Dear friends,
I received a copy of Why We Sleep: Unlocking the Power of Sleep and Dreams as a Christmas gift — back in the pre-Covid era — and finished it last weekend. This book by Matthew Walker, director of UC Berkeley’s sleep and neuroimaging lab, is a useful reminder of the importance of sleep for learning and also for physical and mental health.
Say you spend a few hours learning something new on Wednesday. Getting a solid night of sleep the same day will help consolidate the new memories and strengthen your long-term retention. If your sleep on Wednesday night is disrupted, your long-term retention will be affected even if you catch up on sleep later in the week.
But the story doesn’t end there. Over the next few days, your brain may still be busy consolidating the new learnings. A surprising study showed that even if your sleep is disrupted on Friday — two days later — long-term retention can still be significantly affected.
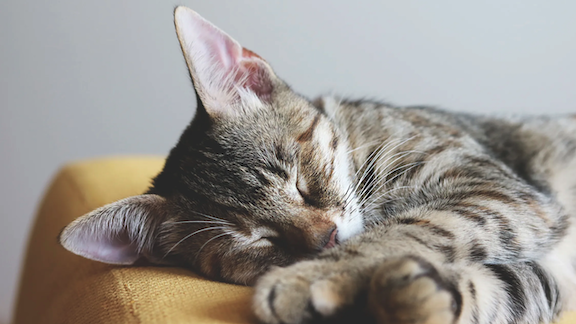
Bottom line: After you spend time studying during the day, I encourage you to get a good night’s sleep. Even better, try to get a good night’s sleep every night.
The world is going through turbulent times. With society buffeted by biological, social, and political forces, who has time for sleep?! I try to sleep from midnight to 8 a.m. every day, including weekends. With an 18-month-old daughter who wakes up whenever she wants, and occasional meetings with business partners in Asia or in Europe at odd hours, my sleep schedule is far from perfect.
You’re probably incredibly busy as well. Despite everything going on, I make sleep a priority, and I hope you will, too.
Keep learning,
Andrew
News
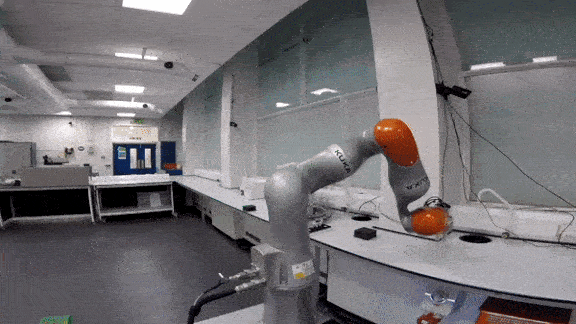
Scientific Discovery on a Roll
A mechanical lab assistant could accelerate chemistry research.
What’s new: Researchers at the University of Liverpool trained a mobile robot arm to navigate a lab, operate equipment, handle samples, and obtain results far faster than a human scientist. The authors believe their system is the first mobile robot capable of running lab experiments.
How it works: In a recent study, the articulated arm on wheels completed 688 experiments, testing various hypotheses to extract hydrogen from water efficiently using chemicals and light.
- The system navigates using lidar, so it can operate in the dark.
- The researchers divided the lab into a series of stations devoted to specific procedures. Upon arriving at each station, the arm calibrated its position by tapping the sides of cubes that the scientists had mounted next to each piece of gear.
- The arm is topped with a gripper for mixing chemical samples and operating laboratory equipment.
- A Bayesian optimization model uses the results of each experiment to update the next round by adjusting one of 10 variables, such as the chemical mixture.
Results: The study discovered chemical formulae that made it easier to separate hydrogen from oxygen in water. More important, it proved that a robot can do such work effectively, speedily, and without interruption. The authors estimate that a human scientist would have taken 1,000 times longer to produce similar results.
Why it matters: The authors hope to offer robots for sale within 18 months. The $150,000-plus price tag might be a bargain if the Covid-19 pandemic makes in-person lab experimentation unfeasible.
We’re thinking: Most factory automation involves stationary robots positioned along a manufacturing line. Perhaps mobile manipulation — where the arm moves to the object being manipulated — will prove to be more efficient for automating science labs.
