Dear friends,
AI is progressing faster than ever. This is thrilling, yet rapid change can be disorienting. In such times, it’s useful to follow Jeff Bezos’ advice to think about not only what is changing but also what will stay the same. If something doesn’t change, investing energy and effort in it is more likely to be worthwhile.
Here are some things in AI that I’m confident won’t change over the next decade:
- We need community. People with friends and allies do better than those without. Even as the AI world brings breakthroughs seemingly every week, you’ll be better off with friends to help sort out what’s real and what’s hype, test your ideas, offer mutual support, and build things with.
- People who know how to use AI tools are more productive. People and businesses that know how to manipulate data are more effective at getting at the truth, making better decisions, and accomplishing more. This will only become more true as AI continues to progress.
- AI needs good data to function well. Just as humans need good data to make decisions ranging from what marketing strategy to pursue to what to feed a child, AI need will good data even as our algorithms continue to scale, evolve, and improve.
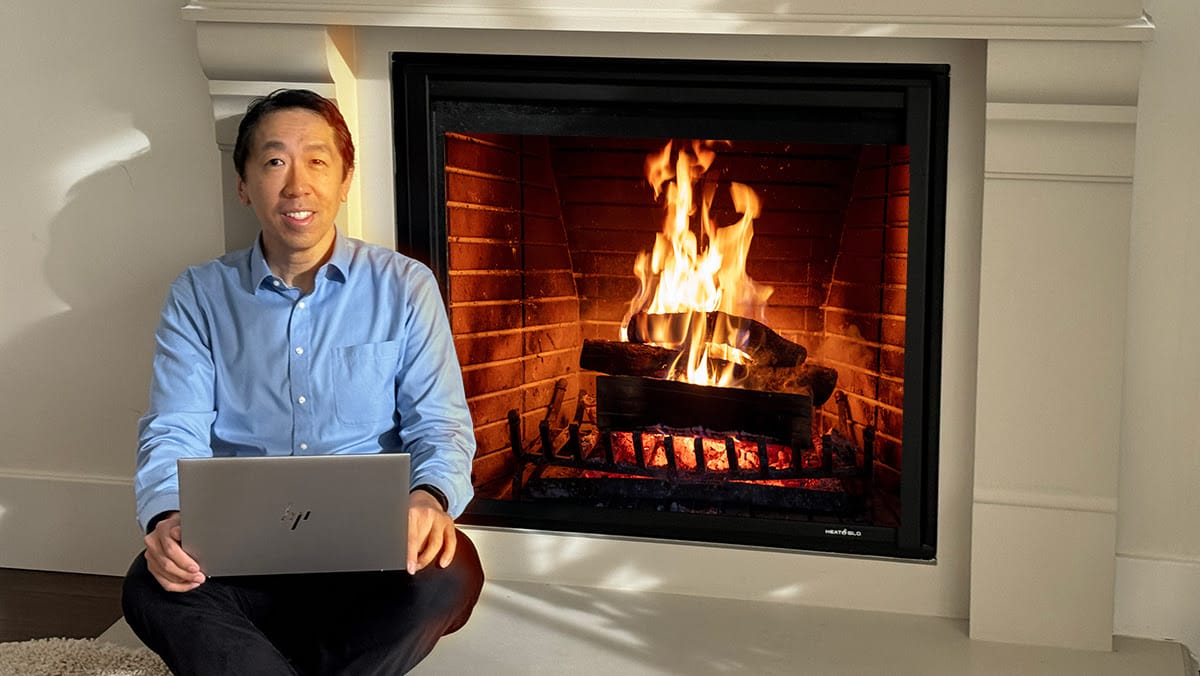
What does this mean for each of us? Taking the points above in turn:
- Let’s keep building the AI community. This is important! I hope you’ll share what you learn with others, motivate each other, and continue to find friends and collaborators. While we do our best in The Batch to cover what matters in AI, having a close group of friends to talk these things over with can deepen your knowledge and sharpen your ideas.
- Keep learning! Even better, make learning a habit. It can keep you more productive, among many other benefits. If you’re thinking about 2024 new year resolutions, include your learning goals. As AI continues to evolve, everyone needs a plan to keep up with — and in some cases even take a role in accelerating — this wave.
- Continue to cultivate data-centric AI practices. As businesses adopt more AI tools, I find that one of the most important practices is to keep control of your own data. I think this will grow in importance for individuals too. I'll say more about this in a future letter.
While the three points above relate to AI, I want to share two other things that I’m confident will, unfortunately, stay the same over the next decade: (i) Climate change will continue to be a major challenge to humanity. (ii) Poverty, where many people can barely (or perhaps not even) afford basic necessities, will remain a problem. I will continue to think about how AI climate modeling can help the former and how we can use AI to lift up everyone.
Through this exciting time, I’m grateful to be connected to you. I look forward to navigating with you the changes — and constants — of 2024.
