Dear friends,
I was dismayed on Monday to read that the U.S. is suspending the H1-B visa program at least through the end of the year. This effort to discourage immigration can only bring distress to workers from other countries and harm to the U.S.
H1-B visas allow U.S. companies to bring in talent from around the world, enriching both their business and the economy. People from many different countries have been central to U.S. innovation in AI (see “Mapping AI’s Talent Pipeline” below).
To me, H1-B holders aren’t just “workers.” They are my friends, students, and collaborators, and it pains me to see them facing the stress and uncertainty that comes with sudden, arbitrary shifts in immigration policy.
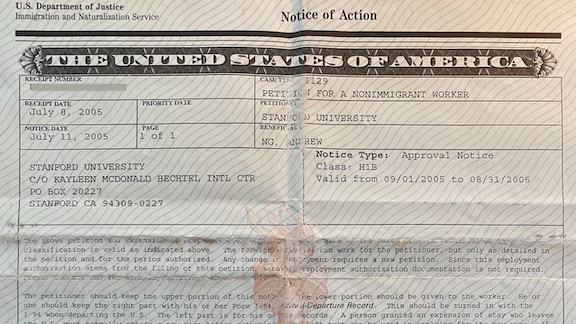
Stanford University sponsored my H1-B visa many years ago, which enabled me to teach and do research there. It feels deeply unfair to deny the same opportunities to the next generation. We should do whatever we can to attract top talent, not turn it away. As a planet, we should be working to empower individuals to do their best work, wherever they may end up doing it.
Through education, I remain committed to creating opportunities to learn and grow for as many people as I can. I hope the AI community will continue to transcend national borders and come together to build AI for the betterment of all.
Keep learning!
Andrew
News
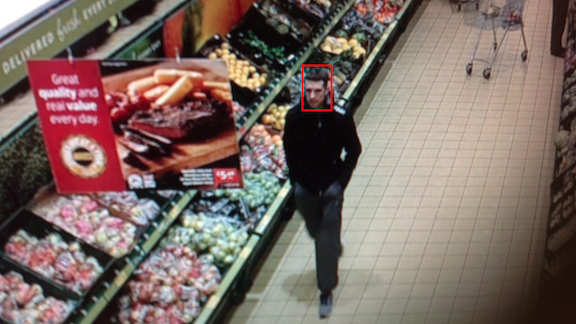
Tech Giants Face Off With Police
Three of the biggest AI vendors pledged to stop providing face recognition services to police — but other companies continue to serve the law-enforcement market.
What’s new: Amid protests over police killings of unarmed Black people in the U.S., Amazon imposed a one year moratorium on licensing its Rekognition technology to police departments, and Microsoft announced a similar hiatus. Both said they would re-enter the market if the government imposed limits on police use of the technology. IBM exited the face recognition market altogether.
Demand, meet supply: The big AI companies are highly visible, but most law enforcement agencies get the technology from lesser-known firms, the Wall Street Journal reported.
- Clearview AI has 2,400 police customers in the U.S. and Canada.
- NEC licenses face recognition to 20 law enforcement agencies.
- Ayonix, iOmniscient, and Herta Security each serve a handful of U.S. law enforcement agencies.
- The French company Idemia works with the New York Police Dept., the U.S. State Dept., and the U.S. Transportation Safety Administration as well as the European and Australian governments.
Why it matters: Concern over fairness in law enforcement has renewed worries that unfettered use of face recognition leads to miscarriages of justice. Research spearheaded by MIT Media Lab researcher Joy Buolamwini showed that commercially available systems consistently misclassified women and people with darker complexions. A study by the American Civil Liberties Union found that Amazon’s system erroneously matched mugshots with the faces of 28 members of the U.S. Congress. Some police departments have misused the technology in ways that experts say could lead to mistaken arrests.
We’re thinking: It’s great to see the big AI providers exercising responsibility. Now we need prudent regulation and auditing mechanisms geared to protect civil rights and support social justice.
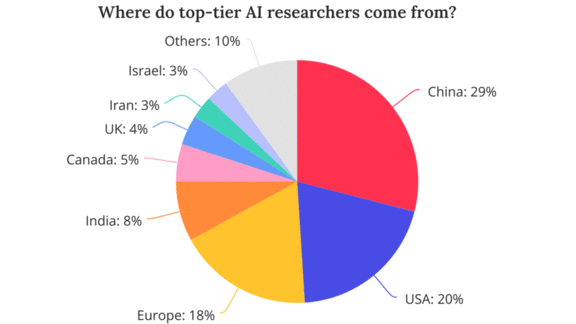
Mapping AI’s Talent Pipeline
China launches most of AI’s top researchers, but the U.S. is their number-one destination.
What’s new: U.S.-based research group MacroPolo published the Global AI Talent Tracker. The report traces international trends in education and employment among elite engineers.
