Dear friends,
Over the weekend, we hosted our first Pie & AI meetup in Kuala Lumpur, Malaysia, in collaboration with the AI Malaysia group, MDEC, and ADAX. The event was part of Malaysia’s AI & Data Week 2019. Several people traveled from neighboring southeast Asian countries to attend!
.png)
I’m glad to see so many AI communities growing around the world, and I’m excited to bring more exposure to them. If you’d like to partner with us for a Pie & AI event, I hope you’ll drop us a note at events@deeplearning.ai.
Keep learning!
Andrew
News
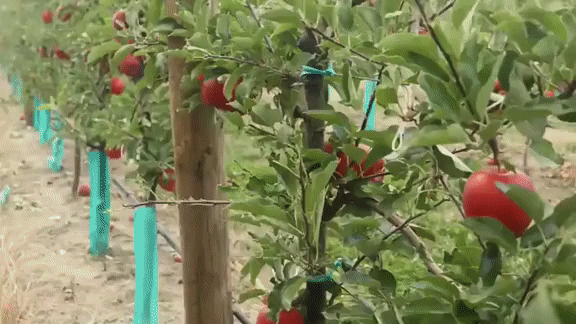
Agbots Want Jobs Americans Don’t
Advances in computer vision and robotic dexterity may reach the field just in time to save U.S. agriculture from a looming labor shortage.
What happened: CNN Business surveyed the latest crop of AI-powered farmbots, highlighting those capable of picking tender produce, working long hours, and withstanding outdoor conditions.
Robot field hands: Harvest bots tend to use two types of computer vision: one to identify ripe fruits or vegetables, the other to guide the picker.
- Vegebot, a lettuce harvester developed at the University of Cambridge, spots healthy, mature heads of lettuce with 91 percent accuracy and slices them into a basket using a blade powered by compressed air. The prototype harvests a head in 30 seconds, compared to a human’s 10-second average. The inventors say with lighter materials, they could catch up.
- Agrobot’s strawberry-picking tricycle straddles three rows of plants. It plucks fragile berries using up to 24 mechanical hands, each equipped with a camera that grades the fruit for ripeness.
- California’s Abundant Robotics built a rugged, all-weather autonomous tractor that vacuums up ripe apples (pictured above).
Behind the news: Unauthorized migrants do as much as 70 percent of U.S. harvest work, according to a study by the American Farm Bureau Association. Tighter immigration policies and improving opportunities at home increasingly keep such workers out of the country.
Why it matters: The shortage of agricultural workers extends across North America. During harvest season, that means good produce is left to rot in the fields. The situation costs farmers millions in revenue and drives up food prices.
Our take: The robots-are-coming-for-your-job narrative often focuses on people put out of work but fails to acknowledge that workers aren’t always available. Between a swelling human population and emerging challenges brought on by climate change, the agriculture industry needs reliable labor more than ever. In some cases, that could be a machine.
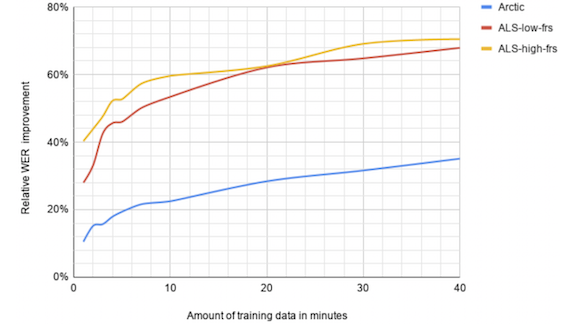
Speech Recognition With an Accent
Models that achieve state-of-the-art performance in automatic speech recognition (ASR) often perform poorly on nonstandard speech. New research offers methods to make ASR more useful to users with heavy accents or speech impairment.
What’s new: Researchers at Google fine-tuned ASR neural networks on a data set of heavily accented speakers, and separately on a data set of speakers with amyotrophic lateral sclerosis (ALS), which causes slurred speech of varying degree. Their analysis shows marked improvement in model performance. The remaining errors are consistent with those associated with typical speech.
Key insight: Fine-tuning a small number of layers closest to the input of an ASR network produces good performance in atypical populations. This contrasts with typical transfer learning scenarios, where test and training data are similar but output labels differ. In those scenarios, learning proceeds by fine-tuning layers closest to the output.