Happy new year!
Andrew
Generating 2024
Only one year into the mainstreaming of generative AI, a wondrous horizon stretches before us. We no longer search the internet, we chat with it; we don’t write emails, we ask our assistant in the cloud to do it. We converse with code, conjure images, mold video clips. This new world holds great promise, but also great worries about whether these powers will serve all of us, and serve us well. So, as in years past, we asked some of AI’s brightest minds: What is your hope for the coming year? Their answers hold clues to the future and our place in it.
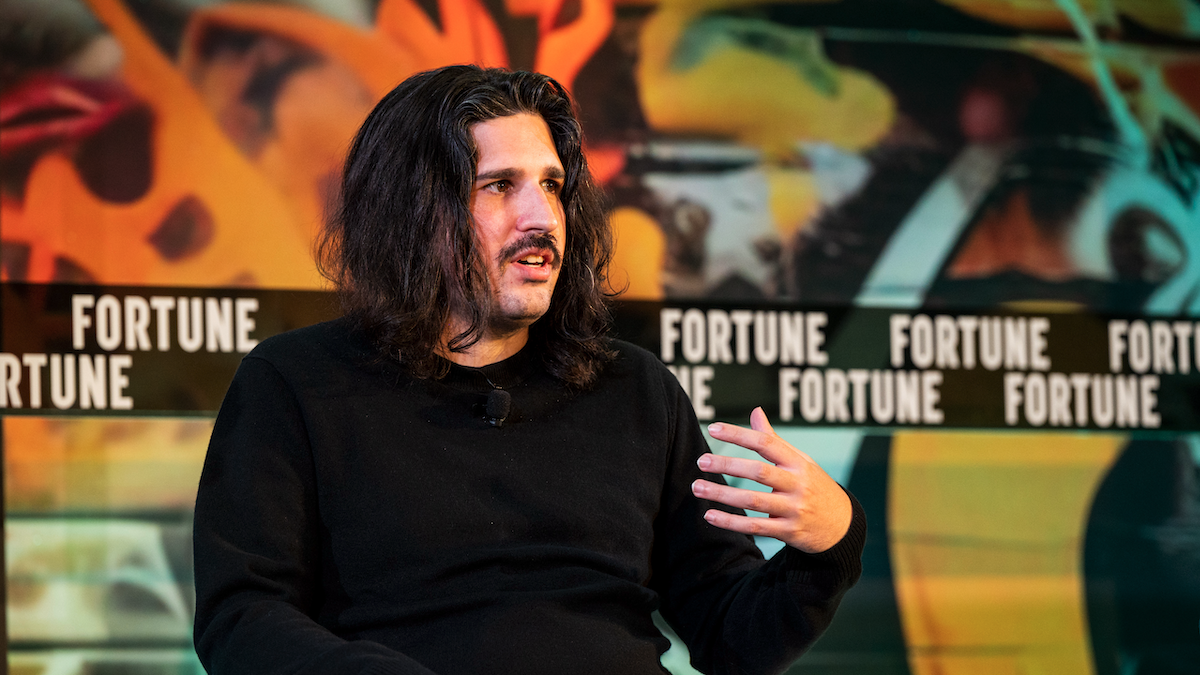
Anastasis Germanidis: New Tools to Tell New Stories
The year 2023 was an inflection point in the development of broadly useful AI systems across text, image, video, audio, and other modalities. At Runway alone, we saw the release of video-generation models such as Gen-1 and Gen-2, as well as tools that enable new forms of creative control with those models. In the coming year, here are some areas where I expect to see continued progress:
- Video generation: Over the past year, generative video models (text-to-video, image-to-video, video-to-video) became publicly available for the first time. In the coming year, the quality, generality, and controllability of these models will continue to improve rapidly. By the end of 2024, a nontrivial percentage of video content on the internet will take advantage of them in some capacity.
- Real-time interactivity: As large models become faster to run and we develop more structured ways to control them, we’ll start to see more novel user interfaces and products emerge around them that go beyond the usual prompt-to-x or chat-assistant paradigms.
- Automating AI research: Developers have embraced coding assistants based on large language models such as GitHub Copilot. But little tooling has been designed to accelerate AI research workflows specifically; for instance, automating a lot of the repetitive work involved in developing and debugging model code, training and evaluating models, and so on. More of these tools will emerge in the coming year.
- More emphasis on systems: Much conversation has focused on the capabilities of individual networks trained end-to-end. In practice, however, AI systems deployed in real-world settings are usually powered by a pipeline of models. More frameworks will appear for building such modular systems.
Beyond technological advancements, the most rewarding part of building these systems is that, with every update and increase in capabilities, new audiences are introduced to them and new stories are told that weren’t told before. I’m excited to see how that will continue to happen in the coming year.
Anastasis Germanidis is co-founder and CTO of Runway, an applied AI research company shaping the next era of art, entertainment, and human creativity.
