Dear friends,
I am appalled by the policy, announced on Monday by U.S. Immigrations and Customs Enforcement (ICE), that international students in the country on an F-1 visa must leave if their school goes fully online to cope with Covid-19.
Two weeks ago, I wrote about the suspension of H1-B visas for foreign workers. The policy unveiled this week will deepen the pain of young people who are aiming to contribute to society and further deprive the U.S. of much-needed talent.
The new policy, which is being called the #StudentBan on social media, is cruel and capricious. Sometimes an entire family may pool their savings to send someone to study and give them a brighter future. Imagine being halfway to earning a degree and suddenly forced to leave the country amid the pandemic, when your home country may have closed its borders, even to citizens. Students have confided to me their worries about letting down their family or being unable to afford a plane ticket home.
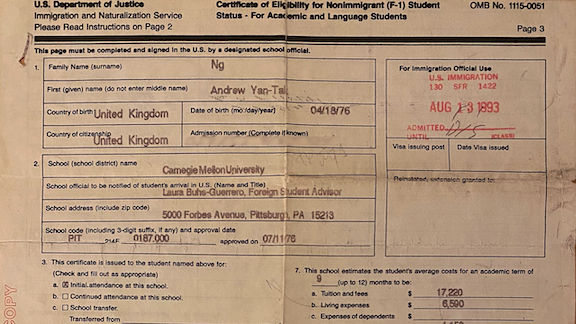
University faculty and administrators are scrambling to offer in-person classes, even if it may not be safe or may have little pedagogical benefit, just for the purpose of protecting their international students from deportation. They were already struggling to manage campus shutdowns. This policy delivers another blow at a time when they least can afford it.
The U.S. is known worldwide as a great place to receive an education. That’s why I came here many years ago — on an F-1 visa — to attend college. If the U.S. loses this reputation, the whole world will be poorer for it.
If my daughter ever studies overseas, I hope that whatever country hosts her will treat her with greater kindness and respect than the U.S. is extending to our international students.
Keep learning,
Andrew
DeepLearning.ai Exclusive
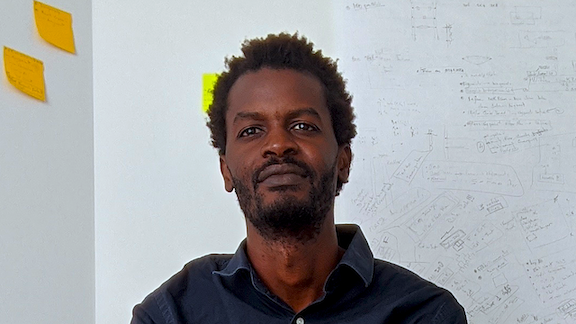
Working AI: The Builder
Growing up in Mauritania, Adama Diallo was fascinated by the human brain. Now, as an AI developer at the software company Paradigm, he’s using artificial intelligence to map architectural spaces. In this edition of our Working AI series, Adama discusses his projects, advice for learners, and views on social bias in the industry. Learn more
News
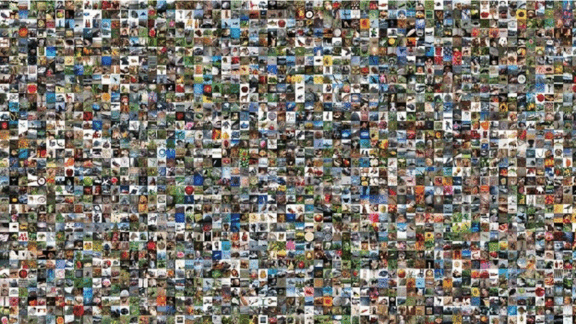
Tiny Images, Outsized Biases
MIT withdrew a popular computer vision dataset after researchers found that it was rife with social bias.
What’s happening: Researchers found racist, misogynistic, and demeaning labels among the nearly 80 million pictures in Tiny Images, a collection of 32-by-32 pixel color photos. MIT’s Computer Science and Artificial Intelligence Lab removed Tiny Images from its website and requested that users delete their copies as well.
What the study found: Researchers at the University College of Dublin and UnifyID, an authentication startup, conducted an “ethical audit” of several large vision datasets, each containing many millions of images. They focused on Tiny Images as an example of how social bias proliferates in machine learning.
- Psychologists and linguists at Princeton in 1985 compiled a database of word relationships called WordNet. Their work has served as a cornerstone in natural language processing.
