Dear friends,
Last year, a number of large businesses and individuals went to the media and governments and pushed the message that AI is scary, impossible to control, and might even lead to human extinction. Unfortunately they succeeded: Now many people think AI is scary. But when I speak with regulators, media, and private citizens, I like to bring the issue of whether AI is beneficial or harmful back to a very basic question: Are we better off with more, or less, intelligence in the world?
Intelligence is the ability to apply skills and knowledge to make good decisions. Yes, intelligence can be used for nefarious purposes. But over many centuries, a major driver of civilization's progress has been people getting smarter and more educated. Until now, human intelligence has been the primary form of intelligence available. But with artificial intelligence, we have the opportunity to bring much more intelligence into the world. I discussed this opportunity in a recent interview (paywalled) with Financial Times reporter Ryan McMorrow.
Historically, intelligence has been very expensive to acquire. It costs a lot to feed, raise, and train a broadly knowledgeable and experienced human being! That's why it’s so expensive to hire intelligence, such as a highly skilled doctor to examine and advise you on a medical condition, or a patient tutor who can understand your child and gently coach them where they need help. But with artificial intelligence, we have the potential to make intelligence cheap for everyone, so you no longer have to worry about a huge bill for seeing a doctor or educating your child.
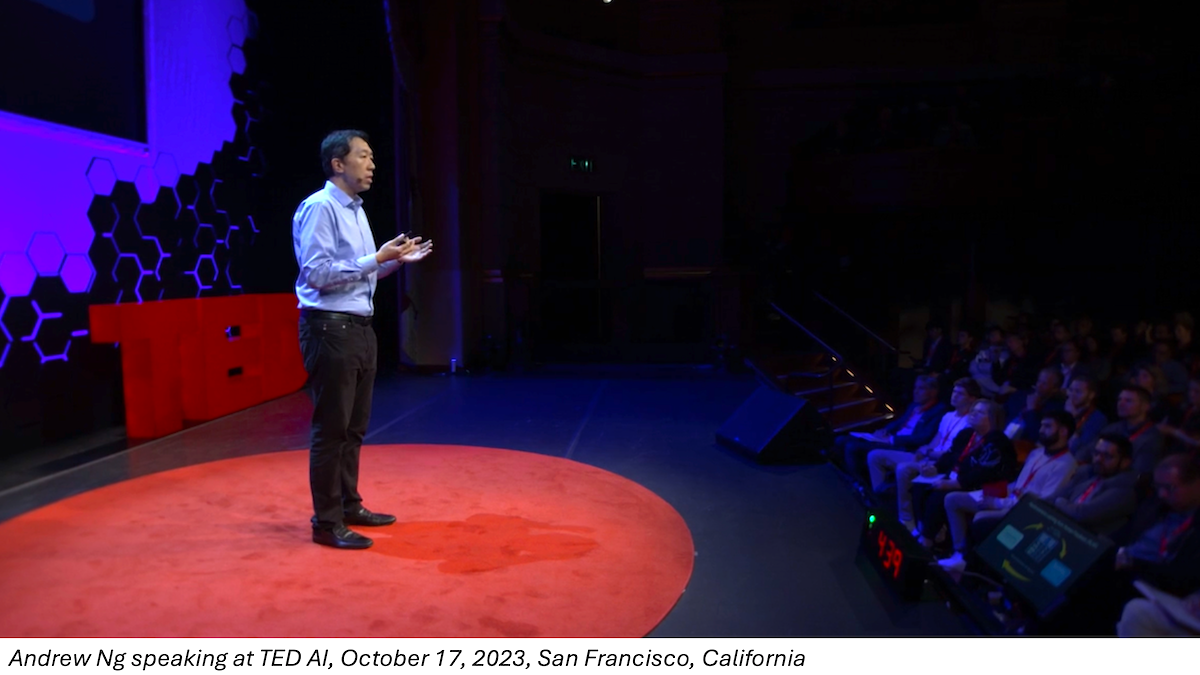
For society's biggest problems, such as climate change, intelligence — including artificial intelligence — also has a significant role to play. While having more intelligence in the world isn't the only thing (there are also nuances such as how to share the wealth it creates, how it will affect jobs, and how to keep it from being used for evil purposes), I believe we are much better off as a society with more intelligence, be it human or artificial intelligence.