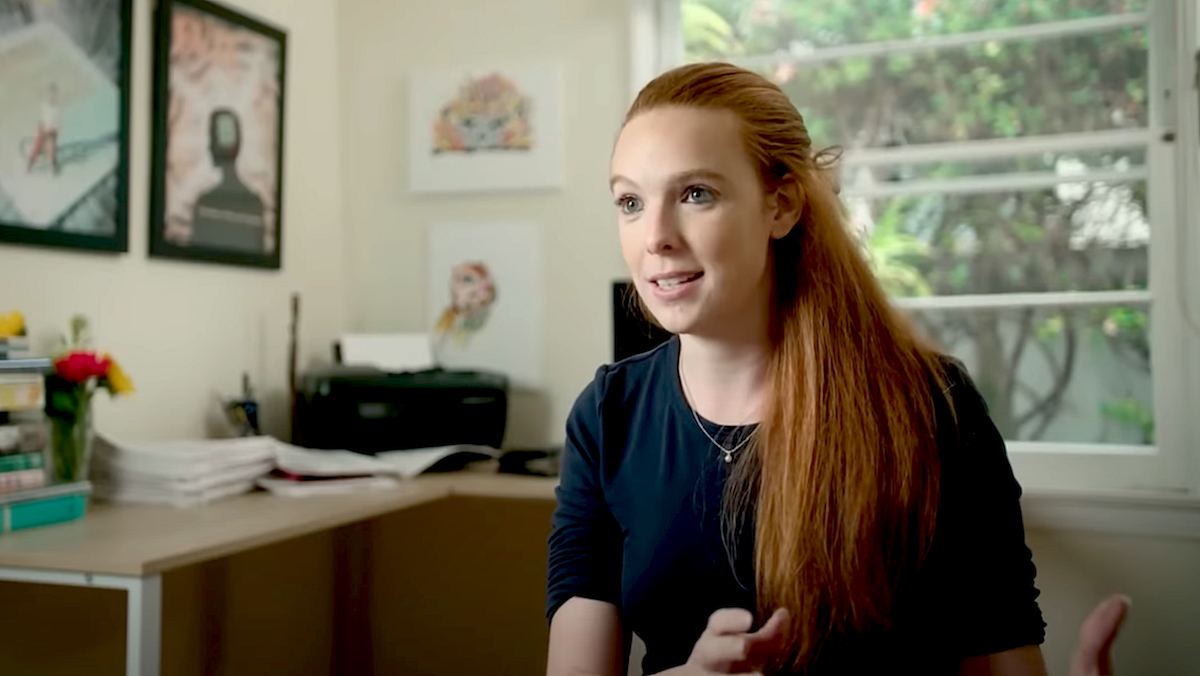
Sara Hooker: Prioritize Inclusion
The past year has seen incredible innovation in AI, and I expect as much or more in 2024. The coming year undoubtedly will be a year of rapid progress in models – multimodal, multilingual, and (hopefully) smaller and faster.
To date, models and datasets used for training have been heavily biased towards English-speaking and Western European countries, offering little representation of languages from the Global South and Asia. Even when languages from the Global South are represented, the data almost always is translated from English or Western European languages. In 2023, the “rich got richer” and the “poor got poorer” as breakthroughs facilitated use of widely spoken languages like English while further impeding access for speakers of languages for which much less data is available.
Next year will be the year of Robin Hood, when we try to reshare the gains by closing the language gap. We will see rapid improvement in state-of-the-art multilingual models, as well as innovation in synthetic data generation to build foundation models for specific languages. I believe we will make progress in closing the language gap and strengthen our collective effort to incorporate research, training data, and individuals from across the globe. This will include projects like Aya, a model from Cohere For AI that will cover 101 languages. Bridging the gap is not just a matter of inclusivity, it’s key to unlocking the transformative power of AI and ensuring that it can serve a global audience, irrespective of language or cultural background.
In addition, I expect 2024 to be a year for research bets. Multimodal will become a ubiquitous term as we move away from subfields dedicated to language, computer vision, and audio in isolation. Models will be able to process multiple sensory inputs at once, more like humans. We will care urgently about model size as we deploy more models in resource-constrained environments. AI models will become smaller and faster. Our lab is already pushing the limits of efficiency at scale, data pruning, and adaptive computing. Localization of models using retrieval augmented generation (RAG) and efficient fine-tuning will be paramount, as everyday users look to unlock the potential in frontier models.
In the coming year, it will be even more important to interrogate the defaults of where, how, and by whom research is done. To date, state-of-the-art models have come from a handful of labs and researchers. The community responsible for recent breakthroughs is so small that I know many of the people involved personally. However, we need to broaden participation in breakthroughs to include the best minds. At Cohere For AI, we are in the second cohort of our Scholars Program, which provides alternative points of entry into research for AI talent around the world.
