Dear friends,
Last week, I asked readers to tell me what they’re doing to address the Covid-19 pandemic. Many of you wrote to say you’re taking actions such as shopping for neighbors, making masks, and creating posters that promote Covid-safe practices (see the campaign by Luter Filho, a creative director and designer in Berlin, below).
Several members of the deeplearning.ai community are rising to meet the challenges of Covid-19 by building AI and other software projects:
- Arturo MP, a natural language engineer in Toronto, along with friends and associates organized a Spanish-language Covid-19 news archive and Twitter feed to address the shortage of information in languages other than English.
- Hermes Ribeiro Sant Anna, a machine learning engineer in SĂŁo Paulo, Brazil, built a web app that highlights surfaces prone to coronavirus contamination by human touch.
- Fernanda Wanderley, a data scientist in SĂŁo Paulo, Brazil, helped develop free X-ray interpretation software (in Portuguese) to triage Covid-19 patients.
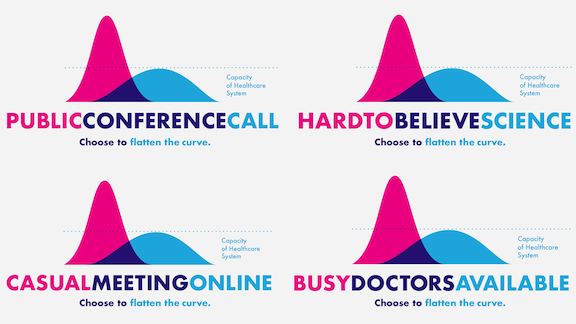
- Oscar Alexander Kirschstein Schafer at the Universidad AutĂłnoma de Madrid is organizing open hackathons to come up with ways to fight the pandemic.
- Josh Brown-Kramer, a data scientist in Lincoln, Nebraska, is testing people for Covid-19 in small groups and testing individuals only if the group that includes them tests positive. This pooling approach theoretically improves test throughput by 50 percent, he writes, although he has not received independent verification.
- Federico Lucca in Trento, Italy, is working with the University of Trento on ultrasound interpretation software to recognize lung problems related to Covid-19.
It’s exciting to see the deeplearning.ai community helping to keep families, neighborhoods, and towns healthy. Your efforts are an inspiration as I develop my own projects to keep the virus at bay and help everyone heal and rebuild. In the future, we will look back on these days with sadness, but also with pride that our community’s creativity and ingenuity can have a positive impact on a global scale.
Stay safe and keep learning!
Andrew
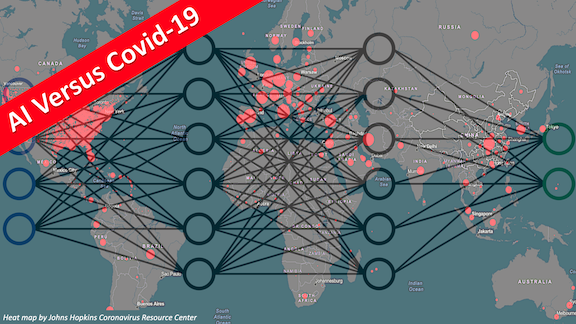
New Machine Learning Resources
The data science community is providing tools and datasets to help fight the pandemic. Below you’ll find the most valuable resources we’ve come across in the past week. If you want to recommend relevant resources, please let us know at thebatch@deeplearning.ai.
- Help with Covid: Have a project in mind or looking to contribute to one? Helpwithcovid.com is a community-driven platform that matches volunteers with Covid-19-related projects.
- Covid Healthcare Coalition Resource Library: This private-sector effort to compile and coordinate research includes a convenient library of resources. The user interface offers filters for categories such as public health, education, and modeling.
- South Korean Case Data: Most publicly available case datasets for Covid-19 provide only aggregate statistics, limiting their utility in research. The Republic of Korea provides access to an anonymized nationwide Covid-19 patient dataset that includes a five-year medical history of each individual. To protect patient privacy, the service runs researchers’ code and returns the results.