In my recent talk at TED AI (you can watch the 12-minute presentation here), I touched on why I'm excited about AI and why I think many of the anxieties about it are misplaced. If you speak with someone who’s worried about AI, please forward the talk to them to see if it helps to reassure them. Or ask if they fundamentally believe we want more intelligence in the world. I find that answering this question can be a useful North Star for how we approach AI.
Keep learning!
Andrew
P.S. Check out our new short course on “Building Applications with Vector Databases,” taught by Pinecone’s Tim Tully! Vector databases (DBs) are commonly associated with retrieval augmented generation (RAG) but actually have many uses in AI applications. In this course, you’ll learn about (i) a basic semantic search app that uses a vector DB to find similar documents, (ii) a RAG application querying datasets it was not trained on, (iii) recommender systems that combine semantic search and RAG, (iv) hybrid search, which lets you work with dense and sparse vectors simultaneously, (v) anomaly detection applied to network logs, and (vi) an image-similarity application with a fun example that determines which parent a child resembles more. Come learn how you can use vector DBs to build many different types of applications! Enroll here
News
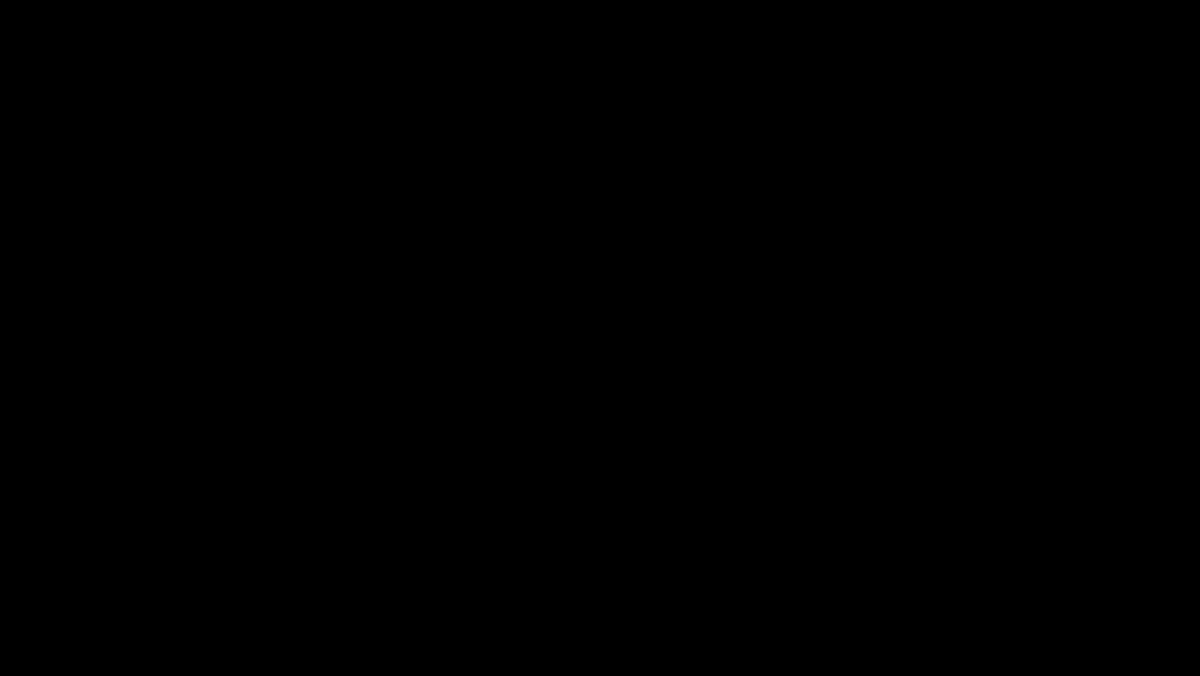
Resources for Research
The United States government wants to connect U.S. AI researchers with resources that can help them develop their projects.
