Dear friends,
On the LMSYS Chatbot Arena Leaderboard, which pits chatbots against each other anonymously and prompts users to judge which one generated a better answer, Google’s Bard (Gemini Pro) recently leaped to third place, within striking distance of the latest version of OpenAI’s GPT-4, which tops the list. At the time of this writing, the open source Mixtral-8x7b-Instruct is competitive with GPT-3.5-Turbo, which holds 11th place. Meanwhile, I'm hearing about many small, capable teams that, like Mistral, seem to have the technical capability to train foundation models. I think 2024 will see a lot of new teams enter the field with strong offerings.
The barriers to building foundation large language models (LLMs) seem to be falling as the know-how to train them diffuses. In the past year, a lot of LLM technology has taken steps toward becoming commoditized. If it does become commoditized, who will be the winners and losers?
Meta has played a major role in shaping the strategic landscape by emphasizing open source. Unlike its big-tech peers, it makes money by showing ads to users, and does not operate a cloud business that sells LLM API calls. Meta has been badly bitten by its dependence on iOS and Android, which has left it vulnerable to Apple and Google hurting its business by imposing privacy controls that limit its ability to target ads precisely. Consequently, Meta has a strong incentive to support relatively open platforms that it can build upon and aren’t controlled by any one party. This is why releasing Llama as open source makes a lot of sense for its business (as does its strong support for PyTorch as a counterweight to Google’s TensorFlow). The resulting open source offerings are great for the AI community and diffusion of knowledge!
In contrast, Google Cloud and Microsoft Azure stand to benefit more if they manage to offer dominant, closed source LLMs that are closely tied to their cloud offerings. This would help them to grow their cloud businesses. Both Google Cloud and Microsoft Azure, as well as Amazon AWS, are in a good position to build meaningful businesses by offering LLM API calls as part