Dear friends,
Every year for the past decade, I flew to Singapore or Hong Kong to celebrate my mother’s birthday with her on December 22. This year, for the first time, we did it via Zoom. Despite the distance, I was warmed that my family could gather from the U.S., Singapore, Honk Kong, and New Zealand and sing a poorly synchronized “Happy Birthday To You.”
I wish I could also be on a Zoom call with each of you to personally wish you happy holidays and an even happier new year!
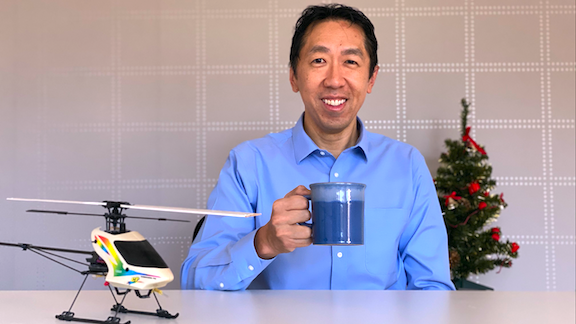
Over the holidays, I often think through the list of important people in my life, recall what they’ve done for me or others, and quietly acknowledge my gratitude to them. This makes me feel more connected to them. Perhaps you’ll find it valuable to think about this, too, during the socially distanced holiday that many of us will have: Who are the important people in your life, and what reasons might you have to be grateful to them?
Whether in-person or online, I hope you’ll find ways to nurture your most important relationships over this holiday season.
Keep learning!
Andrew
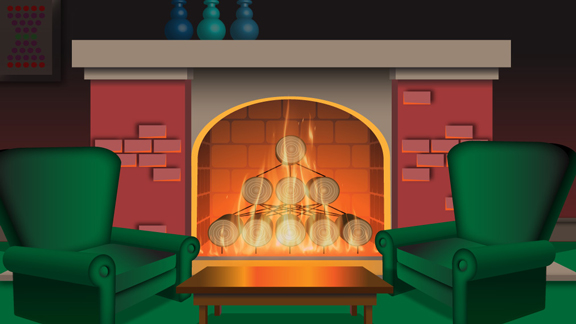
A Look Back at 2020
The past year is one for the history books by any measure. A new, highly infectious bug knocked the wheels off of life-as-usual, while social rifts threatened to eclipse our common interests. Machine learning engineers jumped into the fray, devising tools for Covid-19 diagnosis and treatment, building models to recognize hate speech and disinformation, and highlighting biases throughout the AI community. And there’s a lighter side: Work-from-home tools that exchange pajamas for a business suit, wise-cracking language models, and fascinating experiments in AI-assisted art and performance. Please join us as we survey the year gone by in all its hardship and glory.
News
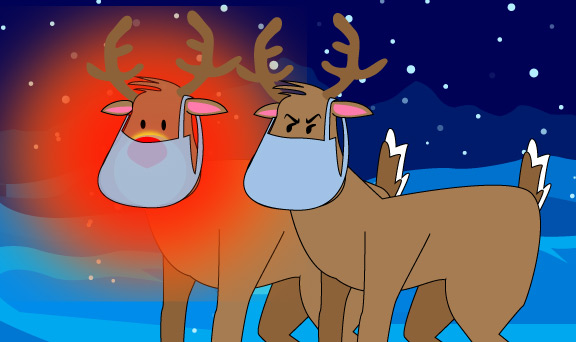
Coping With Covid
AI accelerated the search for a coronavirus vaccine, detected Covid-19 cases, and otherwise softened the pandemic’s blow.
What happened: Machine learning researchers worldwide scrambled to harness the technology against the coronavirus. Among many misfires, they racked up important successes in detection, inoculation, other areas.
Driving the story: The pandemic began with high hopes for AI-driven solutions among researchers and officials. But an April metastudy sounded a cautious note, finding that 145 models surveyed were poorly documented, yielded overly optimistic results, and were likely to be biased. Researchers persisted, ultimately delivering vaccines in record time. Outside the lab, deep learning teams tried to keep people safer and more connected.
- BlueDot, which analyzes news reports for significant events, detected the nascent pandemic several days ahead of the global health monitors and sent an early warning to its customers.
- The cities of Paris and Cannes evaluated compliance with masking regulations using computer vision in transit stations, buses, and markets. The government of Togo trained a model to identify regions of extreme poverty in satellite imagery. It used the output to guide distribution of relief funds to those most in need.
- Chatbots provided the locked-down and lonely with synthetic friends to chat and flirt with. For people working from home, videoconferencing companies trained models to filter background noises and virtually transform pajamas into business attire.
