Dear friends,
I recently spoke about “Opportunities in AI” at Stanford’s Graduate School of Business. I'd like to share a few observations from that presentation, and I invite you to watch the video (37 minutes).
AI is a collection of tools, including supervised learning, unsupervised learning, reinforcement learning, and now generative AI. All of these are general-purpose technologies, meaning that — similar to other general-purpose technologies like electricity and the internet — they are useful for many different tasks. It took many years after deep learning started to work really well circa 2010 to identify and build for a wide range of use cases such as online advertising, medical diagnosis, driver assistance, and shipping optimization. We’re still a long way from fully exploiting supervised learning.
Now that we have added generative AI to our toolbox, it will take years more to explore all its uses. (If you want to learn how to build applications using generative AI, please check out our short courses!)
Where do the opportunities lie? With each new wave of technology, entrepreneurs and investors focus a lot of attention on providers of infrastructure and tools for developers. The generative AI wave has brought tools from AWS, Google Cloud, Hugging Face, Langchain, Microsoft, OpenAI, and many more. Some will be huge winners in this area. However, the sheer amount of attention makes this part of the AI stack hypercompetitive. My teams (specifically AI Fund) build startups in infrastructure and tools only when we think we have a significant technology advantage, because that gives us a shot at building large, sustainable businesses.
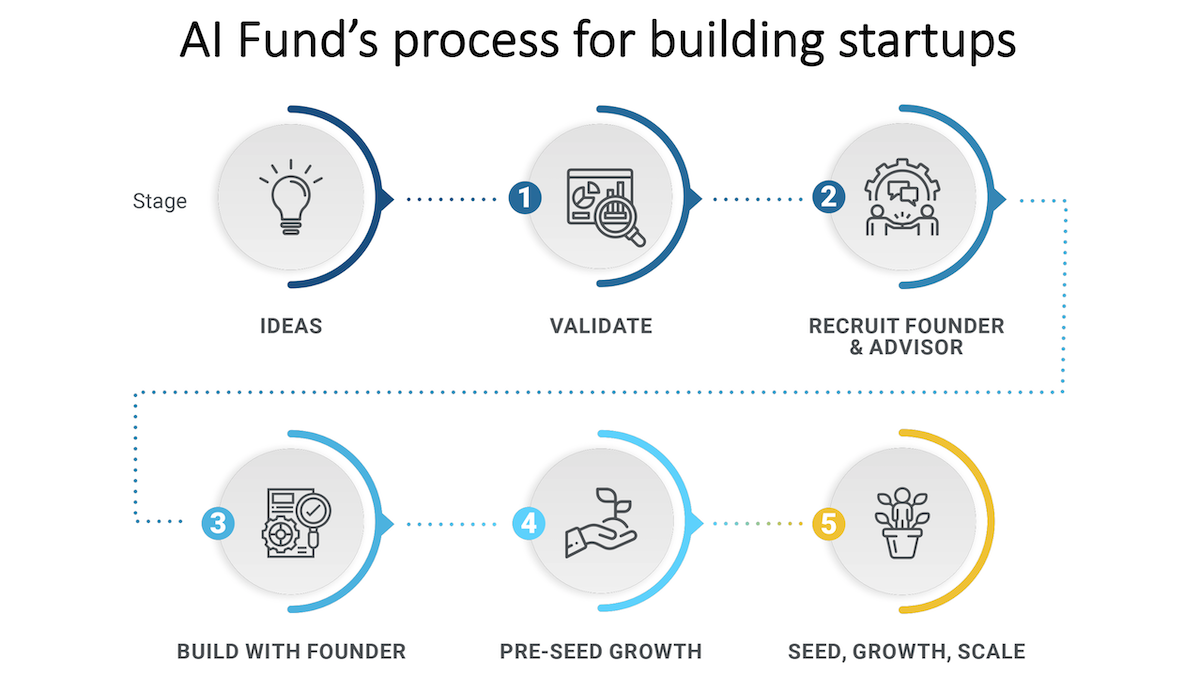
But I believe a bigger opportunity lies in the application layer. Indeed, for the companies that provide infrastructure and developer tools to do well, the application companies that use these products must perform even better. After all, the application companies need to generate enough revenue to pay the tool builders.