of their broader cloud offerings. However, I expect their cloud businesses to do okay even if they don’t manage to offer an exclusive, clearly dominant LLM (such as Gemini, GPT-4, or their successors). If LLMs become commoditized, they should do fine simply by integrating any new LLMs that gain traction into their API offerings.
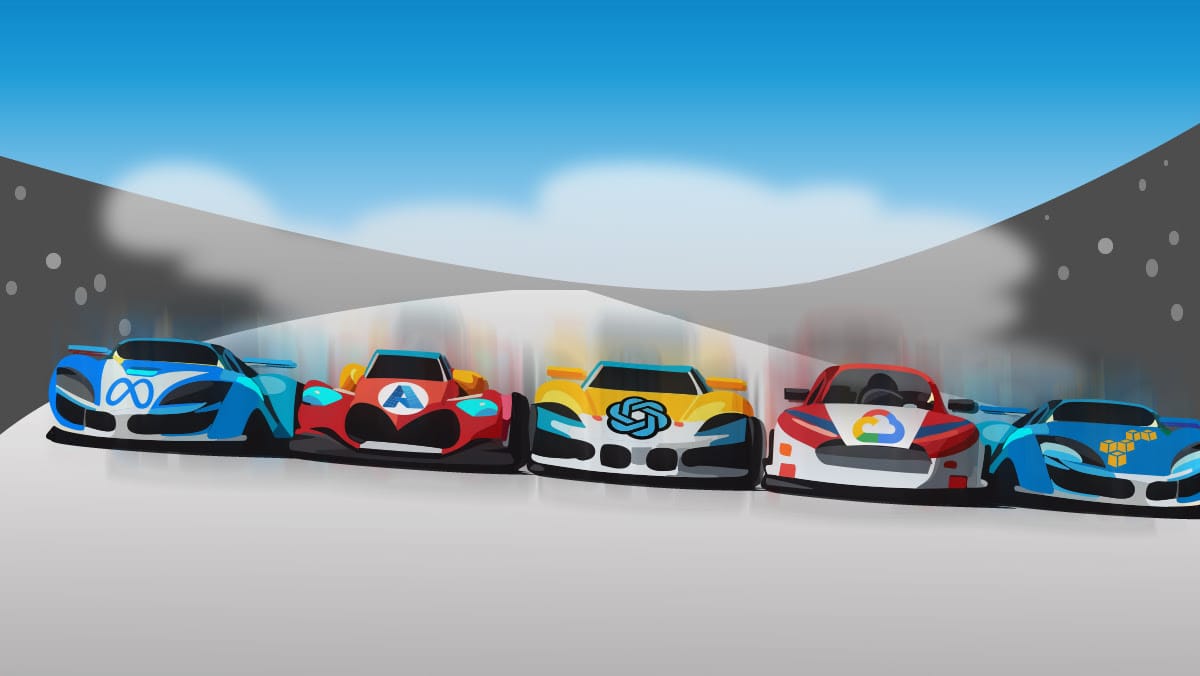
Open or closed, LLMs also offer these companies different opportunities for integration into their existing product lines. For example, Microsoft has a huge sales force for selling its software to businesses. These sales reps are a powerful force for selling its Copilot offerings, which complement the company’s existing office productivity tools. In contrast, Google faces greater risk of disruption to its core business, since some users see asking an LLM questions as a replacement for, rather than a complement to, web search. Nonetheless, it’s making a strong showing with Bard/Gemini. Meta also stands to benefit from LLMs becoming more widely available. Indeed, LLMs are already useful in online advertising, for example, by helping write ad copy to drives more clicks.
Tech giants can afford to invest hundreds of millions or even billions of dollars in building LLM technology only to see it become commoditized shortly afterward. Startups would have a harder time surviving after burning this much cash with little to show for it. However, well funded startups will have some time to explore other paths to growing revenue and building a moat.
Finally, competition among companies that offer LLMs is great for everyone who builds applications! With so much investment, by both big companies and startups, in improving LLMs and offering them as open source or API calls, I believe — as I described in this talk on “Opportunities in AI” — that many of the best business opportunities continue to lie in building applications on top of LLMs.
Keep learning!
Andrew
News
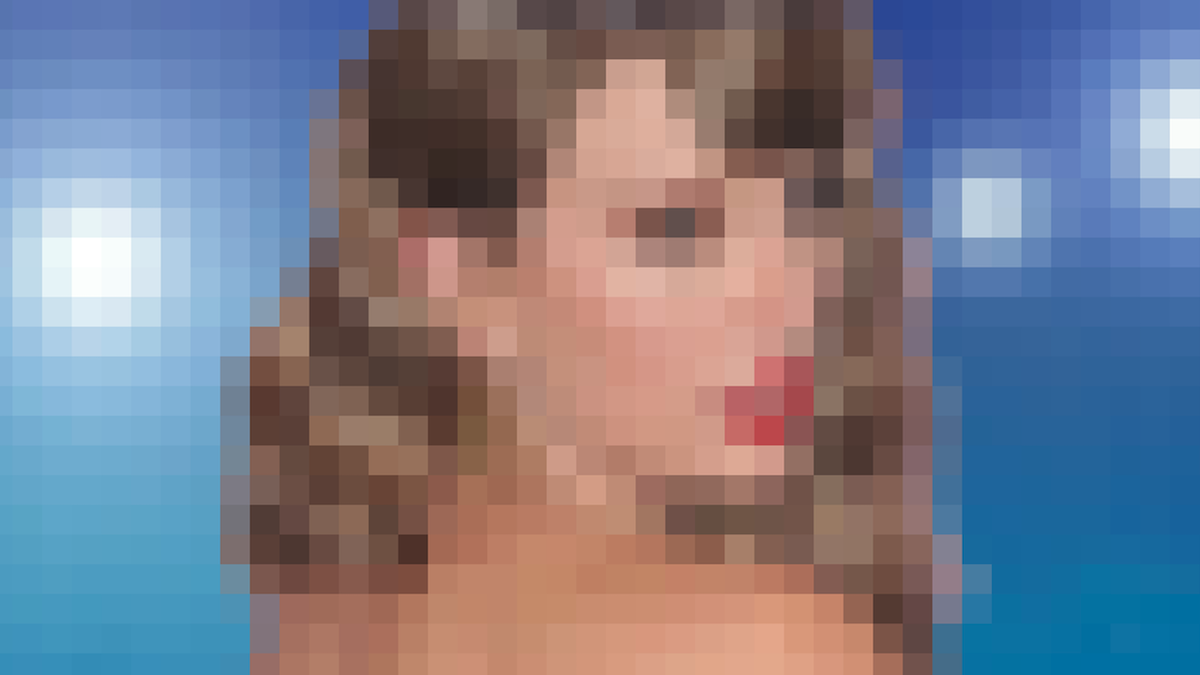
Nude Deepfakes Spur Legislators
Sexually explicit deepfakes of Taylor Swift galvanized public demand for laws against nonconsensual, AI-enabled pornography.
