Dear friends,
As I wrote in an earlier letter, whether AI is sentient or conscious is a philosophical question rather than a scientific one, since there is no widely agreed-upon definition and test for these terms. While it is tempting to “solve” this problem by coming up with precise definitions and well defined tests for whether a system meets them, I worry that poor execution will lead to premature declarations of AI achieving such criteria and generate unnecessary hype.
Take the concept of self-awareness, which refers to a conscious knowledge of one's own self. Suppose we define a robot as self-aware if it can recognize itself in the mirror, which seems a natural way to test a robot’s awareness of itself. Given this definition — and that it’s not very hard to build a robot that recognizes itself — we would be well on a path to hype about how AI was now self-aware.
This example isn’t a prediction about the future. It actually happened about 10 years ago, when many media sources breathlessly reported that a robot “Passes Mirror Test, Is Therefore Self-Aware … conclusively proving that robots are intelligent and self-aware.”
While bringing clarity to ambiguous definitions is one way for science to make progress, the practical challenge is that many people already have beliefs about what it means for something to be self-aware, sentient, conscious, or have a soul. There isn’t widespread agreement on these terms. For example, do all living things have souls? How about a bacterium or virus?
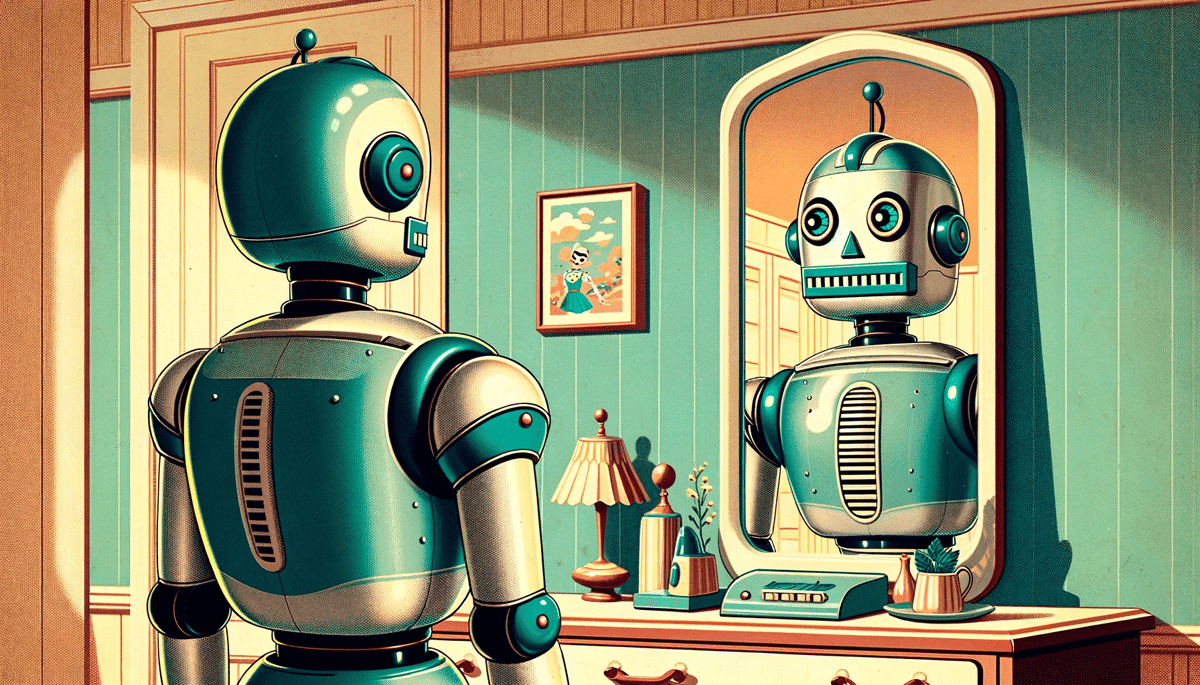
So even if someone comes up with a reasonable new scientific definition, many people — unaware of the new definition — will still understand the term based on their earlier understanding. Then, when media outlets start talking about how AI has met the definition, people won’t recognize that the hype refers to a narrow objective (like a robot recognizing itself in the mirror). Instead, they’ll think that AI accomplished what they generally associate with words like sentience.