For example, AI Fund portfolio companies are applying AI to applications as diverse as global maritime shipping and relationship mentoring. These are just two areas where the general-purpose technology of AI can create enormous value. Because few teams have expertise in both AI and sectors like shipping or relationships, the competition is much less intense.
If you’re interested in building valuable AI projects, I think you’ll find the ideas in the presentation useful. I hope you’ll watch the video and share it with your friends. It describes in detail AI Fund’s recipe for building startups and offers non-intuitive tips on the ideas that we’ve found to work best.
Keep building!
Andrew
News
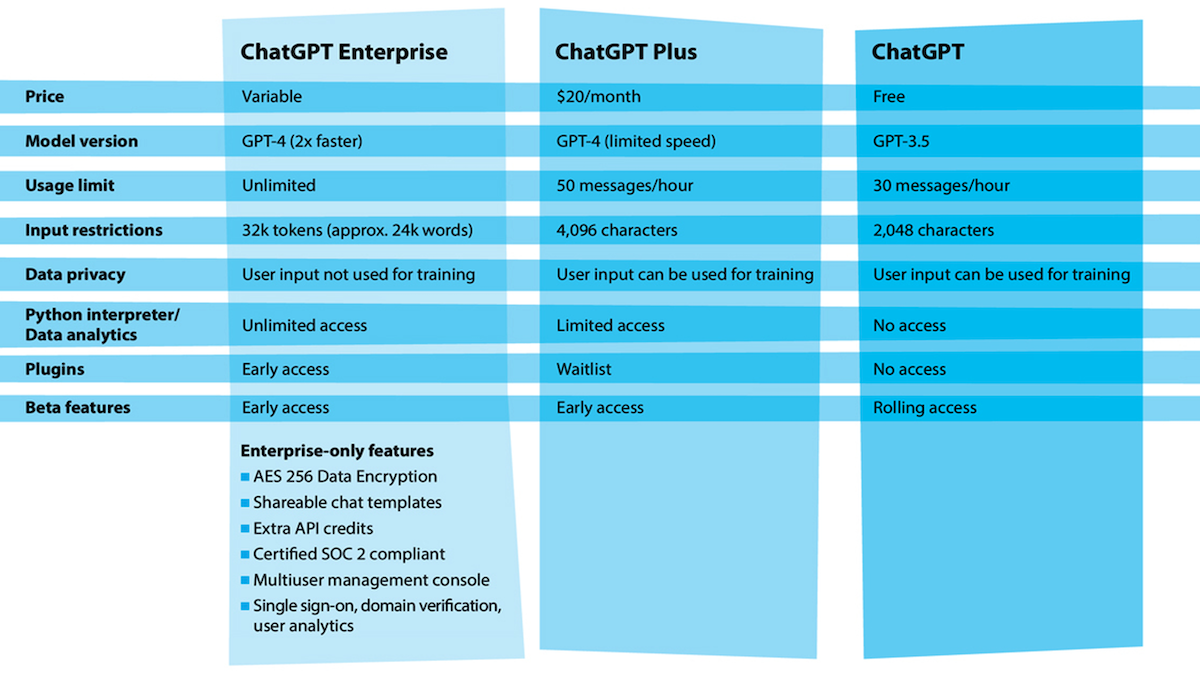
ChatGPT for Big Biz
A new version of ChatGPT upgrades the service for corporate customers.
What’s new: OpenAI launched ChatGPT Enterprise, which combines enhanced data-privacy features with a more capable language model. The price is negotiable on a case-by-case basis, Bloomberg reported.