The compute divide will persist in the coming year. Shortages of compute combined with stockpiling of GPUs mean there won’t be immediate changes in the availability of compute. This year, we launched our research grant program, so independent and academic researchers can access frontier models at Cohere. More needs to be done at national and global scales to bridge the divide for researchers and practitioners.
We are in an interesting time, and it is rare to work on research that is being adopted so quickly. Our ideas not only resonate in AI conferences but have a profound impact on the world around us. In 2024, expect more rapid change and some breakthroughs that make this technology immediate and usable to more humans around the world. By prioritizing inclusivity in model training and fundamental research, we can help ensure that AI becomes a truly global technology, accessible to users from all backgrounds.
Sara Hooker is a senior VP of research at Cohere and leads Cohere For AI, a nonprofit machine learning research lab that supports fundamental enquiry and broad access.
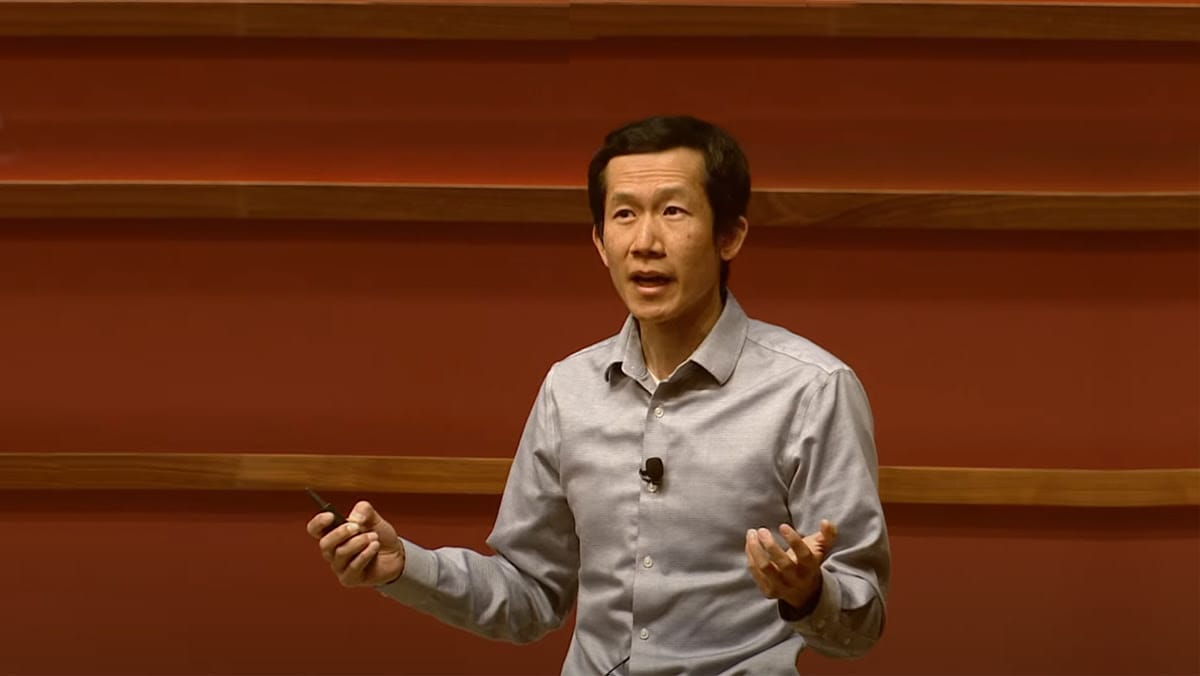
Percy Liang: Transparency for Foundation Models
Only a year ago, ChatGPT woke the world up to the power of foundation models. But this power is not about shiny, jaw-dropping demos. Foundation models will permeate every sector, every aspect of our lives, in much the same way that computing and the Internet transformed society in previous generations. Given the extent of this projected impact, we must ask not only what AI can do, but also how it is built. How is it governed? Who decides?
We don’t really know. This is because transparency in AI is on the decline. For much of the 2010s, openness was the default orientation: Researchers published papers, code, and datasets. In the last three years, transparency has waned. Very little is known publicly about the most advanced models (such as GPT-4, Gemini, and Claude): What data was used to train them? Who created this data and what were the labor practices? What values are these models aligned to? How are these models being used in practice? Without transparency, there is no accountability, and we have witnessed the problems that arise from the lack of transparency in previous generations of technologies such as social media.
To make assessments of transparency rigorous, the Center for Research on Foundation Models introduced the Foundation Model Transparency Index, which characterizes the transparency of foundation model developers. The good news is that many aspects of transparency (e.g., having proper documentation) are achievable and aligned with the incentives of companies. In 2024, maybe we can start to reverse the trend.