Findings: The study tracked the locations of 675 high-performing AI practitioners through undergraduate studies, graduate school, and employment.
- Nearly 30 percent of the AI pros earned their undergraduate degree in China. Some 20 percent earned theirs in the U.S.
- More than half relocated to another country after their undergraduate education. The U.S. was their favorite destination by far.
- Over half of Chinese-educated researchers went to the U.S. for graduate school or employment.
- While the U.S. and China dominated the educational and employment pipeline, a significant number of undergraduates came from India, Europe, and Canada.
Behind the news: MacroPolo sought to sample top AI talent by selecting its cohort at random from authors whose papers were accepted to NeurIPS 2019, one of the most prestigious and selective conferences in the field. The report’s conclusions align closely with previous studies that also used conference acceptance to track where AI researchers were educated and employed.
Why it matters: Developing AI is a global project. Collaboration and freedom of movement are essential to progress.
We’re thinking: The recent U.S. suspension of H1B visas will shatter dreams and disrupt lives. But the MacroPolo data shows that it will also be very damaging to U.S. innovation in AI. At a time when some countries are trying to make immigration harder, the AI community must redouble its effort to make sure that people from all over the world are welcome and able to contribute.
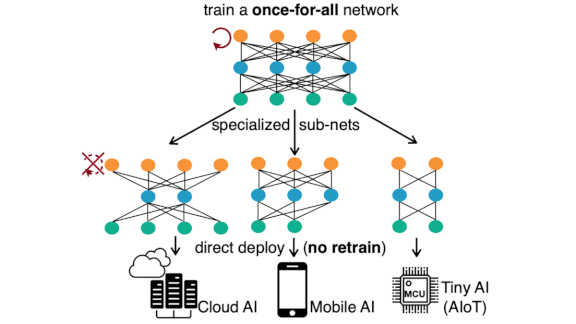
Build Once, Run Anywhere
From server to smartphone, devices with less processing speed and memory require smaller networks. Instead of building and training separate models to run on a variety of hardware, a new approach trains a single network that can be adapted to any device.
What’s new: Han Cai and researchers at MIT developed Once-for-All (OFA). This method trains a single large model and derives subnetworks — subsets of the original model’s weights — that perform well on less powerful processors.
Key insight: Typical pruning methods downsize neural networks one at a time by reducing, say, the size and number of convolutional filters and then fine-tuning the smaller model. It’s more efficient to extract and fine-tune a fleet of progressively smaller models in a single process.
How it works: OFA extracts subnetworks by varying the parent network’s number of layers, number of filters per layer, filter sizes, and the input resolution. The researchers constrained each of these factors to a predetermined set of values that allow up to 1019 possible subnetworks.
- OFA trains the original network, then randomly samples a slightly smaller version. Then it fine-tunes both.
- It repeats this procedure with ever smaller subnetworks until it arrives at the smallest allowable version.
- OFA randomly samples and evaluates 10,000 subnetworks. The results constitute a dataset that represents model performance at a given size.
- Using the new dataset, OFA trains another network to predict the accuracy of any subnetwork, so it can select the best network of a given size.
Results: The authors compared OFA with a variety of neural architecture search methods suitable for finding models for mobile devices. The popular NASNet-A required 48,000 hours to generate the smallest model, and it would require that time again to generate another one optimized for different constraints. OFA’s baseline model required 1,200 hours to find all models. They also compared OFA to MobileNetV3-Large, the state-of-the-art image recognition network for mobile devices. The OFA model that ran on similar hardware achieved 76.9 percent top-one accuracy on ImageNet compared to MobileNetV3’s 75.2 percent. The most accurate neural search method the researchers considered, FBNet-C, required roughly half as much time as OFA to generate a single, less accurate model, but much more time to generate the second.
Why it matters: OFA produces equivalent models of many sizes in slightly more time than it takes to train the original large models. In situations that require deploying a given network to heterogenous devices, this efficiency can translate into big savings in development time and energy consumption.
We’re thinking: Smart speakers, watches, thermostats, pacemakers — it’s inevitable that neural networks will run on more and more heterogenous hardware. This work is an early step toward tools to manage such diverse deployments.