How it works: ChatGPT Enterprise provides enhanced access to GPT-4, previously available via ChatGPT Plus ($20 per month) and API calls at a cost per thousand tokens.
- Customer inputs are encrypted. OpenAI will not use them as training data.
- Access to the model is unlimited with a maximum input length, or context window, of 32,000 tokens. That's equal to the context window for paid API access and four times the length allowed by ChatGPT and ChatGPT Plus.
- A plugin enables users to execute unlimited amounts of Python code within the chatbot.
- The program includes an unspecified number of free credits to use OpenAI’s APIs.
- Individuals can share templates that make it possible to build common ChatGPT workflows.
- A console enables administrators to control individual access.
Behind the news: OpenAI has metamorphosed from a nonprofit into a tech-biz phenomenon, but its business is still taking shape. For 2022, the company reported $540 million in losses on $28 million in revenue. It’s reportedly on track to bring in $1 billion this year, and ChatGPT Enterprise is bound to benefit from OpenAI’s high profile among business users: The email addresses of registered ChatGPT users represent 80 percent of the Fortune 500, according to the company.
Why it matters: Large language models are transforming from public experiments to mainstream productivity tools. ChatGPT Enterprise is a significant step in that transition, giving large companies the confidence they need to integrate GPT-4 into their day-to-day operations with less worry that OpenAI will ingest proprietary information.
We’re thinking: Some reporters have questioned the financial value of generative AI. While OpenAI’s business is evolving, this new line of business is promising. We anticipate that enterprise subscriptions will be stickier than API access, since customers’ switching costs are likely to be higher.
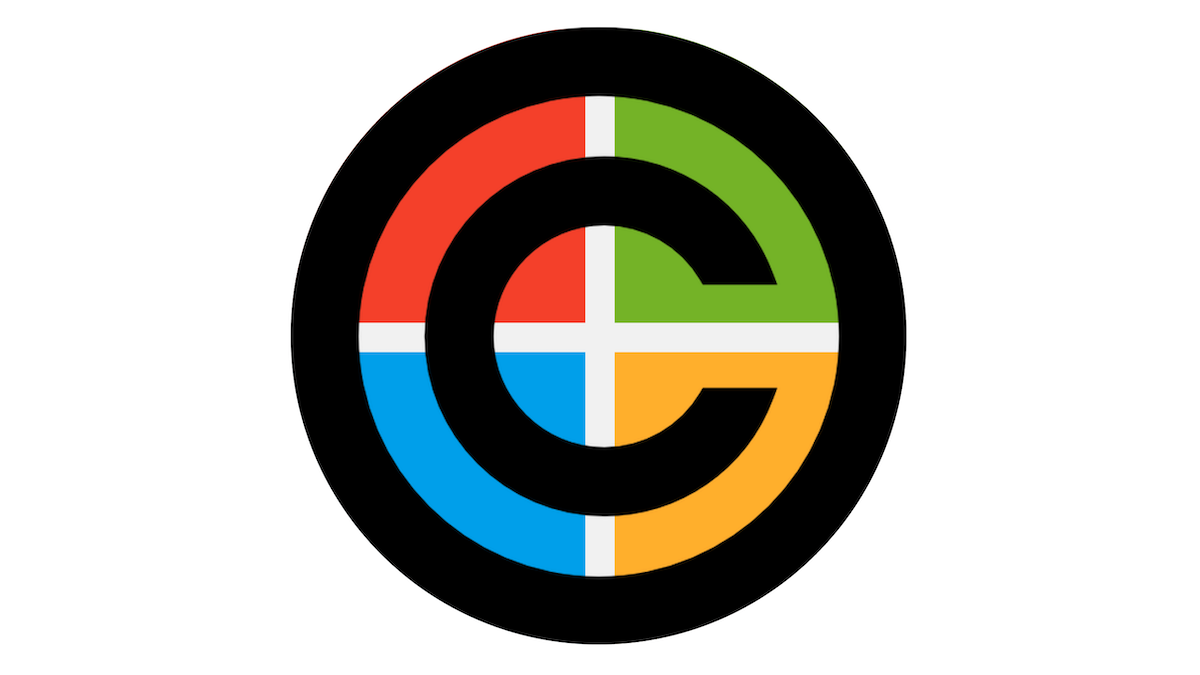
GenAI Violated Copyright? No Problem
Microsoft promised to shield users of its generative AI services against the potential risk of copyright infringement.