By now, policymakers widely recognize the need to govern AI. In addition to transparency, among the first priorities is evaluation, which is mentioned as a priority in the United States executive order, the European Union AI Act, and the UK’s new AI Safety Institute. Indeed, without a scientific basis for understanding the capabilities and risks of these models, we are flying blind. About a year ago, the Center for Research on Foundation Models released the Holistic Evaluation of Language Models (HELM), a resource for evaluating foundation models including language models and image generation models. Now we are partnering with MLCommons to develop an industry standard for safety evaluations.
But evaluation is hard, especially for general, open-ended systems. How do you cover the nearly unbounded space of use cases and potential harms? How do you prevent gaming? How do you present the results to the public in a legible way? These are open research questions, but we are on a short fuse to solve them to keep pace with the rapid development of AI. We need the help of the entire research community.
It does not seem far-fetched to imagine that ChatGPT-like assistants will be the primary way we access information and make decisions. Therefore, the behavior of the underlying foundation models — including any biases and preferences — is consequential. These models are said to align to human values, but whose values are we talking about? Again, due to the lack of transparency, we have no visibility into what these values are and how they are determined. Rather than having these decisions made by a single organization, could we imagine a more democratic process for eliciting values? It is the integrity and legitimacy of the process that matters. OpenAI wants to fund work in this area, and Anthropic has some research in this direction, but these are still early days. I hope that some of these ideas will make their way into production systems.
The foundation-models semi truck will barrel on, and we don’t know where it is headed. We need to turn on the headlights (improve transparency), make a map to see where we are (perform evaluations), and ensure that we are steering in the right direction (elicit values in a democratic way). If we can do even some of this, we will be in a better place.
