Dear friends,
Last week, I asked what values the AI community stands for. Thank you to everyone who replied! The email responses in aggregate ran to 55 pages of text, and I enjoyed reading all of them.
A reader who works for a large company wrote, “A purely commercial objective of work is not my calling and I often find myself dreaming about how to break out of the corporate shackles and contribute the rest of my life to doing something meaningful.” These words struck a chord with me. Many of us have the good fortune to find meaning in our work. But if you don’t currently, I hope the AI community will help you do so.
Some other comments stood out to me (lightly edited):
- “A challenge for all of us working in AI is to reimagine the world with respect to concerns like healthcare, education, justice, and environmental protection.” — Shane Ó Seasnáin, Program Manager, Eindhoven AI Systems Institute, Eindhoven
- “The foundation of our shared values should be refusal to participate in works that would bring harm, regardless of political pressure and monetary rewards.” — Cecilia Cheung, Member, British Computer Society
- We stand for “fair treatment for all, establishment of trust throughout society, and decreasing the gap between the haves and have-nots.” — Shira L. Broschat, Professor, Washington State University, Pullman
- The community “believes in science, data, and facts.” — Nick Brestoff, Chief Inventor, Intraspexion, Seattle
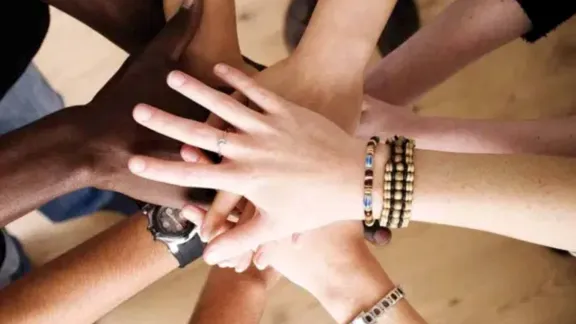
- “AI has to be made accessible to as many people as possible.” — Benjamin Freisberg, Data Scientist, Substring, Bern
- The AI community should “engage and empower the community to contribute to all levels of the conversation.” — Reece Robinson, VP Engineering, Orion Health, Auckland
- We ought to “push harder on compassion and squeeze out the cruelty.” — Natalie Smithson, Digital Innovation Copywriter, Warwick
These thoughts, and many, many others you sent, are wonderful. But one challenge of pushing on compassion (as in the last comment) is that compassion means different things to different individuals. To one person, it may mean mentoring an underprivileged student. To another, it may mean tuning an algorithm to reduce hate speech in social media.
Concepts like compassion, empowerment, and being human are easy to agree on in the absence of specifics, but difficult to define and realize in a concrete way. We all want to be compassionate. But what does that mean in practice?
We will reach a common understanding only by considering such abstractions in light of a wide variety of ways they might translate into action. This will require tireless discussion as a community. When we have a chance to talk to one another, let’s take the opportunity to discuss the values we hold in common and what it would mean to stand for them in real life. That way, the next time we feel the urge to take a stand — say, tuning a hyperparameter to reduce hate speech at the cost of revenue — we’re more likely to act in a consistent and principled way.
I’m heartened by your responses and encouraged that so many of you are looking for greater meaning and positive impact. I will continue to think about how we can come together as a community and keep the conversation going.
Keep learning!
Andrew
News
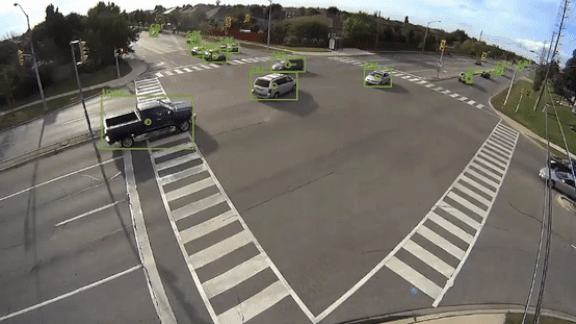
Near-Miss Detection
AI is helping avert traffic accidents by assessing the risk of car crashes at specific intersections.
What’s happening: MicroTraffic, a Canadian video analytics company, predicts the odds that accidents will occur at intersections that traditional methods overlook. More than 40 cities in Canada and the U.S. have used its analyses.
How it works: The usual approach to monitoring traffic safety identifies dangerous intersections based on crashes that already have occurred. Considering close calls brings previously unidentified trouble spots to light.
