Dear friends,
Happy New Year! As we enter 2021, I want to share with you three wishes I have for AI in the upcoming year. I hope we can:
- Narrow the gap between proofs-of-concept and production. While building good models is important, many organizations now realize that much more needs to be done to put them into practical use, from data management to deployment and monitoring. In 2021, I hope we will get much better at understanding the full cycle of machine learning projects, at building MLOps tools to support this work, and at systematically building, productionizing, and maintaining AI models.
- Strengthen the AI community with shared values. As a community, part of our success has come from welcoming with open arms anyone who wants to join us. But over the past decade, we’ve grown from thousands to millions across the globe, and this has led to more opportunities for misunderstanding and misalignment. It is more important than ever to establish a shared set of values, so we can support each other in doing good. Let’s make sure the AI community doesn’t splinter into different factions like the political sphere in some countries. We need to put more energy into understanding each other, have vigorous — yet civil — debates, and hopefully still come together as one community.
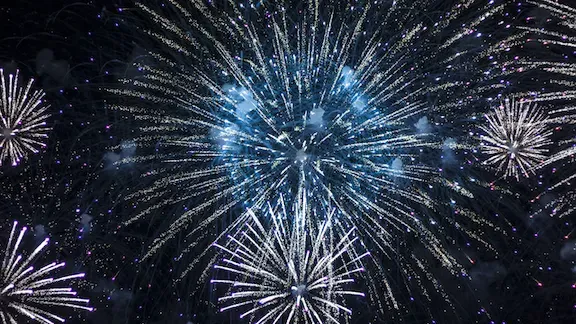
- Ensure that the outcomes of our work are fair and just. The issues of bias and fairness in AI have been widely discussed. Much difficult and important work remains to be done in those areas, and we must not relent. Meanwhile, AI’s contribution to wealth inequality has received less attention. Many tech businesses are winner-take-most businesses. What is the fifth most valuable web search engine? Or the fifth most valuable social media company? As tech infiltrates every industry from agriculture to zymurgy, it’s spreading these winner-take-most dynamics. Are we creating a world where the wealth is concentrated in a small handful of companies in every industry? How can we ensure that the massive wealth we help to generate is shared fairly?
I have great optimism for AI in 2021, and for the role you will play in it. I look forward to wrestling with these and other challenging problems with you.
Keep learning!
Andrew
Onward to 2021
The technology in our hands has the power to deliver vital services, grease the wheels of life and work, bring joy and delight, and create wealth that uplifts all humanity. Yet with it comes responsibility to distribute its benefits fairly and contain its unwanted impacts. How will we navigate these priorities in the coming year? Leaders of the AI community discuss their hopes in this special issue of The Batch.
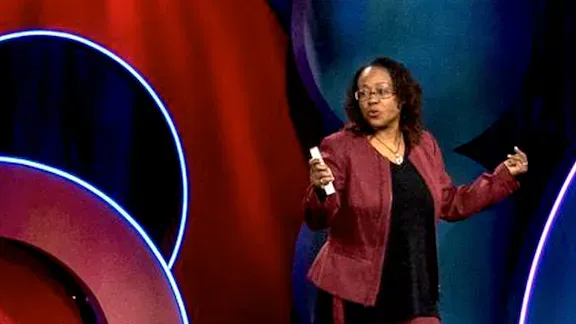
Ayanna Howard: Training in Ethical AI
As AI engineers, we have tools to design and build any technology-based solution we can dream of. But many AI developers don’t consider it their responsibility to address potential negative consequences as a part of this work. As a result, we continue to hear about inequities in the delivery of medical care, access to life-changing educational opportunities, financial assistance to people of meager means, and many other critical needs.
In the coming year, I hope the AI community can reach a broad consensus on how to build ethical AI.
The key, I believe, is training AI engineers to attend more fully to the potential consequences of their work. Typically, we’ll design a cool algorithm that matches faces in a database or generates chatbot conversations, and hand it off. Then we move on to the next project, oblivious to the fact that police departments are using our system to match mugshots to pencil sketches, or hate groups are using our chatbot to spread fear and lies.
