Dear friends,
I’ve been trying to teach my toddler the alphabet. Despite having some educational experience, when she mispronounces a vowel for the nth time, I can’t help but feel like I’m doing it wrong. I hope that Nova somehow will still grow up to be literate and consider my efforts to have been adequate.
Teachers have been instructing young people in languages for centuries, yet our methods strike me as remarkably uneven. I’ve tried many alphabet instruction software apps, a number of them featuring dancing animals and the like. But my favorite tools have turned out to be a word processor, which lets me type words against a plain white canvas for Nova to read, and letter-shaped stickers that I can rearrange on my kitchen wall.
I was struck by how often Nova, like a neural network, wound up in local minima. She learned to count out loud from one to five by uttering a sequence of sounds without understanding the concept of numbers, much like a recurrent neural network generates plausible text without understanding the meanings of the words it uses. I fed her the sequence of sounds, and she overfit to it. Watching her generalize (and sometimes fail to generalize) gave me fresh appreciation for the difficulty of learning from a small number of examples and how crafting a training dataset with care — curriculum learning? — can promote learning.
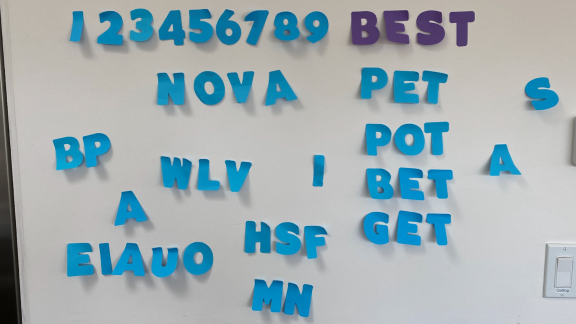
Amid the pandemic, schools worldwide find themselves in varying states of chaos, and many parents are juggling their children’s education with working from home. Many of us have insufficient time and energy to do both well. It can feel like a no-win situation.
My heart goes out to everyone who is caught in this bind. I think the best thing a parent can do is to keep loving your kids. As long as you do that, it will be more than enough. Educational apps can be great, and I hope the AI community will come up with better ones, but an attentive parent armed with a pack of post-its and a loving touch or smile is all a child really needs to learn the basics. Beyond the education you impart, the relationship you build will widen the channels of learning for a lifetime.
Keep learning!
Andrew
DeepLearning.ai Exclusive
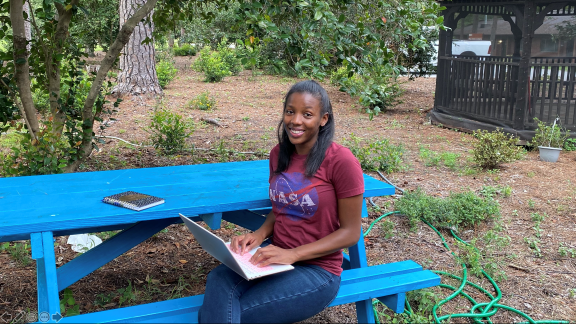
Working AI: The Optimizer
U.S. Air Force Flight Commander Ronisha Carter is charting an uncommon flight path in AI. She collaborates with academia and industry to build applications that keep the force’s planes flying efficiently. Her work could also help solve complex logistics and scheduling problems in the civilian world. Read more
News
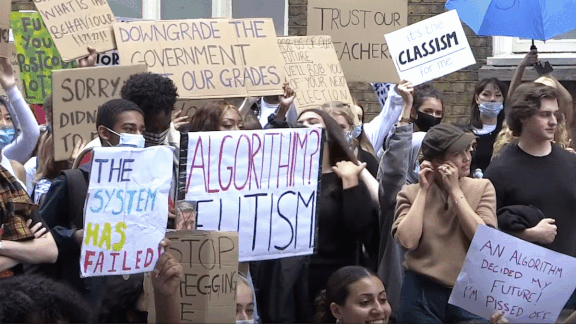
AI Grading Doesn’t Make the Grade
The UK government abandoned a plan to use machine learning to assess students for higher education.
What’s new: The UK Department of Education discarded grades generated by an algorithm designed to predict performance on the annual Advanced Level qualifications, which had been canceled due to the pandemic.
