Dear friends,
Earlier this month, my team AI Fund held its annual co-founder and CEO summit, where many of our collaborators gathered in California for two days to discuss how to build AI companies. Three themes emerged from many presentations: persistence, fast iteration and community.
Persistence. Doing impactful work is hard! Tim Westergren (founder and former CEO of Pandora, Venture Advisor at AI Fund) said it was only on his 348th venture pitch that Pandora raised its Series A round of funding. He also spoke about the tough time when Pandora team members went without salaries for an extended period of time to try to make the company work out. While many people unfortunately are not in a position to make such sacrifices to build a business, sometimes it does take extraordinary effort — and, yes, sacrifices — to do something really meaningful.
Fast iteration. AI Fund’s process of building startups is focused on a three- month, bi-weekly sprint process, in which we iterate quickly through technical prototypes as well as business ideas. Bill MacCartney (former VP of Cohere, Venture Advisor at AI Fund) said, “The best way to start is just by building on top of . . . whatever the best model is . . .. Don’t worry about [cost or latency] at first. You’re really just trying to validate the idea.”
One technique that’s now very widespread for prototyping is retrieval augmented generation (RAG). I’ve been surprised at how many nontechnical business leaders seem to know what RAG is. Investors are sometimes leery of people who build a thin layer around LLMs. As Laurence Moroney (lead AI Advocate at Google, AI Fund Fellow) says, “I’m a huge fan of RAG . . .. I think this is one way to go beyond a thin veneer around [commercial] models and build a somewhat thicker veneer.”
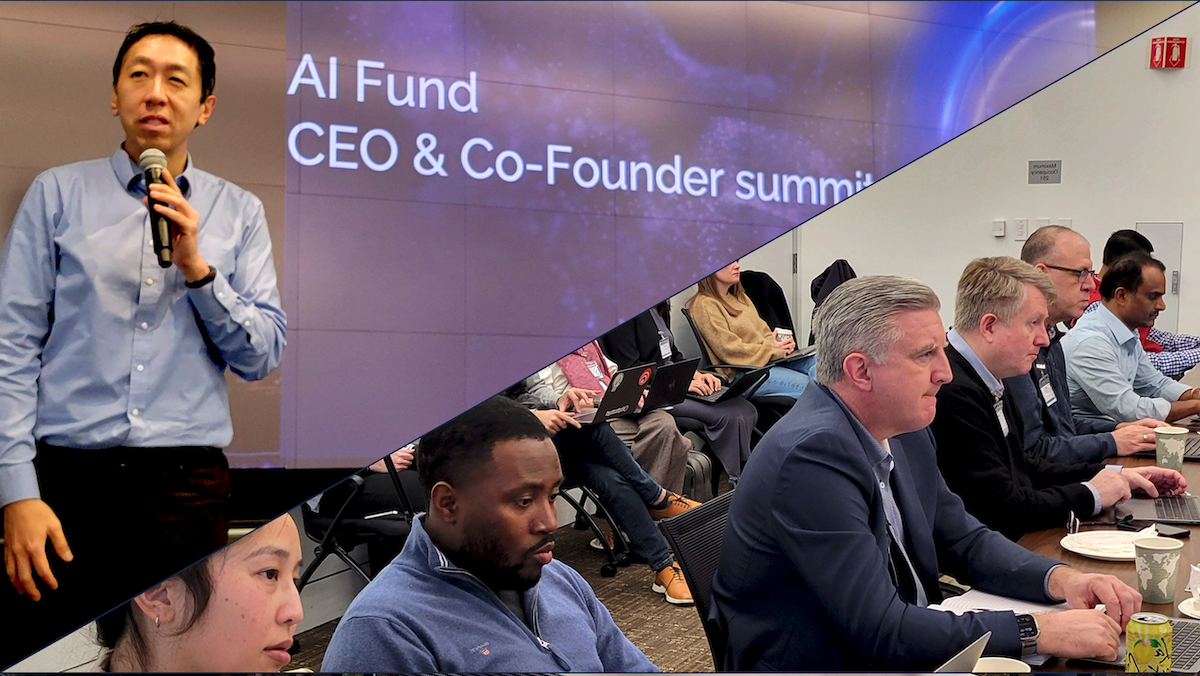
Community. Despite the wide range of startups represented in sectors including deep AI tech, healthcare, finance, edtech, and so on, a recurring theme was that company builders end up stronger when they come together. Emil Stefanutti (co-founder of ContractRoom, Venture Advisor at AI Fund) said he was glad that many of the scars he has acquired by building businesses are turning out to be treasures for others, as he's able to share experiences that other entrepreneurs can benefit from. Tim Westergren said, “You can’t white-knuckle it. You also can’t do it alone.”
