Dear friends,
Last week, I attended the NeurIPS conference in New Orleans. It was fun to catch up with old friends, make new ones, and also get a wide scan of current AI research. Work by the big tech companies tends to get all the media coverage, and NeurIPS was a convenient place to survey the large volume of equally high-quality work by universities and small companies that just don’t have a comparable marketing budget!
AI research has become so broad that I struggle to summarize everything I saw in a few sentences. There were numerous papers on generative AI, including large language models, large multimodal models, diffusion models, enabling LLMs to use tools (function calls), and building 3D avatars. There was also plenty of work on data-centric AI, differential privacy, kernels, federated learning, reinforcement learning, and many other areas.
One topic I’m following closely is autonomous agents: Software, usually based on LLMs, that can take a high-level direction (say, to carry out competitive research for a company), autonomously decide on a complex sequence of actions, and execute it to deliver the outcome. Such agents have been very hard to control and debug, and so, despite amazing-looking demos, there have been few practical deployments. But now I see them on the cusp of working well enough to make it into many more applications, and increasingly I play with them in my spare time. I look forward to getting through my reading list of autonomous agent research papers over the coming holiday!
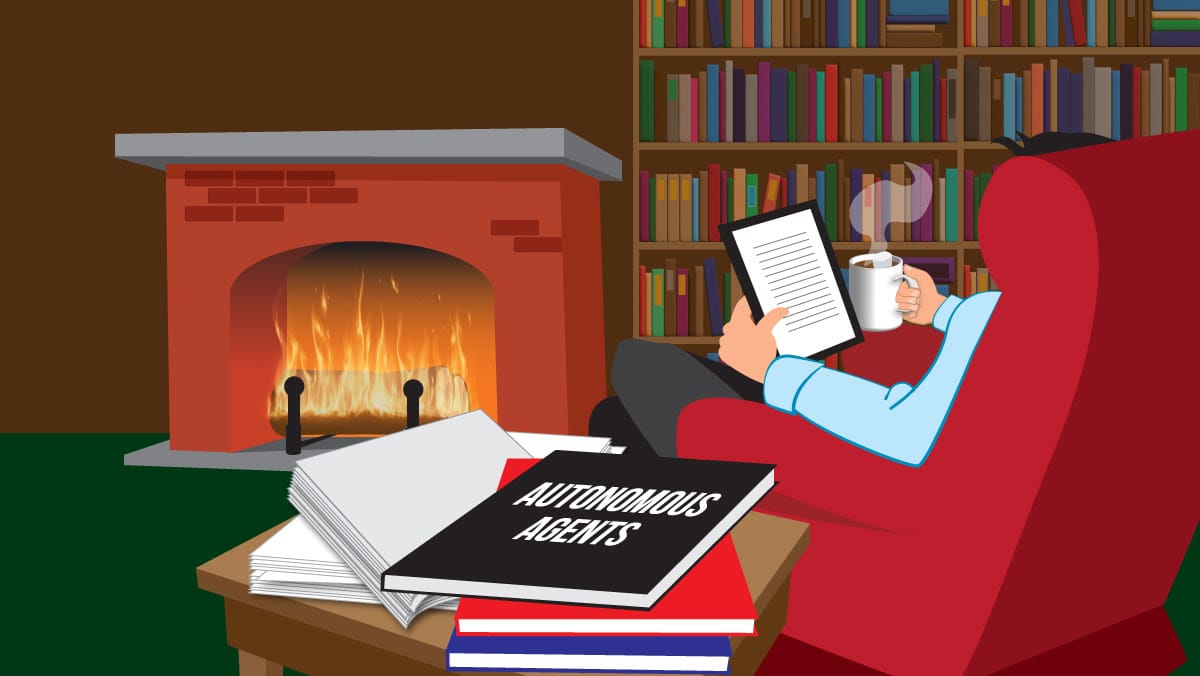
At NeurIPS, many people I spoke with expressed anxiety about the pace of AI development and how to keep up as well as publish, if what you're working on could be scooped (that is, independently published ahead of you) at any moment. While racing to publish first has a long history in science, there are other ways to do great work. The media, and social media especially, tend to focus on what happened today. This makes everything seem artificially urgent. Many conversations I had at NeurIPS were about where AI might go in months or even years.
I like to work quickly, but I find problem solving most satisfying when I’ve developed an idea that I believe in — especially if it’s something that few others see or believe in — and then spend a long time executing it to prove out the vision (hopefully). I find technical work more fulfilling when I have time to think deeply, form my own conclusion, and perhaps even hold an unpopular opinion for a long time as I work to prove it. There’s a lot of value in doing fast, short-term work; and given the large size of our community, it’s important to have many of us doing long-term projects, too.