What’s new: The National Artificial Intelligence Research Resource (NAIRR) announced the first call for proposals in its pilot program, which will accept applications through March 1. Winning proposals can receive processing power, data, software, and training provided by partner organizations. Another round will kick off in the second quarter of 2024.
How it works: Led by the National Science Foundation, NAIRR aims to support innovative AI research by organizing national compute and other infrastructure to be shared among researchers and educators. The initiative pulls together 10 other federal agencies and 25 partners including heavyweights like Amazon, Google, Intel, and OpenAI; startups like Allen Institute for Artificial Intelligence, Anthropic, EleutherAI, Hugging Face, and Weights & Biases; and hardware companies like AMD, Intel, and Nvidia.
- NAIRR is calling for projects that qualify as “safe, secure, and trustworthy AI.” Examples include testing and validating AI systems, reducing bias, improving privacy and security, and aligning AI with social values.
- The organization includes divisions that focus on open development, privacy and security, interoperation of partner resources, and education and outreach.
- Proposals will be evaluated based on how well they align with AI safety, security, and trustworthiness; project readiness; technical feasibility; knowledge and experience of their leaders; computing and data requirements; and need for the specific resources provided by the program.
- Researchers whose projects are accepted in the initial round will gain access to models, datasets, AI toolkits, and training provided by government partners including the Department of Defense, NASA, NOAA, the National Institutes of Health, the U.S. Patent and Trademark Office, and the National Institute for Standards and Technology. Researchers may receive time on supercomputers hosted by university and government laboratories.
- Future programs will tap private resources. Microsoft pledged $20 million in Azure computing credits and access to OpenAI models. Nvidia promised $30 million worth of access to its DGX Cloud infrastructure and enterprise software.
Behind the news: Policymakers planned to organize a national infrastructure for AI research after calls from prominent researchers. NAIRR is now open thanks to an executive order issued by the White House in October.
Why it matters: AI has potential to affect all corners of society yet, generally, only wealthy companies can bear the high costs of building and running large machine learning models. Partnership between government, industry, and academia can pool AI resources to cultivate talent throughout society and support important projects that may not serve a corporate agenda.
We’re thinking: This is an exciting bid to proliferate AI research. Sharing the fruits of such research via open publications and open source software will bring the technology’s benefits to a wider range of people.
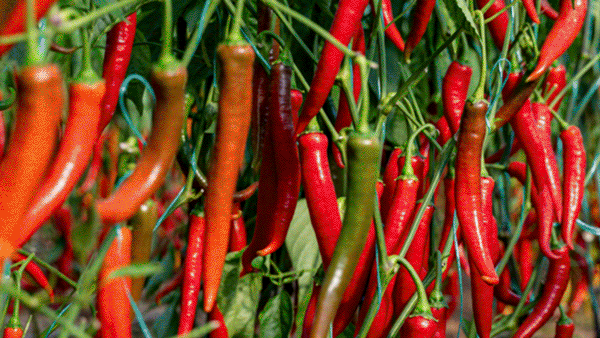
High Yields for Small Farms
Indian farmers used chatbots and computer vision to produce higher yields at lower costs.
What’s new: The state government of Telangana in South India partnered with agricultural aid organization Digital Green to provide AI tools to chili farmers.
How it works: The program, called Saagu Baagu, initially engaged 7,000 small-farm growers of chili peppers. Saagu Baagu provided AI-based tools developed by various Indian tech firms to help the farmers collect market data.