Percy Liang is an associate professor of computer science at Stanford, director of the Center for Research on Foundation Models, senior fellow at the Institute for Human-Centered AI, and co-founder of Together AI.
A MESSAGE FROM DEEPLEARNING.AI
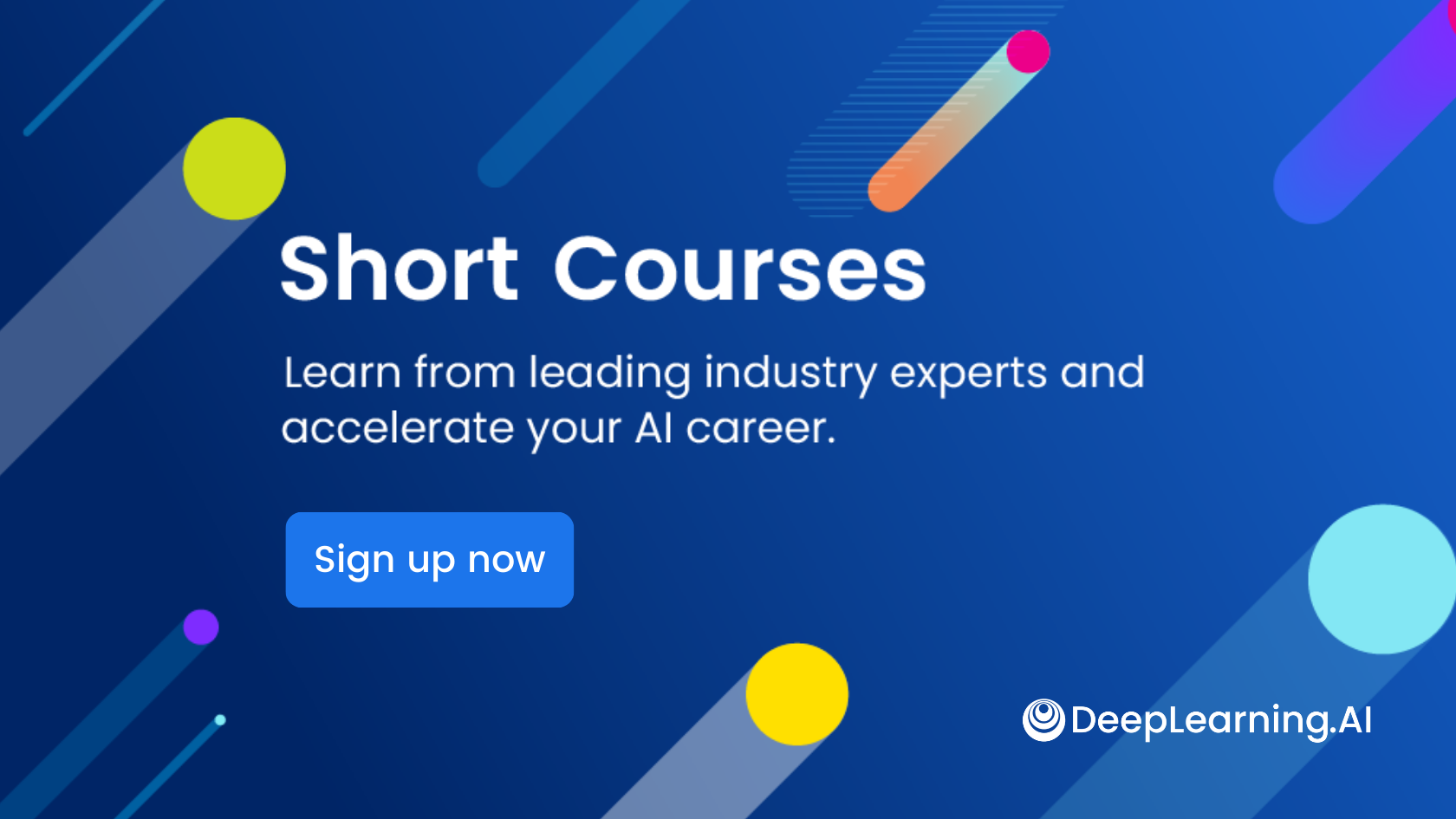
We wish you a skillful new year! Take your generative AI knowledge to the next level with short courses from DeepLearning.AI. Our catalog is available for free for a limited time. Check it out
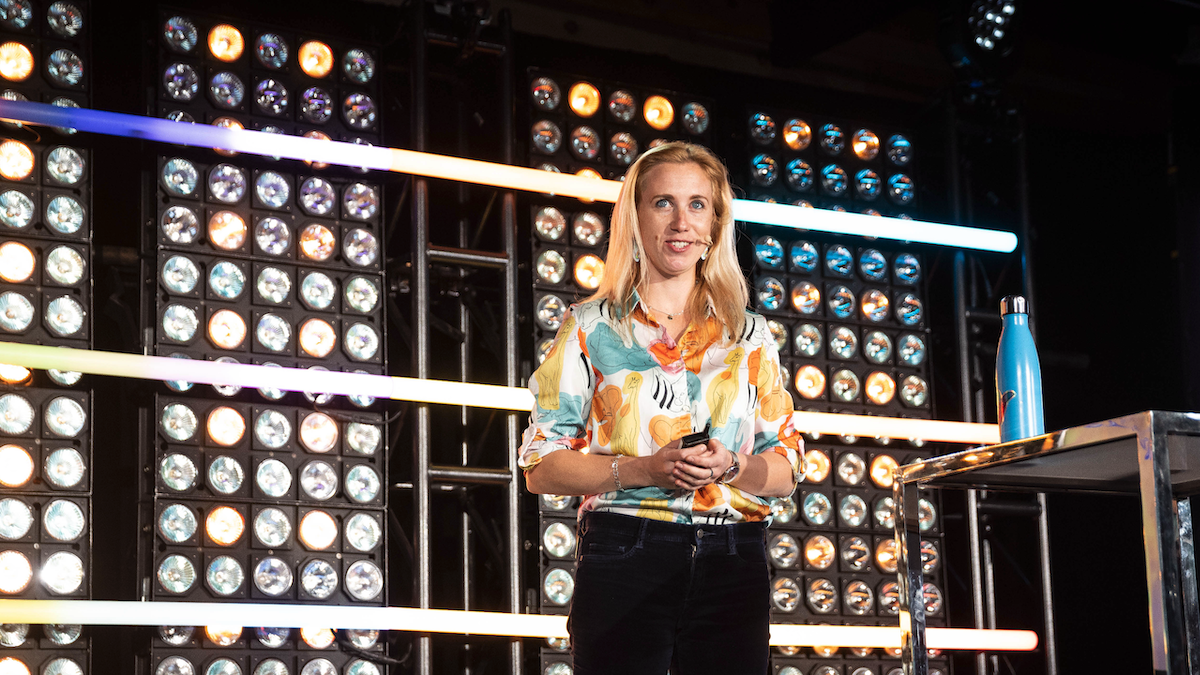
Sasha Luccioni: Respect for Human Creativity and Agency
Before this past year, when I told people I worked in AI, more often than not I was met with a blank stare and sometimes a question along the lines of: “You mean like robots?” In the last year, the seemingly magical abilities of AI models, especially large language models (LLMs), have broken into mainstream awareness, and now I’m greeted with questions like: “How does ChatGPT really work?” But if we were more transparent about the sheer amount of human time and labor that went into training LLMs, I’m sure the questions would be more along the lines of: “How do I keep my data from being used for training AI models?” Because as impressive as ChatGPT’s knock-knock jokes or chocolate chip cookie recipes are, they are definitely not magical — they are built upon the work and creativity of human beings, who should be attributed for their contributions.
AI models are black boxes that, to a user, appear to save labor. But, in fact, huge amounts of labor are required to develop them: from the books, websites, drawings, photos, and videos hoovered up without consent to the invisible armies of underpaid workers who spend their days ranking and improving LLM outputs. And all of this training is powered by massive amounts of natural resources that are extracted by still more human labor: rare metals to make those precious GPUs, water to cool them, energy to make them crunch numbers and output probabilities.