- Scientists at MIT CSAIL compiled Tiny Images in 2006 by searching the internet for images associated with words in WordNet. The database includes racial and gender-based slurs, so Tiny Images collected photos labeled with such terms.
What the dataset’s creators said: “Biases, offensive and prejudicial images, and derogatory terminology alienates [sic] an important part of our community — precisely those that we are making efforts to include,” the researchers who built Tiny Images said in a statement.
Behind the news: Social bias — in data an d models, in the industry, and in society at large — has emerged as a major issue in the machine learning community.
- Concerns over bias in AI reignited last week after a generative model called Pulse converted a pixelated picture of Barack Obama, who is Black, into a high-resolution image of a white man.
- The compilers of ImageNet recently culled labels — also based on WordNet — deemed biased or offensive from the dataset’s person subtree.
Why it matters: Social biases encoded in training data become entwined with the foundations of machine learning. WordNet transmitted its derogatory, stereotyped, and inaccurate information to Tiny Images, which may have passed them along to countless real-world applications.
We’re thinking: As AI practitioners, we have a responsibility to re-examine the ways we collect and use data. For instance, Cifar-10 and Cifar-100 were derived from TinyImages. We’re not aware of biases in those datasets, but when one dataset’s bias may propagate to another, it’s necessary to track data provenance and address any problems discovered in an upstream data source. Recent proposals set standards for documenting models and datasets to weed out harmful biases before they take root.
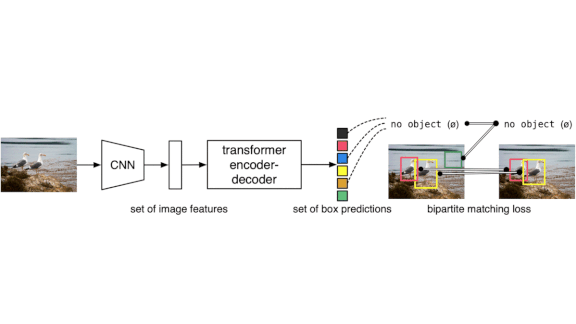
Computer Vision Transformed
The transformer architecture that has shaken up natural language processing may replace recurrent layers in object detection networks.
What’s new: A Facebook team led by Nicolas Carion and Francisco Massa simplified object detection pipelines by using transformers, yielding Detection Transformer (DETR).
Key insight: Images can show multiple objects. Some object detection networks use recurrent layers to predict one object at a time until all objects are accounted for. Language models use transformers to evaluate a sequence of words in one pass. Similarly, DETR uses them to predict all objects in an image in a single process.
How it works: DETR predicts a fixed number of object bounding boxes and classes per image. First, it extracts image features using convolutional layers. Then transformers predict features associated with regions likely to contain objects. Feed-forward layers process the object features into classes and bounding boxes. (“No object” is a possible class.)
- The transformers generate object bounding boxes and labels as a sequence, but their order is arbitrary.
- The loss function uses the Hungarian algorithm to match each object class (except “no object”) with a unique label. This makes predicting anchors (box center points) and complicated matching algorithms unnecessary.
- During training, each transformer layer makes its own prediction. Evaluating this output ensures that all transformers learn to contribute equally — a technique borrowed from language models that’s not available with recurrent layers. The additional loss function especially helps the system predict the correct number of objects.
Results: The researchers pitted DETR against Faster R-CNN on the canonical object detection dataset Coco. At model sizes of roughly 40 million parameters, DETR bettered Faster R-CNN’s average precision, a measure of true positives, from 0.402 to 0.420. And DETR did it faster, spotting objects at 28 images per second compared to Faster R-CNN’s 26 images per second.
Why it matters: Transformers are changing the way machine learning models handle sequential data in NLP and beyond.
We’re thinking: What happened to the Muppet names for transformer-based models? Fozzie Bear is available.
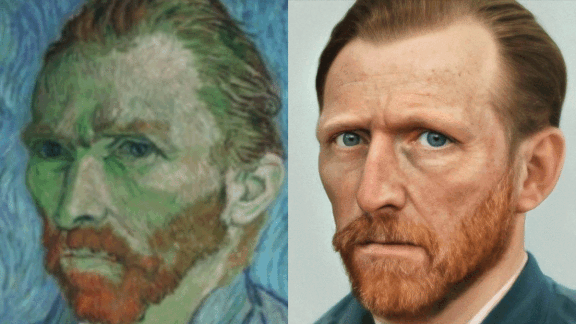
Photos From Before Photos Existed
An artist is using deep learning to create realistic portraits of historical figures like Napoleon Bonaparte, Vincent Van Gogh, and England’s first Queen Elizabeth.