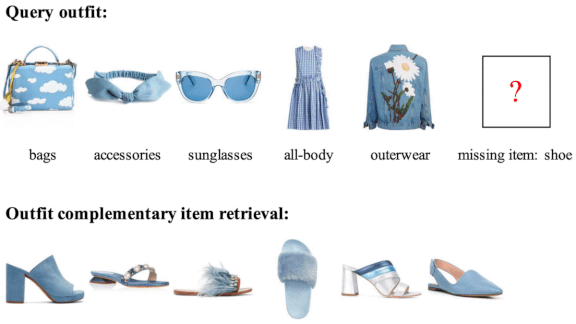
Which Shoes Go With That Outfit?
Need a wardrobe upgrade? You could ask the fashion mavens at Netflix’s Queer Eye — or you could use a new neural network.
What’s new: Yen-Liang Lin, Son Tran, and Larry S. Davis at Amazon propose Category-based Subspace Attention Network (CSA-Net) to predict and retrieve compatible garments and accessories that complement one another. (This is the third of three papers presented by Amazon at the IEEE Conference on Computer Vision and Pattern Recognition (CVPR). We covered the others in previous issues.)
Key insight: Suppose you have several items that go together and want one more to complete the ensemble. Past approaches such as SCE-Net can find compatible outfits by scoring pairs of garments or accessories, but Amazon’s catalogue is too vast to compare every pair of items in it. CSA-Net retrieves items by learning a vector description of each item and finding nearby items. The network adjusts its representation based on the categories already selected. For instance, given a shirt and shoes, it can find a matching handbag or hat.
How it works: The researchers trained CSA-Net by providing outfits to complete, sets of candidate items, and labels that identify compatible candidates. CSA-Net learned to place outfits and compatible items nearby in the feature space while placing incompatible items farther away.
- A convolutional neural network learns features from an image of a garment or accessory.
- An attention mechanism modifies the features to place different types of items that go together — matching shirts and pants, matching pants and shoes — in distinct subspaces, or portions of the feature space.
- Presented with several items that comprise an incomplete outfit, CSA-Net predicts a missing item by pairing it with each item separately. Say you have a hat, pants, and shoes, and you want a top. The system looks for a top that goes with your hat, then a top that goes with your pants, and so on. It settles on the top that’s nearest to every other item.
Results: The researchers evaluated CSA-Net on the Polyvore-Outfit dataset of fashion items and labels that detail their compatibility. Provided an incomplete outfit of four items, CSA-Net predicted the correct fifth piece 59.26 percent of the time, compared with 53.67 percent achieved by the previous state of the art. It also outperformed the previous state of the art in predicting whether a pair of garments is compatible, achieving a higher area under the curve (the probability of predicting a positive match instead of a negative match).
Why it matters: The universe of fashion items and accessories is immense and complex, posing a challenge for matching items situated in a feature space. CSA-Net makes the task more tractable by restructuring the feature space into compatible subspaces.
We’re thinking: Leave it to machine learning engineers to build technology that liberates them from having to decide which shirt goes with what pants and shoes.
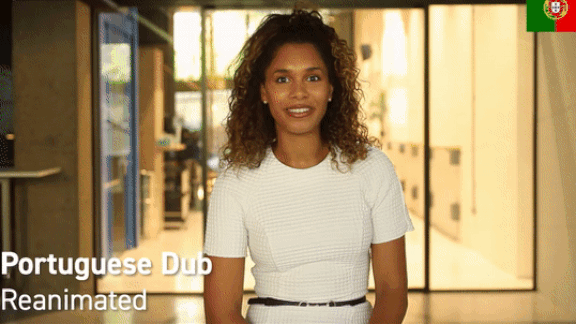
Deepfakes Go Corporate
The same technology that has bedeviled Hollywood stars and roiled politics is easing corporate communications.
What’s new: Synthesia generates training and sales videos featuring photorealistic, synthetic talking heads that read personalized scripts in any of 34 languages, Wired reports. You can try out the service here.
How it works: The company uses GANs for much of its rendering, but its production pipeline includes customized deep learning, computer vision, and visual effects, a representative told The Batch. Clients submit a script and choose from a selection of avatars, languages, and voices, and the AI generates a video of the avatar reading the client’s words.
- Advertising giant WPP used the service to create a series of training programs for its staff. Each program is roughly five minutes long and presented in English, Mandarin, and Spanish, and the avatar addresses each of WPP’s 50,000 employees by name.
- The avatars are based on human actors who are paid whenever a client chooses their likeness. Clients can also use custom avatars based on video footage.
- The system has been used to translate a public service announcement by football star David Beckham into nine languages and to help an English-speaking man propose to his wife in Mandarin.
Behind the news: Generated video is also catching on in advertising and marketing.
- Synthesia adapted a recording by rapper Snoop Dogg for an ad.