How it works: Joel Shor and colleagues used data from the L2-ARCTIC data set for accented speech and ALS speaker data from the ALS Therapy Development Institute. They experimented with two pre-trained neural models, RNN-Transducer (RNN-T) and Listen-Attend-Spell (LAS).
- The authors fine-tuned both models on the two data sets with relatively modest resources (four GPUs over four hours). They measured test-set performance on varying amounts of new data.
- They compared the sources of error in the fine-tuned models against models trained on typical speech only.
Results: RNN-T achieved lower word error rates than LAS, and both substantially outperformed the Google Cloud ASR model for severe slurring and heavily accented speech. (The three models were closer with respect to mild slurring, though RNN-T held its edge.) Fine-tuning on 15 minutes of speech for accents and 10 minutes for ALS brought 70 to 80 percent of the improvement.
Why it matters: The ability to understand and act upon data from atypical users is essential to making the benefits of AI available to all.
Takeaway: With reasonable resources and additional data, existing state-of-the-art ASR models can be adapted fairly easily for atypical users. Whether transfer learning can be used to adapt other types of models for broader accessibility is an open question.
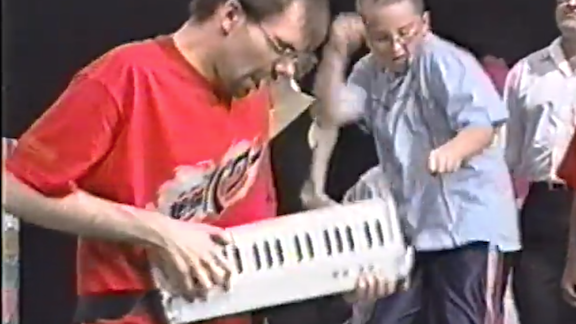
Generative Models Rock
AI’s creative potential is becoming established in the visual arts. Now musicians are tapping neural networks for funkier grooves, tastier licks, and novel subject matter.
What happened: Aaron Ackerson, whom the Chicago Sun-Times called “a cross between Beck and Frank Zappa,” produced his latest release with help from the latest generation of generative AI. MuseNet helped generate the music and GPT-2 suggested lyrics. DeepAI’s Text To Image API synthesized the cover art.
Making the music: “Covered in Cold Feet” began its existence as an instrumental fragment scored for violin, piano, and bass guitar.
- Ackerson fed a two-bar MIDI file into MuseNet, which spat out a few more bars based on his raw material.
- He tweaked MuseNet’s output to his liking using his digital audio workstation. Then he fed that material back to MuseNet, which expanded by a few bars more, repeating the process until he had a composition he was happy with.
- “Early in the process, I had MuseNet generate continuations of my simple idea in a lot of different styles, and most did not find their way into the finished song,” Ackerson said in an interview with The Batch. “The one I decided to use followed the main melody with what would later turn into the beginning of the guitar solo.”
- That solo combusts into righteous shredding near the 1:25 mark, a triumph of manual dexterity as much as AI.
Writing the lyrics: The groove reminded Ackerson of the band Phish. So he fed a list of that band’s song titles to Talk to Transformer, an online text-completion app based on the half-size version of GPT-2.
- “Covered in Cold Feet” was his favorite of its responses to the original list.
- He repeatedly fed Talk to Transformer the phrase “covered in cold feet again,” and curated the lyrics from its responses.
- Talk to Transformer doesn’t generate rhymes, so Ackerson added them manually.
Behind the music: The artist composed his first AI-assisted song in 2017 using the Botnik Voicebox text generator. He fed the model bass and melody lines from 100 of his favorite songs translated into solfège (a note-naming system that maps the tones in any musical key to the syllables do, re, mi, and so on). The model spat out fresh note pairings, many of which he had never before considered using. The result, “Victory Algorithm,” is a slide guitar-fueled psychobilly foot stomper.
We’re thinking: AI skeptics worry that computers, if allowed to do creative work, will erase humanity from art. No worries on that point: Ackerman’s personality comes through loud and clear. We look forward to hearing more from musicians brave enough to let computers expand their creative horizons. (For more on MuseNet, see our interview with project lead Christine Payne.)