- MicroTraffic uses computer vision to identify motor vehicles, cyclists, pedestrians, and scooters in traffic-cam videos. Its system flags moments when a vehicle came close to colliding with something. The algorithm grades risk based on speed, angle, and the types of vehicles and other objects involved.
- The company provides city planners with data that show the rate of near misses at each intersection. The city, in turn, can mitigate risks by changing signal timing, adding signage, or redesigning the flow of traffic.
- Canadian nonprofit Aviva is funding five cities to install the technology at busy intersections.
Behind the news: Commercial and government organizations are working on AI for traffic safety.
- A Thai company installed face recognition systems inside its cars to detect signs of fatigue in drivers hired to travel on an accident-plagued highway. Affectiva, Bosch, Panasonic, and others have developed similar technology.
- The Finnish city of Espoo put AI-powered lidar sensors inside a busy tunnel to measure vehicle speed, congestion, and stoppages.
Why it matters: Globally, motor vehicles kill 3,700 people each day. AI could help traffic engineers cut that grim tally.
We’re thinking: When your AI software crashes, take heart in the thought that AI is reducing crashes elsewhere.
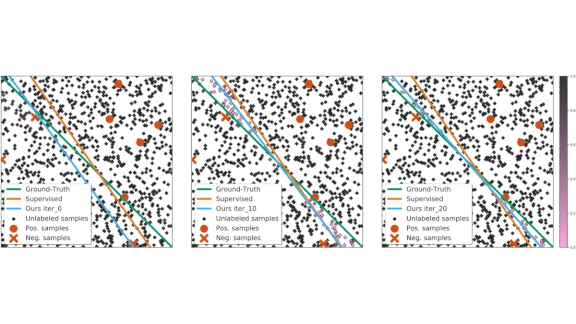
All Examples Are Not Equal
Semi-supervised learning — a set of training techniques that use a small number of labeled examples and a large number of unlabeled examples — typically treats all unlabeled examples the same way. But some examples are more useful for learning than others. A new approach lets models distinguish between them.
What’s new: Researchers Zhongzheng Ren, Raymond A. Yeh, and Alexander G. Schwing from the University of Illinois at Urbana-Champaign developed an algorithm that weighs the most significant examples more heavily.
Key insight: In its most common form, semi-supervised learning tries to minimize a weighted combination of supervised and unsupervised losses. Most previous approaches effectively weight each unlabeled example as equally important. The authors, instead of assigning one weight to all unlabeled examples, calculate weights for every example automatically by evaluating how it changes the model’s output during training.
How it works: The algorithm works with any semi-supervised model. It trains by alternating between optimizing the model and the per-example weights.
- First, the authors trained the model on the training set while keeping the per-example weights fixed.
- Then they trained the per-example weights on the validation set while keeping the model parameters fixed.
- The authors derived an influence function to calculate the gradient of the validation loss. This function measures how changing the weight assigned to an unlabeled training example affects the model parameters.
Results: Using synthetic data, the authors demonstrated that less useful examples were assigned lower weights. In image classification using the Cifar-10 and SVHN datasets, their approach marginally outperformed previous state of the art semi-supervised learning work including FixMatch and UDA. Specifically, using a Wide ResNet-28-2 and Cifar-10 with 250 labeled examples, the authors’ method combined with FixMatch achieved a classification error of 5.05 percent compared to FixMatch’s 5.07 percent. Combined with UDA, the authors’ method on Cifar-10 achieved a classification error of 5.53 percent compared to UDA’s 8.76 percent.
Why it matters: Unlabeled data points are available in far greater profusion than labeled data points. This work explores a path toward unlocking their value.
We’re thinking: Sometimes another 1,000 cat pictures don’t provide a model with any more useful information. But keep sending them anyway. The Batch team appreciates it!
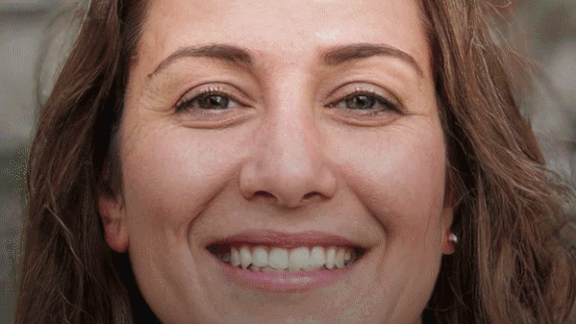
Deepfakes for Good
A strategy manifesto from one of China’s biggest tech companies declares, amid familiar visions of ubiquitous AI, that deepfakes are more boon than bane.