So, this holiday season, when the pace of big announcements might slow down for a couple of weeks, I hope you’ll take a break. Spend time with friends and loved ones, let thoughts simmer in the back of your mind, and remind yourself of holiday values like charity and renewal. If you’re looking for ideas, maybe even some that will keep you productively busy for months or years, injecting more inputs — taking courses, reading blogs or papers — is a good way to do that.
It has been a great year for AI, with lots of progress and excitement. I’m grateful to have gotten through this crazy year with you.
Happy holidays!
Andrew
Top AI Stories of 2023
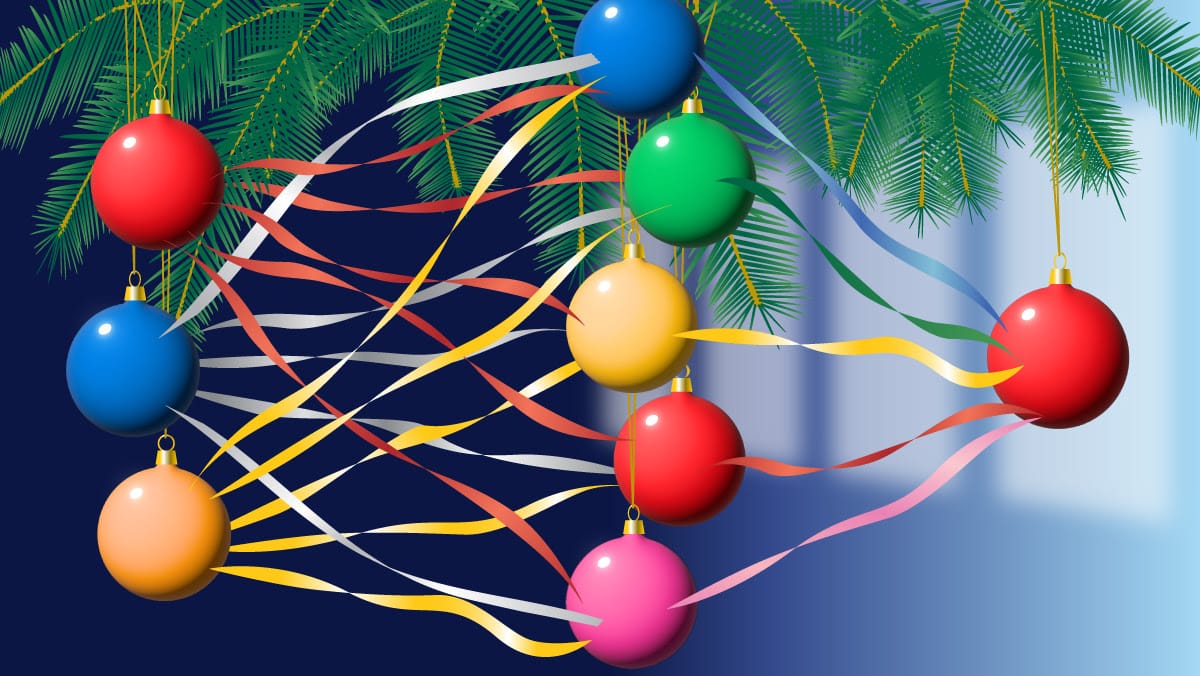
A Year of Innovation and Consternation
Recent years brought systems that, given a text prompt, generate high-quality text, pictures, video, and audio. In 2023, the wave of generative AI washed over everything. And its expanding capabilities raised fears that intelligent machines might render humanity obsolete. As in past years at this season, we invite you to settle by the fire and savor 12 months of technological progress, business competition, and societal impact.