What’s new: Microsoft said it would cover the cost for any copyright violations that may arise from use of its Copilot features, which generate text, images, code, and other media within its productivity apps.
How it works: In its Copilot Copyright Commitment, Microsoft vows to defend customers in court against allegations that they infringed copyrights by using Microsoft software. It also promises to reimburse the cost of adverse judgments or settlements.
- The commitment covers media created using Microsoft 365 Copilot, which generates text, images, and layouts for Word, Excel, PowerPoint, Outlook, and Teams. It also covers the output of Bing Chat Enterprise (but not the AI-enhanced search engine’s free version), and the GitHub Copilot code generator.
- Customers are covered unless they try to breach guardrails such as filters designed to detect and block infringing output.
- Microsoft’s commitment follows a similar promise issued in June by Adobe to indemnify users of its Firefly generative service against intellectual property claims.
Behind the news: Microsoft, its subsidiary GitHub, and its partner OpenAI are currently defending themselves against allegations that GitHub Copilot violated copyright laws. Programmer and attorney Matthew Butterick claims that OpenAI trained GitHub Copilot in violation of open-source licenses and that the system reproduces copyrighted code without authorization. In May, a judge rejected a request by the defendants to dismiss the case, which remains ongoing.
Why it matters: Generative AI represents a huge business opportunity for Microsoft and others. Yet the technology is under attack by copyright holders, creating the potential that customers may face lawsuits simply for using it. That may be persuading enterprise customers — Microsoft’s bread and butter — to avoid generative AI. The company’s promise to protect them from legal action is a bold bet that the cost of defending customers will be far less than the profit it gains from selling generative products and services.