What happened: Combining technical and artistic ingenuity, Dutch photographer Bas Uterwijk is generating photorealistic images of people who lived before photography was invented.
How it works: Uterwijk uses Artbreeder, a web-based tool that lets users upload images and “breed” them to create hybrid versions.
- Artbreeder’s portraits module generates images using StyleGAN-2. The model was fine-tuned on photographs, paintings, and drawings for training in human faces and the behavior of light in photography.
- Artbreeder tends to average out a face’s distinctive features, Uterwijk told The Batch. So he pre-processes the input using Photoshop to amplify or de-emphasize certain features. “You learn when to exaggerate or take stuff out before you feed it to the network,” he said. Then he adjusts the output’s skin color, eye shape, and age.
- For his Napoleon portrait, Uterwijk integrated a large number of historical portraits, drawings, and sculptures. That made the image particularly authentic, he said, unlike his efforts to portray Jeanne d’Arc or Cleopatra, whose likenesses are almost wholly invented by earlier artists.
- Uterwijk has also created realistic images of fictional characters like Frankenstein, Michelangelo’s David, and the Marine from the video game Doom.
Behind the news: Artbreeder is one of several creative tools that take advantage of the uncanny ability of GANs to replicate and manipulate visual details.
- Commercial apps like Prisma and Visionist render photos from social media in styles like impressionism, pointillism, and mosaic.
- Deep Dream, a web tool for creating psychedelic art, started as a visualization technique to help engineers understand how computer vision models perceive images.
Why it matters: Uterwijk’s AI-fueled artistry helps to humanize historical personalities and fictional characters, too. A similar approach could help filmmakers, museums, and video game developers bring history to life.
We’re thinking: We’re waiting for photorealistic renderings of the characters in XKCD.
A MESSAGE FROM DEEPLEARNING.AI
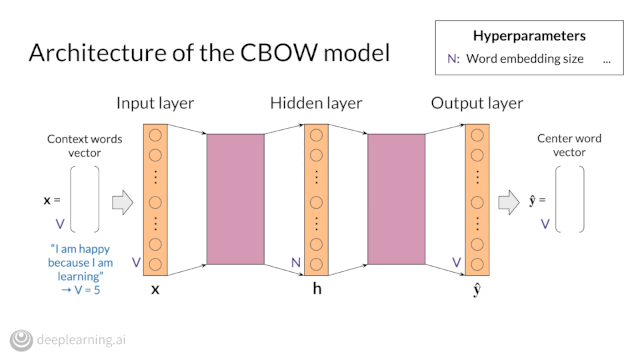
Check out our new Natural Language Processing Specialization on Coursera! Courses #1 and #2 are now available for learners. Enroll today
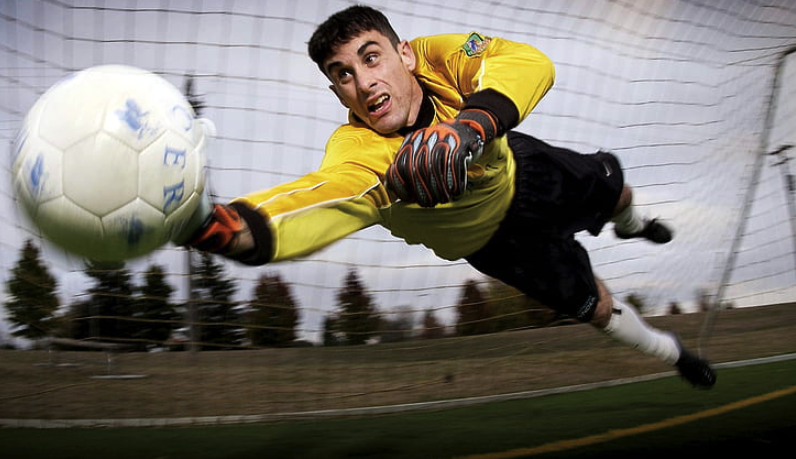
Game Changer
Football clubs are turning to computer vision for winning insights.