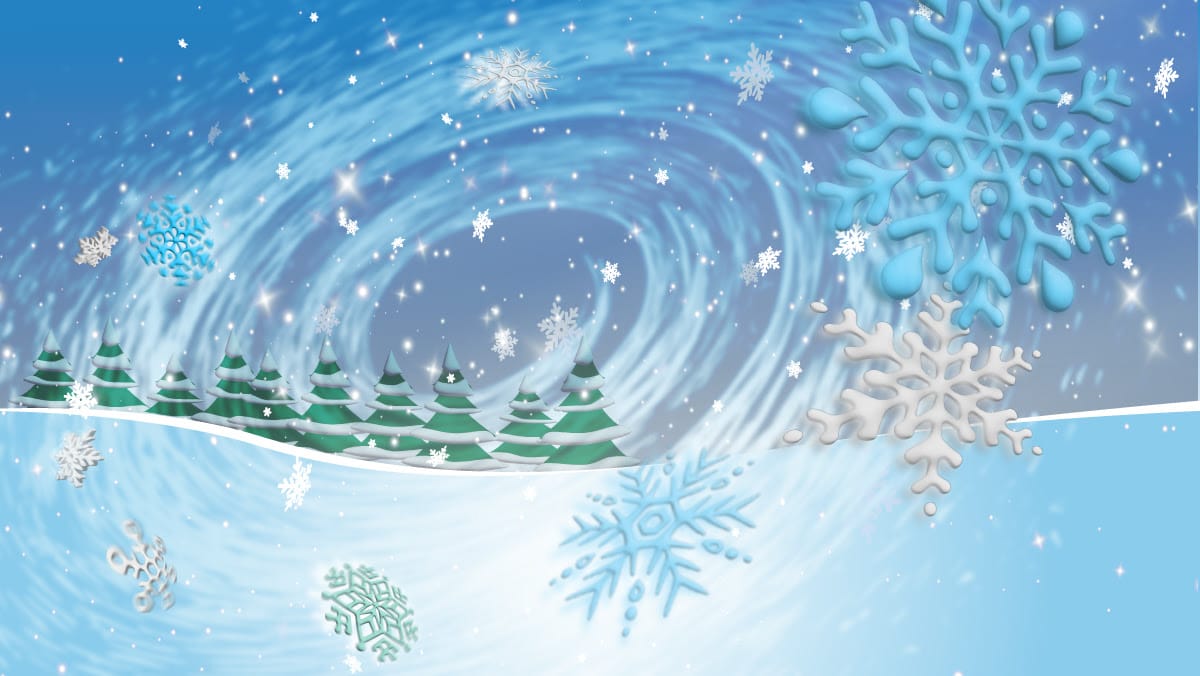
Generative AI Everywhere
This year, AI became virtually synonymous with generative AI.
What happened: Launched in November 2022, OpenAI’s ChatGPT ushered in a banner year for AI-driven generation of text, images, and an ever widening range of data types.
Driving the story: Tech giants scrambled to launch their own chatbots and rushed cutting-edge natural language processing research to market at a furious pace. Text-to-image generators (also sparked by OpenAI with DALL·E in early 2021) continued to improve and ultimately began to merge with their text-generator counterparts. As users flocked to try out emerging capabilities, researchers rapidly improved the models’ performance, speed, and flexibility.
- Microsoft integrated OpenAI’s language models into its Bing search engine. Google, sensing a threat to its search business, leveraged its own formidable models into the Bard chatbot. These rapid-fire launches weren’t all smooth sailing — the AI-enhanced Bing exhibited bizarre behavior, while Bard’s debut was beset by hallucinations — but they set a new bar for search functionality and broad access to text generation.
- Pressing its lead, Microsoft added generative Copilot systems to its flagship applications: a code generator and chatbot for GitHub; a chat interface for Windows; and tools to summarize Word documents, craft Excel formulas, and draft emails in Outlook.
- Numerous teams built open source competitors, seeding an ecosystem of options that developers can download and run freely. Meta initially offered LLaMA for free to researchers, but it jumped the fence to make high-performance text generation available far and wide. Hot on its heels came Falcon, Mistral, and many others. Many open source models deliver performance comparable to that of GPT-3.5, although GPT-4 remains the leader.
- In the cloud, Microsoft Azure, Google Cloud, and Amazon AWS battled to deliver generative AI in the cloud. Amazon offered its own TItan models and a sampling of models from third parties, including Stability AI, Anthropic, and AI21. By the end of the year, many alternatives were available from a variety of cloud providers.
- Less than a year after ChatGPT, GPT-4 integrated DALL-E 3, giving it the ability to interpret images and prompt the image generator to produce them. In December, Google introduced Gemini: a family of language-and-vision models that process mixed inputs of text, images, audio, and video.
Gold rush: Generative AI didn’t just thrill customers and businesses; it generated a flood of funding for AI developers. Microsoft invested $13 billion in OpenAI, and Amazon and Google partnered with the nascent startup Anthropic in respective multibillion-dollar investments. Other generative AI startups raised hundreds of millions of dollars.
Where things stand: In the span of a year, we went from one chat model from OpenAI to numerous closed, open, and cloud-hosted options. Image generators have made strides in their ability to interpret prompts and produce realistic output. Video and audio generation are becoming widely available for short clips, and text-to-3D is evolving. 2024 is primed for a generative bonanza, putting developers in a position to build a wider variety of applications than ever before.