Because of this, I have mixed feelings about attempts to come up with new definitions of artificial general intelligence (AGI). I believe that most people, including me, currently think of AGI as AI that can carry out any intellectual task that a human can. With this definition, I think we’re still at least decades away from AGI. This creates a temptation to define it using a lower bar, which would make it easier to declare success: The easiest way to achieve AGI might be to redefine what the term means!
Should we work to clarify the meanings of ambiguous terms that relate to intelligence? In some cases, developing a careful definition and getting widespread agreement behind it could set a clear milestone for AI and help move the field forward. But in other cases, I’m satisfied to avoid the risk of unnecessary hype and leave it to the philosophers.
Keep learning!
Andrew
P.S. LLMOps is a rapidly developing field that takes ideas from MLOps (machine learning operations) and specializes them for building and deploying LLM-based applications. In our new course, “LLMOps,” taught by Google Cloud’s Erwin Huizenga, you’ll learn how to use automation and experiment tracking to speed up development. Specifically, you’ll develop an LLMOps pipeline to automate LLM fine-tuning. By building a tuning pipeline and tracking the experiment artifacts — including the parameters, inputs, outputs, and experimental results — you can reduce manual steps in the development process, resulting in a more efficient workflow. Sign up here!
News
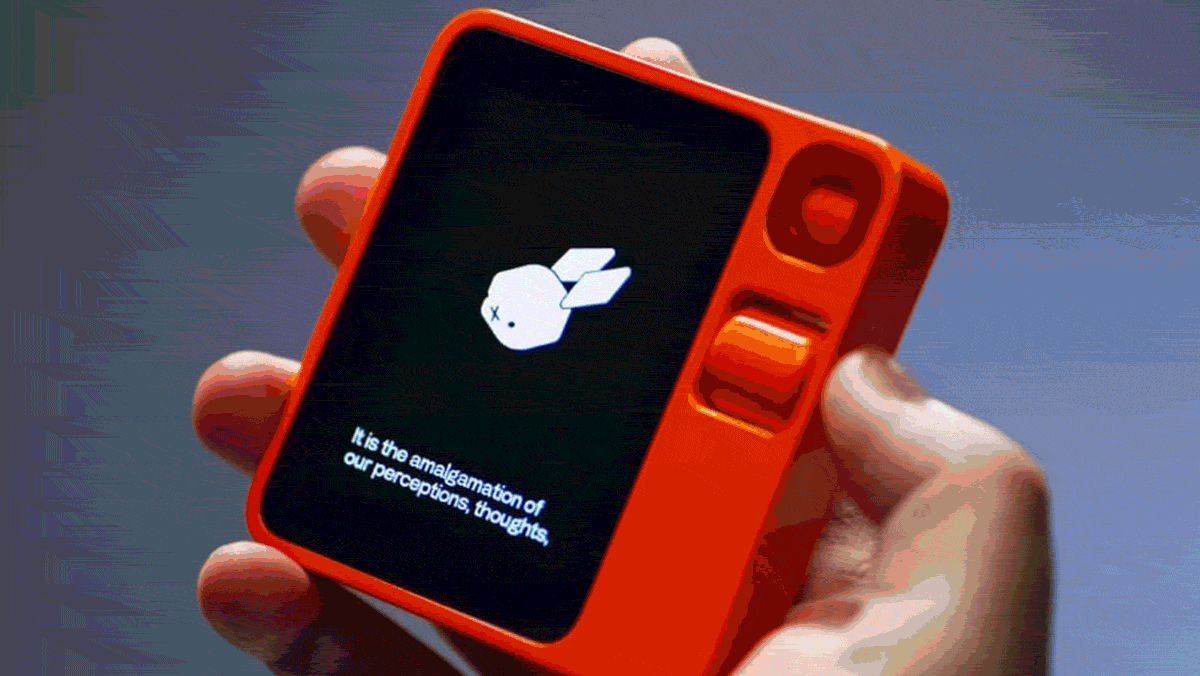
AI Busts Out at CES
The 2024 Consumer Electronics Show in Las Vegas showcased products that take advantage of increasingly powerful, increasingly accessible AI capabilities.