Until very recently, issues of copyright and consent were overlooked when it came to AI training data. Existing laws were assumed not to apply to training AI models, and the “move fast and break things” motto prevailed. But in the past year, authors like Sarah Silverman and George R.R. Martin have sued AI companies to assert their rights as content creators whose work was used without their permission to train AI models. While it’s too early to say how these lawsuits (and others) will pan out and how that will shape the future of copyright law in the United States and beyond, I hope that new mechanisms will be developed to allow content creators more control over their work. We are starting to see this from organizations like Spawning, which helped create ai.txt files that restrict the use of content for commercial AI training. I hope to see more AI developers respect these mechanisms and adopt opt-in (as opposed to opt-out) approaches for gathering consent-based datasets.
Apart from training data, development itself requires increasing amounts of labor. A new step recently has been added to the training process: RLHF, or reinforcement learning from human feedback. This step employs human annotators to rank text generated by large language models, providing feedback that makes them better at responding to human instructions and less likely to produce toxic output. This ranking process is done at scale by outsourced workers in offices in Kenya and prisons in Finland. Some of these workers are paid less than $2 an hour to label texts for hours on end, although we don’t have the overall numbers because AI companies are increasingly opaque about how they train AI models. Creating data for AI has become a new gig economy — but all this immense amount of human labor and creativity remains largely unseen and unrecognized.
And as AI is increasingly pushing out the very designers and artists whose life’s work was used to train the models in the first place (why pay a photographer when you can use AI to generate a custom stock photograph on demand), it’s crucial that we stop and reflect upon the relationship between human labor and creativity and AI. AI is truly an exciting new technology, and one that is set to provide huge profits to many tech companies, but artists and gig workers are barely getting crumbs of the pie, if anything at all. It’s not too late to reimagine AI as a technology that respects human agency and creativity by properly recognizing the human time and effort that goes into training AI models.
My hope in 2024 is that we start recognizing the knowledge, wisdom, and creativity that goes into training AI models, being more transparent about AI’s human costs, and developing increasingly human-centric technologies.
Sasha Luccioni is a research scientist and climate lead at HuggingFace, a founding member of Climate Change AI, and a board member of Women in Machine Learning.
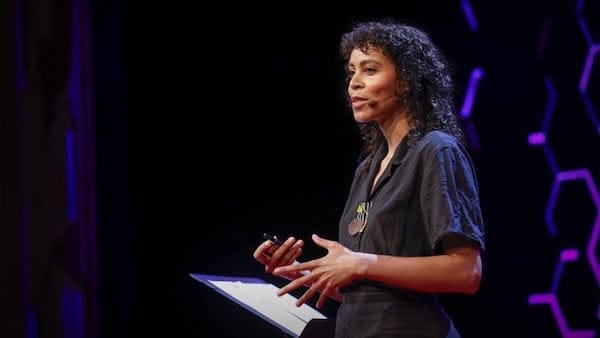
Pelonomi Moiloa: Smaller Models That Learn More From Less Data
One of my favourite flavours of conversation is listening to reinforcement learning experts talk about their children as reinforcement learning agents. These conversations highlight just how comically far behind humans our machine learning models are. Especially when comparing the ability to acquire knowledge without being told explicitly what to learn and when comparing the amount of information required for that learning.
My co-founder has a three-year-old son who is obsessed with cars. It would seem his objective function is to be exposed to as many cars as possible. So much so that he came home from a supercar show ranting and raving about the Daihatsu he saw in the parking lot, because he had never seen a Daihatsu before. On another occasion, when my co-founder told him the vehicle he was pointing at and enquiring about was a truck, the child did not hesitate to know that truck was a descriptor for a class of vehicle and not the name of the car.
What makes his little brain decide what is important to learn? How does it make connections? How does it make the inference so quickly across such a vast domain? Fueled solely by a bowl of Otees cereal?
What we have been able to achieve with our models as a species is quite impressive. But what I find far less impressive is how big the models are and the exorbitant resources of data, compute, capital, and energy required to build them. My co-founder's child learns far more from far less data, with a lot less energy.
This is not only a conundrum of resources for machine learning architects. It has profound implications for implementing AI in parts of the world where not only data but also electricity and computing equipment are severely limited. As AI practitioners, we need to understand how to build smaller, smarter models with less data.
Although efforts to put today's top-performing models on mobile devices are driving development of smaller models, prioritising small models that learn from relatively small datasets runs counter to mainstream AI development.
AI has the potential to help us understand some of the biggest questions of the universe, and it could provide solutions to some of the most pressing issues of our lifetime, like ensuring that everyone has access to clean energy, clean water, nutritious meals, and quality healthcare; resolving conflict; and overcoming the limitations of human greed. Yet the current mainstream of AI largely overlooks the lives affected by such problems. An approach that does not require the level of capital investment typical of AI would open the AI domain to more people, from more places, so they too can leverage the power of AI for the benefit of their communities.