The themes of persistence, fast iteration, and community apply whether you work in a large company, startup, research, government, or elsewhere. When I think of innovators in any field, I often think of Teddy Roosevelt’s message:
“It is not the critic who counts; not the [person] who points out how the strong [person] stumbles, or where the doer of deeds could have done them better. The credit belongs to the [person] who is actually in the arena, whose face is marred by dust and sweat and blood; who strives valiantly; who errs, who comes short again and again, … who knows great enthusiasms, the great devotions; who spends himself in a worthy cause.”
Keep learning!
Andrew
News
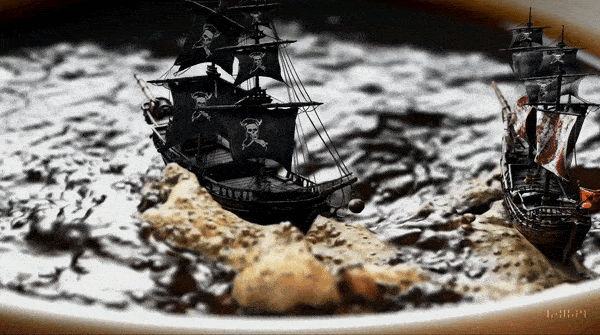
Generated Video Gets Real(er)
OpenAI’s new video generator raises the bar for detail and realism in generated videos — but the company released few details about how it built the system.
What’s new: OpenAI introduced Sora, a text-to-video model that can produce extraordinarily convincing, high-definition videos up to one minute long. You can see examples here.
What we know: Sora is a latent diffusion model that learned to transform noise into videos using an encoder-decoder and transformer. The system was trained on videos up to 1,920x1,080 pixels and up to one minute long.
- Following DALL·E 3, OpenAI trained a video captioning model to enhance the captions of videos in the dataset, adding descriptive details.
- Given a video’s frames divided into patches, the encoder learned to embed the patches and further compress them along the time dimension, producing tokens. Given the tokens, the decoder learned to reconstruct the video.
- Given tokens that had been adulterated by noise and an enhanced prompt, the transformer learned to generate the tokens without noise.
- At inference, a separate transformer enhanced input prompts to be more descriptive. Given the enhanced prompt and noisy tokens, Sora’s transformer removed the noise. Given the denoised tokens, the decoder produced a video.
What we don’t know: OpenAI is sharing the technology with outside researchers charged with evaluating its safety, The New York Times reported. Meanwhile, the company published neither quantitative results nor comparisons to previous work. Also missing are detailed descriptions of model architectures and training methods. (Some of the results suggest that Sora was trained not only to remove noise from tokens, but also to predict future tokens and generate tokens in between other tokens.) No information is available about the source(s) of the dataset or how it may have been curated.
Qualitative results: Sora’s demonstration output is impressive enough to have sparked arguments over the degree to which Sora “understands” physics. A photorealistic scene in which “a stylish woman walks down a Tokyo street filled with warm glowing neon” shows a crowded shopping district filled with believable pedestrians. The woman’s sunglasses reflect the neon signs, as does the wet street. Halfway through its one-minute length, the perspective cuts — unprompted and presumably unedited — to a consistent, detailed close-up of her face. In another clip, two toy pirate ships bob and pitch on a frothing sea of coffee, surrounded by a cup’s rim. The two ships maintain their distinctiveness and independence, their flags flutter in the same direction, and the liquid churns fantastically but realistically. However, as OpenAI acknowledges, the outputs on display are not free of flaws. For instance, the pirate-battle cup’s rim, after camera motion has shifted it out of the frame, emerges from the waves. (Incidentally, the Sora demos are even more fun with soundtracks generated by Eleven Labs.)