What’s new: Many debuts at the massive CES show showed that large language models (LLMs) are moving beyond browsers and smartphones.
Best of show: The show’s surprise hit was a portable personal assistant. LLM-powered automobile dashboards and an AI accelerator card also stood out.
- Rabbit’s R1 ($199, cellular service required) is among a new wave of AI-optimized hardware devices, including the Humane AI Pin, TranscribeGlass voice transcription display, and Timekettle language translators, that seek to usurp smartphone capabilities. The R1 accepts voice commands to play music, call a car, order food, reserve flights, and the like by interacting with services like Spotify and Uber. The hand-held unit houses a touchscreen, camera, wheel-and-button controller, and cellular modem. It uses a proprietary “large action model” based on attention and graph neural networks; the model learns by mimicking how people use web interfaces and runs in the cloud to translate voice commands into actions via a web portal. The R1 will be available in March and has already sold out through June. A future update will enable users to teach the device new skills, like editing images or playing video games, by demonstrating them in view of the camera.
- Volkswagen and Mercedes Benz demonstrated dashboard voice assistants equipped with large language models. Along with the usual navigation and entertainment, the new consoles deliver personalized information like nearby service stations or restaurants. Powered by OpenAI and automotive AI developer Cerence, Volkswagen’s system will be standard in most vehicles beginning in the spring. Mercedes’ MB.OS will be available next year.
- Taiwanese startup Neuchips displayed an add-in board that enables desktop computers to run large language models like the 7 billion-parameter version of Llama 2. The Evo PCIe AI accelerator is optimized for transformer networks to provide comparable performance to GPUs while consuming less electricity (55 watts versus an Nvidia RTX 4080’s 320 watts). The card will be available later this year at an undisclosed price. Versions outfitted with four or more chips are on the company’s roadmap.
Why it matters: Flashy CES demos often mask underdeveloped products and vaporware. But this year, AI for processing voice, text, and images is mature enough to enable product designers to focus on everyday use cases and intuitive user experiences. While some of this year’s AI-powered debuts seemed like overkill — for instance, the computer vision-equipped Flappie cat door that won’t open while your pet has a mouse in its jaws — others suggest that startups and giants alike are rethinking the technology’s capacity to simplify and enhance daily life and work.
We’re thinking: Not long ago, simply connecting a home appliance to the internet earned the designation “smart.” Increasingly, AI is making that label credible.
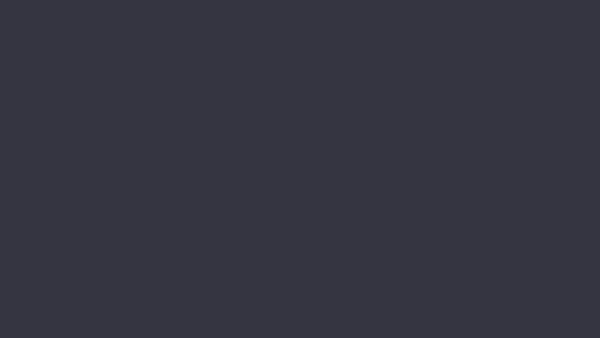
OpenAI Expands Platform Play
The GPT Store is open for business, providing curated, searchable access to millions of chatbots tailored for specific purposes.
What’s new: OpenAI launched the GPT Store for paid ChatGPT accounts, making it far easier to find useful GPTs (instances of ChatGPT conditioned by user-submitted prompts). The store lets subscribers browse by category, search by keywords, and create their own chatbots. The company introduced GPTs in November as a free offering without search or curation.