- Generated video appeared in a commercial broadcast during ESPN’s docu-series “The Last Dance.” The video was part of a simulated news report from the 1990s in which a commentator mused that ESPN one day would produce such a documentary.
- Rosebud AI offers a tool that lets clothing companies dress generated fashion models in their garments.
Why it matters: Producers of commercial video and photography have become interested in AI’s ability to generate realistic human characters as the pandemic has curtailed live film shoots, according to the Synthesia CEO and co-founder Victor Riparbelli. Generated characters save the cost of hiring cast and crew and make it easy to localize productions for a worldwide audience. Plus, there’s no danger of spreading a deadly cough.
We’re thinking: It’s easy to see potential harm in deepfakes, but the same techniques have productive uses for people with imagination to recognize them and ingenuity to implement them at scale.
A MESSAGE FROM DEEPLEARNING.AI
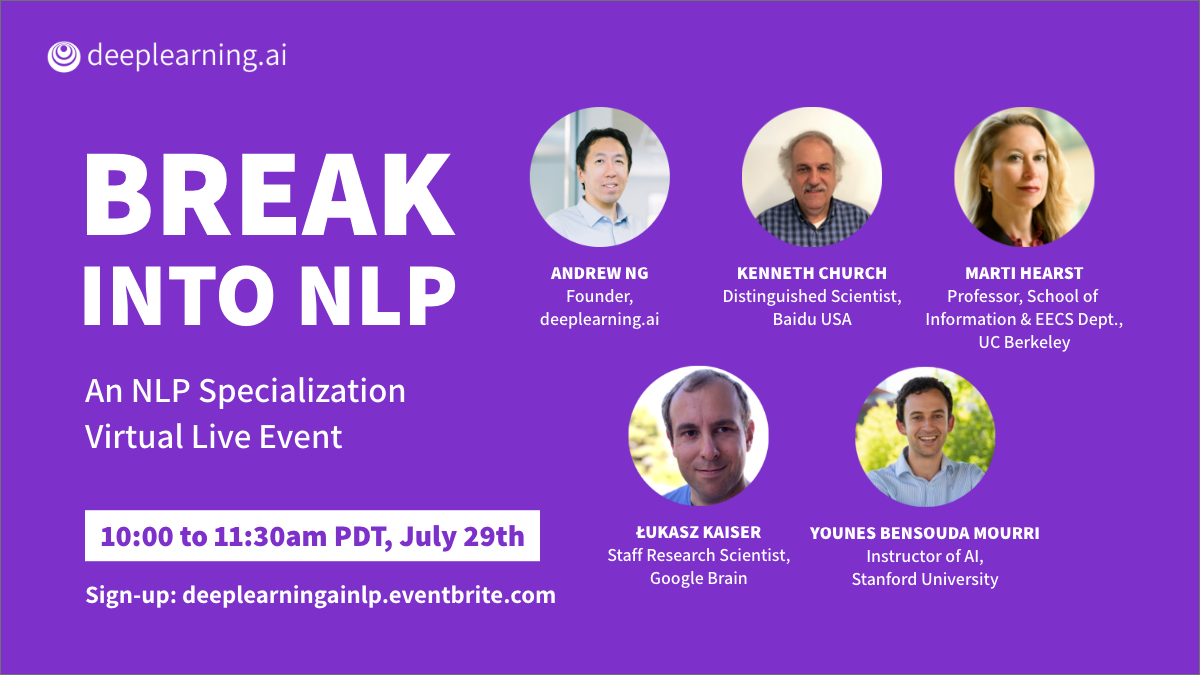
Join us for Break Into NLP, a virtual live event on July 29th from 10 a.m. to 11:30 a.m. PDT. Celebrate the launch of Course 3 of our Natural Language Processing Specialization and hear from from Andrew Ng, Kenneth Church, Marti Hearst, and other NLP experts!
Sign up now to receive the event access link.
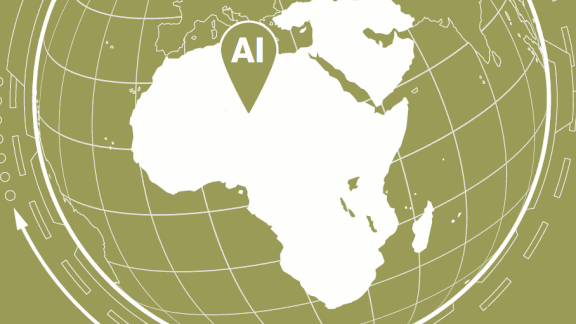
AI in Regions Rich and Poor
Companies in Africa and the Middle East are building AI capacity in very different ways, a new study found.