Why it matters: While we’ve seen transformers for video generation, diffusion models for video generation, and diffusion transformers for images, this is an early implementation of diffusion transformers for video generation (along with a recent paper). Sora shows that diffusion transformers work well for video.
We’re thinking: Did Sora learn a world model? Learning to predict the future state of an environment, perhaps given certain actions within that environment, is not the same as learning depict that environment in pixels —just like the ability to predict that a joke will make someone smile is different than the ability to draw a picture of that smile. Given Sora's ability to extrapolate scenes into the future, it does seem to have some understanding of the world. Its world model is also clearly flawed — for instance, it will synthesize inconsistent three-dimensional structures — but it’s a promising step toward AI systems that comprehend the 3D world through video.
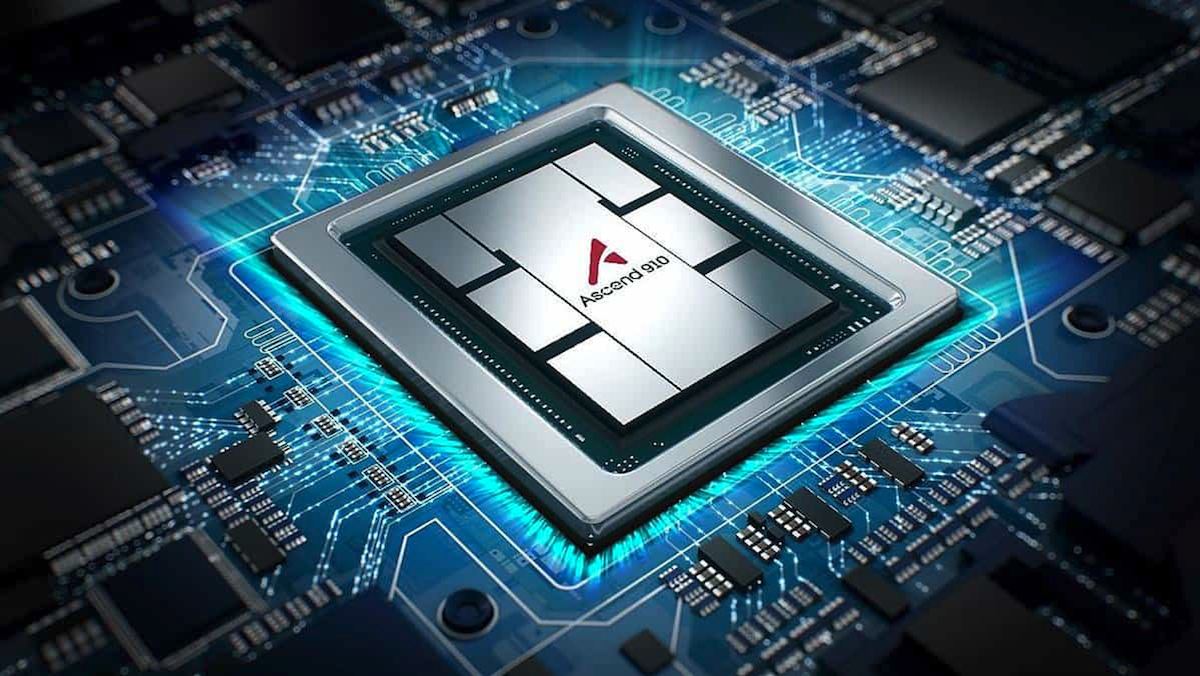
Competition Heats Up in AI Chips
Huawei is emerging as an important supplier of AI chips.
What’s new: Amid a U.S. ban on exports of advanced chips to China, demand for Huawei’s AI chips is so intense that the company is limiting production of the chip that powers one of its most popular smartphones so it can serve the AI market, Reuters reported.