We’re thinking: It’s not yet clear whether using or developing generative AI violates anyone’s copyright, and it will take time for courts and lawmakers to provide a clear answer. While legal uncertainties remain, Microsoft’s commitment is an encouraging step for companies that would like to take advantage of the technology and a major vote of confidence in the business potential of generative AI.
A MESSAGE FROM SPEECHLAB
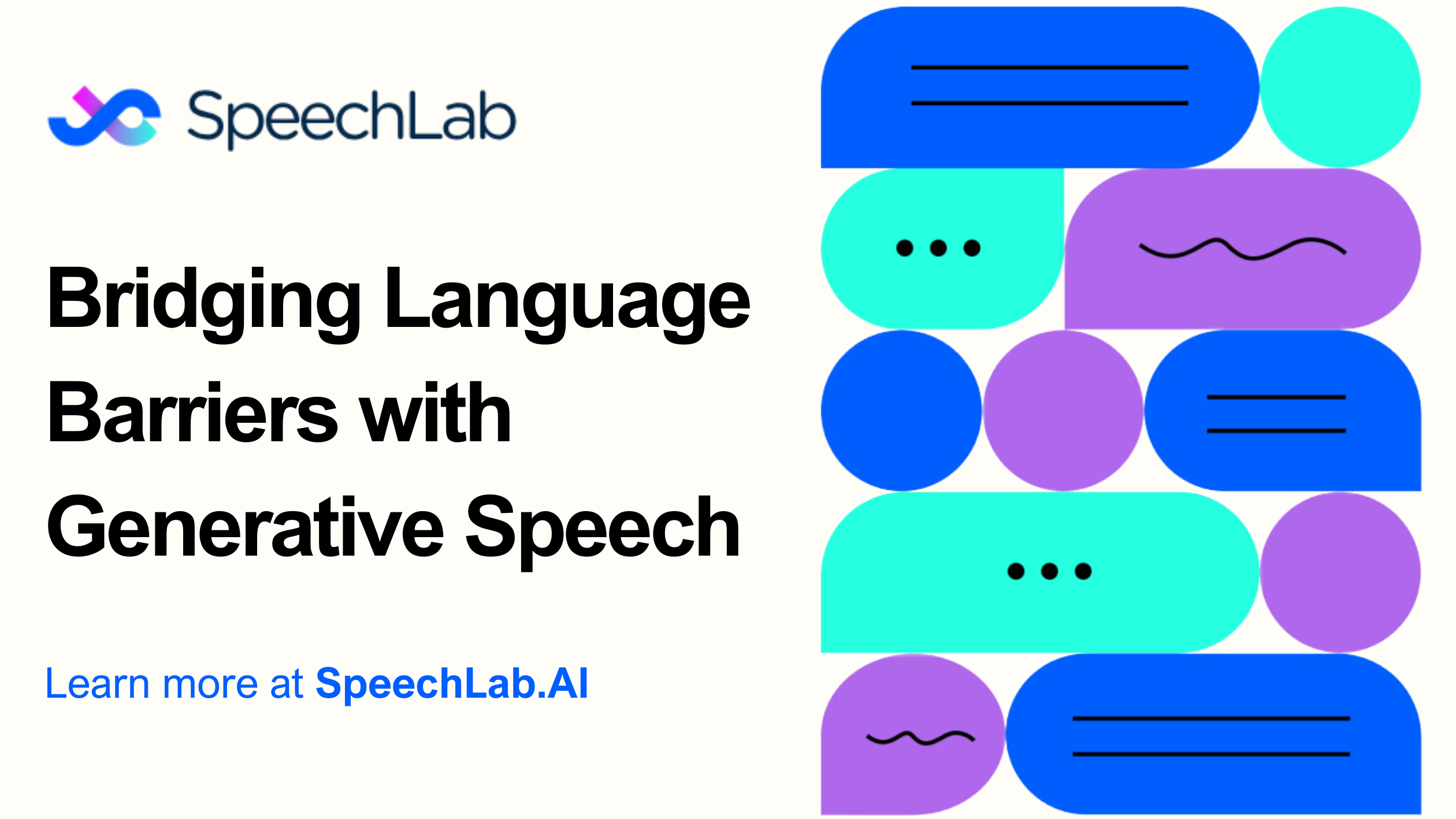
SpeechLab is building speech AI that conveys the nuance and emotion of the human voice, bringing together proprietary models for multi-speaker, multi-language text-to-speech; voice cloning; speech recognition; and more. Learn more at SpeechLab.AI
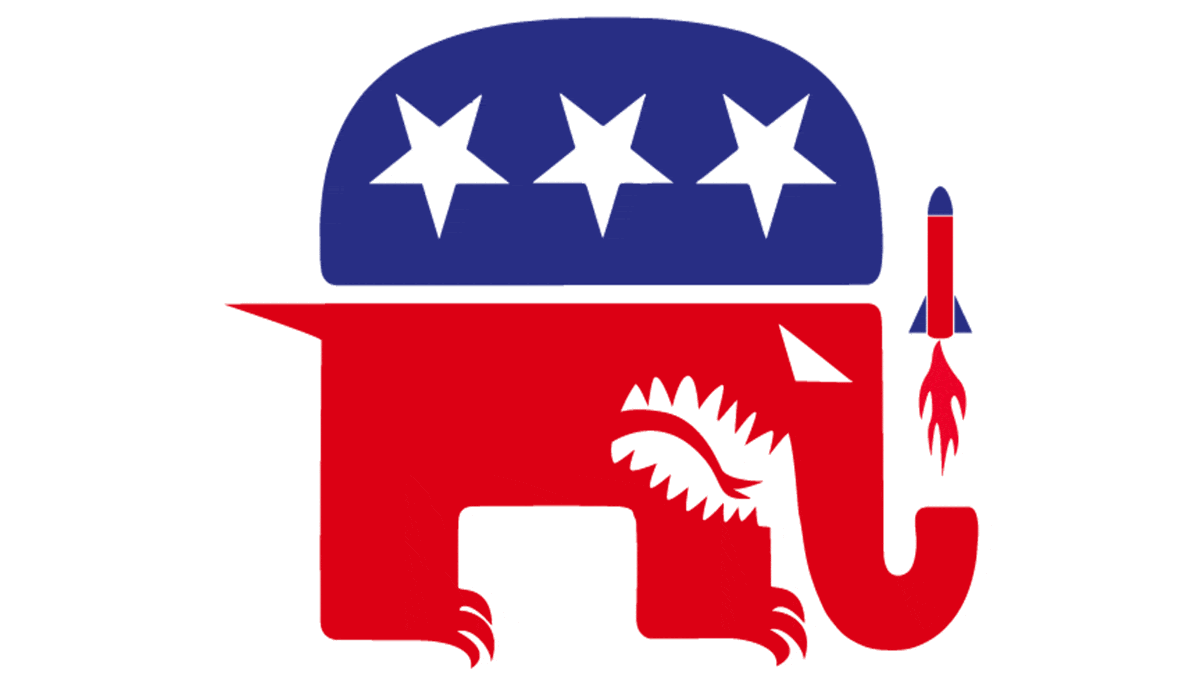
Truth in Online Political Ads
Google, which distributes a large portion of ads on the web, tightened its restrictions on potentially misleading political ads in advance of national elections in the United States, India, and South Africa.
What’s new: Starting in November 2023, in select countries, Google’s ad network will require clear disclosure of political ads that contain fictionalized depictions of real people or events, the company announced. The policy doesn’t explicitly mention generative AI, which can automate production of misleading ads.
How it works: In certain countries, Google accepts election-related ads only from advertisers that pass a lengthy verification process. Under the new rules, verified advertisers that promote “inauthentic” images, video, or audio of real-world people or events must declare, in a place where users are likely to notice it, that their depiction does not represent reality accurately.
- Disclosure will be required for (i) ads that make a person appear to have said or done something they did not say or do and (ii) ads that depict real events but include scenes that did not take place.
