Dear friends,
Many of us apply labels to ourselves that shape our identity. Some say, âIâm a sports fan,â and this attitude motivates behaviors such as cheering for the home team. Others identify themselves as introverts, extroverts, vegetarians, gamers, athletes, scientists, and/or engineers. Each label implies its own set of habits and activities.
I think itâs time for more of us to identify ourselves as life-long learners. To me, a life-long learner:
- Aspires to keep learning new things
- Seeks knowledge or skill beyond what would be immediately useful
- Invests time, energy, and money to learn new things
- Shares knowledge to help other lifelong learners
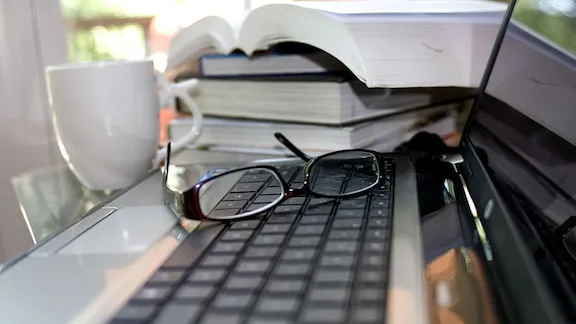
This is the best way to keep growing over your entire lifetime. Iâve seen numerous people proactively learn about new technologies or gain skills in everything from product management to personal health, and develop as individuals as a result. They seem happier, and Iâm sure they contribute more to their communities.
Every weekend I spend several hours reading or taking online courses. This learning helps me do my work better, but I enjoy it so much that Iâd do it even if it didnât affect my work at all.
The world is changing faster than ever, driven by technological change. So humanity needs a lot more lifelong learners to make sure we keep up. I hope youâll join me in proudly telling others, âIâm a lifelong learner!â
Keep learning,
Andrew
News
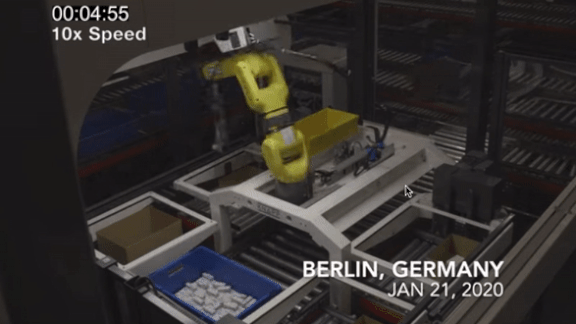
Packing Robots Get a Grip
Robots are moving into a job that traditionally required the human touch.
Whatâs new: A commercial warehouse that ships electrical supplies deployed AI-driven robotic arms from Covariant, a high-profile Silicon Valley robotics firm. Trained using a hybrid of imitation and reinforcement learning, the new machines are far better than earlier bots at sorting items into boxes.
How it works: Robots have been picking objects off conveyor belts for years, but they generally handle only identical items. Covariantâs approach, which uses a single neural network for all objects, enables an arm equipped with a camera and suction gripper to manipulate around 10,000 different items (and counting). The system can share skills with other arms, including those made by other companies.
- Training starts with attempts at few-shot adaptation. In many cases, the robot can learn from a limited number of attempts, the company told IEEE Spectrum.
- For more intensive training, an engineer wearing virtual reality gear uses hand-tracking hardware to control the arm in a simulated environment. The model learns to mimic the motion.
- The model stores basic movements, then hones them using reinforcement learning in a variety of simulated situations.
- The team then uses behavioral cloning to transfer the robotâs learned skills into the real world.
Behind the news: Co-founded by UC Berkeley AI professor Pieter Abbeel (watch our interview with him here), Covariant has raised $27 million from backers including deep learning pioneers Yann LeCun and Geoffrey Hinton as well as Google AI chief Jeff Dean.
Why it matters: More than half of warehouse logistics companies could face labor shortages in the next five years, thanks to the jobâs tedium and low wages. Market analysts expect automatons to pick up the slack.
Weâre thinking: Will robots figure out how to ship a RAM stick without a cubic meter of styrofoam peanuts in a box the size of a washtub?
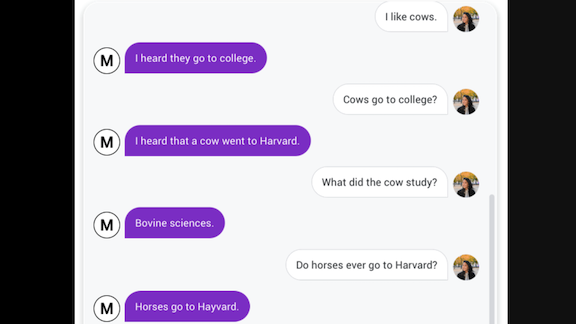
Bot Comic
Androids may not dream of electric sheep, but some crack jokes about horses and cows.