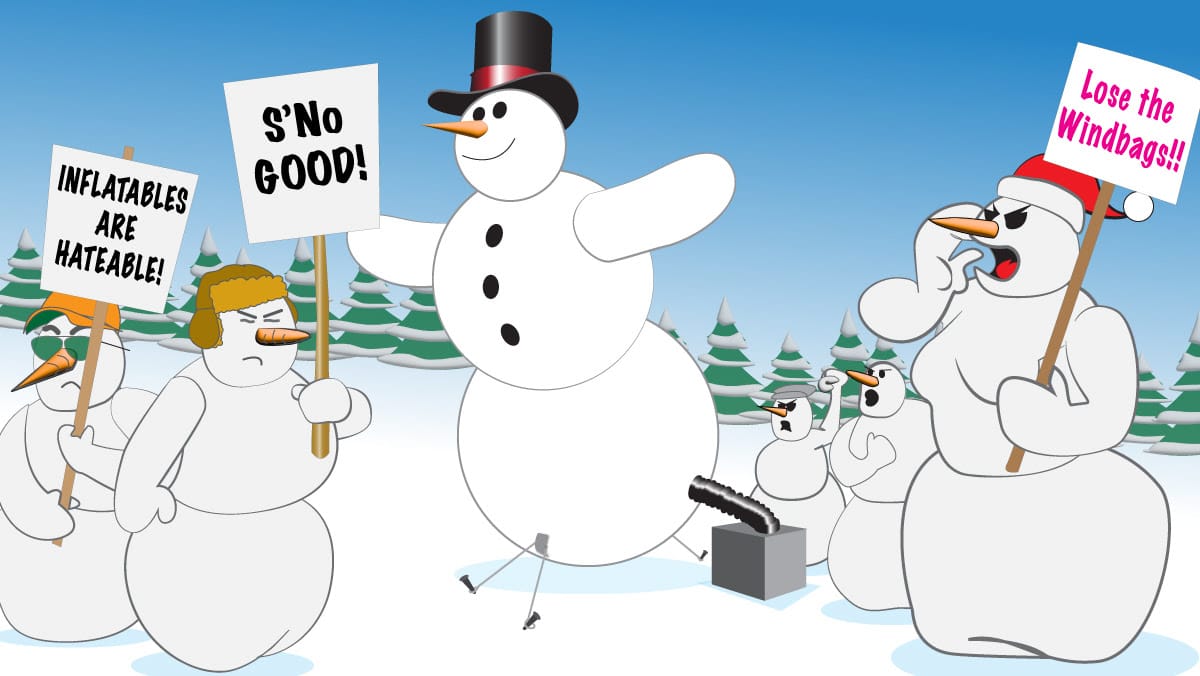
Hollywood Squares Off
The movie capital became a front line in the battle over workplace automation.
What happened: U.S. film and television writers went on strike in May, and actors followed in July. They took up a variety of issues with their employers, but concern that AI would damage their job prospects prolonged the work stoppage. Both groups inked agreements shortly before the year ended.
Driving the story: Screenwriters negotiated for 148 days, and actors for 118, winning limits on their employers’ abilities to replace them with machine learning models.
- The Writers’ Guild of America finalized an agreement with an alliance of film studios in September. It allows the studios to train models on a writer’s work. But an AI model can’t receive writing credit, and a studio can’t use AI in ways that reduce a writer’s compensation or credit. Writers can use AI with a studio’s permission at their discretion, but studios can’t require it.
- The Screen Actors Guild reached a similar deal with studios two months later. Studios can train models on an actor’s performance, but they must seek permission from the actor and compensate them first. Studios must gain permission from a deceased actor’s heirs before using AI to re-create the actor’s likeness.
- Both agreements provide for union representatives to meet regularly with studios to discuss technological developments.
AI on the silver screen: Traditional Hollywood studios negotiated alongside the film departments of Amazon, Apple, and Netflix, tech powerhouses that have access to considerable AI expertise. All are likely to use AI to generate text, images, audio, and video.
- In February, Netflix released a short anime film that includes AI-assisted background art. Netflix cited a labor shortage as motivation for the decision, which garnered criticism from audiences and the animation community.
- In July, Netflix posted a help-wanted ad for an AI product management role. The annual salary offered, between $300,000 and $900,000, suggests that the technology will play an important role in the company’s forthcoming productions.
- Later in the summer, Disney formed a task force to study AI’s potential to cut production costs.
Where things stand: The unions and studios agreed to use AI while enabling writers and actors to continue to ply their trades. The agreements will remain in force for three years — time enough for both sides to learn a bit about what the technology is and isn’t good for, and to form a vision of its role in the future. Now Hollywood faces the next challenge: Using AI to make better movies that grow the pie for producers and creatives alike.