I hope for many things for AI: that regulation and governance will improve, that the people who build the technology will do so with intention and with principles and values grounded in the connection of humanity. But the hope I am focusing on for now is more building of smaller, smarter models with less data to share the benefits of AI throughout the world. What are we working toward if not to make the world a sustainably better place for more people?
Pelonomi Moiloa is CEO of Lelapa AI, a socially grounded research and product lab that focuses on AI for Africans, by Africans.
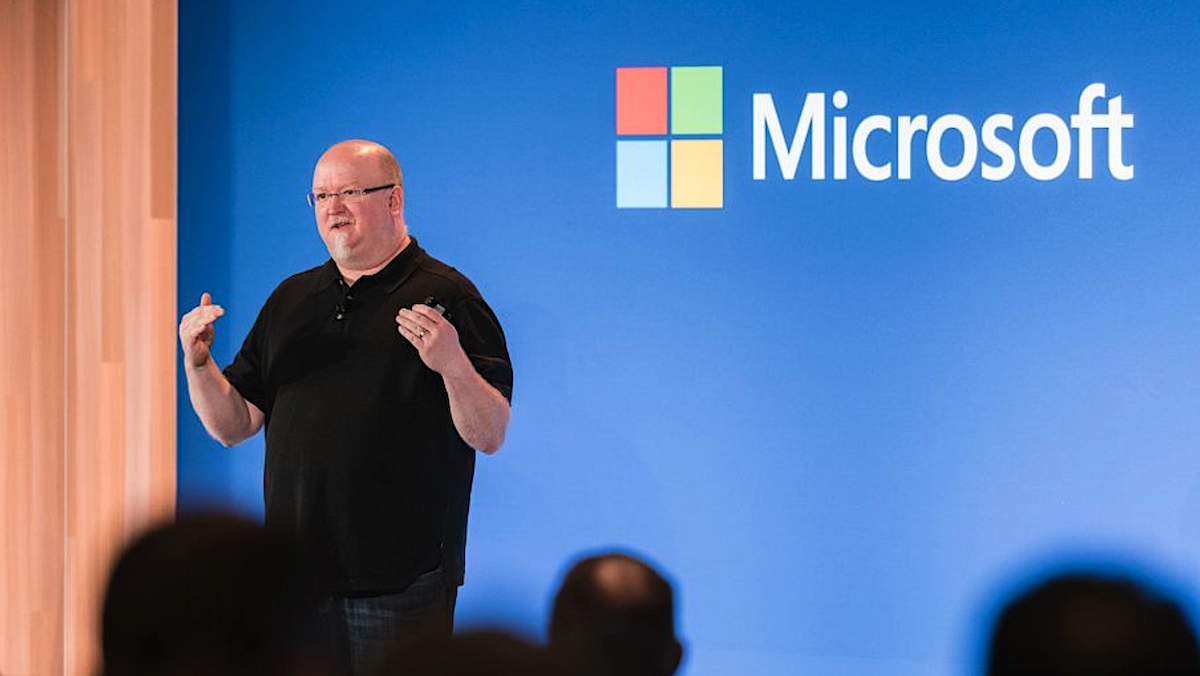
Kevin Scott: Be Prepared for Another Year of Exponential Growth
Without question, 2023 has been the most exciting and interesting year in technology that I’ve seen over a fairly long career. It bears mention that I’m pretty sure I said more or less the same thing at the close of 2022, and I suspect I’ll probably be saying the same around this time next year and each year for the foreseeable future—the point being that, in AI right now, we’re experiencing a period of sustained exponential growth that represents perhaps the most profound technological progress that we have ever seen.
And it’s really only the beginning. Modern generative AI is still in its infancy, and we’re learning as we go. While it feels like we’ve lived with them for ages now, 2023 was really the first year that powerful AI tools like ChatGPT and Microsoft Copilots meaningfully entered the public vernacular as useful helpers to make people’s lives easier. By the time next year wraps up, we’ll have many new experiences, apps, and tools that will create cascading benefits for more and more people across the planet. Though the amplitude of hype and acceleration rate of AI’s growth can keep folks fixated on each subsequent “next big thing,” if we step back just a little bit, it’s easier to see that the opportunity in front of us is astronomically greater than what we’ve already achieved.
Because we only get to sample the product of that exponential curve every couple of years or so, most recently with GPT-4, it’s easy to forget in the interim how astonishing the pace of growth actually is. And, as is our human nature, we acclimatize very quickly and soon take for granted each immediate set of wild new possibilities offered to us.
So, my hope for all of us working in AI and technology over the next year is that we collectively remember that the next sample from the exponential is coming, and prepare ourselves appropriately for the (sure to be incredible) outcomes. If you haven’t done so already, pay close attention, experiment, and build AI production practices. If not, you’ll be too far behind to translate the progress into meaningful benefits for everyone.
May 2024 continue to bring the excitement of discovery and continued innovation for us all.
Kevin Scott is chief technology officer and executive vice president of AI at Microsoft.