How it works: Access to the store is rolling out in phases and isn’t yet available to all subscribers as of this writing.
- The store organizes GPTs in categories such as education, productivity, and programming as well as those that prompt the DALL·E image generator. It also highlights “featured” and “trending” GPTs and branded offerings from companies like AllTrails (hiking/running routes and advice), Canva (graphic design), and Consensus (scientific literature search).
- Users can create GPTs by selecting the editor and prompting ChatGPT with instructions for chatbot’s function and what information it can access; for example, “Make an app that creates an auction listing for an uploaded photo of any item.” The system asks follow-up questions to refine the GPT’s scope, likely users, and the like. Completed GPTs can be listed publicly in the store directory.
- OpenAI plans to launch a revenue sharing program to reward creators of popular GPTs. Further details are not yet available.
Why it matters: The GPT Store strengthens ChatGPT’s utility as a platform for others to build upon and seems designed to drive paid subscriptions. It enables developers to share applications based on OpenAI’s technology and holds out hope that they’ll be rewarded for their effort.
We’re thinking: The GPT concept enables anyone, even without a background in coding, to build and share powerful applications quickly and easily. The current implementation seems like a toe in the water. If it proves popular, it could significantly deepen OpenAI’s moat, as the Apple and Android stores have done for Apple and Google respectively.
A MESSAGE FROM DEEPLEARNING.AI
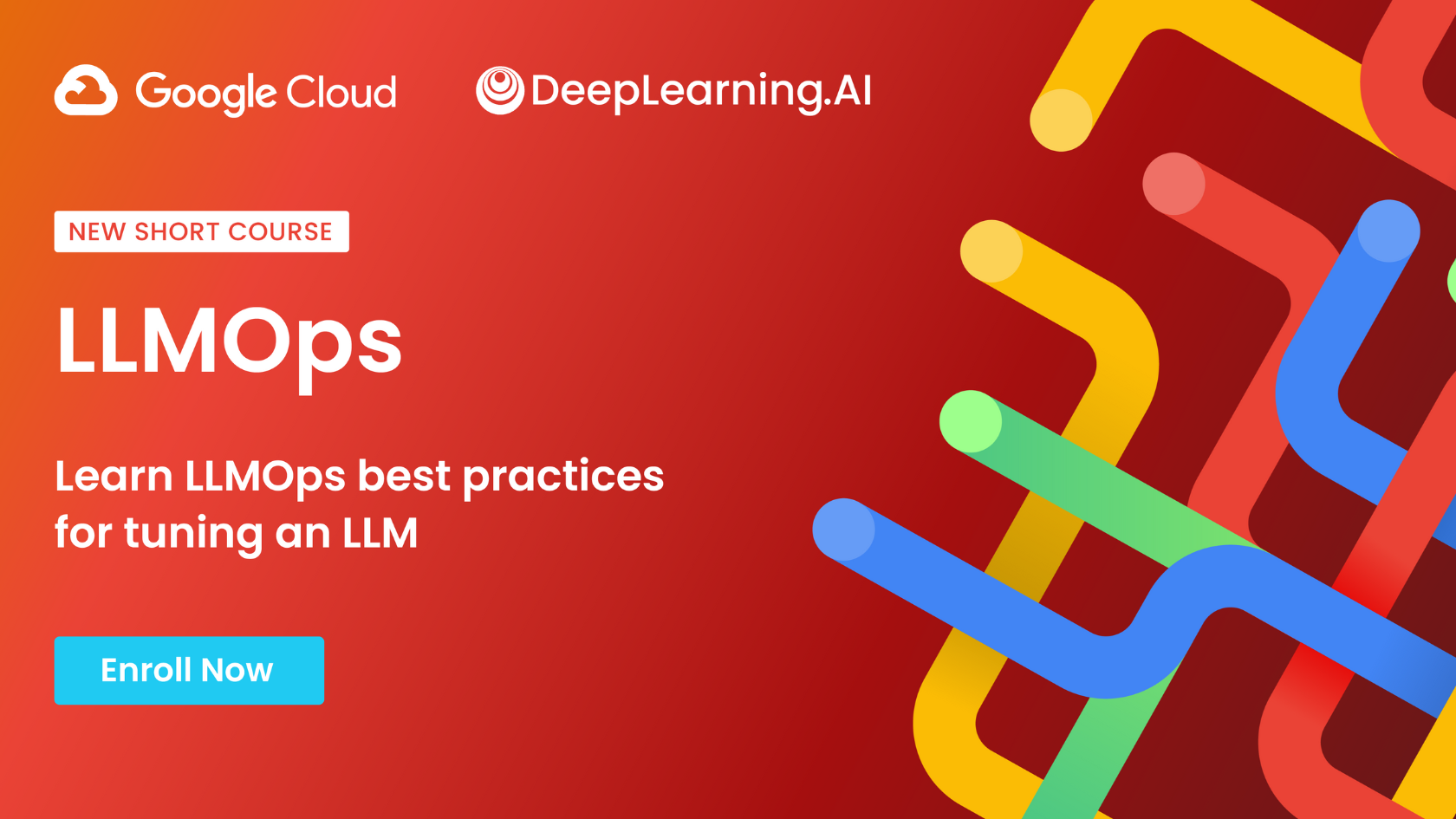
Learn about machine learning operations for large language models (LLMOps) in our new short course, built in collaboration with Google Cloud. Explore the LLMOps pipeline for pre-processing data, fine-tuning LLMs, and deploying custom LLMs tailored to your applications. Enroll now