News
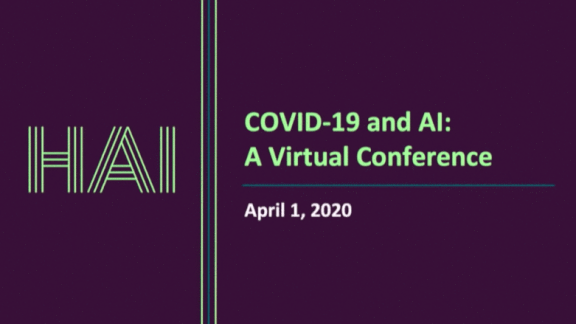
Online Conference Goes Antiviral
AI experts convened to discuss how to combat the coronavirus crisis.
What’s new: An online conference hosted by Stanford University’s Institute for Human-Centered AI explored how machine learning is being deployed to address this pandemic — and prepare for the next one. You can watch the video here.
The agenda: Nearly two dozen experts in fields as diverse as machine learning and public health delivered updates on topics from containing the pandemic to finding treatments. A few notable presentations from the six-hour conference:
- Epidemiology: Given the many people who fall ill but don’t enter a hospital, not to mention those who never show symptoms, it can be maddeningly difficult to track how the disease spreads and how many it kills. Lucy Li of the Chan Zuckerberg BioHub used a branching model to estimate how many people have been infected. Each time a virus infects a host, it mutates slightly. Analyzing viral DNA extracted from each known patient, the model uses the rate of mutation to interpolate how many other people the virus passed through along the way. According to Li’s estimates, 87 percent of all Chinese cases and 95 percent of total cases have gone undetected.
- Social Distancing: Stanford pediatrician C. Jason Wang described how Taiwan’s Central Epidemic Command Center tracked individual Covid-19 cases and enforced social distancing rules. Activated in response to the epidemic, the data analytics hub uses GPS, health insurance records, and immigration data to track infections and alert individuals who may have been exposed.
- Treatment: Creating new drugs from scratch takes a lot of time. So Stefano Rensi and colleagues in Stanford’s Department of Bioengineering searched for existing compounds to fight Covid-19. They used natural language processing to sift the medical literature for clues about how the novel coronavirus delivers its payload to a cell’s nucleus. Then they used a model that predicts protein structure to look for proteins that might inhibit this process. They found 15 known drug candidates that contain this protein, and they’re conducting their own trials to gauge their effectiveness.
Behind the news: Infectious disease expert Dr. Michele Barry explained that machine learning was critical to keeping infection rates low in Singapore, South Korea, and Taiwan. All three countries deployed the technology to encourage social distancing, move medical supplies where they were needed most, and keep the public informed.
Why it matters: Machine learning engineers and disease specialists have a lot to learn from one another. Conferences like this can bring them together and build lasting alliances that may result in tools for fighting future outbreaks.
We’re thinking: If you’re itching to join the fight against Covid-19, you can find a list of tools, datasets, and information just above the news in this issue of The Batch. Also: Stay at home and wash your hands!
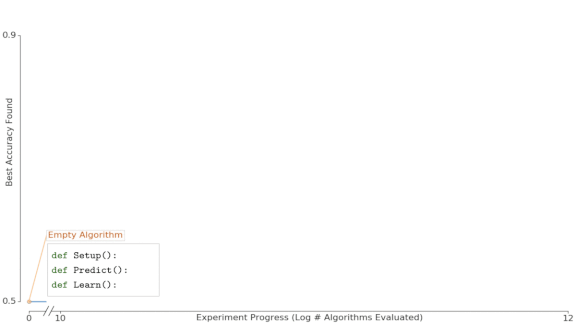
Beyond Neural Architecture Search
Faced with a classification task, an important step is to browse the catalog of machine learning architectures to find a good performer. Researchers are exploring ways to do it automatically.
What’s new: Esteban Real, Chen Liang, and their colleagues at Google Brain developed AutoML-Zero, an evolutionary meta-algorithm that generates a wide variety of machine learning algorithms to classify data. Applied to the small CIFAR-10 image dataset, it discovered several common deep learning techniques.