A MESSAGE FROM DEEPLEARNING.AI
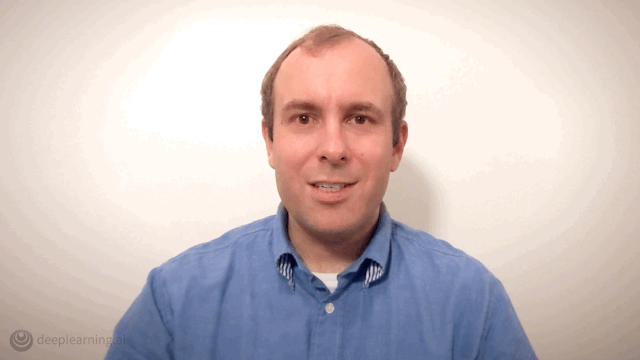
We’ve launched our much-anticipated Natural Language Processing Specialization! Courses 1 and 2 are live on Coursera. Enroll now
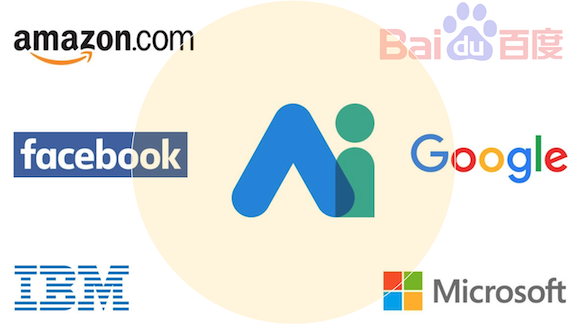
Baidu Leaves Partnership on AI
Baidu backed out of a U.S.-led effort to promote ethics in AI, leaving the project without a Chinese presence.
What’s new: The Beijing-based search giant withdrew from the Partnership on AI, a consortium of businesses, nonprofits, and research organizations that promotes cooperation on issues like digital privacy and algorithmic bias, Wired reported.
Mixed Motivations: Baidu joined the organization in 2018 as its first and only Chinese member. Other members include Amazon, Apple, Facebook, Google, and Microsoft as well as organizations like the U.N. and Human Rights Watch.
- Baidu explained its decision as a response to the partnership’s high membership fees and its on financial woes. Wired estimates that for-profit companies pay a few hundred thousand dollars for membership. Baidu’s 2019 revenue was $15.4 billion.
- Experts believe that Baidu’s departure had more to do with rising tensions between the U.S. and China.
Behind the news: Much of the Partnership on AI’s work involves research and education. Recent projects include a symposium on bias in machine learning, a study of how organizations can deploy explainable AI, and an interactive blog that explains face recognition to the general public. The group doesn’t lobby politicians directly, but it does aim to make its research relevant to policymakers.
Why it matters: The U.S. and China are the world’s top AI powerhouses. Baidu’s exit from the organization will affect its ability to influence AI globally toward fairness and justice.
We’re thinking: The AI community spans many nations, and many bonds hold it together. Let’s keep building bridges and working to ensure that AI is used for the common good.
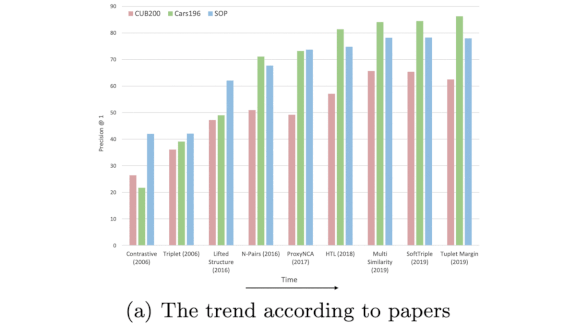
Misleading Metrics
A growing body of literature shows that some steps in AI’s forward march may actually move sideways. A new study questions advances in metric learning.
What’s new: Kevin Musgrave and researchers at Cornell Tech and Facebook AI re-examined hallmark results in models that learn to predict task-specific distance metrics, specifically networks designed to quantify the similarity between two images. They found little evidence of progress.
Key insight: Researchers have explored metric learning by experimenting with factors like architectures, hyperparameters, and optimizers. But when those factors aren’t held constant, comparisons with earlier approaches can’t be apples-to-apples. Improved results often reflect advances in the surrounding components (such as hyperparameter tuning), not in the metric learning algorithm itself.
How it works: Models that assess similarity between images typically extract features and predict a distance between them. The distances may be learned through a metric loss function, while features are often extracted from pre-trained networks. The authors reviewed 12 of the most popular papers on this topic. They point out common causes of invalid comparisons and present a new approach that levels the playing field.
- Several papers compare a ResNet50 to a GoogLeNet trained using different methods. A ResNet50 is larger and outperforms a GoogLeNet on other image processing tasks, so it’s no surprise the ResNet50 performs better at metric learning.
- Many researchers don’t use a validation set but still tune hyperparameters. Presumably, they chose hyperparameter values based on the models’ performance on test sets — a big no-no.
- These two flaws, and a list of smaller mistakes, inspired the authors to propose a consistent test bed for metric learning research. Their benchmark calls for BN-Inception networks, RMSprop optimizers, and cross-validation for hyperparameter search.
Results: The authors reproduced and benchmarked many past approaches on the CUB200, Cars 196, and Stanford Online Products datasets. Controlling for confounding variables, their analysis shows that metric learning hasn’t improved since 2006 (as shown in the plots above).
Why it matters: Image similarity is a key component of many products (such as image-based search). Knowing what really works is key to helping practitioners build useful applications as well as drive further research.
We’re thinking: Metric learning still has a distance to go.