Demand and supply: China’s biggest chip fabricator, Semiconductor Manufacturing International Corp. (SMIC), fabricates both the Ascend 910B, which is optimized to process neural networks, and the Kirin chip that drives Huawei’s popular Mate 60 phone. Production capacity is limited, so making more Ascend 910Bs means making fewer Kirins.
- The Huawei Ascend 910B is widely considered to be the best AI chip available in China. The chip has been reported to deliver performance roughly comparable to that of Nvidia’s A100 (immediate predecessor to the current H100, which is more than three times faster).
- The Nvidia H100, which is the industry standard for processing deep learning models, has become scarce in China since late 2022, when the U.S. restricted exports of advanced chips and use of chip-making equipment. The shortage of Nvidia chips is driving demand for the Ascend 910B.
- The U.S. action also forced Huawei to switch manufacturers from Taiwan Semiconductor Manufacturing Company to SMIC. But the limits on manufacturing equipment have made it difficult to fabricate the Ascend 910B. SMIC has been able to produce a relatively small number of units that are free from defects.
- Huawei’s decision to shift manufacturing from phone chips to AI chips is sacrificing one of its most popular products. Huawei’s Mate 60 phone outsold the Apple iPhone in China last year, helping to elevate Huawei in January to the top-selling phone maker in China for the first time in three years.
Behind the news: Nvidia accounted for 90 percent of the market for AI chips in China prior to the advent of U.S. export restrictions. China has responded to the limits by building its ability to manufacture advanced chips domestically — a tall order, since it requires technology that is very difficult to develop. In August, Baidu ordered 1,600 Ascend 910B chips for delivery by the end of the year, according to an earlier Reuters report. The order, which is tiny compared