Whatâs new: Meena, a 2.6-billion parameter chatbot developed by Google Brain, showed impressive conversational ability, discussing a variety of topics. In one exchange, it unexpectedly sprinkled in some barnyard humor: It commented that âhorses go to Hayvard,â not Harvard. The phrase didnât appear in the training data, but the word Hayvard did appear once, a pun after a mention of horses, according to a company spokesperson. The bot followed up with another farm-animal pun, noting that its interlocutor tried âto steer [the conversation] elsewhere.â The first-ever AI-generated dad joke?
How it works: Google engineer Daniel De Freitas Adiwardana told us how, in training on 341 gigabytes of public social media conversation, Meena might have developed a sense of humor. Straight from the horseâs mouth, as it were:
- âAt a high level, when the model is training, itâs required to try to predict a lot of sequences of words, all at the same time. So itâs forced to come up with strategies that solve all these prediction problems at once.
- âIn the beginning of the convergence, when the perplexity is still high, these greedy choices lead to doing things like repeating common words, like âthe the the the.â It lowers its loss function value that way.
- âOver time, it learns new ways to cheat that lower the loss even further, like repeating what the other person said (âdo you like pizza?â > âDo you like pizza?â). Then it makes a twist on that, using repetitions (âdo you like pizza?â > âI like pizza, I like pizzaâ), contradictions (âdo you like pizza?â > âI like pizza, I donât like pizzaâ), and/or added conjunctions (âdo you like pizza?â > âI like pizza, but I donât like pizzaâ).
- âEventually it gets to something that is still cheating, in a sense, but much more sensible, like (âdo you like pizza?â > âI like pizza, but I try not to eat it everydayâ). Maybe no one said that sentence exactly, but it sort of looks like something a lot of people said.
- âThe empirical and hand-wavy moral of the story is that, as it gets harder to make learning progress, the cheating gets more sophisticated. One of possibly many views is that the cow and horse jokes are just a pretty sophisticated form of cheating, which many people would start to feel comfortable calling generalization.â
Behind the news: Efforts to give AI a sense of humor have met with limited success. One of the most impressive is a jokebot that writes captions for cartoons in The New Yorker. That model separates the normal aspects of a picture (for instance, a salesman showing off a new car) from oddball elements (the car has cat-like legs), then writes a caption based on the contrast. But even its best efforts (âjust listen to that baby purrâ) are more intriguing â because they were written by a computer â than side-splitting.
Why it matters: Humor affects us in deep and subtle ways. A Tina Fey-level bot may be out of reach, but a funny bone would be a valuable feature in an empathetic AI.
Weâre thinking: Weâd like to see a football game between Hayvard and Dartmooth.
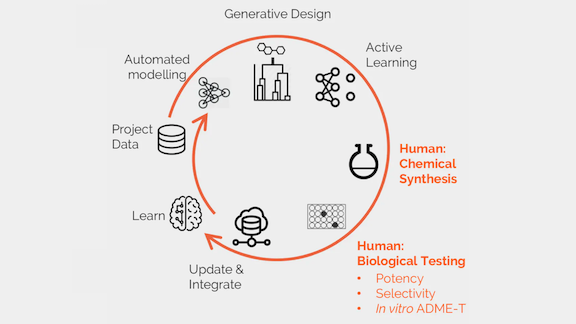
AI Tackles OCD
A drug designed by AI has been approved for testing in humans.
Whatâs new: A UK startup focused on automated drug discovery teamed up with a Japanese pharmaceutical company to produce a new medicine for obsessive compulsive disorder. The compound, known as DSP1181, is designed to take effect more quickly and last longer than existing treatments. Japanese authorities cleared it for a clinical trial.
How it works: Exscientiaâs drug-discovery platform can start with a biological target known to influence a particular medical condition.
- In this case, the target was a tiny cellular structure that, when stimulated, releases the hormone serotonin.
- The platform drew on databases of DNA sequences, protein structures, and drug actions to generate molecules likely to stimulate the serotonin-producing machinery.
- The model also scoured scientific literature, patent databases, and studies of genetic toxicology to gauge the candidatesâ likely impact.
- Exscentiaâs system likely shaved a few months off the usual discovery process, wrote Derek Lowe, a chemist at Novartis Institutes for BioMedical Research, in a blog post for Science.