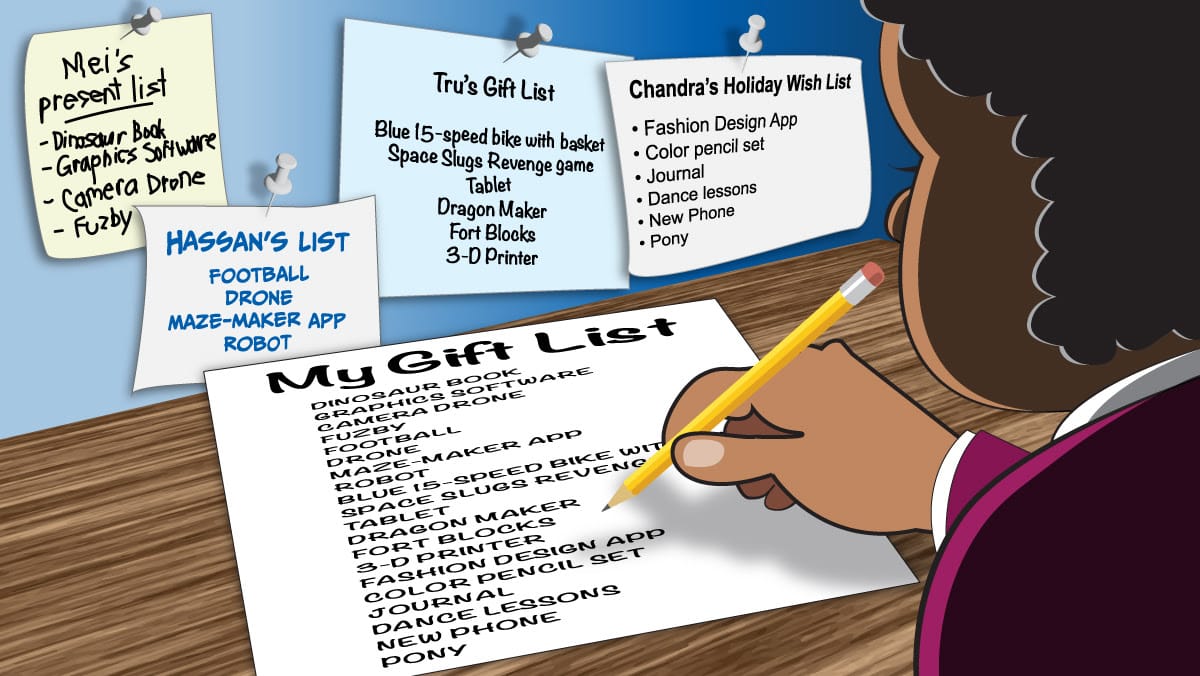
Can I Use This Data?
Information may not want to be free after all.
What happened: The age-old practice of training AI systems on data scraped from the web came into question as copyright owners sought to restrict AI developers from using their works without permission.
Driving the story: Individual copyright holders filed lawsuits against AI companies for training models on their data without obtaining explicit consent, giving credit, or providing compensation. Concurrently, formerly reliable repositories of data on the open web started to require payment or disappeared entirely.
- A group of visual artists filed a class-action lawsuit claiming that Midjourney, Stability AI, and online artists’ community DeviantArt infringed their copyright by enabling users to create images in the styles of artists. Getty, a provider of stock images, also sued Stability AI for allegedly using Getty pictures without permission.
- High-profile writers and The Authors’ Guild filed a similar lawsuit against OpenAI, claiming that the company infringed their copyrights by training models on their work. Universal Music Group sued Anthropic for training language models on copyrighted song lyrics.
- The websites Reddit and Stack Overflow, which have been popular resources for training language models, began charging developers to use their data. The New York Times changed its terms of service to explicitly forbid training AI models from its data.
- The Books3 corpus, which contains nearly 200,000 digitized books copied without permission, was part of The Pile, an 800GB corpus that has been used to train popular large language models. In August, the Rights Alliance, an anti-piracy group, forced a web host to remove the corpus.
- With open data sources at risk of copyright enforcement, OpenAI entered into agreements with Shutterstock and Axel Springer to use their images and news, respectively. Adobe, Anthropic, Google, IBM, Microsoft, OpenAI, and Shutterstock pledged to take responsibility for some copyright actions that arise from using their generative models.
Copyright conundrum: Whether copyright restricts training machine learning models is largely an open question. Laws in most countries don’t address the question directly, leaving it to the courts to interpret which uses of copyrighted works do and don’t require a license. (In the U.S., the Copyright Office deemed generated images ineligible for copyright protection, so training corpuses made up of generated images are fair game.) Japan is a notable exception: The country’s copyright law apparently allows training machine learning models on copyrighted works.
Where things stand: Most copyright laws were written long ago. The U.S. Copyright Act was established in 1790 and was last revised in 1976! Copyright will remain a battlefield until legislators update laws for the era of generative AI.