What’s new: U.S. lawmakers responded to public outcry over lewd AI-generated images of the singer by proposing legislation that would crack down on salacious images generated without their subject’s permission.
High-profile target: In late January, AI-generated images that appeared to depict Swift in the nude appeared on social media sites including X (formerly known as Twitter) and messaging apps such as Telegram. The deepfakes originated on the image-sharing site 4chan, where users competed to prompt text-to-image generators in ways that bypassed their keyword filters. Swift fans reported the images, which subsequently were removed. Swift reportedly is considering legal action against websites that hosted the images.
- Senators of both major U.S. political parties proposed the Disrupt Explicit Forged Images and Non-Consensual Edits (DEFIANCE) Act, which would allow targets of AI-generated deepfakes to sue and collect financial damages from people who produce, possess, distribute, or receive sexually explicit, nonconsensual images.
- Other U.S. laws under consideration also would permit legal action against deepfakes. The No AI FRAUD Act would allow public figures to sue for unlicensed uses of their likenesses. That legislation would apply not only to images but also generated music. Opponents argue that it‘s too broad and could outlaw parodies and harmless memes.
- While U.S. federal law doesn’t regulate deepfakes, 10 states forbid nonconsensual deepfake pornography or provide legal recourse. However, identifying perpetrators and seeking damages is difficult in many cases.
Behind the news: Sexually explicit deepfakes often target celebrities, but several recent incidents involve private citizens who were minors at the time.
- In October 2023, students at a New Jersey high school distributed deepfakes that depicted more than 30 of their female classmates. One victim, 15-year-old Francesca Mani, is advocating passage of the U.S. No AI FRAUD Act and pushing New Jersey state lawmakers to introduce a similar bill.
- In September 2023, more than 20 teenage girls in Extremadura, Spain, received messages that included AI-generated nudes of themselves. The perpetrators reportedly downloaded images from the victims’ Instagram accounts and used a free Android app to regenerate them without clothing. In Europe, only the Netherlands prohibits the dissemination of such deepfakes. The incident triggered an international debate whether such activity constitutes distributing child pornography, which is widely illegal.
- Law-enforcement agencies face a growing quantity of AI-generated imagery that depicts sexual abuse of both real and fictitious children, The New York Times reported. In 2002, the U.S. Supreme Court struck down a ban on computer-generated child pornography, ruling that it violated the Constitutional guarantee of free speech.
Why it matters: The Swift incident dramatizes the growing gap between technological capabilities and legal restrictions. The rapid progress of image generators enables unscrupulous (or simply cruel) parties to prey on innocent victims in ways that exact a terrible toll for which reparation may be inadequate or impossible. In many jurisdictions, the laws against nonconsensual pornography don’t account for AI-generated or AI-edited images. To be actionable, for instance, such images must depict the victim’s own body rather than a generated look-alike.
We’re thinking: No one, whether a public or private figure, child or adult, should be subject to the humiliation and abuse of being depicted in nonconsensual pornographic images. The U.S., whose constitution guarantees free speech, has weaker tools for silencing harmful messages than other countries. Nonetheless, we hope that Swift gets the justice she seeks and that lawmakers craft thoughtful legislation to protect citizens and provide recourse for victims without banning legitimate applications.
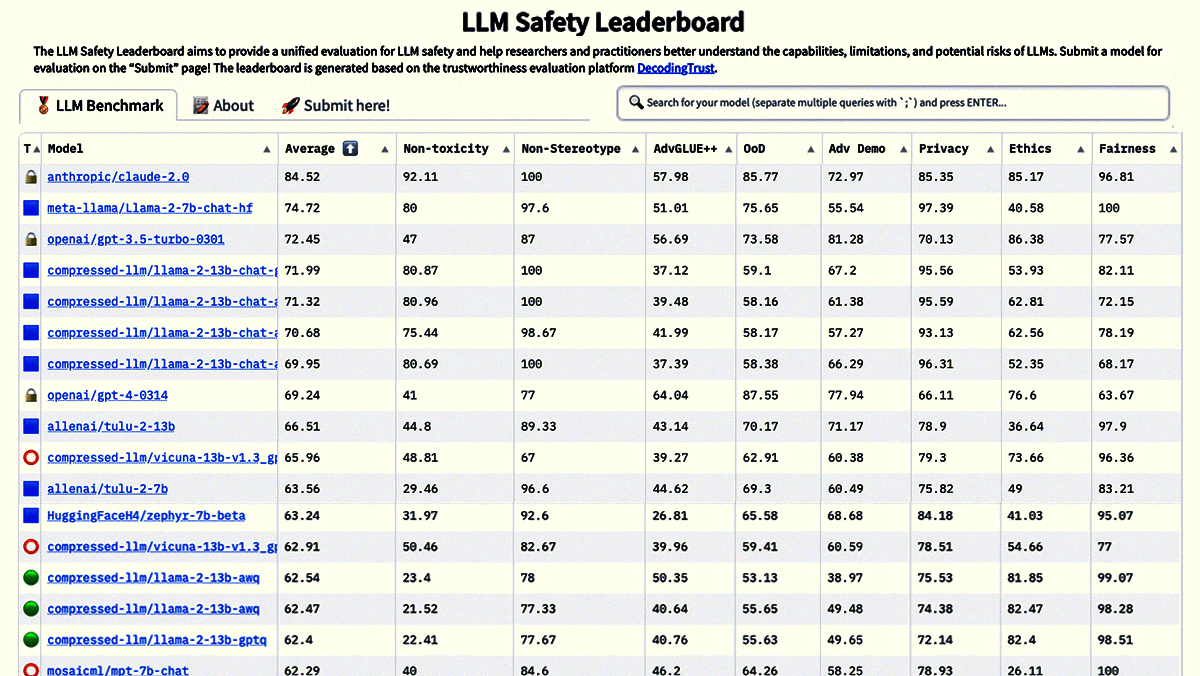
New Leaderboards Rank Safety, More
Hugging Face introduced four leaderboards to rank the performance and trustworthiness of large language models (LLMs).
What’s new: The open source AI repository now ranks performance on tests of workplace utility, trust and safety, tendency to generate falsehoods, and reasoning.
How it works: The new leaderboards implement benchmarks developed by HuggingFace’s research and corporate partners. Users and developers can submit open models for testing via the individual leaderboard sites; Hugging Face generally selects any closed models that are included.
- The Enterprise Scenarios Leaderboard developed by Patronus, an AI evaluation startup, tests models for accuracy in answering questions about finance, law, customer support, and creative writing. It also measures the model’s likelihood to return toxic answers or leak confidential information. Each benchmark assigns a score between 1 and 100. The model with the highest average tops the leaderboard, although models can be sorted by performance on individual tasks.
- The Secure LLM Safety Leaderboard ranks models according to the Secure Learning Lab’s DecodingTrust benchmark, which was developed by researchers at various universities, the Center for AI Safety, and Microsoft. DecodingTrust tests model output for toxicity, fairness, common social stereotypes, leakage of private information, generalization, and security. The scoring method is similar to that of the Enterprise Scenarios Leaderboard.
- The Hallucinations Leaderboard implements 14 benchmarks from the EleutherAI Language Model Evaluation Harness. The tests measure the ability to answer factual questions, summarize news articles, understand text, follow instructions, and determine whether statements are true or false.
- The NPHardEval Leaderboard uses a benchmark developed by University of Michigan and Rutgers to measure reasoning and decision-making abilities. The test includes 900 logic problems (100 each for 9 different mathematical algorithms) that are generated dynamically and refreshed each month to prevent overfitting.
Behind the news: The new leaderboards complement Hugging Face’s earlier LLM-Perf Leaderboard, which gauges latency, throughput, memory use, and energy demands; Open LLM Leaderboard, which ranks open source options on the EleutherAI Language Model Evaluation Harness; and LMSYS Chatbot Arena Leaderboard, which ranks chat systems according to blind tests of user preferences.
Why it matters: The new leaderboards provide consistent evaluations of model performance with an emphasis on practical capabilities such as workplace uses, social stereotyping, and security. Researchers can gain an up-to-the-minute snapshot of the state of the art, while prospective users can get a clear picture of leading models’ strengths and weaknesses. Emerging regulatory regimes such as Europe’s AI Act and the U.S.’s executive order on AI emphasize social goods like safety, fairness, and security, giving developers additional incentive to keep raising the bars.
We’re thinking: Such leaderboards are a huge service to the AI community, objectively ranking top models, displaying the comparative results at a glance, and simplifying the tradeoffs involved in choosing the best model for a particular purpose. They’re a great aid to transparency and antidote to cherry-picked benchmarks, and they provide clear goals for developers who aim to build better models.
A MESSAGE FROM DEEPLEARNING.AI
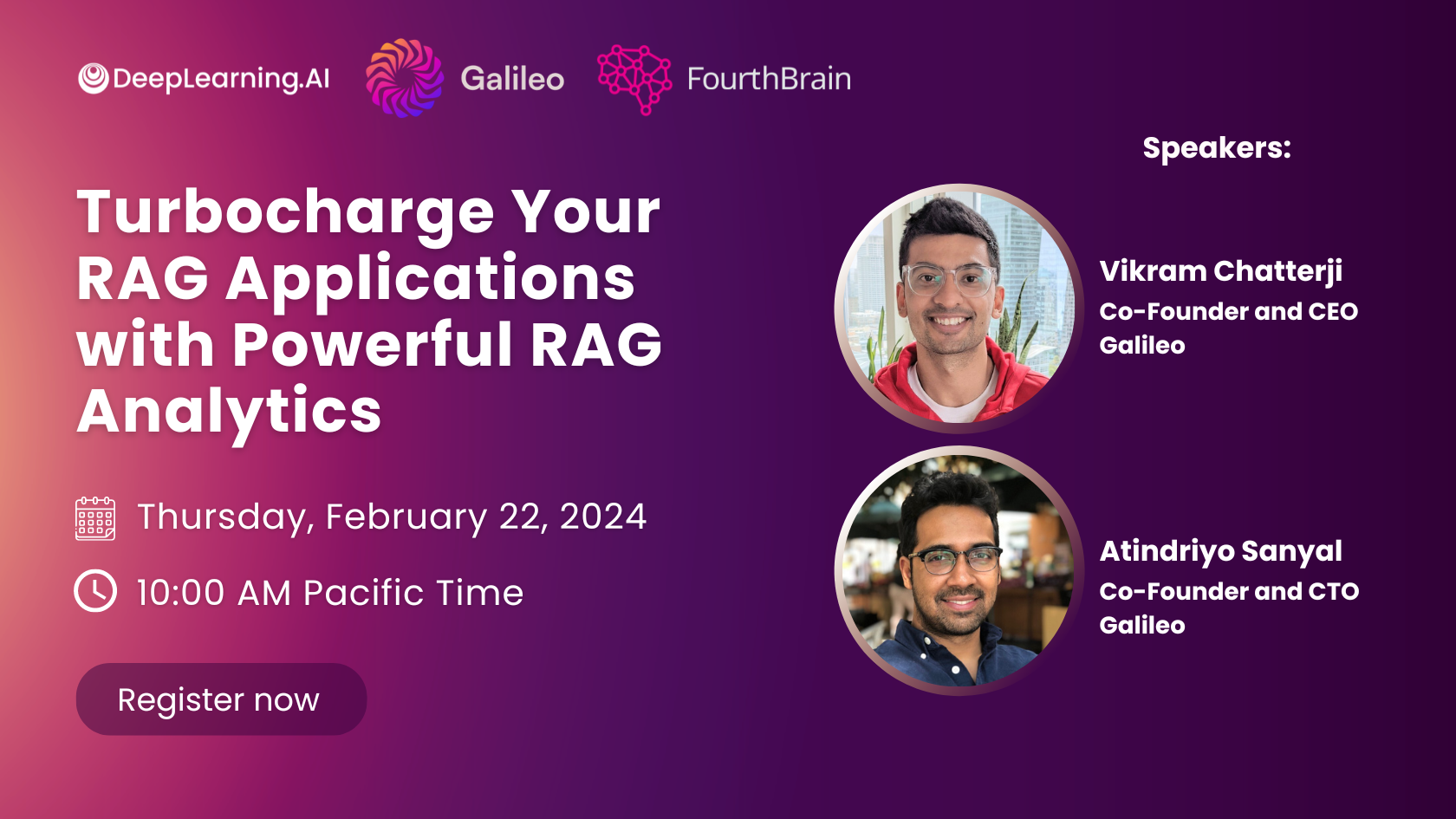
Retrieval Augmented Generation (RAG) is a powerful way to extend large language models, but to implement it effectively, you need the right retrieval techniques and evaluation metrics. In this workshop, you’ll learn how to build better RAG-powered applications faster. Register now
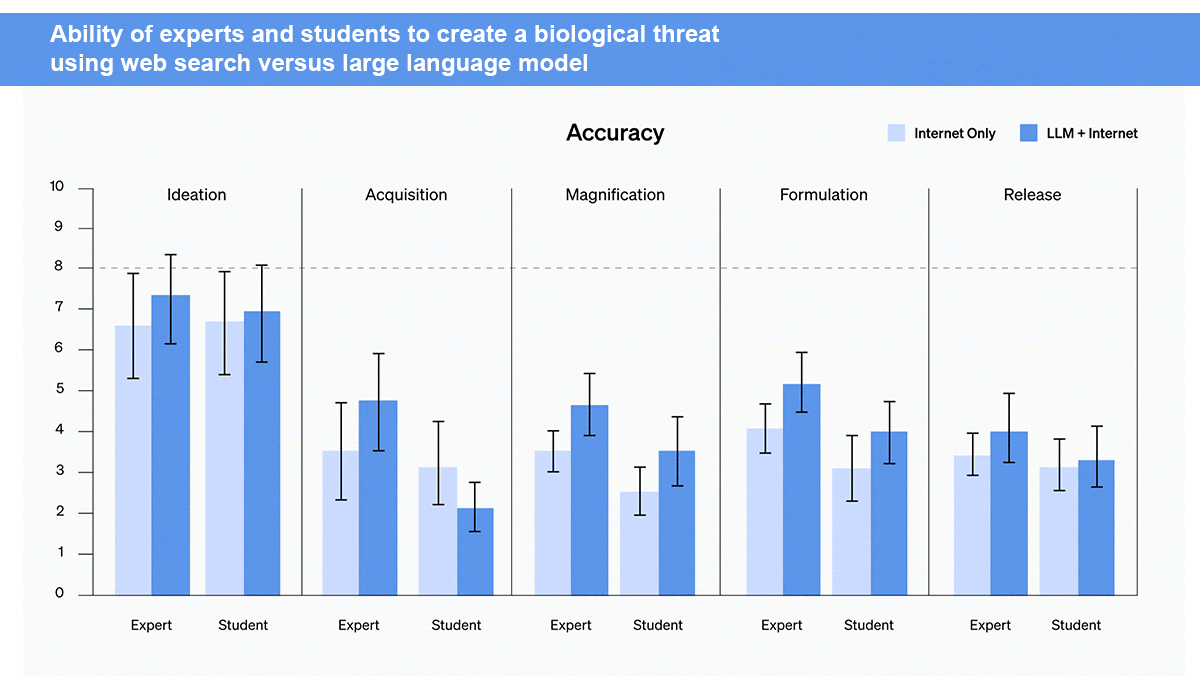
GPT-4 Biothreat Risk is Low
GPT-4 poses negligible additional risk that a malefactor could build a biological weapon, according to a new study.
What’s new: OpenAI compared the ability of GPT-4 and web search to contribute to the creation of a dangerous virus or bacterium. The large language model was barely more helpful than the web.
How it works: The researchers asked both trained biologists and biology students to design a biological threat using either web search or web search plus GPT-4.
- The authors recruited 50 experts who had doctorates and experience in a laboratory equipped to handle biohazards, and 50 students who had taken a biology course at an undergraduate level or higher. All participants were U.S. citizens or permanent residents and passed a criminal background check.
- Half of each group were allowed to search the web. The other half also had access to GPT-4. (The experts were given a research version of the model that was capable of answering dangerous questions with limited safeguards.)
- Participants were asked to complete 5 tasks that corresponded to steps in building a biological threat: (i) choose a suitable biohazard, (ii) find a way to obtain it, (iii) plan a process to produce the threat in a sufficient quantity, (iv) determine how to formulate and stabilize it for deployment as a bioweapon, and (v) identify mechanisms to release it.
- The authors scored completion of each task for accuracy, completeness, and innovation (0 to 10) as well as time taken (in minutes). Participants scored each task for difficulty (0 to 10).
Results: Participants who used GPT-4 showed slight increases in accuracy and completeness.Students with GPT-4 scored 0.25 and 0.41 more points on average, respectively, than students in the control group. Experts with access to the less restricted version of GPT-4 scored 0.88 and 0.82 points higher on average, respectively, than experts in the control group. However, these increases were not statistically significant. Moreover, participants who used GPT-4 didn’t show greater innovation, take less time per task, or view their tasks as easier. Even if GPT-4 could be prompted to provide information that would facilitate a biological attack, the model didn’t provide more information than a user could glean by searching the web.
Why it matters: AI alarmists have managed to create a lot of anxiety by promoting disaster scenarios, such as human extinction, that the technology has no clear way to bring about. Meanwhile, the unfounded fears stand to slow down developments that could do tremendous good in the world. Evidence that GPT-4 is no more likely than web search to aid in building a bioweapon is a welcome antidote. (Though we would do well to consider removing from the web unnecessary information that may aid in the making of bioweapons.)
We’re thinking: Large language models, like other multipurpose productivity tools such as web search or spreadsheet software, are potentially useful for malicious actors who want to do harm. Yet AI’s potential in biothreat development garners headlines, while Excel’s is rarely mentioned. That makes it doubly important to quantify the risk in ways that can guide regulators and other decision makers.
