Dear friends,
There’s a lot we don’t know about the future: When will a Covid-19 vaccine be available? Who will win the next election? Or in a business context, how many customers will we have next year?
With so many changes going on in the world, many people are feeling stressed about the future. I have a practice that helps me regain a sense of control. Faced with uncertainty, I try to:
- Make a list of plausible scenarios, acknowledging that I don’t know which will come to pass.
- Create a plan of action for each scenario.
- Start executing actions that seem reasonable.
- Review scenarios and plans periodically as the future comes into focus.
For example, back in March, I did this scenario planning exercise. I imagined quick (three months), medium (one year), and slow (two years) recoveries from Covid-19 and made plans for managing each case. These plans have helped me prioritize where I can.
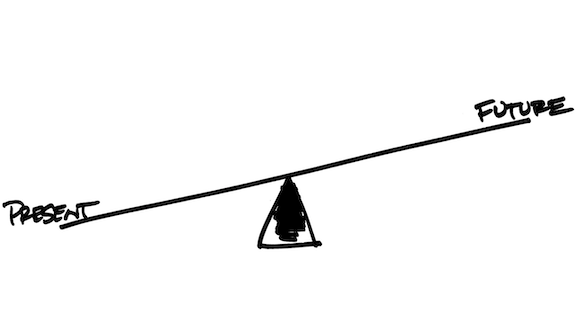
The same method can apply to personal life, too. If you’re not sure you’ll pass an exam, get a job offer, or be granted a visa — all of which can be stressful — you can write out what you’d do in each of the likely scenarios. Thinking through the possibilities and following through on plans can help you navigate the future effectively no matter what it brings.
Bonus: With a training in AI and statistics, you can calculate a probability to each scenario. I’m a fan of the Superforecasting methodology, in which the judgements of many experts are synthesized into a probability estimate. I refer to this site as a source of probability estimates as well.
There will always be uncertainty, but with a little discipline, imagination, and foresight, we can still move forward with confidence.
Keep learning!
Andrew
News
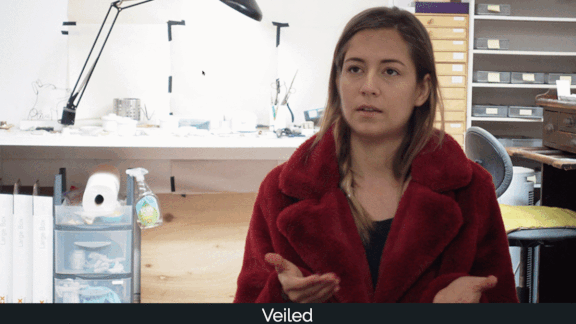
Protected By Deepfakes
Documentary filmmakers often shield the identities of people who might be harmed for speaking out. But typical tactics like blurring faces and distorting voices can make it hard for audiences to connect emotionally. A new documentary uses deepfakes to protect the privacy of at-risk subjects.
What’s new: The makers of the HBO documentary “Welcome to Chechnya” deepfaked faces of gay men and women fleeing the Russian republic of Chechnya, where LGBTQ people are being persecuted, the New York Times reported.
How it works: Visual effects supervisor Ryan Laney developed the process, which he calls Censor Veil, to paint a realistic decoy face over each of the film’s 23 subjects.
- The process combines an autoencoder with conventional visual effects, Laney told The Batch.
- U.S. LGBTQ activists volunteered to have their faces stand in for those of interviewees. Their images were captured using an array of nine cameras.
- The filmmakers blurred the faces deliberately to signal to the audience that identities were hidden.
What they’re saying: “This technology allowed us to just stretch the faces . . . over the images that I shot in the film. The face moves exactly the same way. It smiles, it cries in exactly the same way, but it is somebody else’s face.” — David France, director of “Welcome to Chechnya,” in Variety.
Behind the news: An estimated 40,000 gay men and women live in Chechnya. They are at risk of arrest, torture, and detention in secret camps. Many have been killed.
Why it matters: This technique provides a new way for journalists to preserve the impact of credible witnesses while protecting their privacy.