This is not how things work in other areas of engineering. If you’re a civil engineer and you want to build a bridge, you need to model the entire scenario. You don’t model a generic bridge, but a particular bridge that crosses a particular river in a particular town. You consider all the conditions that come with it, including cars, people, bicycles, strollers, and trains that might cross it, so you can design the right bridge given the circumstances.
Similarly, we need to think about our work within the context of where it will be deployed and take responsibility for potential harms it may cause, just like we take responsibility for identifying and fixing the bugs in our code.
Training AI engineers with this mindset can start by bringing real-world examples into the training environment, to show how the abstract concepts we learn play out in reality. In a course about word embeddings, for instance, we can look closely at their role in, say, hate speech on social media and how such messages bear on people of a particular gender, religion, or political affiliation — people just like us.
And this training is not just for students. Practicing doctors and nurses are required to get continuing education credits to continue practicing. Why not in AI? Employers can make sure their developers get continuing education in ethical AI as a condition of ongoing employment.
This may seem like a big change, but it could happen very quickly. Consider the response to Covid-19: Educational institutions and companies alike immediately implemented work-from-home policies that previously they had considered impossible. And one of the nice things about technology is that when the top players change, everyone else follows to avoid losing competitive advantage. All it takes is for a few leaders to set a new direction, and the entire field will shift.
Ayanna Howard directs the Human-Automation Systems Lab and chairs Interactive Computing at Georgia Institute of Technology.
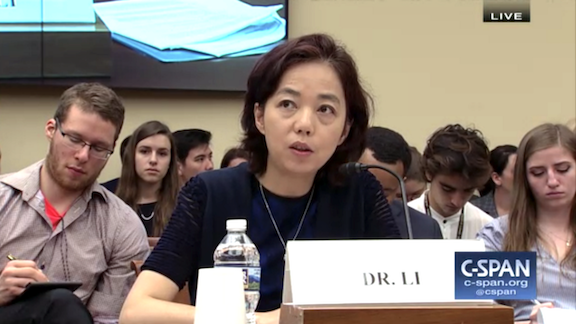
Fei-Fei Li: Invigorating the U.S. AI Ecosystem
The United States has been a leader in science and technology for decades, and all nations have benefitted from its innovations. But U.S. leadership in AI is not guaranteed. Should the country slip as a center of AI innovation and entrepreneurship, its contributions would be curtailed and the technology less likely to embody democratic values. I hope that 2021 will see a firm commitment from the U.S. federal government to support innovation in AI.
The U.S. has excelled in science and technology largely because its ecosystem for innovation leverages contributions from academia, government, and industry. However, the emergence of AI has tipped the balance toward industry, largely because the three most important resources for AI research and development — computing power, data, and talent — are concentrated in a small number of companies. For instance, to train the large-scale language model GPT-3, OpenAI in partnership with Microsoft may have consumed compute resources worth $5 million to $10 million, according to one analysis. No U.S. university has ready access to this scale of computation.
Equally critical for advancing AI are large amounts of data. The richest troves of data today are locked behind the walls of large companies. Lack of adequate compute and data handicaps academic researchers and accelerates the brain drain of top AI talent from academia to private companies.
The year 2020 brought renewed federal support for universities and colleges. But more needs to be done. At the Stanford Institute for Human-Centered Artificial Intelligence (HAI), which I co-direct with John Etchemendy, we have proposed a National Research Cloud. This initiative would devote $1 billion to $10 billion per year over 10 years to recharge the partnership between academia, government, and industry. It would give U.S. academic researchers the compute and data they need to stay on the cutting edge, which in turn would attract and retain new crops of faculty and students, potentially reversing the current exodus of researchers from academia to industry.
The fruits of this effort would be substantial. For instance, I’ve spent many years working on ambient AI sensors for healthcare delivery. These devices could help seniors who need chronic disease management by enabling caregivers to remotely track treatments and results, potentially saving hundreds of thousands of lives annually in the U.S. Such technology has no borders: The innovation created at Stanford could help aging societies worldwide. Renewed ferment in AI research also could bring innovations to mitigate climate change, develop life-saving drugs, optimize food and water supplies, and improve operations within the government itself.
We’re encouraged by the progress we’ve already seen toward the National Research Cloud. The U.S. Congress is considering bipartisan legislation that would establish a task force to study this goal. Meanwhile, agencies including the National Science Foundation and National Institutes of Health have issued calls for proposals for AI projects that such an initiative would support.