- Disclosure is not required for synthetic content that does not affect an ad’s claims, including minor image edits, color and defect corrections, and edited backgrounds that do not depict real events.
- The updated requirement will apply in Argentina, Australia, Brazil, the European Union, India, Israel, New Zealand, South Africa, Taiwan, the United Kingdom, and the United States. Google already requires verified election advertisers in these regions to disclose funding sources.
Behind the news: Some existing AI-generated political messages may run afoul of Google’s restrictions.
- A group affiliated with Ron DeSantis, who is challenging Donald Trump to become the Republican Party’s nominee for U.S. president, released an audio ad that included an AI-generated likeness of Trump’s voice attacking a third politician’s character. The words came from a post on one of Trump’s social media accounts, but Trump never spoke the words aloud.
- In India, in advance of a 2020 state-level election in Delhi, Manoj Tiwari of the Bharatiya Janata Party pushed videos of himself speaking in multiple languages. AI rendered the clips, originally recorded in Hindi, in Haryanvi and English, and a generative adversarial network conformed the candidate’s lip movements to the generated languages. In the context of Google’s requirements, the translated clips made it appear as though the candidate had done something he didn’t do.
- In January 2023, China’s internet watchdog issued new rules that similarly require generated media to bear a clear label if it might mislead an audience into believing false information.
Yes, but: The rules’ narrow focus on inauthentic depictions of real people or events may leave room for misleading generated imagery. For instance, a U.S. Republican Party video contains generated images of a fictional dystopian future stemming from Joe Biden’s hypothetical re-election in 2024. The images don’t depict real events, so they may not require clear labeling under Google’s new policy.