What’s new: AI is growing fast in both regions despite shortages of talent and data, according to MIT Technology Review Insights, the research arm of Massachusetts Institute of Technology’s magazine. Yet the implementations in each region reflect stark differences in economic development.
What it says: The report focuses on wealthy countries in the Persian Gulf, particularly Saudi Arabia and the United Arab Emirates, as well as African tech hotspots in Ghana, Kenya, and Nigeria.
- Across both regions, 82 percent of respondents use AI in their business.
- Many Gulf-based companies are using AI to help shift their business away from oil and toward innovation.
- African AI startups tend to focus on meeting domestic challenges like access to food or medicine.
- Many African companies provide AI-based services like ride-hailing and credit scoring to lower-income individuals and small businesses.
- Over half of respondents said AI is saving them money, and 44 percent believe that the technology will drive a quarter of their operations by 2023.
Growing pains: AI adoption hasn’t been smooth sailing. Nearly 60 percent of respondents said they’ve struggled to apply AI in their business. Nearly as many cited difficulty obtaining high-quality data. Africa and the Middle East are also struggling to find talent, with 40 percent of respondents noting a shortage of AI professionals in the regions.
Why it matters: AI could prove to be a boon for individuals, and the planet at large, by helping to lift African economies and wean Middle Eastern ones from reliance on oil.
We’re thinking: The Persian Gulf is one of the world’s richest regions, and sub-Saharan Africa its poorest. The fact that both are turning to AI says a lot about the technology’s potential to streamline existing economies and foster new ones.
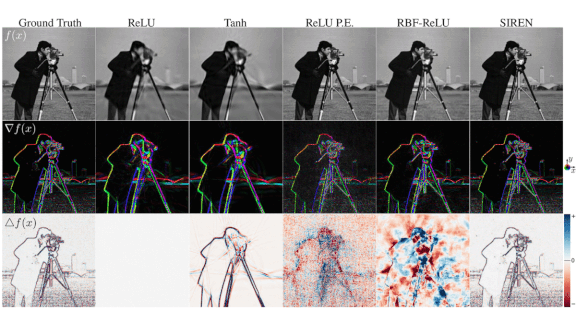
Upgrade for ReLU
The activation function known as ReLU builds complex nonlinear functions across layers of a neural network, making functions that outline flat faces and sharp edges. But how much of the world breaks down into perfect polyhedra? New work explores an alternative activation function that yields curvaceous results.
What’s new: Stanford researchers led by Vincent Sitzmann and Julien Martel developed the periodic activation function sin(x) to solve equations with well defined higher-order derivatives. They showed preliminary success in a range of applications.
Key insight: Training a neural network updates its weights to approximate a particular function. Backprop uses the first derivative to train networks more efficiently than methods such as hill-climbing that explore only nearby values. Higher-order derivatives contain useful information that ReLU can’t express and other activation functions describe poorly. For example, in the range 0 to 1, the values of x and x2 are similar, but their derivatives are dramatically different. Sine has better-behaved derivatives.
How it works: Sine networks, which the researchers call sirens, are simply neural networks that use sine activation functions. However, they need good initial values.
- A sine network can use layers, regularization, and backprop just like a ReLU network.
- The derivative of a ReLU is a step function, and the second derivative is zero. The derivative of sin(x) is cos(x), which is a shifted sine. Since the derivative of a sine network is another sine network, sine networks can learn as much about the derivative as the original data.
- Since successive layers combine sine functions, their oscillations may become very frequent. Hectic oscillations make training  difficult. The researchers avoided this pitfall by generating initialization values that maintain a low frequency.
Results: The authors used sine networks to solve differential equations (where they can learn directly from derivatives), interpret point clouds, and process images and audio. They provide examples and a collab notebook so you can try it yourself. They demonstrated success in all these domains and provided quantitative evidence for the value of gradients when applied to Poisson image reconstruction. The authors trained models to predict the gradient of an image and compared the quality of generated images after reconstruction using Poisson’s equation. Evaluated on the starfish image above, a sine network achieved 32.91 peak signal-to-noise ratio, a measurement of reconstruction quality, compared with 25.79 for Tanh.
Why it matters: ReLUs have been a deep learning staple since 2012. For data that have critical higher-order derivatives, alternatives may improve performance without increasing model complexity.
We’re thinking: ReLUs may be good for drawing the angular Tesla Cybertruck, but sines may be better suited for a 1950 Chevy 3500.