- A collaboration among many institutions in China developed a model that detects Covid-19 in CT scans with better than 90 percent accuracy. The model has been deployed in seven countries and the code has been downloaded 3 million times so far.
- Moderna, a U.S. biotech company whose vaccine was approved by the U.S. Food and Drug Administration in December, used machine learning to optimize mRNA sequences for conversion into molecules that could be tested.
Behind the news: AI may yet play an important role in treating Covid-19. The nonprofit Covid Moonshot project used a semisupervised deep learning platform to filter 14,000 candidate antiviral drugs. The system validated four compounds that are expected to advance to animal trials.
Where things stand: AI is no silver bullet, but the advent of this new, virulent, highly infectious strain of coronavirus has been a bracing test run of its capabilities to fight infectious diseases — and helped us live with them, too.
Learn more: The Batch featured regular AI-Against-Covid news reports starting in April.
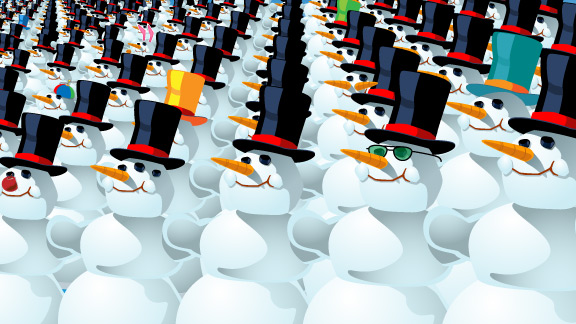
This Snowman Does Not Exist
While generative adversarial networks were infiltrating cultural, social, and scientific spheres, they quietly transformed the web into a bottomless well of synthetic images of . . . well, you name it.
What happened: Deepfakes showed up in mainstream entertainment, commercials, political campaigns, and even a documentary film in which they were used to protect onscreen witnesses. Amid the hoopla, a groundswell of online front-ends to image generators went largely unremarked.
Driving the story: Inspired by 2019’s This Person Does Not Exist, a web app that produces realistic-looking personal portraits, engineers with a sense of humor implemented generative adversarial networks (GANs) that mimic real-world minutiae. Some of our favorites:
- Trained on images from Google Earth, This City Does Not Exist produces birds-eye-views of settlements large and small.
- Even non-equestrian types can appreciate This Horse Does Not Exist’s ability to produce a wide variety of poses, breeds, and situations. Sure, it occasionally spits out a horrific jumble of limbs, but that’s half the fun.
- Like many GANs, This Pizza Does Not Exist tends to average out distinctive features. Hence, its cheeses lack a gooey sheen, its sauce is rarely vibrant, and its crusts look underbaked. But, as the adage goes, even bad pizza is still pizza.
- The authors didn’t release a web version of This Chinese Landscape Painting Does Not Exist, but in tests, its output fooled human art aficionados around half of the time.
Where things stand: Some observers worry that AI-generated fakes could undermine trust in public institutions by sowing confusion over what is and isn’t real. (Which is not to say GANs are required for that.) But the technology turns out to have a critically important use that outweighs any negative social consequences: Balancing pictures of cats on the internet with pictures of dogs.
Learn more: The Batch’s GAN special issue features stories about detecting deepfakes, making GANs more inclusive, and an interview with GAN inventor Ian Goodfellow. To learn how to build GANs yourself, check out the Generative Adversarial Networks Specialization on Coursera.
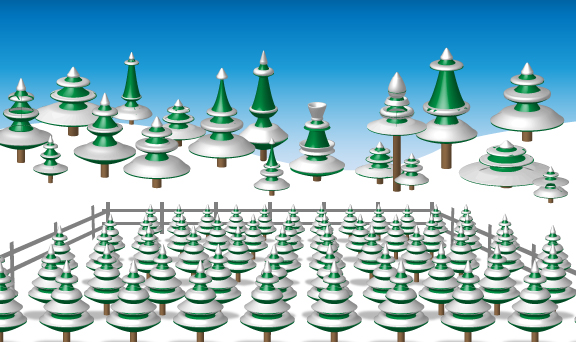
Representing the Underrepresented
Some of deep learning’s bedrock datasets came under scrutiny as researchers combed them for built-in biases.
What happened: Researchers found that popular datasets impart biases against socially marginalized groups to trained models due to the ways the datasets were compiled, labeled, and used. Their observations prompted reforms as well as deeper awareness of social bias in every facet of AI.
Driving the story: Image collections were in the spotlight — including ImageNet, the foundational computer-vision dataset.