We’re thinking: Deepfakes are infamous for their potential to propagate mistaken identities. This work (and similar initiatives like the BLM Privacy Bot) demonstrates that swapping one person’s face for another’s can have a socially beneficial use.
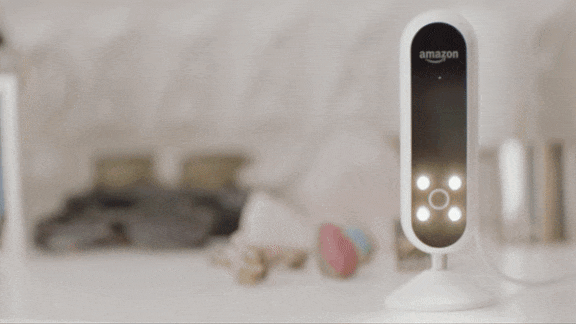
Alexa, Read My Lips
Amazon’s digital assistant is using its eyes as well as its ears to figure out who’s talking.
What’s new: At its annual hardware showcase, Amazon introduced an Alexa skill that melds acoustic, linguistic, and visual cues to help the system keep track of individual speakers and topics of conversation. Called natural turn-taking, the skill should be available next year.
How it works: Natural turn-taking fuses analyses of data from the microphone and camera in devices like the Echo Show, Echo Look, and Echo Spot.
- To determine whether a user is speaking to Alexa, the system passes photos of the speaker through a pose detection algorithm to see which way they’re facing. It also passes the voice recording through an LSTM that extracts features and a speech recognition model to decide whether the words were directed at the device. It fuses the models’ outputs to make a determination.
- The new skill also makes Alexa more responsive to interruptions. For instance, if a user asks for a Bruce Springsteen song and then says, “Play Charlie Parker instead,” Alexa can pivot from the Boss to the Bird.
- The skill understands indirect requests, like when a user butts in with “That one” while Alexa is reading a list of take-out restaurants. The system time-stamps such interruptions to figure out what the user was referring to, then passes that information to a dialogue manager model to formulate a response.
Why it matters: In conversation, people interrupt, talk over one another, and rarely use each other’s names. Making conversational interactions with AI more fluid could be handy in a wide variety of settings.
We’re thinking: Alexa now tolerates users interrupting it. Will users eventually tolerate Alexa interrupting them?
A MESSAGE FROM DEEPLEARNING.AI
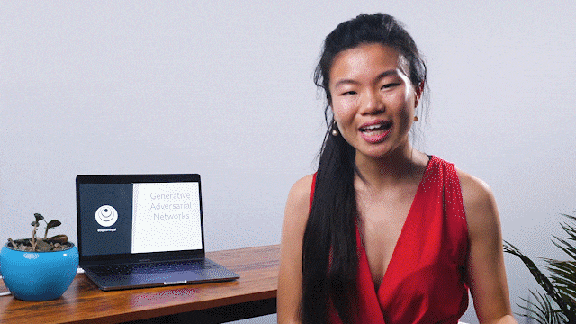
Courses 1 and 2 of our brand-new Generative Adversarial Networks Specialization are available on Coursera! Enroll now
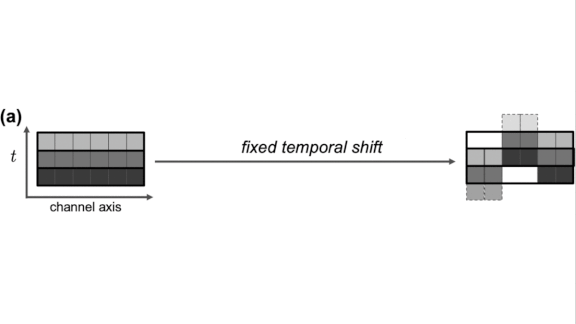
More Efficient Action Recognition
Recognizing actions performed in a video requires understanding each frame and relationships between the frames. Previous research devised a way to analyze individual images efficiently known as Active Shift Layer (ASL). New research extends this technique to the steady march of video frames.