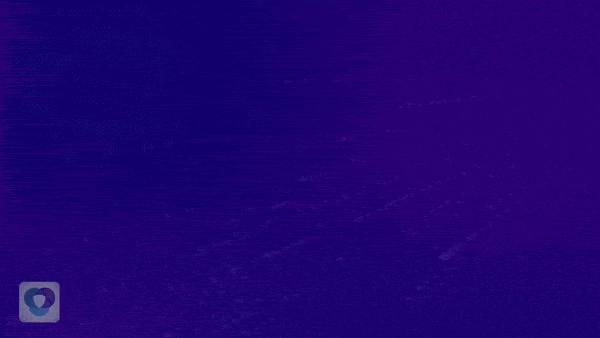
Standard for Media Watermarks
An alliance of major tech and media companies introduced a watermark designed to distinguish real from fake media starting with images.
What’s new: The Coalition for Content Provenance and Authenticity (C2PA) offers an open standard that marks media files with information about their creation and editing. C2PA’s 30 members, including both tech powers (Adobe, Google, Intel, Microsoft, X) and media outlets (BBC, CBC, The New York Times) will deploy the standard in the coming year, IEEE Spectrum reported.
How it works: The C2PA’s Content Credentials specification accommodates a variety of file types, but currently it’s implemented mainly for images.
- When a C2PA-compliant image generator or editor produces an image, it invisibly embeds a cryptographic watermark that contains the following metadata: the user or device that initially created the image, when and how it was created, and how it was edited or otherwise transformed. (Actions using non-compliant tools are not recorded.)
- Images can display a small “cr” icon in the corner. Clicking on the icon reveals the metadata.
- Any alteration of the file or any attempt to tamper with it will cause a mismatch between the watermark and its associated metadata.
- Social media recommenders and image search algorithms can use the metadata to identify, restrict, or promote certain types of media.
Who’s using it: Image generators from Adobe and Microsoft stamp their outputs with Content Credential watermarks, marking them as synthetic; Microsoft also promotes watermarking by political campaigns to help voters differentiate synthetic from non-generated campaign messages. Camera manufacturers Canon, Leica, and Nikon have built prototype cameras that use Content Credentials to mark the origin of photographs. BBC is using the technology to mark images on its website on a trial basis, and Canada’s CBC plans to deploy it in mid-2024.