Why it matters: Pharmaceutical companies invest upward of $2.6 billion to develop a single drug, and it can take three to six years to find a compound thatâs viable for testing in humansâ with no guarantee that it will prove safe and effective. Automating even small parts of the process can save big money. Thatâs one reason why Exscientia is one of nearly 200 companies worldwide using AI to find new drugs.
Weâre thinking: Â AI is no magic bullet for drug discovery. But cutting the enormous cost of development would enable pharma companies to study more molecules and potentially to bring more medicines to market.
A MESSAGE FROM DEEPLEARNING.AI
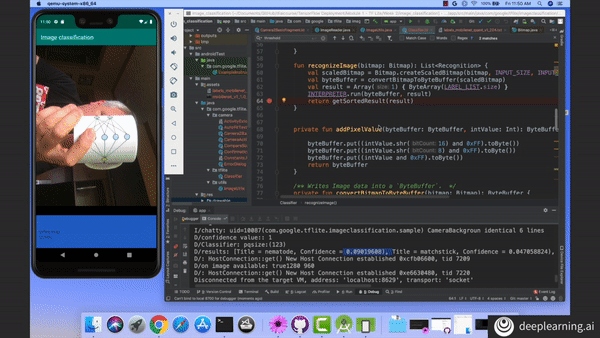
Test your image classification models with your phoneâs camera! Learn how to deploy models with TensorFlow Lite in Course 2 of the TensorFlow: Data and Deployment Specialization. Enroll now
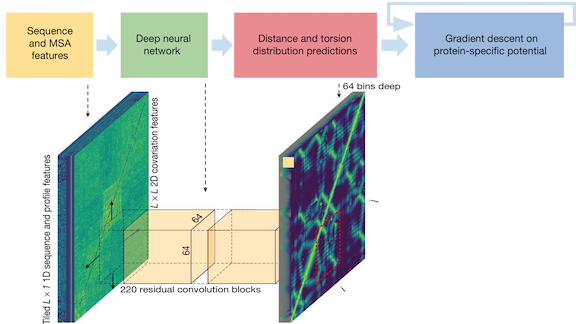
Protein Shapes Revealed
A proteinâs biological function depends largely on its three-dimensional shape, but deducing its shape from its sequence of amino acids has been a longstanding problem. Researchers at DeepMind reveal how they used deep learning to solve the puzzle.
Whatâs new: Andrew Senior and colleagues released long-awaited details about AlphaFold, a protein-folding model that wowed experts in a high-profile competition in late 2018. The paper is behind a paywall. This video offers some details.
Key insight: Research has shown that protein shapes are determined by the proximity of essential portions, or residues, of amino acids. The researchers found likely shapes by optimizing over possible structures that keep residues close to one another. Earlier methods predict whether residues are in contact with one another. AlphaFold predicts the distances and angles between residues, making the optimization easier.
How it works: AlphaFold extracts features from an input protein sequence, predicts relationships between residues, and uses those predictions to find the proteinâs likely shape.
- The feature extractor compares the input sequence with sequences in a protein database. It represents relationships between amino-acid pairs based on the similarities it finds.
- The features feed a CNN trained on a dataset of 3D protein structures, which predicts the distribution of distances and angles between residues.
- The model infers the proteinâs physical stability based on the distances and angles. The physical stability equation is differentiable, so the predicted structure can be optimized by gradient descent. The most stable structure is the final output.
Results: At the 2018 CASP13 conference, AlphaFold predicted 24 out of 43 previously unknown protein shapes with high accuracy. The next-best model achieved 14 predictions of similar accuracy.
Why it matters: The ability to determine protein structures could have wide-ranging impacts on drug discovery, countering neurodegenerative diseases, and more. Stay tuned for further progress when CASP14 convenes in April.
Weâre thinking: Hard problems donât always offer enough training data to train an end-to-end neural network. In this case, combining a physical model with neural networks led to significant progress. This design pattern holds promise in many other domains from climate change to robot dynamics.
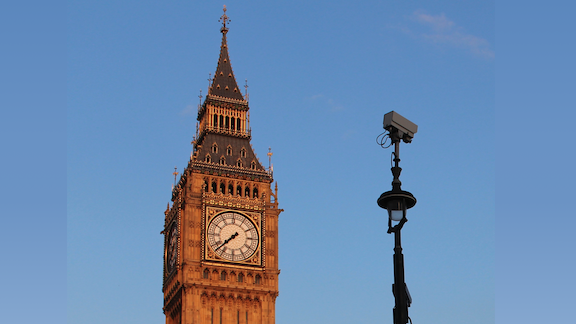
Nowhere to Hide
Real-time face recognition has become standard operating procedure for cops in a few cities, in both authoritarian and democratic countries.