AI is a tool, and a profoundly powerful one. But every tool is a double-edged sword, and the ways it’s applied inevitably reflect the values of its designers, developers, and implementers. Many challenges remain to ensure that AI is safe and fair, respects values fundamental to democratic societies, protects individual privacy, and benefits a wide swath of humanity. Invigorating the healthy public ecosystem of AI research is a critical part of this effort.
Fei-Fei Li is the Sequoia Professor of Computer Science and Denning Co-Director of the Institute for Human-Centered Artificial Intelligence at Stanford. She is an elected member of the National Academy of Engineering and National Academy of Medicine.
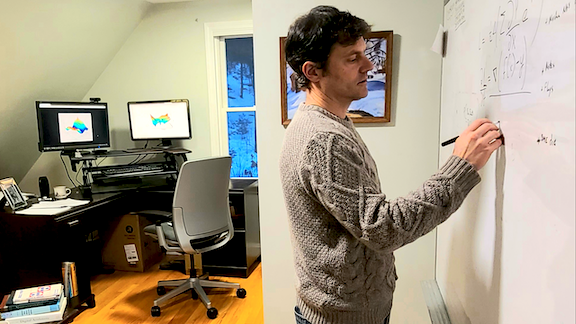
Matthew Mattina: Life-Saving Models in Your Pocket
Look at the tip of a standard #2 pencil. Now, imagine performing over one trillion multiplication operations in the area of that pencil tip every second. This can be accomplished using today’s 7nm semiconductor technology. Combining this massive compute capability with deep neural networks in small, low-cost, battery-powered devices will help us address challenges from Covid-19 to Alzheimer’s disease.
The neural networks behind stand-out systems like AlphaGo, Alexa, GPT-3, and AlphaFold require this kind of computational power to do their magic. Normally they run on data-center servers, GPUs, and massive power supplies. But soon they’ll run on devices that consume less power than a single LED bulb on a strand of holiday lights.
A new class of machine learning called TinyML is bringing these big, math-heavy neural networks to sensors, wearables, and phones. Neural networks rely heavily on multiplication, and emerging hardware implements multiplication using low-precision numbers (8 bits or fewer). This enables chip designers to build many more multipliers in a much smaller area and power envelope compared to the usual 32-bit, single-precision, floating-point multipliers. Research has shown that, in many real-world cases, using low-precision numbers inside neural networks has little to no impact on accuracy. This approach is poised to deliver ultra-efficient neural network inferencing wherever it’s needed most.
Let me give one example. In addressing the Covid-19 pandemic, a major bottleneck developed around testing and identifying infected patients. Recent research suggests that a collection of neural networks trained on thousands of “forced cough” audio clips may be able to detect whether the cougher has the illness, even when the individual is asymptomatic. The neural networks used are computationally expensive, requiring trillions of multiplication operations per second. TinyML could run such cough-analyzing neural networks.
My hope for AI in 2021 is that sophisticated healthcare applications enabled by large neural networks running on small devices will usher in a new era of personalized healthcare that improves the lives of billions of people.
Matthew Mattina leads the Machine Learning Research Lab at Arm as a distinguished engineer and senior director.
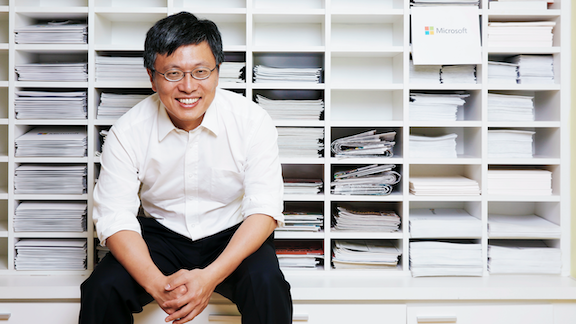
Harry Shum: Assisted Artistry
In 2021, I envision that the AI community will create more tools to unleash human creativity. AI will help people across the globe to communicate and express emotions and moods in their own unique ways.
We have created machines that excel at logical tasks, capable of calculating at a scale and speed that far exceed human abilities. This accomplishment is evident in the recent successes of lunar probes, which have gone to the moon and returned with material for study. In our everyday lives, we use tools such as Microsoft Word and Excel to boost our productivity. However, there are some tasks at which humans continue to reign supreme — especially in the arts.