to typical data center purchases, nonetheless demonstrated that SMIC could manufacture the chips and that Baidu was experimenting with alternatives to Nvidia in anticipation of even tighter U.S. restrictions on AI chips that took effect in October. Currently, SMIC is gearing up to produce Huawei’s next-generation Ascend chips.
Why it matters: For years, Nvidia’s GPUs have been the only practical choice for processing deep learning models. The company’s lead over competitors both in hardware implementation and software support are likely to protect its dominant position for some time to come. However, competitors like AMD and Huawei are beginning to nip at Nvidia’s heels. That means more hardware options for developers, and the competition may drive lower prices and still higher performance.
We’re thinking: AI chips are at the heart of the current technological competition between the U.S. and China. While Huawei and SMIC still have a lot to prove in terms of scaling up production, their rate of progress is impressive and illustrates the limits of the current U.S. restrictions.
A MESSAGE FROM DEEPLEARNING.AI
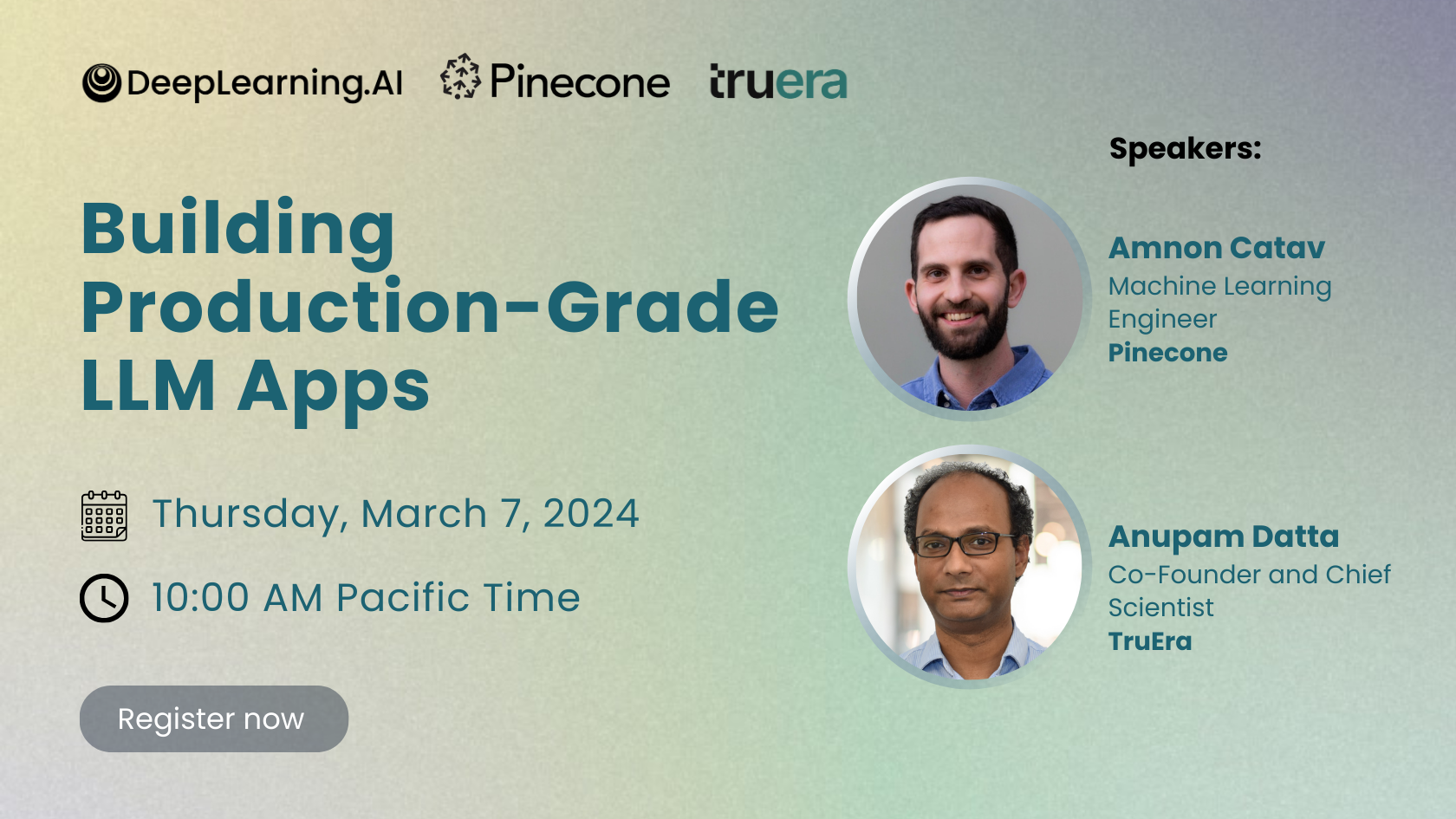
In our next live workshop, we’ll share how to build high-quality and production-ready applications using tools like Pinecone Canopy and TruLens. Notebooks will be available for participants to explore! Register now
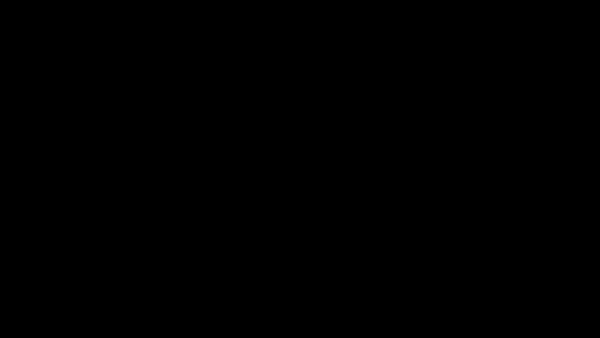
Gymnastics Judge’s Helper
Judges in competitive gymnastics are using an AI system to double-check their decisions.
What’s new: Olympic-level gymnastic contests have adopted Judging Support System (JSS), an AI-based video evaluation system built by Fujitsu, MIT Technology Review reported. In September and October, for the first time, judges at the 2023 World Artistic Gymnastics Championships in Antwerp used JSS in competitions that involved the full range of gymnastics equipment including mat, balance beam, parallel bars, pommel horse, and so on.