Key insight: Past meta-algorithms for machine learning constrain their output to particular architectures. Neural architecture search, for instance, finds only neural networks. AutoML-Zero finds any algorithm that can learn using high school-level math.
How it works: The researchers used AutoML-Zero to generate models for various resolutions of CIFAR-10.
- In the authors’ view, a machine learning model comprises a trio of algorithms: Setup initializes parameter values, Predict provides a scalar output given input vectors, and Learn updates weights based on the inputs, training labels, outputs, and current values.
- AutoML-Zero starts with a set of models with empty Setup, Predict, and Learn. It generates a population of models and evolves them for improved performance on a set of tasks.
- The meta-algorithm trains an instance of every model in each training iteration. It applies each model’s Predict and Learn to a task’s training set and evaluates performance on the task’s validation set.
- It culls a random subset of the population and mutates the best-performing model by adding an operation exchanging one operation for another, or switching input variables. The mutated model replaces the oldest model in the subset.
Results: AutoML-Zero regularly generated models that achieved 84 percent accuracy on CIFAR-10, compared to only 82 percent achieved by a two-layer, fully connected network. In the process, it rediscovered gradient descent, ReLu activations, gradient normalization, and hyperparameters.
Why it matters: The researchers estimate that, given AutoML-Zero’s wide-ranging purview, the chance of coming up with a model suitable for a CIFAR-10 classification task is vanishingly small (around 1 in 107 for linear regression, and 1012 if that line is offset by a constant). Yet it did so frequently — a demonstration of the meta-algorithm’s power to come up with useful architectures. If AutoML-Zero can find nearly state-of-the-art models on such a complex task, it may well be able to discover techniques that humans haven’t yet devised.
We’re thinking: CIFAR-10 was developed over a decade ago for machine learning experiments on the CPU-based neural networks of the day. We’re curious to learn how AutoML-Zero scales to larger datasets.
We’re not thinking: Today we have learning algorithms that design other learning algorithms. When will we have learning algorithms that design learning algorithms that design learning algorithms?
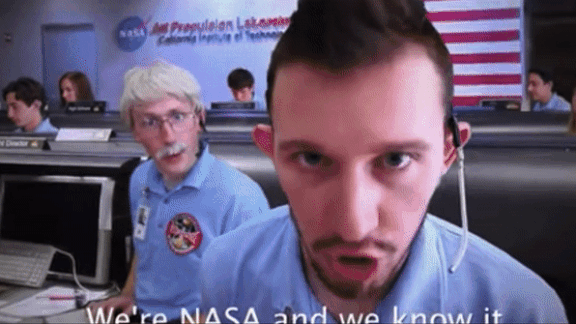
Robots to Hollywood: Call My Agent
Seeking a robot star for your movie, music video, or bat mitzvah? You need a new breed of talent scout.
What’s new: Ai-gen-cy is an artist management firm that exclusively represents robots, reports GeekWire. This video explains.
How it works: Seattle-based entrepreneurs Forest Gibson and Jared Cheshier believe that movie directors and event producers want to feature automatons but are put off by their diva-like needs: programmers, on-site technicians, and handle-with-care transportation. Ai-gen-cy aims to supply mechanical talent along with logistical and technical support.
- The company currently represents two robots: a pair of Boston Dynamics’ four-legged Spots. Gibson told The Batch it also owns two remote-controlled bots that support filming: a DJI RoboMaster and a Skydio 2 drone.
- The two partners train their stable to perform new behaviors in a simulated environment. They’re also exploring voice synthesis to boost their clients’ charisma.
- Ai-gen-cy had booked appearances for its robots ahead of its launch last week, but cancelled them due to Covid-19.
Behind the news: Gibson and Cheshier gained experience presenting robots on-screen in 2012, when they collaborated on a music video that set simulated footage of Nasa’s Curiosity rover to an electronic beat.
Why it matters: Anything that puts more robots in movies is fine by us.
We’re thinking: Tabloid coverage of robot superstars’ off-screen escapades should be interesting.