- ImageNet creator Fei-Fei Li and colleagues combed the venerable dataset to remove racist, sexist, and otherwise demeaning labels that were inherited from WordNet, a lexical database dating back to the 1980s.
- A study found that even models trained on unlabeled ImageNet data can learn biases that arise from the dataset’s limited human diversity.
- MIT Computer Science & Artificial Intelligence Laboratory withdrew the Tiny Images dataset after outside researchers found that it was rife with disparaging labels.
- FlickrFaces-HQ (FFHQ), the dataset used to train StyleGAN, apparently also lacks sufficient diversity. This problem emerged when PULSE, a model based on StyleGAN that boosts the resolution of low-res photos, up-rezzed a pixelated image of President Barack Obama, the first Black U.S. president, into a portrait of a White man.
Behind the news: In the wake of the PULSE fiasco, Facebook’s chief AI scientist Yann LeCun and Timnit Gebru, then head of Google’s ethical AI efforts, argued publicly over whether social biases in machine learning originate primarily in faulty datasets or systemic biases within the AI community. LeCun took the position that models aren’t biased until they learn from biased data, and that biased datasets can be fixed. Gebru pointed out — and we agree, as we said in a weekly letter — that such bias arises within a context of social disparities, and that eliminating bias from AI systems requires addressing those disparities throughout the field. Gebru and Google subsequently parted amid further disagreements around bias.
Where things stand: The important work of ensuring that biases in datasets are documented and removed for particular tasks such as generating training data, has only just begun.
Learn more: The Batch in the past year reported on bias mitigation techniques including Double-Hard Debias and Deep Representation Learning on Long-Tailed Data.
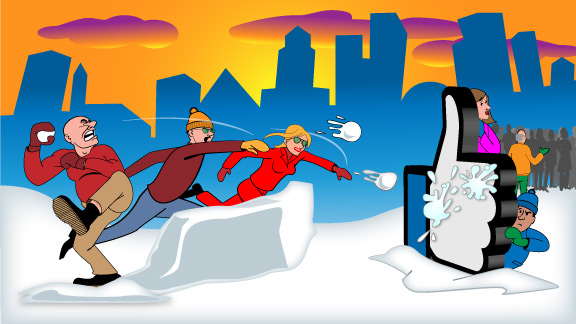
Algorithms Against Disinformation
The worldwide pandemic and a contentious U.S. election whipped up a storm of automated disinformation, and some big AI companies reaped the whirlwind.
What happened: Facing rising public pressure to block inflammatory falsehoods, Facebook, Google’s YouTube division, and Twitter scrambled to update their recommendation engines. Members of the U.S. Congress grilled the companies, a popular Netflix documentary excoriated them, and public opinion polls showed that they had lost the trust of most Americans.
Driving the story: The companies addressed the issue through various algorithmic and policy fixes — though they apparently stopped short of making changes that might seriously threaten the bottom line.
- After discovering hundreds of fake user profiles that included head shots generated by AI, Facebook cracked down on manipulated media it deemed misleading and banned deepfake videos outright. The company continues to develop deep learning tools to detect hate speech, memes that promote bigotry, and misinformation about Covid-19.
- YouTube developed a classifier to identify so-called borderline content: videos that comply with its rules against hate speech but promote conspiracy theories, medical misinformation, and other fringe ideas.
- Facebook and Twitter shut down accounts they considered fronts for state-backed propaganda operations.
- All three companies added disclaimers to content deemed to contain misleading information about the U.S. election. Twitter took its policy furthest, flagging falsehoods from President Donald Trump.
Yes, but: The reforms may not stick. The companies have diluted some, and others have already backfired.
- In June, the Wall Street Journal reported that some Facebook executives had squelched tools for policing extreme content. The company later reversed algorithmic changes made during the election that boosted reputable news sources. Perceptions that Facebook’s effort was halfhearted prompted some employees to resign.
- YouTube’s algorithmic tweaks targeting disinformation has succeeded in cutting traffic to content creators who promote falsehoods. But they also boosted traffic to larger entities, like Fox News, that often spread the same dubious information.
Where things stand: There’s no clear way to win the online cat-and-mouse game against fakers, cranks, and propagandists. But the big cats must stay ahead or lose public trust — and regulators’ forbearance.
Learn more: For more details on using AI to stem the tide of disinformation and hate speech online, see our earlier stories on Facebook’s efforts here and here, and on YouTube’s here and here.