What’s new: A white paper issued by Tencent outlines AI’s growing impact on the global economy, particularly in media. The company argues that photorealistic images of people who don’t exist (like the portraits above, generated by thispersondoesnotexist.com), along with manipulation of images of people who do, have tremendous commercial potential despite their reputation for making mischief.
The bright side: The paper argues that what it calls deepfake synthesis — the basket of AI techniques capable of synthesizing realistic human faces, voices, and bodies, as well as other objects — could yield a range of economic and social benefits. It’s already being applied for legitimate purposes:
- Deepfake technology has been used in the movie industry to produce body doubles of deceased actors and to match motions of an actor’s mouth to voiceovers in various languages.
- It has fueled the rise of apps that let users alter their own appearance or swap faces, such as FaceAPP and Zao.
- E-commerce companies are using similar tools to provide shoppers with virtual views of themselves wearing different outfits.
- Project Revoice uses neural sound synthesis to re-create the voices of people who no longer can speak due to conditions like amyotrophic lateral sclerosis, also known as Lou Gehrig’s disease.
Beyond fakery: The company’s vision for AI isn’t just about digital doppelgangers. The paper argues that techniques like few-shot learning and offline reinforcement learning could drive broader commercialization in disciplines like natural computer vision, language processing, and voice recognition. The company also looks to AI to help improve crop yields and balance energy demand.
Behind the news: Tencent has a vested interest in promoting AI. The white paper accompanied the release of specialized machine learning platforms for entertainment, broadcasting, content review, and industrial processes, as well as Light 2.0, a program that encourages commercialization of AI research.
Why it matters: Tencent is among China’s most valuable tech companies with a strong presence in gaming, entertainment, and media. Its plans influence the direction of technology as well the attitudes of regulators who would bind it. Its push to commercialize deepfakes could open new markets — and thorny issues — for the AI community.
We’re thinking: Deepfakes have potential uses in online education, such as enabling an instructor on video to deliver a course in any number of languages. But, as the paper itself notes, regulation is necessary to thwart potential abuses.
A MESSAGE FROM DEEPLEARNING.AI
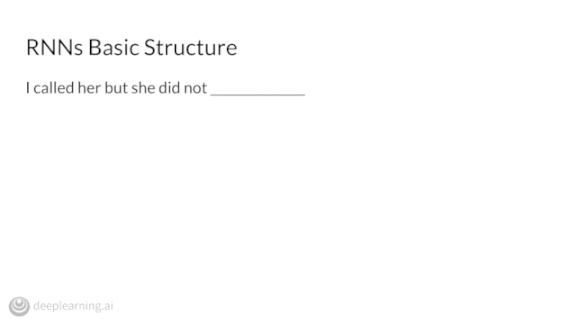
Courses 1 through 3 of our Natural Language Processing Specialization are live on Coursera. Enroll today and take the first step toward breaking into NLP!
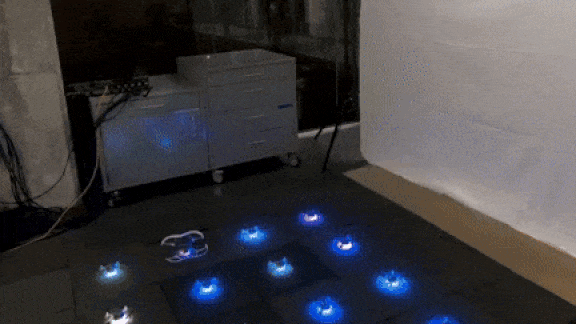
Drones of a Feather
Deep learning is coordinating drones so they can flock together without colliding.
What’s new: Caltech researchers Soon-Jo Chung and Yisong Yue developed a pair of models that enables swarms of networked drones to navigate autonomously through cluttered environments.
How it works: Sensors on each drone collect real-time data that are shared among a swarm. A neural network called GLAS plans drone actions, while another one called Neural-Swarm helps compensate for wind caused by nearby fliers.
