at Coursera’s IPO event earlier today.)
Keep learning!
Andrew
News
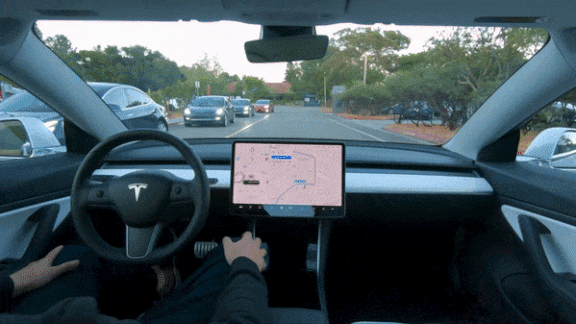
Tesla Safety Under Investigation
U.S. authorities are investigating Tesla’s self-driving technology.
What’s new: Federal regulators launched a probe of nearly two dozen accidents, some of them fatal, that involved Tesla vehicles equipped for self-driving, Reuters reported.
The inquiry: The National Highway Traffic Safety Administration is looking into 23 crashes of Tesla vehicles that occurred when the cars’ autonomous driving systems may have been engaged.
- The agency previously completed four investigations into Tesla crashes, most famously one from 2016 in which a Florida driver was killed when his car plowed into a big rig. Tesla’s technology was found to be partly to blame for that incident but not the other three.
- In separate investigations of the Florida incident and one in California two years later, the National Transportation Safety Board (a different federal oversight group) found Tesla’s system at fault.
- Tesla insisted its vehicles are safe. Data it collects from its fleet shows that cars under autonomous control experience fewer accidents per mile than those driven by humans, the company said. The company has not revealed whether Autopilot was engaged during the accidents under investigation.
Behind the news: Tesla has two self-driving modes.
- Autopilot, which comes standard on all new vehicles, controls the steering wheel, brakes, and accelerator. It’s meant to be used on highways with a center divider.
- Drivers can upgrade to what Tesla calls the Full Self-Driving option for $10,000. Despite the option’s name, last November, a Tesla lawyer disclosed to California regulators that the system should not be considered fully autonomous.
- Tesla advises drivers using either mode to keep their hands near the steering wheel and eyes on the road. However, the systems remain engaged even if drivers don’t follow these instructions, and videos on social media show drivers using Autopilot on roads that are not divided highways.
Why it matters: The new investigations are aimed at finding facts and will not directly result in new rules for Tesla or the self-driving industry at large. Still, the company’s reputation could take a battering, and hype about self-driving technology makes it harder for the AI community as a whole to gain trust and make progress.
We’re thinking: While it may be true that Tesla’s self-driving technology is safer on average than human drivers, it doesn’t fit the description “full self-driving.” While Tesla’s work to promote clean energy has had widespread positive impact, it’s time for the company to drop that branding and for car makers to provide clear, consistent information about their autonomous capabilities.
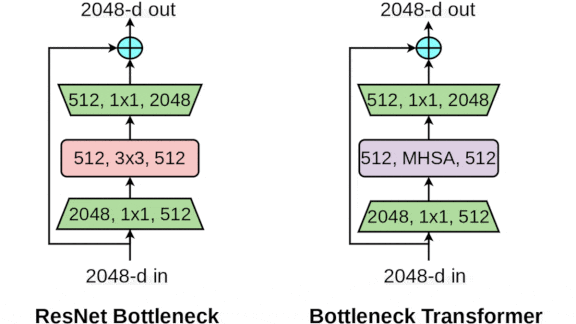
Vision Models Get Some Attention
Self-attention is a key element in state-of-the-art language models, but it struggles to process images because its memory requirement rises rapidly with the size of the input. New research addresses the issue with a simple twist on a convolutional neural network.
What’s new: Aravind Srinivas and colleagues at UC Berkeley and Google introduced BoTNet, a convolutional architecture that uses self-attention to improve average precision in object detection and segmentation.