Yes, but: It may be difficult to fake Content Credentials, but it’s easy to remove the watermark from images, even from AI-generated ones. Using a Content Credentials-compliant tool like Photoshop, you can disable Content Credentials and save a watermarked image to a different format. This produces an identical image without the watermark.
Behind the news: The C2PA unites the Content Authenticity Initiative (led by Adobe) and Project Origin (led by media companies). Nonetheless, the field remains fragmented. For instance, Meta (not a C2PA member) has aimed to identify AI-generated media using detection software. However, C2PA argues that detectors aren’t sufficiently effective; the winner of a Meta deepfake-detection challenge identified generated content only 65 percent of the time. Top AI companies committed to developing their own watermarking mechanisms, but they haven’t settled on Content Credentials or another standard.
Why it matters: Distinguishing generated text, imagery, and audio from media that accurately depicts real-world events is a key challenge for the generative AI era. The coming year will test that ability as 78 countries gear up elections that will affect roughly half the world’s population. Already, campaigns have used generated imagery in Argentina, New Zealand, South Korea, the United States, and other nations. Google and Meta responded by tightening restrictions on political advertisers’ use of generative AI. The EU’s AI Act will require clear labeling of AI-generated media, and the U.S. Federal Election Commission plans to restrict ads that depict political opponents saying or doing things they did not actually say or do. If Content Credentials proves effective in the coming election season, it may ease the larger problem of identifying generated media in a variety of venues where authenticity is important.
We’re thinking: A robust watermark can identify both traditional and AI-generated media for users and algorithms to treat accordingly. It can also potentially settle claims that a doctored image was authentic or that authentic work was doctored. However, we worry that watermarking generated outputs may prove to be a disadvantage in the market, creating a disincentive for makers of software tools to provide it and users to use it. With heavyweight members from both tech and media, C2PA may be able to build sufficient momentum behind the watermarking to make it stick.
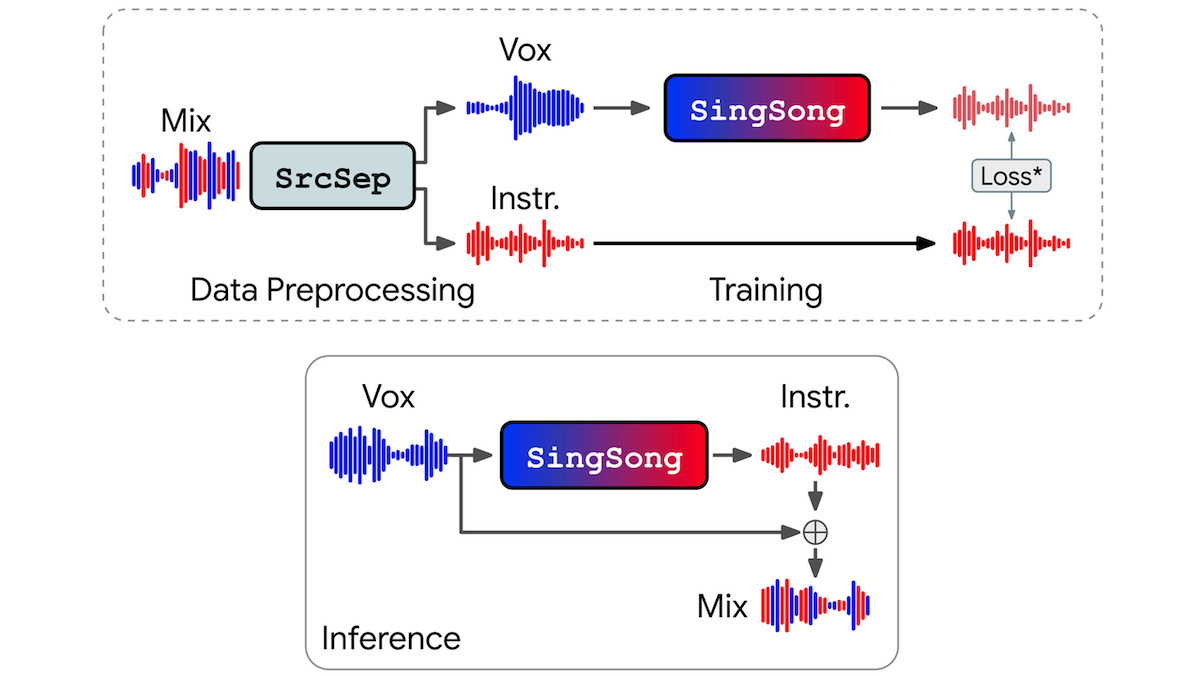
Sing a Tune, Generate an Accompaniment
A neural network makes music for unaccompanied vocal tracks.