- Digital Green developed a WhatsApp chatbot in partnership with open-source developer Glific. The chatbot, which converses in the Telugu language, alerts a farmer throughout the day with suggestions depending on a crop’s maturity. Farmers can also ask questions about their crops.
- Agritech startup KrishiTantra opened a chain of local soil testing centers. Farmers test soil samples using a machine-learning-powered device that analyzes acidity, nutrient levels, and other qualities. Where traditional soil testing might take several weeks to return results, KrishiTantra’s system sends results and fertilizer recommendations to a farmer’s mobile phone in less than an hour.
- AgNext provided a computer vision system that assesses the quality of individual chilis in the field. The system detects surface defects and estimates properties such as color, shape, and size, all of which can help reduce crop waste and increase sale prices.
Results: The pilot program lasted 18 months, or three cycles of planting, growing, and harvesting peppers. Farmers in the program grew 21 percent more plants per acre while using 9 percent less pesticide and 5 percent less fertilizer, according to the World Economic Forum. Moreover, with a higher-quality harvest, the farmers increased their sale prices by 8 percent. The Telangana government has expanded the program to 500,000 farmers who grow a wider range of crops including chickpeas, cotton, groundnuts, rice, and turmeric.
Behind the news: The promise of AI-driven agriculture is attracting investments around the world. Last year, Microsoft open-sourced a suite of AI tools to analyze overhead imagery and sensor data to map soil conditions in real time and forecast temperature, precipitation, and soil moisture for days ahead.
Why it matters: Many of the Telangana farmers rely on what they can grow and sell to support themselves and their families. That makes them especially vulnerable to market fluctuations and climate change. Their situation is not unique to India. Programs like Saagu Baagu could help support small-scale farming across the world.
We’re thinking: Saagu Baagu worked in part because WhatsApp is widely popular throughout India and the chatbot spoke the local language. Smart localization that addresses local technological infrastructures, languages, and agricultural practices can proliferate the benefits of AI in agriculture.
A MESSAGE FROM DEEPLEARNING.AI
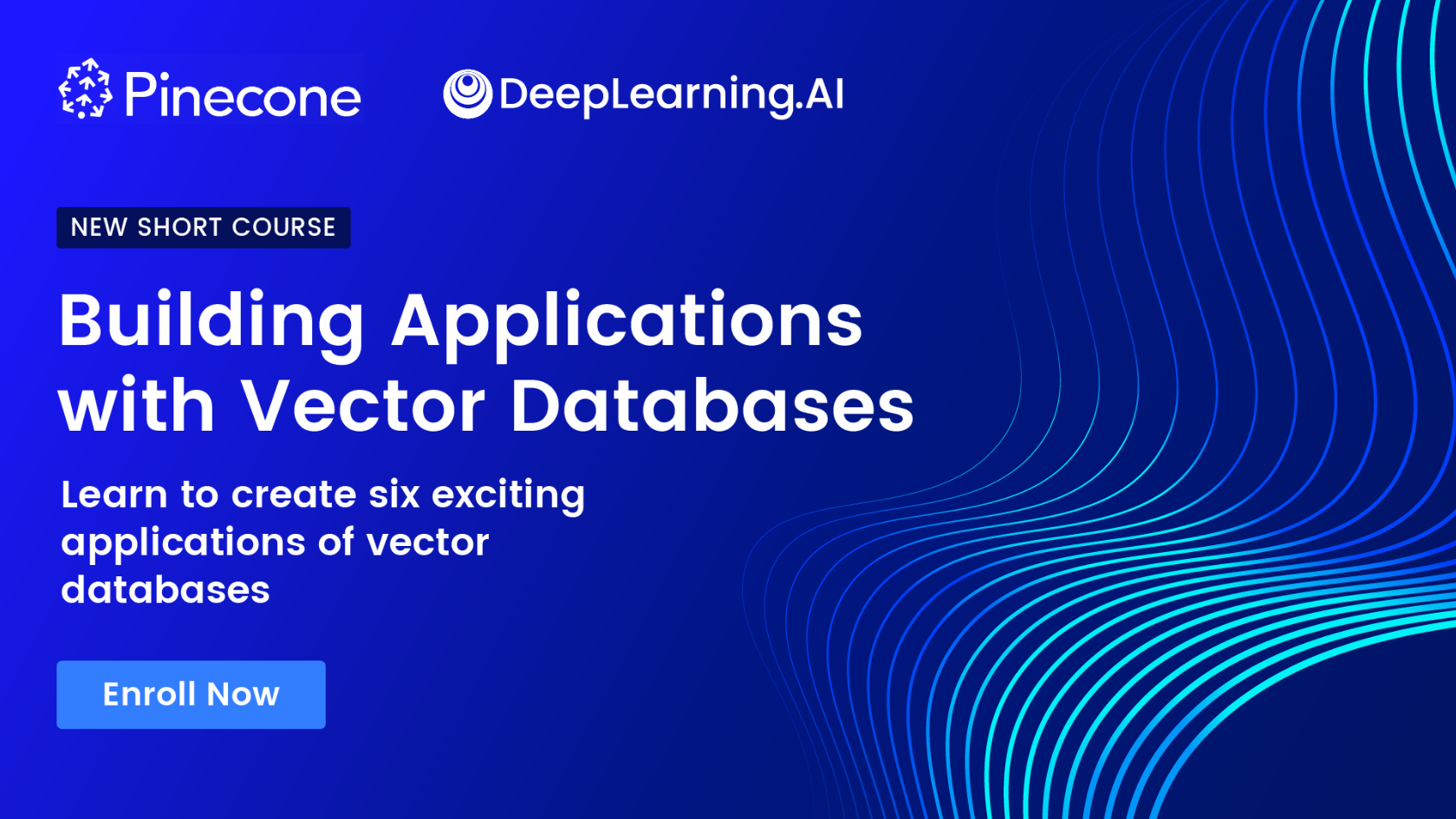
In our new course with Pinecone, you’ll learn how to build six applications that use vector databases, including retrieval augmented generation, facial similarity, and anomaly detection. Sign up now
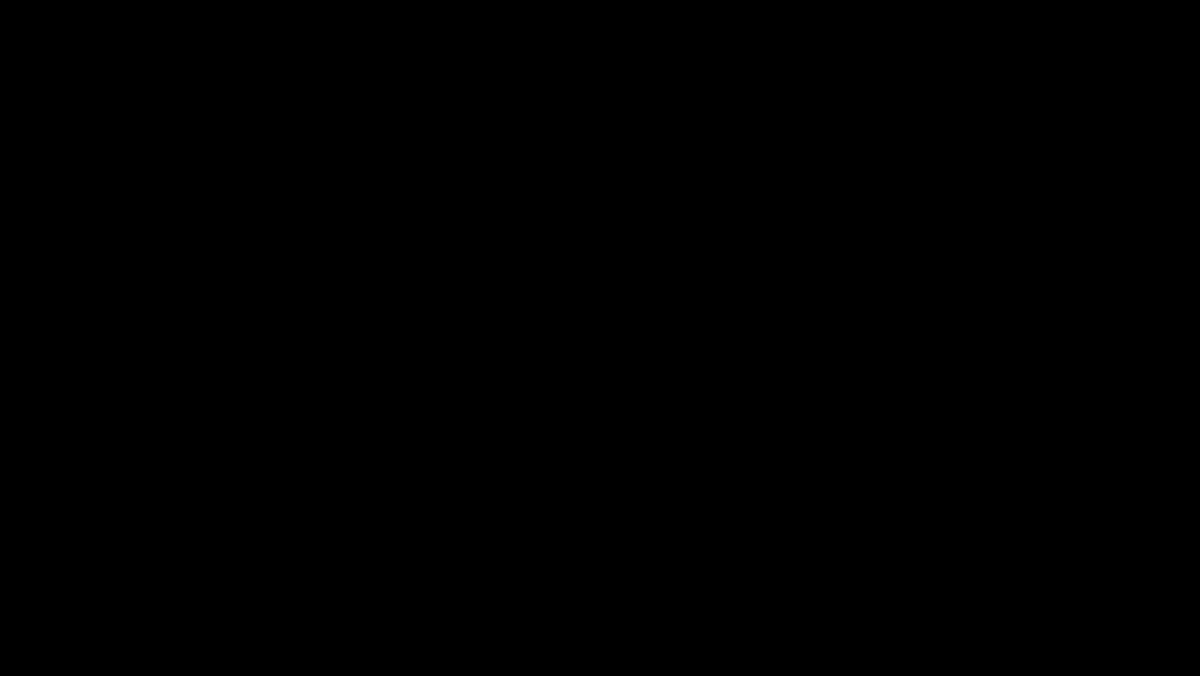
AI Jobs Grow Beyond Established Hubs
An analysis of United States job listings shows AI jobs are growing rapidly outside traditional tech hubs.