Key insight: Self-attention and convolution have complementary strengths. Self-attention layers enable a model to find relationships between different areas of an image, while convolutional layers help the model to capture details. Self-attention layers work best when inputs are small, while convolutional layers can shrink input size. Combining the two offers the best of both worlds.
How it works: BoTNet-50 is a modified ResNet-50. The authors trained it for COCO’s object detection and segmentation tasks — that is, to draw bounding boxes around objects and determine what object each pixel belongs to — via Mask R-CNN, a method that details how to train and set up the network architecture for these tasks.
- Some ResNets use bottleneck blocks, which perform three layers of convolutions. The first layer reduces the input size, the second extracts representations, and the third converts its input back to the original size.
- BoTNeT adopts this structure, but in the last three blocks of the network, the authors replaced the second convolutional layer with a self-attention layer.
Results: BoTNet-50 beat a traditional ResNet-50 in both object detection and segmentation. Averaged over all objects in COCO, more than half of pixels that BoTNet associated with a given object matched the ground-truth labels 62.5 percent of the time, while the ResNet-50 achieved 59.6 percent. For a given object, more than half of BoTNet’s predicted bounding box overlapped with the ground-truth bounding box 65.3 percent of the time, compared to 62.5 percent for the ResNet-50.
Why it matters: Good ideas in language processing can benefit computer vision and vice versa. We’re thinking: Convolution is almost all you need.
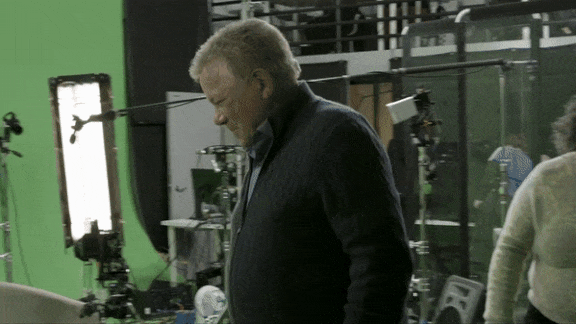
Star Trek: The Videobot Generation
A digital doppelgänger of Star Trek’s original star will let fans chat with him — possibly well beyond his lifetime.
What’s new: AI startup StoryFile built a lifelike videobot of actor William Shatner, best known for playing Captain James T. Kirk of the Starship Enterprise in the 1960’s-vintage Star Trek television series. The Shatbot is scheduled to go online in May.
How it works: The company honed its approach by building avatars of Holocaust survivors, a socially distanced interactive Santa Claus, and a platform that lets people talk with scientists about climate change.
- StoryFile recorded hours of Shatner, who recently turned 90, answering questions while volumetric cameras captured his image in three dimensions.
- The volumetric picture was shot in front of a green screen, enabling the team to isolate Shatner’s image and display it against a living-room setting.
- The team trained a proprietary language model called Conversa to associate questions and answers. When a user asks a question, the model will find a closely related answer and serves it up.
Behind the news: Shatner imagines that the system might enable his descendents to interact with him after his death. Other companies are also using chatbots to help people feel connected to departed loved ones.
- Last December, Microsoft was awarded a patent for a bot that re-creates a specific person by processing their text messages, audio, videos, and other digital remains.
- The creator of Replika, a chatbot for people experiencing loneliness, trained the original system using old texts from a friend who had died in a car accident.
Why it matters: Technological replicas of human beings are a long-standing science fiction trope, and few stories have shaped our vision of the future as profoundly as Star Trek. A lifelike avatar of William Shatner is a fitting — and fun — way to celebrate that legacy.
We’re thinking: We support free Enterprise.