A MESSAGE FROM DEEPLEARNING.AI
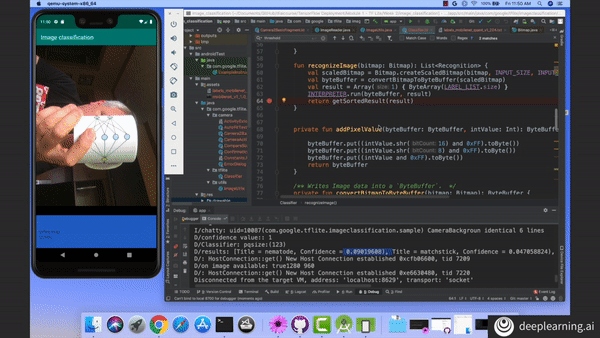
Test your image classification models with your phone’s camera! Learn how to deploy models with TensorFlow Lite in Course 2 of the TensorFlow: Data and Deployment Specialization. Enroll now
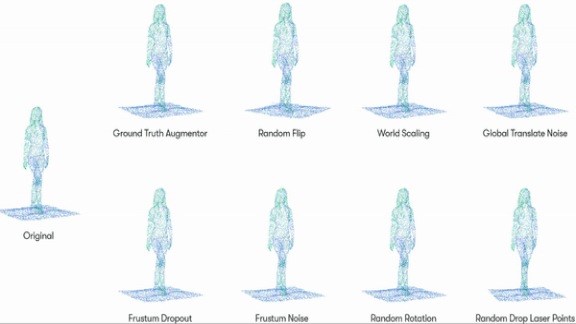
Data Augmentation in 3D
An unconventional approach to modifying data is cutting the amount of work required to train self-driving cars.
What’s new: Waymo unveiled a machine learning pipeline that varies lidar scans representing pedestrians and other objects. The company’s self-driving systems, in turn, learn from the augmented data, effectively giving the researchers a larger training dataset without collecting and labelling additional point clouds.
How it works: Waymo’s engineers started with two-dimensional data augmentation methods used to train object recognition models: flipping, rotating, color-shifting, and partially obscuring still images. Since lidar output generates three-dimensional points, Waymo developed ways to perform similar transformations while maintaining the geometrical relationships between points.
- The researchers collected pre-labeled 3D representations of pedestrians, cyclists, cars, and road signs from the Waymo Open Dataset.
- They developed eight modes of point-cloud distortion. For instance, one mode changed the object’s size, while another rotated it along its y-axis.
- Then they trained neural networks to generate these variations, creating new versions of each object in the dataset.
Results: Called Progressive Population-Based Augmentation, the method improved object detection with both small and large datasets. It increased data efficiency between 3.3- and 10-fold compared with training on unaugmented datasets.
Behind the news: Released in March, Waymo’s latest self-driving platform is based on an electric Jaguar SUV. Its sensor suite includes a 360-degree lidar on top, a 95-degree lidar near each head and tail light, plus cameras and radars.
Why it matters: The new system breaks ground in 3D augmentation. Waymo is effectively giving its system more examples of obstacles while saving the time, expense, and labor it would take to collect real-world samples.
We’re thinking: As long as social distancing keeps real drivers and pedestrians off the street, self-driving cars will need all the augmented data they can get.
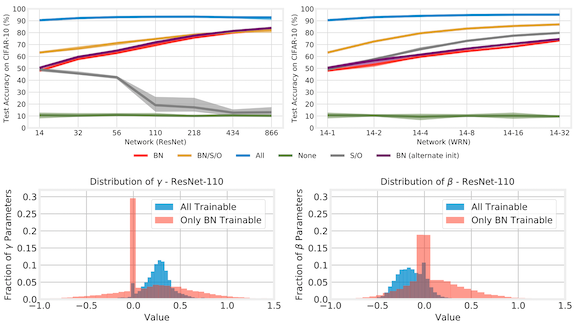
Outside the Norm
Batch normalization is a technique that normalizes layer outputs to accelerate neural network training. But new research shows that it has other effects that may be more important.
What’s new: Jonathan Frankle and colleagues at MIT, CUNY, and Facebook AI showed that batch normalization’s trainable parameters alone can account for much of a network’s accuracy.