What’s new: Researchers at University of Maryland analyzed the distribution of AI jobs among U.S. job postings. California hosts the largest concentration, followed by the Washington D.C. metropolitan area (which includes more than one state).
How it works: The authors used an unspecified large language model to identify AI jobs, which they define as ones that require AI skills. They categorized each job by the U.S. state in which it was located. To determine whether a given state’s AI economy was growing or shrinking, they calculated the percentage of total U.S. AI jobs in each state in 2018 and 2023. They also calculated the percentage of each state’s total jobs that required AI skills for both dates.
- California continues to post the most U.S. AI jobs. However, California’s share of AI jobs dipped from 26 percent in 2018 to 19 percent in 2023. Still, 1.07 percent of postings in California are AI jobs, well above the national average of 0.56 percent.
- Similarly, the share of AI jobs in the state of Washington, home to Amazon and Microsoft, declined from 13 percent in 2018 to 5 percent in 2023. However, more than 1 percent of Washington postings are AI jobs.
- The combined share of Maryland, Virginia, and Washington D.C. — the U.S. capital region — rose from 7 percent in 2018 to 13 percent in 2023. The authors attributed this growth to the federal government’s embrace of AI: Companies that supply the government have responded by hiring AI experts.
- New York’s and New Jersey’s combined share of AI jobs declined from approximately 12 percent in 2018 to 11 percent in 2023.
- Meanwhile, other parts of the U.S. saw meaningful growth. Texas’ share of AI jobs grew from 6 percent of AI jobs in 2018 to over 8 percent in 2023. Florida’s share rose from 2 to 4 percent in the same time period. The combined share of 12 Midwestern states grew from 10 percent to 13 percent. However, these regions posted much smaller percentages of AI jobs relative to total jobs.
Behind the news: A 2021 Brookings report on U.S. AI jobs focused on metropolitan areas and analyzed not only job postings but also federal grants, research papers, patent filings, and companies. Despite the differences in methodology, it agreed with the new report that investment was driving AI growth outside of the Bay Area. The new report suggests a much wider geographical distribution of AI jobs in 2024 than in 2021. It appears some of the then-emerging industrial investment in AI is bearing fruit.
Why it matters: For people who aim to make a career in AI, this report contains double good news: (i) Established AI hubs in the U.S. still host the most new openings and (ii) AI jobs are growing far and wide! As the industry becomes more dispersed geographically, AI builders have more options, organizations can select from a more diverse talent pool, and the technology’s benefits can be shared more broadly.
We’re thinking: Although this report focused on the U.S., we believe that growth in AI jobs is a global trend. One contributor is growing acceptance of remote work (which remains more prevalent than it was a few years ago despite its decline as the Covid pandemic has wanted). This means more AI opportunities for everyone, everywhere!