A MESSAGE FROM DEEPLEARNING.AI
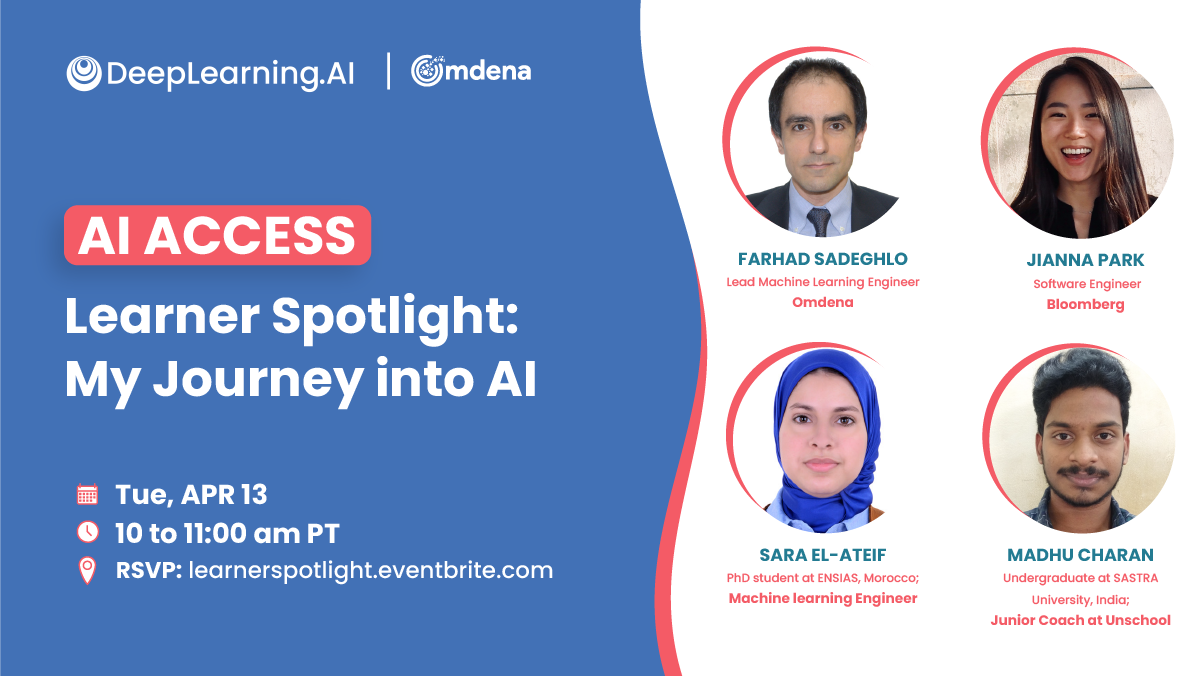
How do I navigate my journey into AI? Join us for a virtual panel of machine learning practitioners from different backgrounds as they share first-hand experiences in building a career. Presented in partnership with Omdena on April 13, 2021, at 10 a.m. Pacific Time. Register now
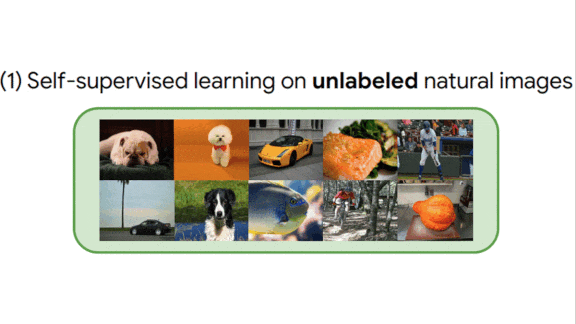
Same Patient, Different Views
When you lack labeled training data, pretraining a model on unlabeled data can compensate. New research pretrained a model three times to boost performance on a medical imaging task.
What’s new: Shekoofeh Azizi and colleagues at Google developed Multiple-Instance Contrastive Learning (MICLe), a training step that uses different perspectives of the same patient to enhance unsupervised pretraining.
Key insight: Presented with similar images, a model trained via contrastive learning produces representations that are nearby in vector space. Training via contrastive learning on images of the same patient taken from various angles can produce similar representations of an illness regardless of the camera’s viewpoint.
How it works: The authors started with a ResNet-50 (4x) pretrained on ImageNet. They added contrastive pretraining steps and fine-tuning to diagnose 26 skin conditions from acne to melanoma. The training data was a private set of 454,295 images that included multiple shots of the same patients.