What’s new: Led by Linxi Fan and Shyamal Buch, the Stanford Vision and Learning Lab, University of Texas Austin, and Nvidia developed RubiksShift, an efficient replacement for convolutional layers when processing time-series inputs. The name’s similarity to Rubik’s Cube apparently refers to extracting features by shifting three-dimensional data.
Key insight: A shift filter is a variation on the convolutional filter that generates only values of 0 or 1. This is more computationally efficient than traditional convolution, which generates real-valued outputs, but it prevents backpropagation, which makes shift filters difficult to train. ASL reformulated backprop for shift filters applied to still images. RubiksShift adapts ASL to video by generalizing it for an additional dimension; in this case, time.
How it works: 3D convolutional filters typically are used to process images in the three dimensions: red, blue, and green. For videos, a time is added. RubiksShift is a layer of 4D shift convolutions. The researchers also propose an architecture, RubiksNet, composed of multiple RubiksShift layers.
- Shift filters effectively translate inputs in a certain direction. Applied to images, they change the center (without stretching or rotation). Applied to videos, they change the center of individual frames and move data within them forward or backward in time (within the confines of the frame rate).
- ASL trains shift filters by introducing two parameters that determine the translation of pixels vertically and horizontally. It allows the parameters to be non-integers, but it averages them. For instance, a half-pixel shift to the right is equivalent to the average of the original position and the one to its right.
- RubiksShift adds a third parameter that represents the shift across time. It forces the parameters to converge to integer values during training, so there’s no need to average them at test time.
Results: The authors evaluated RubiksNet against state-of-the-art action recognition networks designed for efficient computation, such as I3D, using the Something-Something dataset of clips that represent human actions. RubiksNet achieved top-1 accuracy of 46.5 percent compared to I3D’s 45.8 percent, and it executed 10 times fewer floating point operations during classification. RubiksNet more than doubled the accuracy of other methods that used a similar number of operations.
Why it matters: Video is ubiquitous, and we could do a lot more with it — in terms of search, manipulation, generation, and so on — if machines had better ways to understand it.
We’re thinking: Hopefully reading this overview of RubiksNet was less confusing than trying to solve a Rubik’s Cube!
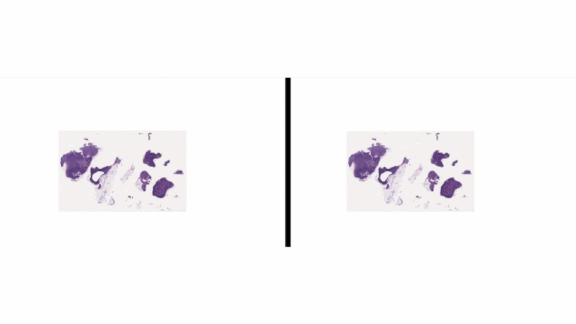
Standards for Testing Medical AI
New guidelines for reporting on experiments with medical AI aim to ensure that such research is transparent, rigorous, and reliable.
What’s new: Spirit-AI and Consort-AI are complementary protocols designed to improve the quality of clinical trials for AI-based interventions.
How it works: The guidelines are intended to address concerns of doctors, regulators, and funders of technologies such as the Google tumor detector shown above.
- Spirit-AI calls for clinical trials to observe established best practices in medicine. For example, it asks that researchers clearly explain the AI’s intended use, the version of the algorithm to be used, where its input data comes from, and how the model would contribute to doctors’ decisions.
- Consort-AI aims to ensure that such studies are reported clearly. Its provisions largely mirror those of Spirit-AI.
- Both sets of recommendations were developed by over 100 stakeholders around the world. Researchers at University of Birmingham and University Hospitals Birmingham NHS Foundation Trust led the effort.
Behind the news: Less than 1 percent of 20,500 studies of medical AI met benchmarks for quality and transparency, according to a 2019 study by researchers involved in the new initiatives.
Why it matters: These protocols could help medical AI products pass peer and regulatory reviews faster, so they can help patients sooner.
We’re thinking: The medical community has set high standards for safety and efficacy. Medical AI needs to meet — better yet, exceed — them. But the technology also poses new challenges such as explainability, and a comprehensive set of standards must address issues like that as well.