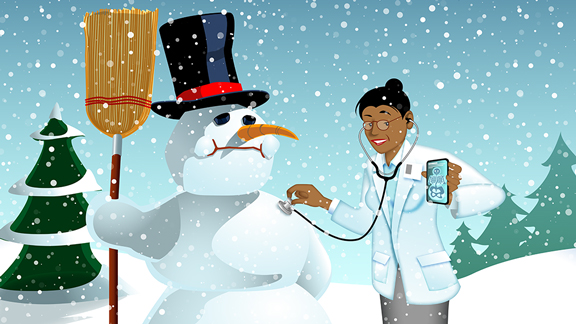
The Model Will See You Now
Institutional hurdles to AI for medicine began to fall, setting the stage for widespread clinical use of deep learning in medical devices and treatments.
What happened: DeepMind’s AlphaFold model determined the three-dimensional shape of a protein in just hours, stealing the spotlight with promises of new blockbuster drugs and biological insights. Behind the scenes, the medical establishment took important steps to bring such technologies into mainstream medical practice.
Driving the story: Institutional shifts boosted medical AI’s profile and heralded its growing acceptance.
- The largest medical insurers in the U.S., Medicaid and Medicare, agreed to reimburse doctors who use certain devices that incorporate machine learning. VizLVO from Viz.ai alerts doctors when a patient may have suffered a stroke. IDx-DR from Digital Diagnostics recognizes signs of a diabetes-related complication that can cause blindness.
- The U.S. Food and Drug Administration cleared several new AI-based treatments and devices, such as a system that conducts cardiac ultrasounds.
- An international, interdisciplinary group of medical experts introduced two protocols, Spirit and Consort, designed to ensure that AI-based clinical trials follow best practices and are reported in ways that enable external reviewers to verify the results.
Where things stand: Many applications of AI in medicine require doctors and hospitals to reorganize their workflow, which has slowed adoption to some extent. Once they’ve cleared the FDA and Medicare, clinicians have a much greater incentive to make the changes needed to take full advantage of them.
Learn more: Our AI For Medicine special issue features stories about deep learning in diagnosis, prognosis, and treatment, along with an exclusive interview with medical-AI godfather Eric Topol. Learn how to build your own medical models in the AI For Medicine Specialization on Coursera.
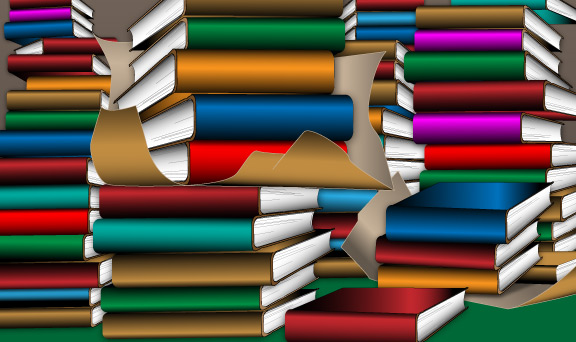
Writer’s Unblock
Neural networks for natural language processing got bigger, more prolific, and more fun to play with.
What happened: Language models, which already had grown to gargantuan size, continued to swell, yielding chatbots that mimic AI luminaries and have very strange ideas about horses.
Driving the story: OpenAI’s colossal 175 billion-parameter text generator GPT-3 showcased ongoing progress in natural language processing. It also exemplified widespread trends in machine learning: exponential rise in parameter counts, growing prevalence of unsupervised learning, and increasing generalization.
- GPT-3 writes more coherent text than its predecessor, GPT-2 — so much so that tricksters used it to produce blog articles and Reddit comments that fooled human audiences. Other users showed off the technology’s inventiveness in unique ways, such as drafting philosophical essays and inventing conversations with historical figures.
- Language modeling boosted tools for businesses, for instance by helping Apple’s autocorrect differentiate among languages, enabling Amazon’s Alexa to follow shifts in conversation, and updating the DoNotPay robot lawyer to file lawsuits against telemarketers who unlawfully call U.S. citizens.
- Meanwhile, OpenAI trained GPT-2 on pixel data to produce iGPT, which is capable of filling in partially obscured pictures to generate images of uncanny weirdness.
Where things stand: In language models, bigger clearly is better — but it doesn’t stop there. iGPT portends models trained on both images and words. Such models, which are in the works at OpenAI, at least, may be smarter, and weirder, than the giant language models of 2020.
Learn more: Our NLP special issue includes stories about counteracting bias in word embeddings, making conversation, and choosing the right words, plus an exclusive interview with NLP pioneer Noam Shazeer. Learn how to build your own NLP models in the Natural Language Processing Specialization on Coursera.