Why it matters: Digital disinformation has influenced elections for years, and the rise of generative AI gives manipulators a new toolbox. Google, which delivers an enormous quantity of advertising via Search, YouTube, and the web at large, is a powerful vector for untruths and propaganda. With its new rules, the company will assume the role of regulating itself in an environment where few governments have enacted restrictions.
We’re thinking: Kudos to Google for setting standards for political ads, generated or otherwise. The rules leave some room for interpretation; for instance, does a particular image depict a real event inauthentically or simply depict a fictional one? On the other hand, if Google enforces the policy, it’s likely to reduce disinformation. We hope the company will provide a public accounting of enforcement actions and outcomes.
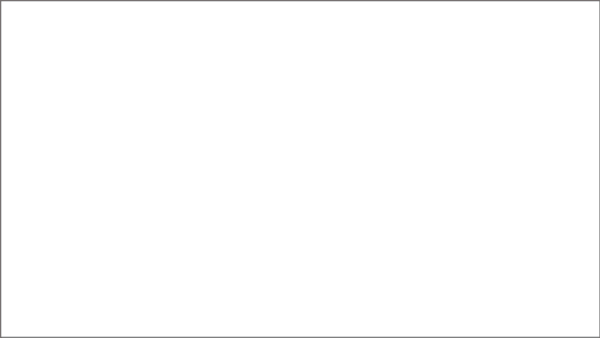
Masked Pretraining for CNNs
Vision transformers have bested convolutional neural networks (CNNs) in a number of key vision tasks. Have CNNs hit their limit? New research suggests otherwise.
What’s new: Sanghyun Woo and colleagues at Korea Advanced Institute of Science & Technology, Meta, and New York University built ConvNeXt V2, a purely convolutional architecture that, after pretraining and fine-tuning, achieved state-of-the-art performance on ImageNet. ConvNeXt V2 improves upon ConvNeXt, which updated the classic ResNet.
Key insight: Vision transformers learn via masked pretraining — that is, hiding part of an image and learning to reconstruct the missing part. This enables them to learn from unlabeled data, which simplifies amassing large training datasets and thus enables them to produce better embeddings. If masked pretraining works for transformers, it ought to work for CNNs as well.
How it works: ConvNeXt V2 is an encoder-decoder pretrained on 14 million images in ImageNet 22k. For the decoder, the authors used a single ConvNeXt convolutional block (made up of three convolutional layers). They modified the ConvNeXt encoder (36 ConvNeXt blocks) as follows:
- The authors removed LayerScale from each ConvNeXt block. In ConvNeXt, this operation learned how much to scale each layer’s output, but in ConvNeXt V2, it didn’t improve performance.
- They added to each block a scaling operation called global response normalization (GRN). A block’s intermediate layer generated an embedding with 384 values, known as channels. GRN scaled each channel based on its magnitude relative to the magnitude of all channels combined. This scaling narrowed the range of channel activation values, which prevented feature collapse, a problem with ConvNeXt in which channels with small weights don’t contribute to the output.
- During pretraining, ConvNeXt V2 split each input image into a 32x32 grid and masked random grid squares. Given the masked image, the encoder learned to produce an embedding. Given the embedding, the decoder learned to reproduce the unmasked image.
- After pretraining, the authors fine-tuned the encoder to classify images using 1.28 million images from ImageNet 1k.
Results: The biggest ConvNeXt V2 model (659 million parameters) achieved 88.9 percent top-1 accuracy on ImageNet. The previous state of the art, MViTV2 (a transformer with roughly the same number of parameters) achieved 88.8 percent accuracy. In addition, ConvNeXt V2 required less processing power: 600.7 gigaflops versus 763.5 gigaflops.
Why it matters: Transformers show great promise in computer vision, but convolutional architectures can achieve comparable performance with less computation.