- Also known as A Levels, these tests serve as entrance exams for colleges and universities.
- The algorithm’s predictions were generally lower than teachers’ assessments of their students’ likely performance, sparking days of demonstrations in London.
- The government ultimately agreed to accept whichever grade was higher.
What went wrong: Education officials initially asked teachers to award their students an expected grade based on past performance on practice exams. This resulted in a higher-than-normal share of good grades. The department developed its model in an effort to bring grades into line with their usual distribution.
- The algorithm predicted A-level grades based primarily on two inputs: students’ past academic ranking within their school and the school’s historical performance relative to others.
- Forty percent of students across England, Ireland, and Wales received predicted scores lower than those their teachers had estimated, compared to 2.2 percent whose scores improved.
- Most students whose predicted grade was lower than the teacher’s assessment attended schools that serve primarily poor, non-white communities.
Behind the news: The pandemic also induced the International Baccalaureate Organization, a nonprofit foundation that provides two-year high school diplomas, to develop its own grading model. The organization said its model produced a distribution similar to that produced by teachers last year. Nonetheless, over 15,000 parents, students, and teachers are petitioning the foundation to reevaluate its model, which they say predicts unfairly low grades.
Why it matters: In many countries, high school exit exams determine whether students can pursue higher education. Flawed grading models can have lifelong consequences.
We’re thinking: If an AI algorithm predicted grades that were, on average, more accurate, less biased, and less noisy than those estimated by human teachers, it would be worth considering deploying it. If an AI algorithm unfairly lowered many individuals’ grades, it would seem like a terrible idea to use it. The truth is, we live in a world where AI systems can fit both descriptions simultaneously, leading to strong arguments for and against using it. Whether such systems yield a net benefit is an ethical question that requires vigorous debate. We don’t see an easy answer.
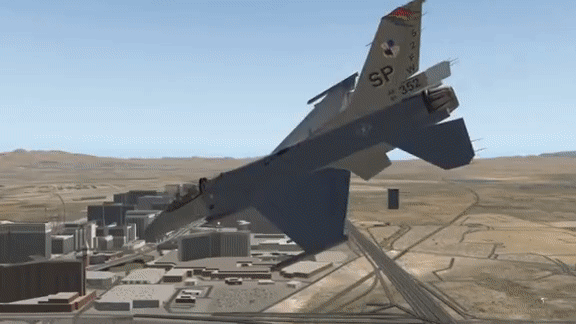
AI Versus Ace
An autonomous fighter pilot shot down a human aerial ace in virtual combat.
Watch the birdie: Built by defense contractor Heron Systems, the system also defeated automated rivals from seven other companies to win the AlphaDogfight trial. The U.S. Defense Advanced Research Projects Agency (Darpa) organized the contest as a way for defense contractors to test autonomous air-to-air combat systems.
No points for second place: The companies began building their systems last August. They competed last week in a round robin tournament, each contestant trying to nail its opponent in simulated dogfights using only nose guns.
- Heron trained its F-16 piloting agent using reinforcement learning. The model had flown 4 billion dogfights, which is equivalent to roughly 12 years of combat experience.
- The contest rules did not allow models to learn during the tournament.
- The final foe was a human U.S. Air Force fighter pilot and F-16 instructor code named Banger. Heron’s model beat Banger five matches to zero. The AI’s advantages: faster reaction time and unconventional tactics such as flying extra-close to its opponent and shooting from unusual angles.
Target rich environment: The Darpa contest is part of a broader effort to develop autonomous aerial fighters.
- In 2016, military researchers devised an automated fighter pilot that also beat a human. However, that trial was not a structured competition and didn’t test the AI against competing systems.
- In July, the U.S. Air Force awarded contracts to four defense companies to develop an AI-powered drone wingman capable of controlling its own flight while watching a human pilot’s back. The current design doesn’t carry weapons.
- Earlier in the year, the Royal Australian Air Force purchased three weapon-free drone wingmen from Boeing.
Revvin’ up the engines: Heron says its system isn’t likely to replace human pilots any time soon. Darpa says it aims to automate certain aspects of air combat, leaving the pilot more room to strategize.