A MESSAGE FROM KIRA LEARNING
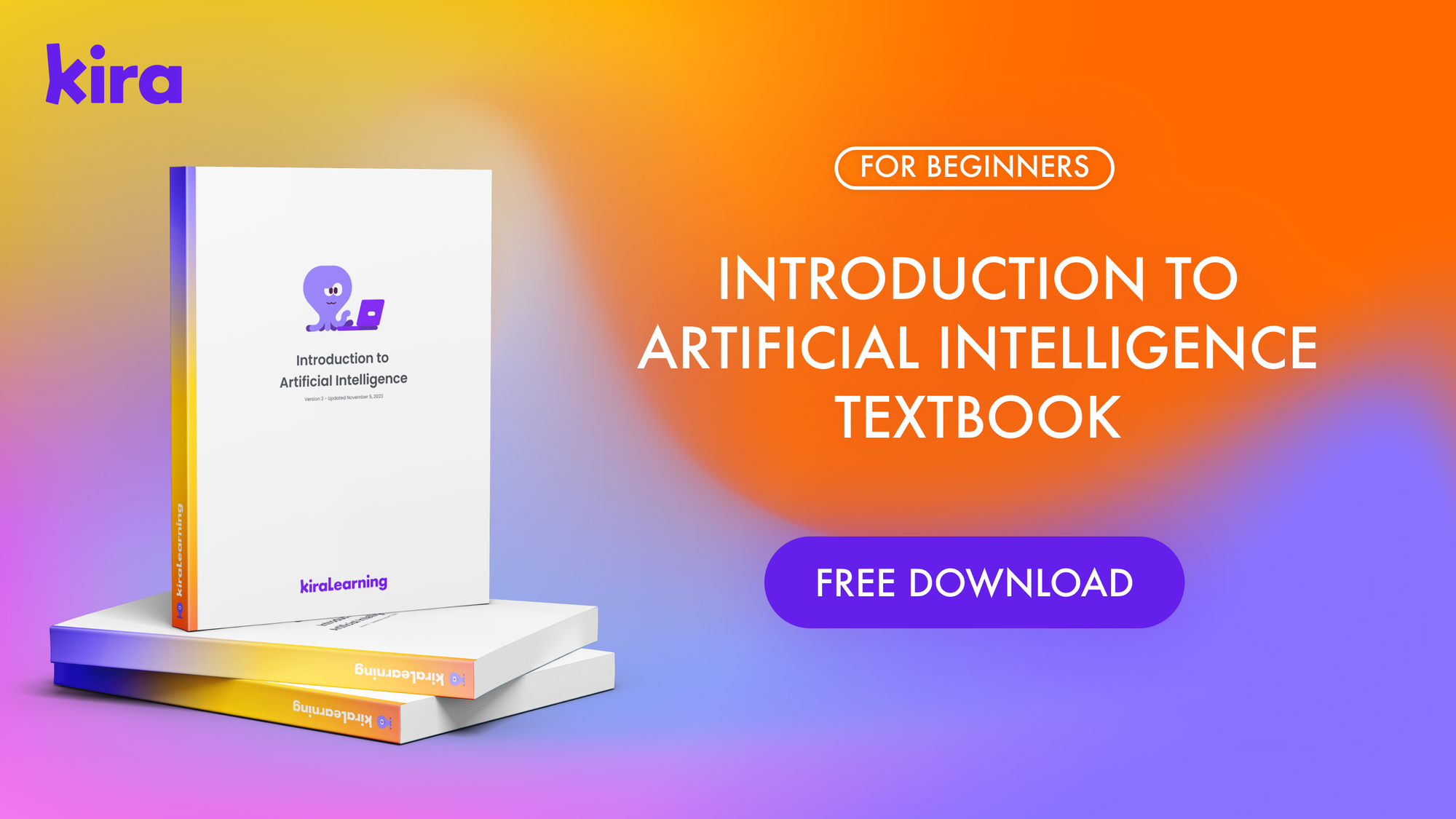
Looking for a gentle lead-in to AI? Introduction to Artificial Intelligence is designed for middle- and high-school learners who have no prior AI experience. Edited by Jagriti Agrawal, co-founder of Kira Learning (a sister company of DeepLearning.AI), this textbook teaches what AI is, how it works, and why it matters. Download for free
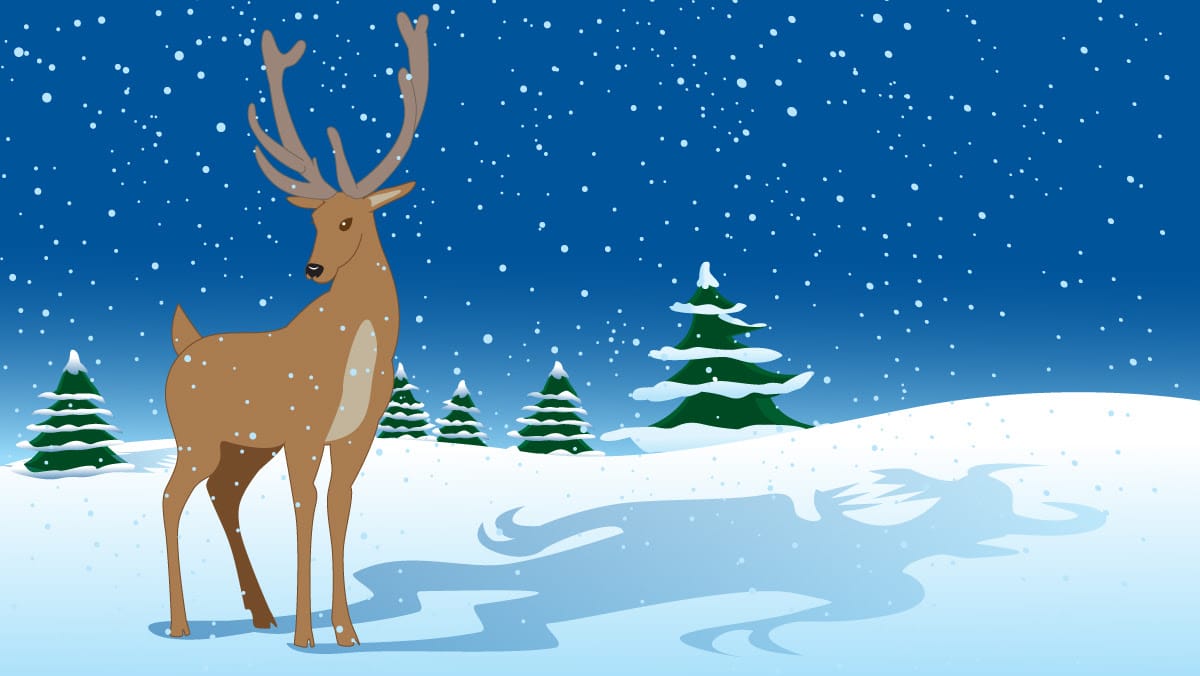
High Anx-AI-ety
Angst at the prospect of intelligent machines boiled over in moves to block or limit the technology.
What happened: Fear of AI-related doomsday scenarios prompted proposals to delay research and soul searching by prominent researchers. Amid the doomsaying, lawmakers took dramatic regulatory steps.
Driving the story: AI-driven doomsday scenarios have circulated at least since the 1950s, when computer scientist and mathematician Norbert Weiner claimed that “modern thinking machines may lead us to destruction.” Such worries, amplified by prominent members of the AI community, erupted in 2023.
- The not-for-profit Future of Life Institute published an open letter that called for a six-month pause in training powerful AI models. It garnered nearly 34,000 signatures.
- Deep learning pioneers Geoffrey Hinton and Yoshua Bengio expressed their worries that AI development could lead to human extinction, perhaps at the hands of a superhuman intelligence.
- Google, Microsoft, and OpenAI urged the U.S. Congress to take action.
- The UK government convened the international Bletchley Summit, where 10 countries including France, Germany, Japan, the U.S., and the UK agreed to form a panel that will report periodically on the state of AI.
Regulatory reactions: Lawmakers from different nations took divergent approaches with varying degrees of emphasis on preventing hypothetical catastrophic risks.
- China aimed to protect citizens from intrusions on their privacy without limiting government power. It added requirements to label AI-generated media and prohibit face recognition, with broad exceptions for safety and national security.