A human brain has a logical side, or left brain, which is complemented by the creative and imaginative right brain. This creative side sparks many of the daily interactions that have allowed our species to flourish. We communicate with each other using language, conveying abstract concepts and expressing emotions. We also express ourselves artistically, creating music, art, dance, and design that hold meaning.
Recent progress in AI, especially with deep learning techniques like generative adversarial networks and language models like GPT-3, has made it possible to synthesize realistic images and plausible texts almost out of nothing. XiaoIce.ai, a spin-out from Microsoft where I chair the board of directors, provides a chatbot that has shown human-like performance in generating poems, paintings and music. For example, XiaoIce helped WeChat users to write more poems in a week than all the poems previously created in the history of China!
Aspiring practitioners of painting, music, poetry, or dance, to name a few of many art forms, must train in their disciplines for years. It is said that one needs to practice 10,000 hours to reach perfection. Tools like Xiaolce can reduce that investment substantially, helping anyone to create more sophisticated creative and imaginative expressions.
I look forward to seeing more AI creation tools in the coming year to help people express their artistic ideas and inspirations. AI has already shown that it can help humans to be more productive. Now let’s turn our attention to helping people to unlock their creativity.
Harry Shum is chairman of xiaoice.ai and an adjunct professor at Tsinghua University.
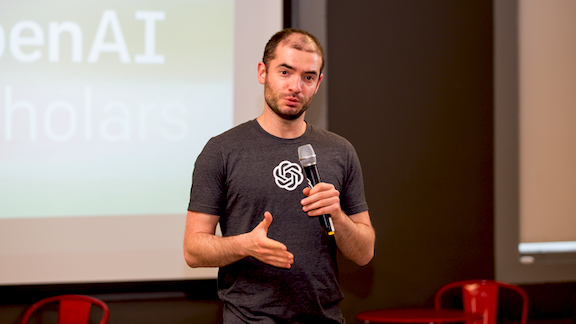
Ilya Sutskever: Fusion of Language and Vision
The past year was the first in which general-purpose models became economically useful. GPT-3, in particular, demonstrated that large language models have surprising linguistic competence and the ability to perform a wide variety of useful tasks. I expect our models to continue to become more competent, so much so that the best models of 2021 will make the best models of 2020 look dull and simple-minded by comparison. This, in turn, will unlock applications that are difficult to imagine today.
In 2021, language models will start to become aware of the visual world. Text alone can express a great deal of information about the world, but it is incomplete, because we live in a visual world as well. The next generation of models will be capable of editing and generating images in response to text input, and hopefully they’ll understand text better because of the many images they’ve seen.
This ability to process text and images together should make models smarter. Humans are exposed to not only what they read but also what they see and hear. If you can expose models to data similar to those absorbed by humans, they should learn concepts in a way that’s more similar to humans. This is an aspiration — it has yet to be proven — but I’m hopeful that we’ll see something like it in 2021.
And as we make these models smarter, we’ll also make them safer. GPT-3 is broadly competent, but it’s not as reliable as we’d like it to be. We want to give the model a task and get back output that doesn’t need to be checked or edited. At OpenAI, we’ve developed a new method called reinforcement learning from human feedback. It allows human judges to use reinforcement to guide the behavior of a model in ways we want, so we can amplify desirable behaviors and inhibit undesirable behaviors.
GPT-3 and systems like it passively absorb information. They take the data at face value and internalize its correlations, which is a problem any time the training dataset contains examples of behaviors that we don’t want our models to imitate. When using reinforcement learning from human feedback, we compel the language model to exhibit a great variety of behaviors, and human judges provide feedback on whether a given behavior was desirable or undesirable. We’ve found that language models can learn very quickly from such feedback, allowing us to shape their behaviors quickly and precisely using a relatively modest number of human interactions.
By exposing language models to both text and images, and by training them through interactions with a broad set of human judges, we see a path to models that are more powerful but also more trustworthy, and therefore become more useful to a greater number of people. That path offers exciting prospects in the coming year.
Ilya Sutskever is a co-founder of OpenAI, where he is chief scientist.