We’re thinking: AI-driven fighter jets should be in movies, not future armies. We support the United Nations’ push for a global ban on autonomous weapons. Without such a ban, an unfortunate AI arms race seems inevitable.
A MESSAGE FROM DEEPLEARNING.AI
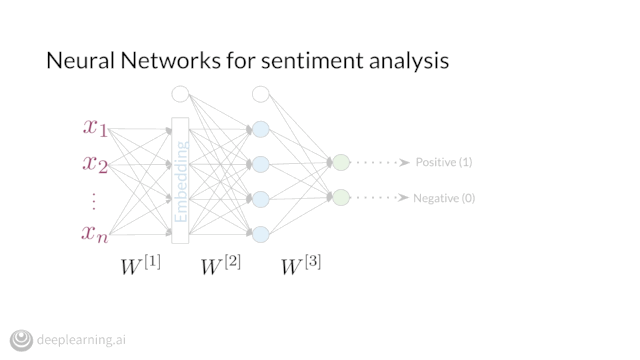
Tens of thousands of learners have enrolled in our Natural Processing Specialization since it launched two months ago. Have you?
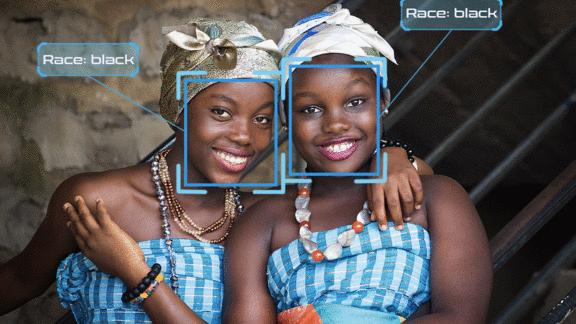
Race Recognition
Marketers are using computer vision to parse customers by skin color and other perceived racial characteristics.
What’s new: A number of companies are pitching race classification as a way for businesses to understand the buying habits of different groups, according to the Wall Street Journal. This capability is distinct from face recognition, which seeks to identify individuals. Similar systems classify physical or demographic characteristics such as age, gender, and even attractiveness.
What they found: The report identified more than a dozen companies marketing race classification for commercial use. Among them:
- Face++, one of the world’s biggest face detection companies, offers race classification for tracking consumer behavior and targeting ads.
- Spectrico said that billboard companies use its software along with gaze tracking models to learn which demographic groups look at their ads. Dating apps also use the technology to ensure their users are labeled accurately by race.
- Cognitec Systems offers race, age, and gender classification for retailers hoping to collect data about their visitors. None of its customers, which include law enforcement, has used its race classification, the company said.
- British AI company Facewatch installs face recognition cameras inside retail stores to spot suspected thieves on a watch list. It recently stopped tracking the race, gender, and age of faces deemed suspicious.
Yes, but: Experts worry that this capability could be used to discriminate against particular groups. For instance, a retailer might charge certain people higher prices. More troubling, there are signs that such systems are being used by oppressive regimes to target specific ethnicities.
Why it matters: Machine learning can be a valuable tool for identifying and analyzing demographic trends. But these tools risk invasions of privacy, discrimination both accidental and deliberate, and misuse by authorities.
We’re thinking: We can imagine a system that effectively helps detect and avoid racial bias in, say, law enforcement, yielding a net social benefit. Still, the practice of sorting people by their perceived race has a largely odious and sometimes murderous history. Machine learning engineers working in this field should tread very carefully.