Whatâs new: After years of trials, police departments in Moscow and London are using face recognition to scan the streets for suspected criminals.
How it works: Systems in both cities connect to pre-existing closed-circuit television networks. Enforcers in Moscow aim to deploy the tech city-wide, according to The Verge. So far, though, theyâre using only a fraction of the cityâs tens of thousands of cameras. London plans a more limited rollout in popular shopping and tourist areas.
- Moscow paid NTechLabs, a homegrown company, $3.2 million to license its technology. The company maintains a watch list of suspects and notifies authorities if it finds a match.
- Prior to serving the law enforcement market, NTechLabs offered a consumer app for matching pictures of people to their social media profile. Its FindFace app made headlines in 2016 when internet trolls used it to dox sex workers.
- Londonâs Metropolitan Police licenses face recognition tech from NEC. It runs cameras for five to six hours at a time as it tries to match watch lists of suspected violent criminals and child sex traffickers.
Why it matters: Face recognition technology is becoming routine for police forces around the globe. It has been used to catch a murderer in Chongqing, helped stop street crime in New York City, and figured in 30 percent of solved cases in one small U.S. city.
Yes, but: Independent researchers evaluating recent trials in London found that the system misidentified 81 percent of suspects it flagged. The police department contests those numbers, saying its own studies show only one in 1,000 false positives.
Weâre thinking: Law enforcement agencies worldwide need thoughtfully designed and clearly worded regulatory guidance so they can use these tools without overstepping civil liberties.
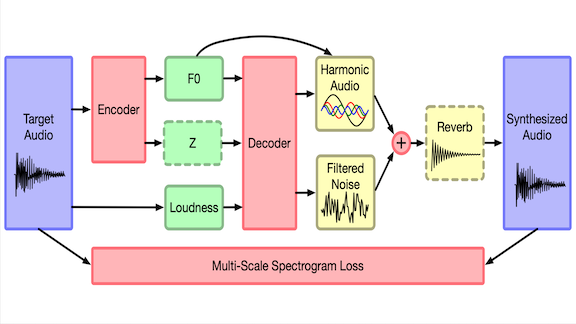
Old Tools for New Synths
Neural audio synthesizers like WaveRNN or GANSynth produce impressive sounds, but they require large, data-hungry neural networks. A new code library beefs up the neural music studio with efficient sound modules based on traditional synthesizer designs.
Whatâs new: Jesse Engel and colleagues at Google Brain introduced Differentiable Digital Signal Processing (DDSP), a set of digital signal processing tools that integrate with neural networks to boost their performance.
Key insight: Traditional synthesizers incorporate powerful sound-generation and -processing tools, but their controls are often limited to sliders and switches that donât take full advantage of their abilities. A neural network can learn to manipulate such tools more dynamically, potentially producing more realistic renditions of existing instruments as well as novel sounds.
How it works: DDSP offers tools such as oscillators (which generate sound), filters (which modify tone color), envelopes (which shape the sound over time), and reverberators (which mimic sound waves that reflect off walls). Most are implemented as layers that can be inserted into neural networks without affecting backprop training, so a network can learn to control them.
- The researchers use DDSP to emulate the Spectral Modeling Synthesizer (SMS), a 1990s-vintage digital synth. Once it has been trained, their SMS emulator can mimic input sounds. Also, parts of an SMS network trained on, for instance, violins can be swapped with those of one trained on, say, guitars to reinterpret a violin recording using a guitar sound.
- They re-created the SMS architecture as an autoencoder with additional components. The autoencoderâs encoder maps input sounds to low-dimensional vectors. The decoderâs output drives DDSPâs oscillator and filter, which in turn feed a reverberator to produce the final output.
Results: The SMS emulator showed that DDSP can make for a high-quality neural sound generator. Compared to WaveRNN, it scored better for L1 loudness loss, a measure of the difference between audio input and synthesized output (.07 compared to .10). It also had a better L1 loss of fundamental frequency, which measures the accuracy of the synthesized waveform relative to the input (.02 versus 1.0). And it has one tenth as many parameters!
Why it matters: Audio synthesis is one of several applications migrating from digital signal processing tech to deep learning. Machine learning engineers need not leave the older technology behind â they can build DSP functions into their neural networks.
Weâre thinking: The SMS demo is preliminary, but it points toward next-generation audio models that combine deep learning with more intuitive structures and controls.