A MESSAGE FROM DEEPLEARNING.AI
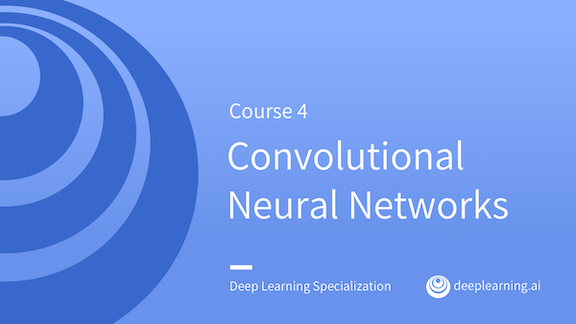
Padding is an important modification in building a basic convolutional neural net. Learn more about padding in Course 4 of the Deep Learning Specialization. Enroll now
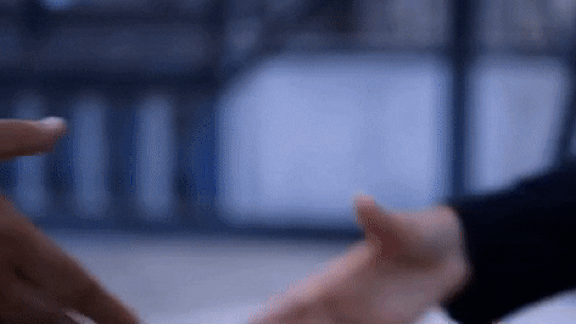
My Chatbot Will Call Your Chatbot
Companies with large numbers of contractual relationships may leave millions of dollars on the table because it’s not practical to customize each agreement. A new startup offers a chatbot designed to claw back that money.
What happened: Pactum, a startup that automates basic vendor and service contracts at immense scale, emerged from stealth with a $1.15 million investment from Estonian tech upstart Jaan Tallinn and his posse of Skype alumni.
How it works: Let’s say a prominent computer company develops a new laptop and hires Pactum to cut distribution deals with hundreds of thousands of computer stores around the globe.
- Pactum’s AI model reviews the computer maker’s existing contracts to establish baseline terms.
- Then it examines variables such as pricing, schedule, and penalties in search of more favorable arrangements. For instance, it may seek to improve cash flow by asking retailers to pay for orders faster.
- The AI then initiates negotiations via chatbot.
- The model automatically updates contract terms as negotiations proceed.
Behind the news: Contracts are a hot area for AI. In 2015, Synergist.io and Clause launched automated platforms that mediate contract negotiations. And last year, Dutch information services firm Wolters Kluwer acquired legal AI startups CLM Matrix and Legisway.
Why it matters: Standardized contracts can save time and effort spent customizing agreements. But they also bring costs. A 2018 study by KPMG estimated that standard contracts can soak up between 17 and 40 percent of a contract’s expected revenue.
The Tallinn Effect: Funding from Jaan Tallinn brings the credibility of a serial entrepreneur who co-founded Skype and Kazaa and invested in DeepMind. It’s also a stamp of approval from a technologist who thinks deeply about AI’s potential for both benefit and harm. Tallinn co-founded the Centre for the Study of Existential Risk and once wrote, “In a situation where we might hand off the control to machines, it’s something that we need to get right.” Apparently he believes Pactum meets that standard.
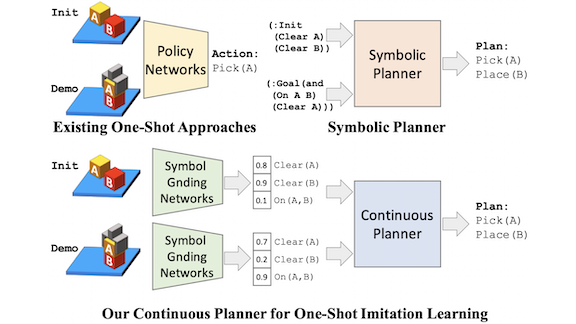
Working Through Uncertainty
How to build robots that respond to novel situations? When prior experience is limited, enabling a model to describe its uncertainty can enable it to explore more avenues to success.