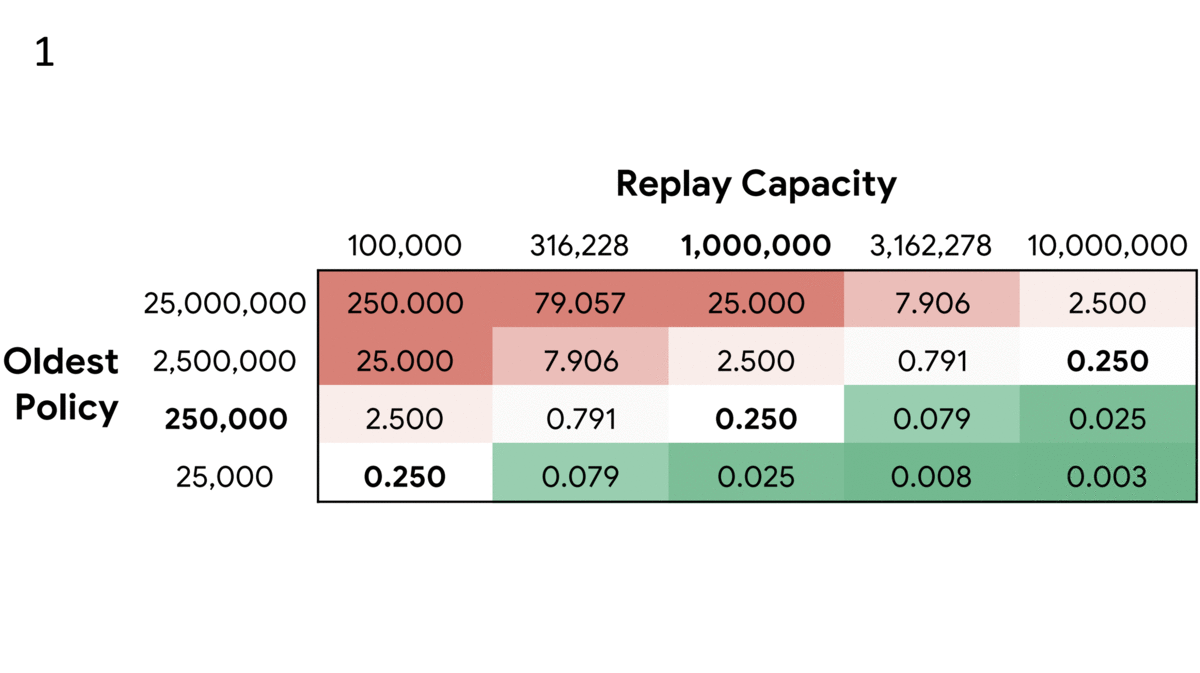
Experience Counts
If the world changes every second and you take a picture every 10 seconds, you won’t have enough pictures to observe the changes clearly, and storing a series of pictures won’t help. On the other hand, if you take a picture every tenth of a second, then storing a history will help model the world. New research applies this principle to reinforcement learning.
What’s new: William Fedus and Prajit Ramachandran led researchers at Google Brain, MILA, University of Montreal, and DeepMind to refine experience replay, a fundamental technique in reinforcement learning. The outcome: a new hyperparameter.
Key insight: Experience replay enables an agent to store observations so it can apply past experiences to present conditions. However, the faster the environment changes, the less relevant past experiences become. The authors conclude that the ratio of stored observations to updates of the agent’s strategy is a previously unrecognized hyperparameter.
How it works: In reinforcement learning, an agent observes the environment at a given frame rate, chooses actions based on its observations, receives rewards for desirable actions, and learns to maximize the rewards.
- Experience replay retains a fixed number of the agent’s most recent observations in a buffer. The agent randomly samples observations and updates its strategy accordingly. This procedure enables the agent to learn from past experiences, so it doesn’t have to repeat painful lessons.
- The primary hyperparameter in experience replay is the number of observations the buffer holds, known as its capacity. The new hyperparameter, replay ratio, is a proxy for how fast the agent learns.
- If the ratio between buffer capacity and agent policy updates is too high, learning becomes dominated by outdated perspectives. If it’s too low, the limited selection of memories allows the agent to maintain outdated habits. Figure 1 above illustrates these relationships.
Results: The team tested the new hyperparameter using Atari games, a common RL benchmark. Increasing capacity to maintain a consistent ratio improved the agent’s performance. Reducing the ratio to focus the agent on more recent observations often helped as well (Figure 2).
Yes, but: If the ratio is too low, the agent may fall back into old habits or fail to discover the optimal strategy to achieve its goal.
Why it matters: Replay ratio wasn’t a focus of attention prior to this study. Now we know the ratio affects performance. That insight may add context to previous literature that considers only capacity.
We’re thinking: Like Goldilocks tasting porridge to find the bowl whose temperature is just right, it’s likely to take a bit of trial and error to find a given agent’s optimal replay ratio.