- The United States moved to promote individual privacy and civil rights as well as national safety under existing federal laws. Although the U.S. didn’t pass national regulations, the White House collaborated with large AI companies to craft both voluntary limits and an executive order that requires extensive disclosure and testing of models that exceed a particular computational threshold.
- The European Union’s AI Act aims to mitigate the highest perceived risks. The bill limits certain AI applications including biometric identification or determinations of eligibility for employment public services. It also mandates that developers of general-purpose models disclose information to regulators. The law imposes a lighter burden on smaller companies and provides some exceptions for open source models. Like China, it exempts member states’s military and police forces.
Striking a balance: AI has innumerable beneficial applications that we are only just beginning to explore. Excessive worry over hypothetical catastrophic risks threatens to block AI applications that could bring great benefit to large numbers of people. Some moves to limit AI would impinge on open source development, a major engine of innovation, while having the anti-competitive effect of enabling established companies to continue to develop the technology in their own narrow interest. It’s critical to weigh the harm that regulators might do by limiting this technology in the short term against highly unlikely catastrophic scenarios.
Where things stand: AI development is moving too quickly for regulators to keep up. It will require great foresight — and a willingness to do the hard work of identifying real, application-level risks rather than imposing blanket regulations on basic technology — to limit AI’s potential harms without hampering the good that it can do. The EU’s AI Act is a case in point: The bill, initially drafted in 2021, has needed numerous revisions to address developments since then. Should it gain final approval, it will not take effect within two years. By then, AI likely will raise further issues that lawmakers can’t see clearly today.