- The authors trained GLAS via imitation learning using synthetic maps populated randomly with obstacles and drones. A global planner computed an optimal route for each synthetic drone based on relative positions of other objects and a goal for each timestep.
- At flight time, each robot computes an action for each timestep using only information from its immediate surroundings.
- The authors trained Neural-Swarm using curriculum learning, which starts with easy examples and gradually progresses to more difficult ones. Starting with two quadcopters, then three and four, Neural-Swarm learned to predict aerodynamic effects created by the myriad propellers.
- In operation, the drones use these predictions to counteract turbulence generated by nearby rotors.
Results: The authors tested GLAS and Neural-Swarm separately. In comparisons with a state-of-the-art motion planning algorithm, 16 drones piloted by GLAS navigated 20 percent more effectively through a variety of obstacle courses. Drones controlled by Neural-Swarm were four times better than a baseline linear tracking controller at staying on course.
Why it matters: Drones capable of maneuvering safely in swarms could aid urban search and rescue operations, accelerate industrial inspections, and provide comprehensive aerial mapping.
We’re thinking: Is anyone else excited to see drone shows even more spectacular than the one that lit up the 2018 Olympics?
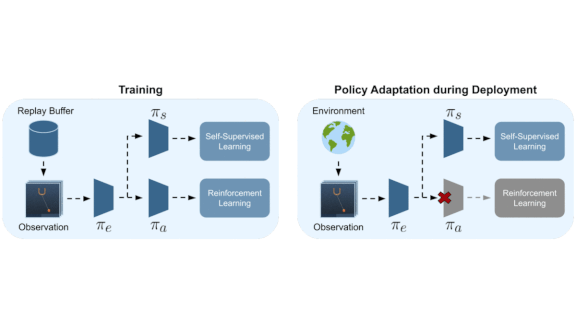
Same Job, Different Scenery
People who take driving lessons during daytime don’t need instruction in driving at night. They recognize that the difference doesn’t disturb their knowledge of how to drive. Similarly, a new reinforcement learning method manages superficial variations in the environment without re-training.
What’s new: Nicklas Hansen led a UC Berkeley group in developing Policy Adaptation during Deployment (Pad), which allows agents trained by any RL method to adjust for visual changes that don’t impact the optimal action.
Key insight: Deep reinforcement learning agents often learn to extract important features of the environment and then choose the optimal course of action based on those features. The researchers designed a self-supervised training task that updates a feature extractor to account for environmental changes without disturbing the strategy for selecting actions.
How it works: In most agents, a feature extractor captures visual information about the environment while a controller decides on actions. A change in the surroundings — say, from day to night — causes the feature extractor to derive different features, which can confuse the controller. Pad, once it’s deployed and no longer receives rewards, continues to update the feature extractor while leaving the controller unaffected. Thus the agent learns to use the same strategy regardless of environmental changes.
- Pad uses an inverse dynamics network to make the correct adjustments without receiving a reward. This network decides which action caused a transition from one state to the next. In a self-driving car, for example, it would predict that the steering wheel turned left when the car transitions from the middle lane to the left lane.
- During training, the feature extractor learns features from the controller’s loss. The inverse dynamics network learns environmental mechanics from the extracted features. This task is self-supervised; the agent keeps track of where it was, what it did, and where it ended up.
- At deployment, with a new environment and without rewards, the inverse dynamics network continues to learn. Its output updates the feature extractor, encouraging the extractor to adapt to small visual changes. The updated extractor should produce similar features for the new environment as the original version did for the training environment.
Results: The researchers evaluated Pad by training an agent via the soft actor-critic method, then substituting a plain-color background with a video at test time. On the DeepMind Control Suite, which includes motor-control tasks such as walking, Pad improved the soft actor-critic baseline in the new environment on seven of eight tasks.
Yes, but: If the environment doesn’t change, Pad hurts performance (albeit minimally).
Why it matters: To be useful in the real world, reinforcement learning agents must handle the transition from simulated to physical environments and cope gracefully with changes of scenery after they’ve been deployed. While all roads have similar layouts, their backgrounds may differ substantially, and your self-driving car should keep its eyes on the road. Similarly, a personal-assistant robot shouldn’t break down if you paint your walls.
We’re thinking: Robustness is a major challenge to deploying machine learning: The data we need to operate on is often different than the data available for training. We need more techniques like this to accelerate AI deployments.