Key insight: As it adjusts a network’s intermediate feature representations for a given minibatch, batch normalization itself learns how to do so in a consistent way for all minibatches. The researchers probed the impact of this learning by training only the batch normalization parameters, gamma (????) and beta (β), while setting all other parameters to random values.
How it works: The researchers trained variously sized ResNet and Wide ResNet models, which include batch normalization layers, on the CIFAR-10 image dataset.
- Batch normalization normalizes the output of intermediate layers according to their average and variance across a minibatch. Then it scales them by ???? and shifts them by β. The values of those variables are learned.
- After training batch normalization parameters only, the researchers found that nearly half of ???? values were close to zero. Pruning those values had a negligible impact on performance.
- Deep ResNets had much higher accuracy than wide ResNets with similar numbers of trainable Batch normalization parameters. Batch normalization is known to have greater impact on deeper networks, and apparently scaling and shifting do as well.
Results: Training all the parameters in a ResNet-866 yielded 93 percent accuracy, while training only ???? and β brought 83 percent accuracy. This finding is further evidence that networks can be accurate even with a large number of random weights.
Why it matters: Batch normalization is often standard procedure in deep learning, but previous studies failed to recognize the power of its trainable parameters. And batchnorm isn’t the only normalization method that scales and shifts parameter values; so do weight normalization and switchable normalization. Further research may illuminate the impact of these normalizations on network performance.
We’re thinking: Why batch normalization works has been a subject of heated debate since Sergey Ioffe and Christian Szegedy introduced it in 2015. The authors’ original explanation of “reducing internal covariate shift” sounded a bit like black magic. This work sheds light on the mystery.
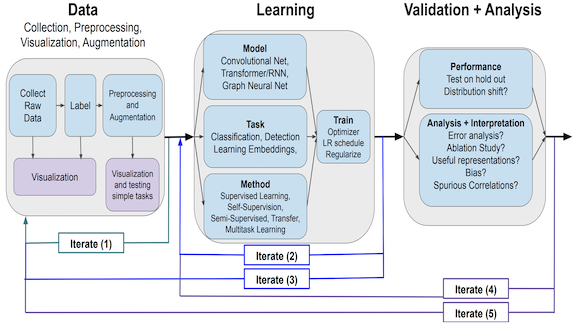
(Science) Community Outreach
Are your scientist friends intimidated by machine learning? They might be inspired by a primer from one of the world’s premier tech titans.
What’s new: Former Google CEO Eric Schmidt and Cornell PhD candidate Maithra Raghu school scientists in machine learning in a sprawling overview.
Scientific Revolution 2.0: Science produces mountains of data, and machine learning can help make sense of it. Schmidt and Raghu offer a brisk tour of architectures and techniques, explaining how neural networks have served disciplines from astronomy to radiography.
- Image classifiers are showing great potential in medicine, where they can predict, say, whether viruses appear in a picture from a cryo-electron microscope. Object detection has spotted individual cancer cells in microscope images, and semantic segmentation has differentiated various types of brain tissue in MRIs.
- Graph neural networks, which learn relationships between nodes and connections, have been used to analyze how atoms and bonds determine molecular structure. They’ve also been used to design molecules to match particular chemical properties.
- The qualities that make recurrent neural networks good at figuring out grammar helps them find patterns in a variety of sequential data. This includes finding patterns in gene sequences.
- Weakly supervised learning is handy for scientists with lots of data but few grad students to label and organize it. If has been applied widely in biomedicine, but also to track penguins in satellite photos.
- Reinforcement learning shows promise in accelerating simulations in astronomy, chemistry, climate science, high energy-density physics, and seismology.
Behind the news: Maithra Raghu isn’t as famous as her co-author, but her star is on the rise. Named among Forbes’ “30 Under 30” last year, she focuses on improving human-machine collaboration.
Why it matters: The range of mysteries that machine learning can help solve is limited by the number of scientists who are proficient in machine learning.
We’re thinking: We’d like to see more CEOs publish technical papers on arXiv.org!