What’s new: In reinforcement learning, meta-learning describes teaching a model how to complete multiple tasks, including tasks the model hasn’t seen before. One way to approach meta-learning is to divide it into two subproblems: creating a plan based on current surroundings and the task at hand, and taking action to implement the plan. Stanford researchers developed deep learning models that facilitate the planning phase by learning to generate better representations of the task.
Key insight: Deep learning has been used to learn vector descriptions of the initial state prior to accomplishing a task and the final state afterward. The new work uses probabilistic descriptions, allowing more flexibility in novel tasks. For example, instead of having to choose between the contradictory descriptions object 1 is on object 2 and object 2 is on object 1, the network updates its confidence in each statement throughout the planning steps.
How it works: Previous methods use a neural network model as a classifier to decide state descriptions from potential configurations. Instead, De-An Huang and his colleagues use the model’s confidence in each potential configuration to represent states. This approach produces a probabilistic description of current and final states.
- For training, the model takes a set of demonstrations of similar tasks plus the actions available to the planning algorithm. For testing, it takes a single demonstration of a novel task, the initial state, and the allowed operations.
- For both initial and final states, a network is trained to predict the probability that certain configurations are observed. For example, based on an image, learn the probability that object 1 is on top of object 2.
- The planning algorithm takes the probabilistic descriptions and selects the action most likely to move the initial state closer to the final state. Since the choice is a function of the state descriptions and potential operations, the planning algorithm requires no training.
Results: The authors’ approach achieves state-of-the-art meta-learning performance in sorting objects and stacking blocks. When sorting, it matches performance based on human heuristics. When stacking, it outperforms human heuristics plus fixed state descriptions with less than 20 training examples (although the heuristics win with 30 training examples).
Yes, but: The researchers achieved these results in tasks with a small number of operations and potential state configurations. Their method likely will struggle with more complex tasks such as the Atari games that made deep reinforcement learning popular.
Takeaway: In past models, misjudgments of surroundings and goals tend to accumulate, leading the models far from the intended behavior. Now, they can relax their fixed state descriptions by representing potential points of confusion as probabilities. This will enable them to behave more gracefully even with little past experience to draw on.
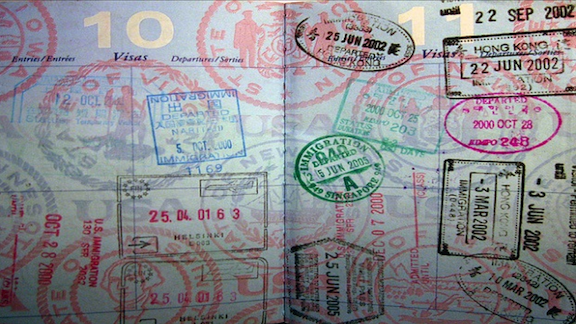
Easing Cross-Border Collaboration
Tighter national borders impede progress in AI. So industry leaders are calling for a different kind of immigration reform.
What happened: The Partnership on AI published a report calling out the corrosive effect of restrictive immigration policies and suggesting alternatives.
What it says: The nonprofit industry consortium’s report calls for countries aiming to grow their AI industry to ease visa requirements for high-skilled tech workers. Among its recommendations:
- Countries should present visa rules clearly and make the review process transparent, so applicants know they are being judged on skill. Get rid of caps, too.
- Provide assistance to students and low-income applicants in navigating the visa process. Countries serious about fostering AI should provide students with pathways to citizenship.
- Update immigration laws to redefine the concept of family to include long-term partnerships and nontraditional marriages.
- No nation should bar tech workers based on nationality. Moreover, countries should apply patent law, not immigration policy, to protect intellectual property.
Why it matters: Strict immigration policies limit opportunities for researchers and practitioners. They also shut out students, small businesses, startups, and small colleges from the broader AI community. In the U.S., tighter borders are cutting into the country’s competitiveness in AI, according to a new study from Georgetown University’s Center for Security and Emerging Technology. CSET recommends eliminating existing U.S. barriers to recruiting and retaining foreign-born AI talent.
We’re thinking: Human capital is critical to AI, and the global community has an interest in channeling it toward worthwhile projects. The Partnership on AI’s recommendations offer a solid foundation for international trade groups, such as the Organization for Economic Cooperation and Development, to build policies that accelerate progress by opening the world to workers in emerging tech.