How it works: Judges penalize gymnasts for imperfections in any pose or move. JSS identifies deviations that correspond to particular penalties. The system can evaluate roughly 2,000 poses and moves with 90 percent accuracy compared to human judges. It can assess both isolated actions and entire routines.
- Fujitsu trained JSS on video footage of 8,000 gymnastic routines that encompass the official gymnastics scoring guide. The system matches body positions to corresponding poses and motions described in the scoring guide.
- JSS receives position data on a gymnast’s body from 4 to 8 cameras. A 2018 paper offers hints about how the current system may work: Given the images, it detects the posture (front-facing, handstand, or rear-facing). Given the posture, it feeds the images into a corresponding 3D model. Then it converts the images into a virtual skeleton, conforms a human model to the skeleton, and modifies the skeleton (and conformed model) to match the images.
- Under the current rules, judges can use JSS only when competitors challenge a score or a judge and supervisor disagree. The International Gymnastics Federation, the sport’s global governing body, has not yet revealed whether or how the system will be used at this year’s Summer Olympics in Paris.
Behind the news: Sporting authorities have embraced AI both inside and outside the arena.
- The English Premier League football clubs Chelsea and Nottingham Forest have expressed interest in the AISCOUT app as a way to discover fresh talent. Amateur players upload videos of themselves performing drills, and the app scores their performance.
- At the 2020 Summer Olympics in Tokyo, official timekeeper Omega Timing provided several AI-based systems: a pose estimator for gymnasts on the trampoline, an image recognition system that analyzed swimmers’ performance, and a ball tracker for volleyball.
- Acronis, a Swiss company that provides video storage for pro football teams, has built AI applications that track players’ movements and analyze their tactics. The company also predicts match attendance for teams in the English Premier League based on ticket sales, weather, and other factors.
Why it matters: Gymnastic competitors are scored on subjective criteria such as expression, confidence, and personal style as well as technical competence, raising questions of unconscious bias and whether some judges might favor certain competitors over others. An AI system that tracks technical minutiae may help judges to avoid bias while focusing on the sport’s subjective aspects.
We’re thinking: Tracking gymnasts in motion sets a high bar for AI!
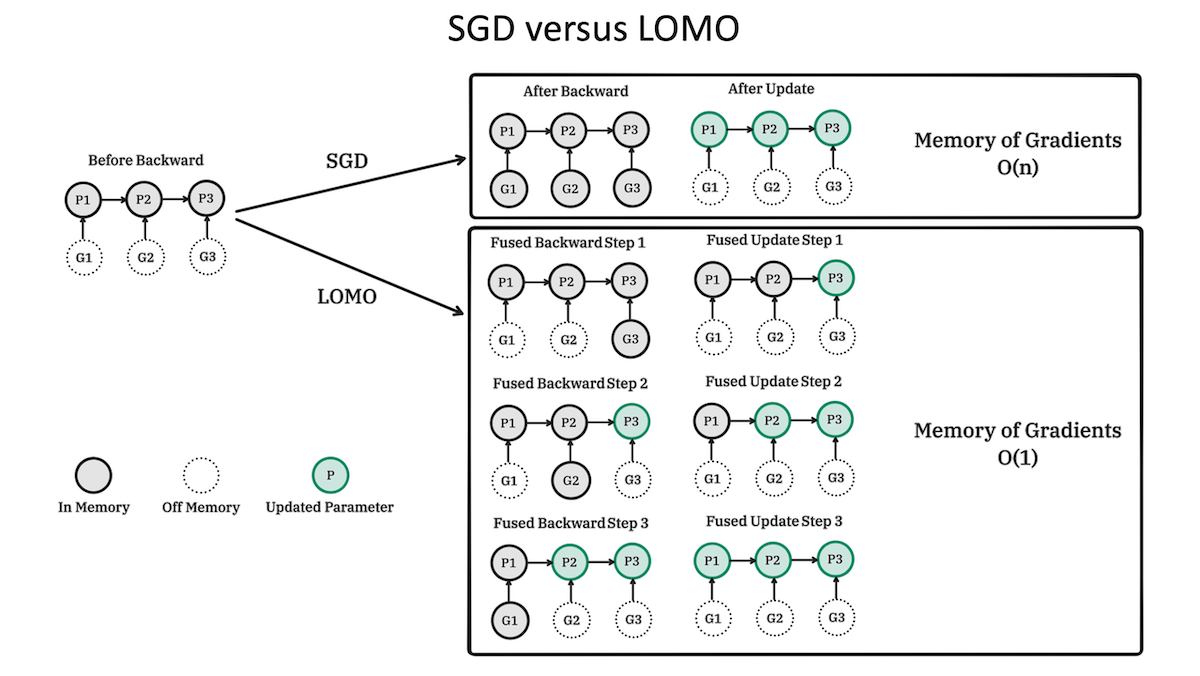
Memory-Efficient Optimizer
Researchers devised a way to reduce memory requirements when fine-tuning large language models.