- To refine the general representations learned from ImageNet for medical images, the authors pretrained the model according to SimCLR, an earlier contrastive learning technique. The model regarded augmented versions of the same parent image as similar and augmented versions of different images as dissimilar.
- To sharpen the representations for changes in viewpoint, lighting, and other variables, they further pretrained the model on multiple shots of 12,306 patients. In this step — called MICLe — the model regarded randomly cropped images of the same patient as similar and randomly cropped images of different patients as dissimilar.
- To focus the representations for classifying skin conditions, they fine-tuned the model on the images used in the previous step.
Results: The authors compared the performance of identical ResNet-50s pretrained and fine-tuned with and without MICLe. The authors’ method boosted the model’s accuracy by 1.18 percent to 68.81 percent, versus 67.63 percent without it.
Why it matters: A model intended to diagnose skin conditions no matter where they appear on the body may not have enough data to gain that skill through typical supervised learning methods. This work shows that the same learning can be accomplished using relatively little data through judicious unsupervised pretraining and contrastive losses.
We’re thinking: The combination of SimCLR and MICLe is a study in contrasts.
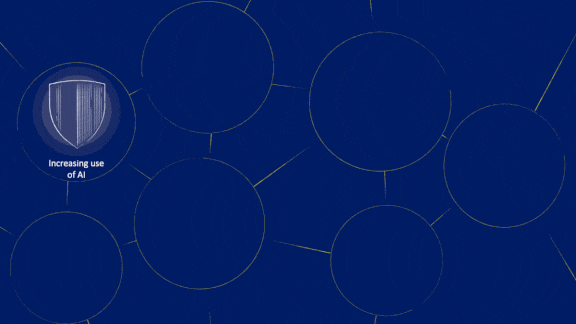
On Her Majesty’s Secret Algorithm
The UK’s electronic surveillance agency published its plan to use AI.
What’s new: Government Communications Headquarters (GCHQ) outlined its intention to use machine learning to combat security threats, human trafficking, and disinformation — and to do so ethically — in a new report.
What it says: GCHQ said its AI will augment, rather than supplant, human analysts. Moreover, the agency will strive to use AI with privacy, fairness, transparency, and accountability by emphasizing ethics training and thoroughly reviewing all systems. Such systems will:
- Analyze data on large computer networks to prevent cyber attacks, identify malicious software, and trace them back to their origins.
- Intercept sexually explicit imagery featuring minors and messages from sexual predators.
- Combat drug smuggling and human trafficking by analyzing financial transactions and mapping connections between the individuals behind them.
- Counter misinformation using models that detect deepfakes, assist with checking facts, and track both content farms that pump out fake news and botnets that spread it.
Behind the news: While intelligence agencies rarely detail their AI efforts, several examples have come to light.
- German law enforcement agencies use AI-generated images of minors to trap online predators.
- The U.S. National Reconnaissance Office is developing a system to guide surveillance satellites.
- The U.S. National Security Agency is training models to audit regulatory compliance by other intelligence agencies as they search for international criminals and look for warning of emerging crises.
Why it matters: The GCHQ plan emphasizes the utility of AI systems in securing nations and fighting crime — and highlights the need to ensure that sound ethical principles are built into their design and use.
We’re thinking: GPT-007 prefers its data shaken, not perturbed.
A MESSAGE FROM FOURTHBRAIN
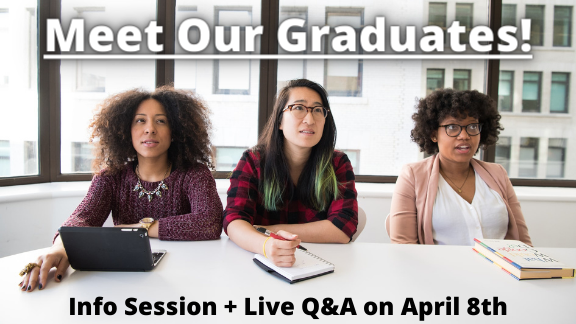
Become a machine learning engineer in only 16 weeks with FourthBrain! Meet our grads at a free info session on April 8, 2021. Ask them questions and learn more about the program. Register now