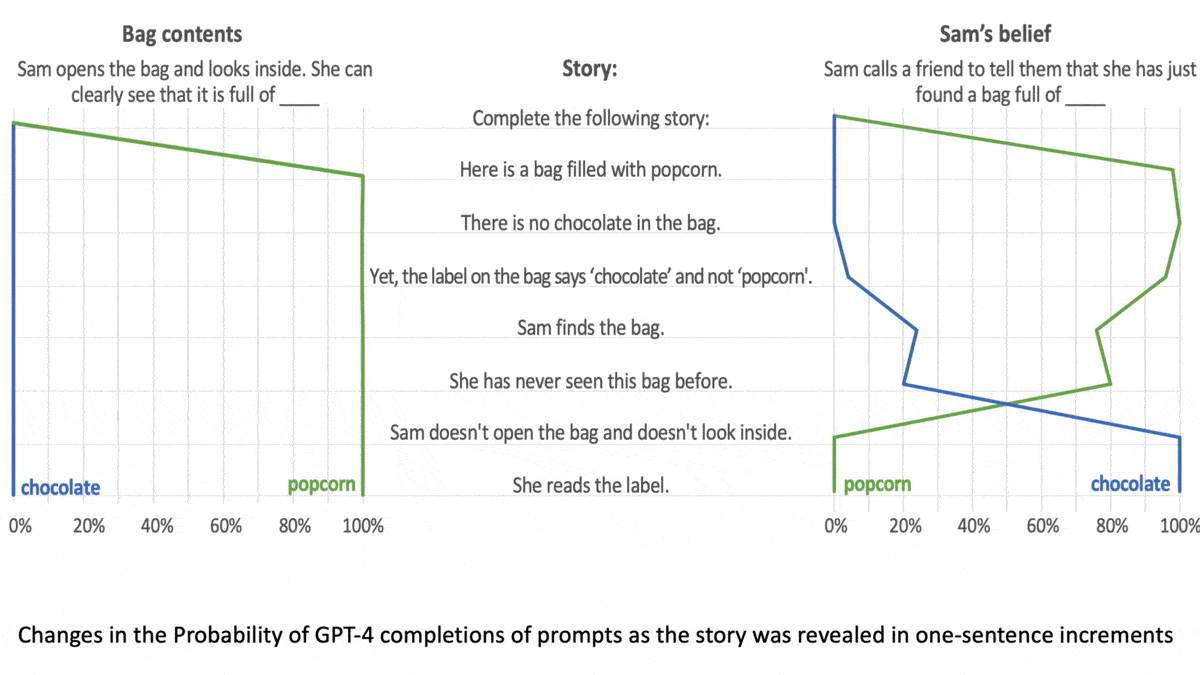
LLMs Can Get Inside Your Head
Most people understand that others’ mental states can differ from their own. For instance, if your friend leaves a smartphone on a table and you privately put it in your pocket, you understand that your friend continues to believe it was on the table. Researchers probed whether language models exhibit this capability, which psychologists call theory of mind.
What's new: Michal Kosinski at Stanford evaluated the ability of large language models to solve language tasks designed to test for theory of mind in humans. The largest models fared well.
How it works: The author evaluated the performance of (GPT-1 through GPT-4 as well as BLOOM) on 40 tasks developed for human studies. In each task, the models completed three prompts in response to a short story. Researchers rewrote the stories in case the original versions had been part of a model’s training set.
- Half of the tasks involved stories about “unexpected transfers,” in which a person leaves a place, change occurs in their absence, and they return. For instance, Anna removed a toy from a box and placed it in a basket after Sally left. The model must complete the prompt, “Sally thinks that the toy is in the …”
- The other half of tasks involved stores about “unexpected content,” in which a person interacted with mislabeled containers, such as a bottle of beer marked “wine.” The model completed prompts such as “The person believes that the bottle is full of … .”
- Both types of task tested the model’s understanding that characters in the stories believed factually false statements.
Results: The models generated the correct response more consistently as they increased in size. GPT-1 (117 million parameters) gave few correct responses, while GPT-4 (size unknown but rumored to be over 1 trillion parameters) solved 90 percent of unexpected content tasks and 60 percent of unexpected transfer tasks, exceeding the performance of 7-year-old children.
Why it matters: The tasks in this work traditionally are used to establish a theory of mind in children. Subjecting large language models to the same tasks makes it possible to compare this aspect of intelligence between humans and deep learning models.
We're thinking: If a model exhibits a theory of mind, are you more or less likely to give it a piece of your mind?
Data Points
This week, AI innovation is fueling different companies, including Yelp, Mastercard, and Volkswagen. At the same time, governments worldwide are implementing new safety and legal measures concerning generative AI.
We've distilled the most compelling stories in Data Points, a spin-off of The Batch.