What's new: Kai Lv and colleagues at Fudan University proposed low memory optimization (LOMO), a modification of stochastic gradient descent that stores less data than other optimizers during fine-tuning.
Key insight: Optimizers require a lot of memory to store an entire network’s worth of parameters, gradients, activations, and optimizer states. While Adam has overtaken stochastic gradient descent (SGD) for training, SGD remains a popular choice for fine-tuning partly because it requires less memory (since it stores fewer optimizer states). Nonetheless, SGD must store an entire network’s gradients — which, with state-of-the-art models, can amount to tens or hundreds of gigabytes — before it updates the network all at once. Updating the network layer by layer requires storing only one layer’s gradients — a more memory-efficient twist on typical SGD.
How it works: The authors fine-tuned LLaMA on six datasets in SuperGLUE, a benchmark for language understanding and reasoning that includes tasks such as answering multiple-choice questions.
- The authors modified SGD to compute gradients for one layer and update that layer’s weights before advancing to the next.
- To avoid the potential for exploding or vanishing gradients, in which gradients from later layers either expand or diminish as they backpropagate through the network, LOMO normalized the gradients, scaling them to a predetermined range throughout the network. LOMO used two backward passes: one to compute the magnitude of the gradient for the entire network, and another to scale each layer’s gradient according to the total magnitude and then update its parameters.
Results: LOMO required less memory than popular optimizers and achieved better performance than the popular memory-efficient fine-tuning technique LoRA.
- The authors fine-tuned separate instances of LLaMA-7B using LOMO and two popular optimizers, SGD and AdamW (a modified version of Adam). They required 14.6GB, 52.0GB, and 102.2GB of memory respectively. In particular, they all required the same amount of memory to store model parameters (12.55GB) and activations (1.79GB). However, when it came to gradients, LOMO required only 0.24GB while SGD and AdamW each required 12.55GB. The biggest difference was optimizer state memory: LOMO required 0GB, SGD required 25.1GB, and Adam required 75.31GB.
- The authors also compared LOMO to LoRA, which works with an optimizer (in this case, AdamW) to learn to change each layer’s weight matrix by a product of two smaller matrices. They performed this comparison with LLaMAs of four sizes on six datasets. LOMO achieved better accuracy in 16 of the 24 cases, and its average accuracy across datasets exceeded LoRA’s at each model size. For example, the 65 billion-parameter LOMO-tuned LLaMA averaged 89.9 percent accuracy, and the 65 billion-parameter LoRA/AdamW-tuned LLaMA averaged 89.0 percent accuracy.
Why it matters: Methods like LoRA save memory by fine-tuning a small number of parameters relative to a network’s total parameter count. However, because it adjusts only a small number of parameters, the performance gain from fine-tuning is less than it could be. LOMO fine-tunes all parameters, maximizing performance gain while reducing memory requirements.
We're thinking: SGD’s hunger for memory is surprising. Many developers will find it helpful to have a memory-efficient alternative.
Data Points
More AI news of the week. From Gemini 1.5’s whopping context window to the energy footprint of AI models and Google's next research hub city, we covered it all.
Dive into this week's key AI developments in our latest Data Points edition.