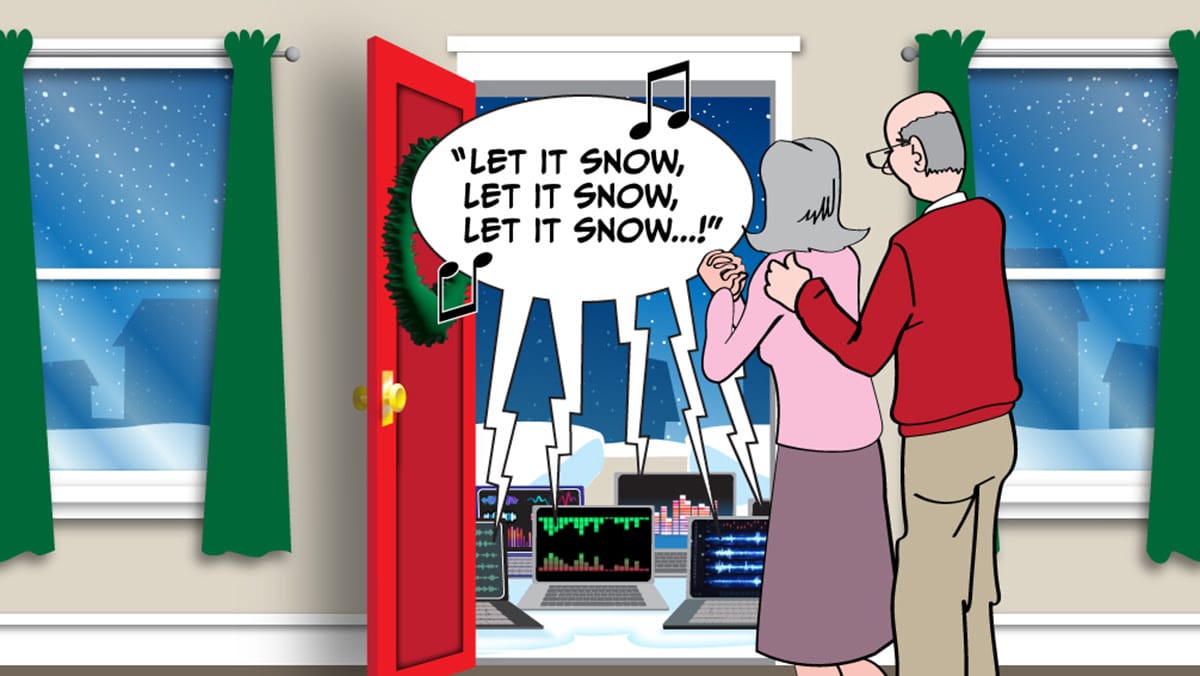
Deep Learning Rocks
Fans of AI-driven music pressed play, while a major recording company reached for the stop button.
What happened: AI grabbed listeners by the ears when it helped produce a new single by The Beatles, mimicked the voices of beloved stars, and generated music from text prompts.
Driving the story: AI hasn’t quite had its first hit record, but developments in generated music put both fans and the record industry on notice that it may not be far away.
- Giles Martin, son of the producer of The Beatles’ classic 1960s records, used a proprietary audio demixing algorithm to pick apart a crude recording of an unreleased song by deceased band member John Lennon. Martin isolated Lennon’s voice so the surviving members could add fresh instruments and vocals. The result put the Beatles at the top of the UK music chart for the first time in more than 50 years.
- Talented fans used voice cloning models to produce soundalike recordings in the styles of well-known artists such as Drake and Oasis.
- Experimental pop star Grimes enabled the public to transform their own singing into a likeness of her voice, resulting in more than 300 faux-Grimes productions. Korean pop artist Midnatt used a similar system to translate a vocal track into five other languages.
- In September, Stability AI released Stable Music, a diffusion model that generates up to 90 seconds of music or sound effects from text prompts, for paid public use. Stable Music followed Google’s MusicLM, a text-to-music model based on the transformer architecture.
Industry crackdown: Universal Music Group (UMG), which accounts for nearly one-third of the global music market, reacted swiftly to the wave of generated music. It blocked streaming services from distributing fan-made, voice-cloned productions and demanded that they block AI developers from downloading music by UMG artists so they can’t use it to train machine learning models. Shortly afterward, UMG partnered with Endel, a startup that generates background music. UMG artist James Blake released music he created using Endel’s system.
Where things stand: Generative AI is poised to play an increasing role in recorded music. AI-powered tools exist for many phases of recording production, including composition, arrangement, and mixing. The recent agreements between actors and writers and Hollywood studios may offer pointers to musicians and recording executives who would like to use these tools to make exciting, marketable music.
Data Points
Many releases, from language models by Microsoft and Mistral to a learning-based locomotion controller by UC-Berkeley researchers, are keeping the AI landscape exceptionally dynamic as the year draws to a close!
Explore the week's top AI news in Data Points, a spin-off of our newsletter, The Batch.