We’re thinking: While ImageNet 22k is one of the largest publicly available image datasets, vision transformers benefit from training on proprietary datasets that are much larger. We’re eager to see how ConvNeXt V2 would fare if it were scaled to billions of parameters and images. In addition, ImageNet has been joined by many newer benchmarks. We’d like to see results for some of those.
Data Points
Australia bans AI-generated depictions of child abuse from search engines
Australia's eSafety Commissioner required search engines including Google and DuckDuckGo to remove synthetic child abuse material from their results. The mandate also requires such companies to research technologies that help users identify deepfake images on their platforms. (The Guardian)
Peer reviewers uncover AI-generated science research
Generative AI tools are being used to write scientific papers without disclosure. Some generated papers have passed peer review. Researchers and reviewers identified such manuscripts through telltale phrases left unedited by users, like “regenerate response.” This suggests that the actual number of undisclosed AI-generated peer-reviewed papers could be significantly higher. (Nature)
Education integrates AI
Educators, institutions, and nongovernmental organizations are grappling with the challenges and possibilities of AI in the classroom. Institutions are establishing policies and regulatory bodies are developing guidelines. Meanwhile, students and educators alike are exploring AI's limitations and benefits. (Reuters)
Baidu releases Ernie chatbot
The move made Baidu’s stock price raise by over 3% following the announcement. On the day of release, the chatbot topped the charts on Apple’s iOS store in China for free apps. (AP News)
Walmart workers get generative AI app trained on corporate data
Approximately 50,000 non-store employees will have access to the app, called "My Assistant.” It will be capable of performing tasks like summarizing long documents. The app is based on an LLM provided by an unnamed third party. (Axios)
U.S. restricts export of AI chips to Middle East countries
The U.S. extended export restrictions on advanced AI chips produced by Nvidia and Advanced Micro Devices (AMD), which previously blocked chips sold to China, to include countries in the Middle East. These restrictions are part of a wider effort by the U.S. government to control exports of advanced technology that could be used for military purposes to certain regions. (Reuters)
How countries around the world are regulating AI
From Brazil's detailed draft AI law focusing on user rights to China's regulations emphasizing "Socialist Core Values," nations are working to establish restrictions on AI. The EU, Israel, Italy and the United Arab Emirates are also working on regulations. (The Washington Post)
Apple intensifies investment in conversational AI
Apple’s plan would enable iPhone users to perform multi-step tasks using voice commands. This initiative aligns with Apple's earlier establishment of an AI team to develop conversational AI. (The Information)
Gizmodo owner replaced Spanish-language journalists with machine translation
G/O Media laid off the editors of Gizmodo en Español and is employing AI for article translation. Readers reported issues such as articles switching from Spanish to English mid-text. The staff’s union criticized the decision as a promise broken by the company's leadership. (The Verge)
AI-generated mushroom foraging guides raise safety concerns
Manuals on identifying mushrooms have proliferated on Amazon. Experts warn that these books, aimed at beginners, often lack accuracy in identifying poisonous mushrooms, posing a potential risk to those who rely on them. Amazon has taken steps to remove some of these books, which illustrate the potential stakes of ensuring safety and accuracy in AI-generated content. (404 media)
AI generates grown-up likenesses of children whose parents disappeared decades ago
An Argentine publicist uses Midjourney to combine photos of parents who disappeared during that country’s dictatorship of the 1970s and ‘80s to generate portraits of their children as adults. The activist group Grandmothers of Plaza de Mayo created the images, which aim to raise awareness of more than 500 children who were stolen from their parents and never photographed. (AP news)
Morgan Stanley to launch chatbot for wealth management services
The investment bank is set to introduce a chatbot developed in collaboration with OpenAI. It will help bankers swiftly access research and forms. Morgan Stanley and OpenAI are also developing a feature to summarize meetings, draft follow-up emails, update sales databases, schedule appointments, and provide financial advice on various areas. (Reuters)