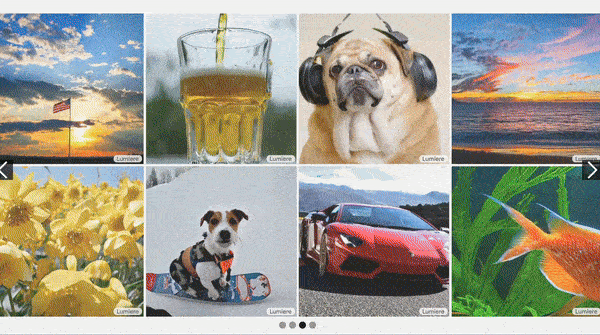
More Consistent Generated Videos
Text-to-video has struggled to produce consistent motions like walking and rotation. A new approach achieves more realistic motion.
What’s new: Omer Bar-Tal, Hila Chefer, Omer Tov, and colleagues at Google, Weizmann Institute, Tel-Aviv University, and Technion built Lumiere, a system that simplifies the usual process of generating video with improved results. You can see examples of its output here.
Key insight: Most text-to-video generators economize on memory use through a staged process: One model generates a few frames per second, another model generates additional frames between the initial ones, and a third generates a higher resolution version of every frame. Generating in-between frames can make repetitive motions inconsistent. To avoid these inconsistencies, the authors generated all frames at the same time. To bring down memory requirements, the video generator reduced the size of the video embedding before intensive processing and then restored their original size.
How it works: Lumiere borrows two components from previous work. It uses a frozen, pretrained text-to-image diffusion model (in this case, Imagen, with additional convolutional and attention layers) to generate low-resolution video frames from a text description. It uses a super-resolution model (unspecified in this case) to boost the frames’ resolution. The authors trained the layers added to Imagen on an unspecified dataset of 30 million videos (16 frames per second, 128x128 pixels per frame) and their captions.
- Given a 5-second video with added noise and its text caption, the layers added to Imagen learned to remove the noise. Following earlier work, the model saved memory by shrinking video embeddings spatially. Specifically, additional convolutional layers progressively shrank the input embedding from size (Time, Height, Width, Depth) to size (Time, Height/2, Width/2, Depth). This effectively shrank the parts of the embedding that correspond to individual frames before subjecting the entire embedding to computationally intensive attention layers. Afterward, further convolutional layers enlarged the embeddings to match the input size.
- In addition to shrinking and enlarging the video embedding spatially, the added layers learned to shrink and enlarge it temporally; that is, from size (Time, Height, Width, Depth) to size (Time/2, Height/2, Width/2, Depth). This further economized on memory usage.
- To accommodate the super-resolution model, Lumiere broke up Imagen’s 5-second video output into overlapping clips. The super-resolution model increased their resolution to 1024×1024.
- To avoid temporal artifacts from this process, Lumiere employed MultiDiffusion, which learned a weighted sum over the overlapping portions of the clips.
Results: Given one video produced by Lumiere and another produced by a competitor (AnimateDiff, Gen2, Imagen Video, Pika, or ZeroScope), judges compared video quality and alignment with the text prompt used to generate a video. For each competitor, they evaluated 400 videos for each of 113 prompts. Comparing video quality, Lumiere beat the best competitor, Gen2, 61 percent to 39 percent. Comparing alignment with the prompt, Lumiere beat the best competitor, ImagenVideo, 55 percent to 45 percent.
Why it matters: Earlier video generators produced output with limited motion or motion with noticeable issues (for example, a character’s body shape might change in unexpected ways). By producing all video frames at once, Lumiere generates images of motion without such issues.
We’re thinking: Lumiere's approach hints at both the challenge of generating video and the pace of development. Many further refinements are needed to make such systems as useful as, say, ChatGPT, but recent progress is impressive.
Data Points
This week on Data Points we spotlight updates in the legal AI sector, China’s freshly introduced regulations for robotaxis, the widely discussed paper on sleeper agents, plans in the EU to build ‘AI factories,’ and more.