What’s new: Acronis, a Swiss cloud storage and security company, offers AI services designed to give a boost to some of the world’s top football clubs (soccer teams, to Americans), Wired reported.
Eyes on the ball: The company stores training and match video for professional teams including London-based Arsenal, Manchester City, and Inter Milan. An internal group devoted to machine learning for sports is using the data to train AI tools aimed at improving gameplay and marketing.
- Game analysis applications track players’ movements and analyze tactics, an Acronis spokesperson told The Batch.
- Acronis didn’t specify which teams use which services, but it said that a team in the English Premier League uses its tools to analyze ticket sales, weather, and other factors to predict match attendance.
- The company plans to use gaze detection on stadium surveillance footage to spot when fans are paying more attention to the game. Stadiums can use the information to pick the best moments to show ads on their video screens.
Behind the news: Nearly two decades after Michael Lewis’ book Moneyball: The Art of Winning an Unfair Game revealed the use of data analytics in baseball, sports are becoming an active playing field for AI.
- Basketball teams including the NBA’s Golden State Warriors and France’s FFBB league use AI-enhanced video systems from Keemotion to analyze play for coaches and track the action for broadcasts.
- Israel-based Minute.ly’s video system identifies the most exciting moments in a sportscast.
- Japanese tech giant Fujitsu created a tool that uses laser data to track gymnasts’ motion.
Why it matters: Once the four-minute mile was a breakthrough. Now it’s par for the course. Machine learning is set to help athletes continue to upgrade their own state of the art.
We’re thinking: For those of us who aren’t particularly athletic, it’s nice to know that we can help score goals by running our fingers across a keyboard!
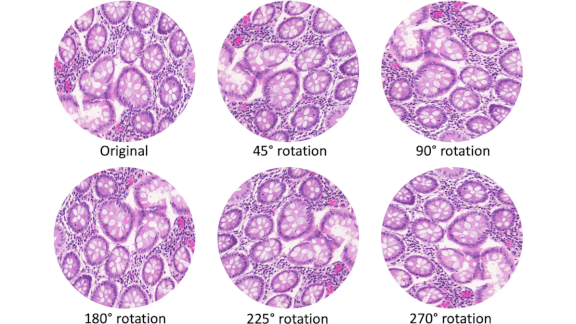
Seeing Straight at Any Rotation
A cat rotated by any number of degrees is still a cat. It takes a lot of rotated training images to teach convolutional filters this simple fact. A new filter design has this common-sense knowledge built-in.
What’s new: Simon Graham led a team at the University of Warwick to create the Dense Steerable Filter CNN (DSF-CNN), a convolutional neural network that can see a picture in various rotations and generate consistent output.
Key insight: Pixels are tiny squares. Rotating them by increments other than 90 degrees results in distortion and lost information. Earlier work developed so-called steerable filters that eliminate distortion by subdividing their weights and recombining them to create the desired rotation. DSF-CNN builds on that work by incorporating dense connections that improve performance and data efficiency.
How it works: DSF-CNN operates slightly differently on the input and hidden layers. At the input, it extracts initial features for each of several rotational angles (illustrated by figure b above). In the hidden layers, it extracts more complex features at each angle (figure c).
- The researchers evaluated systems with 4, 8, or 12 filters corresponding to rotations of 90, 45, or 30 degrees respectively. The filters share weights, so the model can look at one image from a number of perspectives.
- At the input, DSF-CNN extracts features from each channel of an image by applying a set of filters rotated through various angles. Each filter’s action multiplies the number of channels.
- In the hidden layers, the system re-examines features from previous layers. To keep memory requirements from ballooning, hidden layers apply one filter per channel rather than the full complement.
- For more efficient training, the authors implemented dense connections by concatenating related features from multiple layers.
Results: The researchers tested the 8-filter model on pathology slides, since medical images come in a variety of orientations. DSF-CNN achieved 0.975 area under the curve (AUC), a measurement of true positives and false positives where 1 is perfect. That score beat a state-of-the-art CNN (0.949 AUC) and rotational G-CNN (0.968). DSF-CNN also turned in superior performance on two other medical image datasets.
Why it matters: Rotational symmetry gives neural networks fits. DSF-CNN doesn’t cover every angle, but it vastly reduces the data requirements and simplifies training them to recognize that rotated images are equivalent.
We’re thinking: Rotational symmetry can cause trouble for humans, too. Check out the Thatcher Effect.