What's new: Chris Donahue, Antoine Caillon, Adam Roberts, and colleagues at Google proposed SingSong, a system that generates musical accompaniments for sung melodies. You can listen to its output here.
Key insight: To train a machine learning model on the relationship between singers’ voices and the accompanying instruments, you need a dataset of music recordings with corresponding isolated voices and instrumental accompaniments. Neural demixing tools can separate vocals from music, but they tend to leave remnants of instruments in the resulting vocal track. A model trained on such tracks may learn to generate an accompaniment based on the remnants, not the voice. Then, given a pure vocal track, it can’t produce a coherent accompaniment. One way to address this issue is to add noise to the isolated voices. The noise drowns out the instrumental remnants and forces the model to learn from the voices.
How it works: The authors based their approach on AudioLM, a system that generates audio by attending to both small- and large-scale features.
- The authors built a dataset of 1 million recordings that totaled 46,000 hours of music. They separated the recordings into voices and instrumental accompaniments using a pretrained MDXNet and divided the recordings into 10-second clips of matching isolated vocal and instrumental tracks. They added noise to the vocal tracks.
- Following AudioLM and its successor MusicLM, the authors tokenized the instrumental tracks at two time scales to represent large-scale compositional features and moment-to-moment details. A w2v-BERT pretrained on speech plus the authors’ initial dataset produced 25 tokens per second. A SoundStream audio encoder-decoder pretrained on speech, music, and the authors’ initial dataset produced 200 tokens per second.
- To represent the noisy vocal tracks, they produced 25 tokens per second using the w2vBERT.
- They trained a T5 transformer, given vocal tokens, to generate the corresponding instrumental tokens.
- Given the instrumental tokens, a separate transformer learned to generate tokens for SoundStream’s decoder to reconstruct the instrumental audio.
- To generate an instrumental track, the authors fed tokens produced by the transformer to SoundStream’s decoder.
Results: Listeners compared 10-second clips from the test set of MUSDB18, a dataset that contains 10 hours of isolated vocal and instrumental tracks. Each clip came in multiple versions that paired the original vocal with accompaniment supplied by (i) SingSong, (ii) a random instrumental track from MUSDB18’s training set, (iii) the instrumental track from MUSDB18’s training set most similar to the vocal in key and tempo according to tools in the Madmom library, and (iv) the original instrumental track. The listeners preferred SingSong to the random accompaniment 74 percent of the time, to the most similar accompaniment 66 percent of the time, and to the original instrumental track 34 percent of the time.
Why it matters: The authors used data augmentation in an unusual way that enabled them to build a training dataset for a novel, valuable task. Typically, machine learning practitioners add noise to training data to stop a model from memorizing individual examples. In this case, the noise stopped the model from learning from artifacts in the data.
We’re thinking: Did you always want to sing but had no one to play along with you? Now you can duet yourself.
Data Points
From new marketplace rules for video games to car companies integrating generative AI into their products, dive into more top news, curated and summarized for you on Data Points, a spin-off of The Batch: