Dear friends,
Amidst rising worry about AI harms both realistic (like job loss) and unrealistic (like human extinction), It’s critical to understand AI’s potential to do tremendous good. Our new specialization, AI for Good is designed to empower both technical and nontechnical people to identify, scope, and build impactful AI projects.
In this series of courses, you’ll learn when and how to use AI effectively for positive impact in situations where stakes are high and human lives may hang in the balance. AI for Good presents a practical framework for applying machine learning to socially important projects (and products of any kind). It illustrates this framework with several real-world examples of AI projects that are improving climate change, disaster response, and public health.
AI for Good is designed to be useful whether or not you have coding experience. It does include Python code examples that you can execute and interact with to gain deeper insight into different applications. However, it doesn’t assume previous experience with AI or programming. So please recommend this to your nontechnical friends!
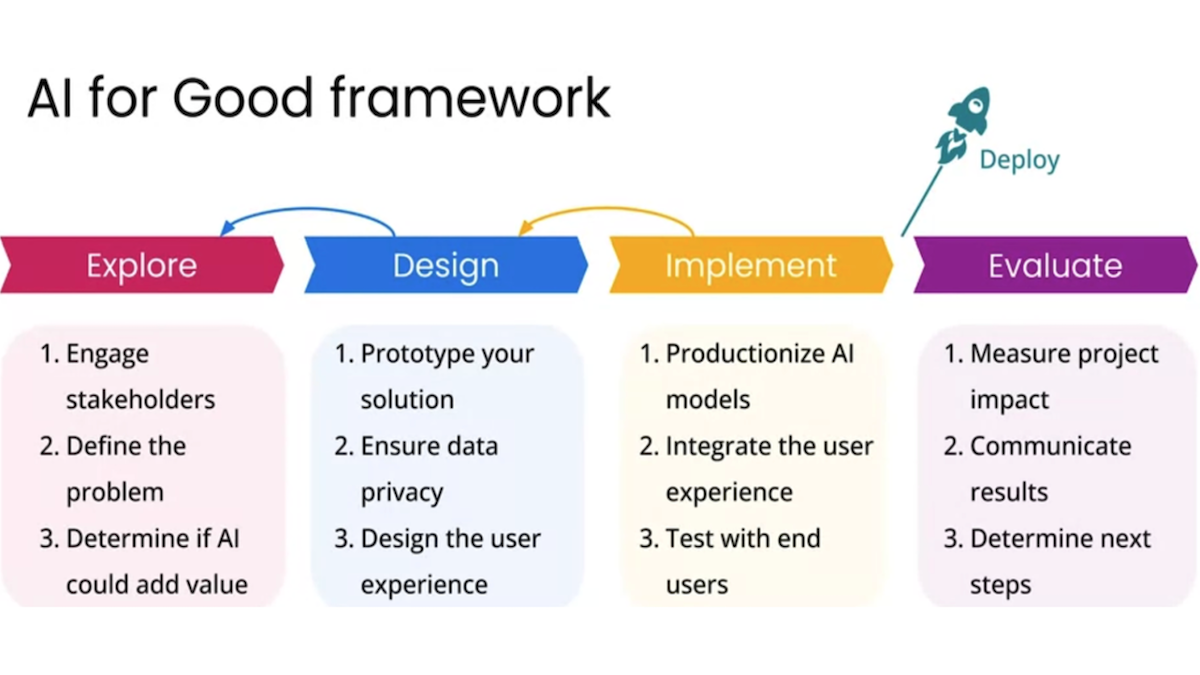
There’s often a huge gap between training a model that does well on a test set and one that actually works on real data and affects real people. This specialization will help you tell the difference, so your projects reach people and better their lives.
AI for Good is taught by Robert Monarch, who has applied AI in public health and disaster response for over 20 years. He has founded AI startups and shipped successful AI products at Amazon, Google, Microsoft, and Apple. He’ll show you how to move your own AI projects through the stages of exploration, design, implementation, and evaluation.
AI is experiencing a time of rapid growth, and the AI community’s role in making sure it does significant good is more important than ever. I hope you’ll check out AI for Good!
Do good,
Andrew
P.S. We also have a new short course: “Understanding and Applying Text Embeddings with Vertex AI,” developed in collaboration with Google Cloud and taught by Nikita Namjoshi and me. Learn the fundamentals of text embeddings — an essential piece of the GenAI developer’s toolkit — and apply them to classification, outlier detection, text clustering, and semantic search. You’ll also learn how to combine text generation and semantic search to build a question-answering system. Please join us!
News
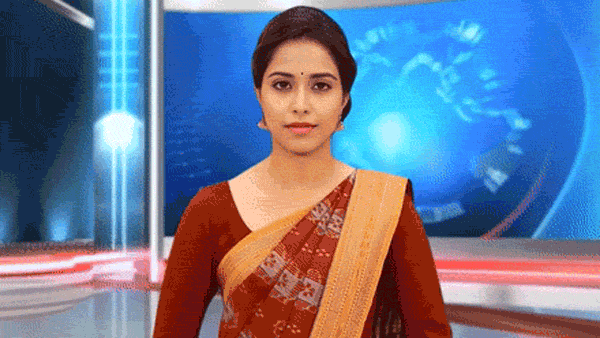
Fake Newscasters
Tonight at 11: I’m an AI-generated character, and I’ll be bringing you the latest headlines.
What’s new: Indian broadcasters have embraced synthetic news presenters, Nikkei Asia reported. Their counterparts in other Asian countries also rely increasingly on automated anchors.
Invasion of the newsbots: Synthetic presenters can deliver reports generated directly by large language models and do so in multiple languages. One news producer noted that they also give newsrooms a break from the typical presenter’s outsized ego. None of the broadcasters has disclosed the technology they’re using.
- In July, Eastern India’s Odia-language Odisha TV introduced Lisa. Southern India’s Kannada-language Power TV debuted Soundarya at around the same time.
- Taiwan’s FTV News introduced an unnamed synthetic presenter in June. The broadcaster promoted the character by announcing a naming contest.
- In May, Malaysian news channel Astro AWANI introduced two AI-generated hosts. Joon presents the evening news. Monica hosts a nightly talk show.
- The previous month, Indonesian free-to-air channel tvOne introduced a trio of AI news anchors: Nadira, a look- and soundalike of human tvOne presenter Fahada Indi; and Sasya and Bhoomi, who appear as an Indonesian Chinese and an Eastern Indonesian, respectively, to engage different audiences. The same month, Kuwait News unveiled Fedha, described as the Middle East’s first AI news presenter.
- Delhi-based India Today may have kicked off the trend in March, when Sana started delivering news and weather in English, Hindi, and Bengali.
Behind the news: Synthetic news presenters go back at least to 2018, when Chinese state news agency Xinhua and search engine Sogou introduced pioneering 2D newsbots. Their images were drawn from videos, while their motions and voices were driven by machine learning. Two years later, the broadcaster upgraded to 3D-rendered avatars produced using “multimodal recognition and synthesis, facial recognition and animation and transfer learning.”
Yes, but: While broadcasters can use AI-generated talking heads to save time and money, propagandists can use them to gain an aura of newsy credibility. For example, an unidentified group used Synthesia, a web service that makes AI-generated characters, to generate fake news clips from a fictional outlet called Wolf News. One clip attacked the U.S. government for failing to take action against gun violence, while another promoted cooperation between the U.S. and China.
Why it matters: Synthetic presenters potentially multiply the power of broadcast news by generating an unlimited variety of talking heads. They can appeal to specific audience segments by representing any ethnicity, gender, age, or style. And they can reach an even broader audience by speaking a variety of languages — a boon to broadcasters especially in highly multilingual Asian societies.
We’re thinking: It may not be a coincidence that synthetic presenters are appearing first in countries whose people feel more positively about AI. According to one survey, people in India, Indonesia, and Malaysia trust AI more than do people in Western countries.
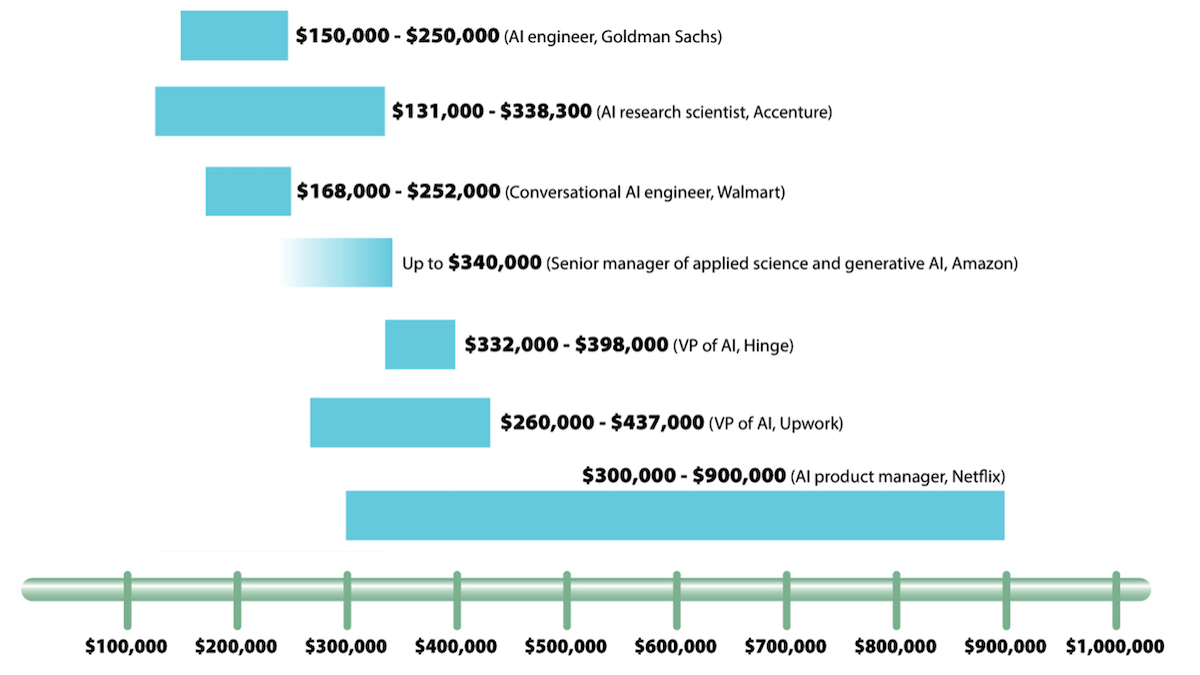
High Wages for AI Talent
Enthusiasm for AI is driving top salaries for engineers and executives into the stratosphere.
What’s new: Companies that advertise open AI positions are listing annual pay scales well into six figures. In at least one case, the proposed salary approaches seven figures, The Wall Street Journal reported.
Generative jobs: On the help-wanted site Indeed, listings by U.S. companies that mention generative AI have jumped around 100 percent year-on-year, even as total listings declined slightly. Tech and non-tech companies alike have posted AI job notices that mention generous salaries. For reference, the average machine learning product engineer job in the U.S. pays around $143,000 annually, according to a study by insurance company Willis Towers Watson. Wages may be lower in other countries.
- Accenture mentioned a pay range between $131,000 and $338,300 for advanced AI research scientists. Goldman Sachs listed an AI engineer role with a salary between $150,000 and $250,000 plus an unspecified bonus. Walmart posted a position on its conversational AI team with a salary between $168,000 and $252,000.
- The figures rise for leadership roles. Amazon sought a senior manager of applied science and generative AI with a top salary of $340,000. Hinge, a dating app, advertised for a vice president of AI with a salary between $332,000 and $398,000. Upwork, which connects freelancers with employers, posted an AI vice president position with a salary range of $260,000 to $437,000.
- Netflix established a high-water mark when it advertised an AI product manager role that paid between $300,000 and $900,000. The offer didn’t escape notice by Hollywood screenwriters and actors who went on strike partly for protection against being replaced by generative AI models.
Behind the news: Skilled AI professionals remain in demand even as large tech companies are hiring fewer workers overall.
- 1.9 percent of U.S. job listings last year (omitting agriculture, forestry, fishing, and hunting) were related to AI, up from 1.7 percent the prior year, according to the 2023 AI Index.
- The number of U.S. workers in AI leadership roles has tripled in the past five years.
- The World Economic Forum forecast that global demand for specialists in AI and machine learning will grow by 40 percent to 1 million jobs between 2023 and 2027.
Why it matters: Even as demand is rising, AI talent remains scarce. The shortage prompts employers to offer high salaries in hope of attracting candidates with the skills and experience they need. That situation spells opportunity for people who put in the time, effort, and passion to develop a career in the field.
We’re thinking: We’re thrilled by the number of people who are participating in AI and earning good wages. Yet there’s more to job satisfaction than maximizing your salary. In the long term, the opportunity to work on interesting projects, make a meaningful impact, or work with great people is more likely to affect your happiness and professional attainment than the pay scale. Follow your interests, do your best work, aim to make the world a better place and — above all — keep learning!
A MESSAGE FROM DEEPLEARNING.AI
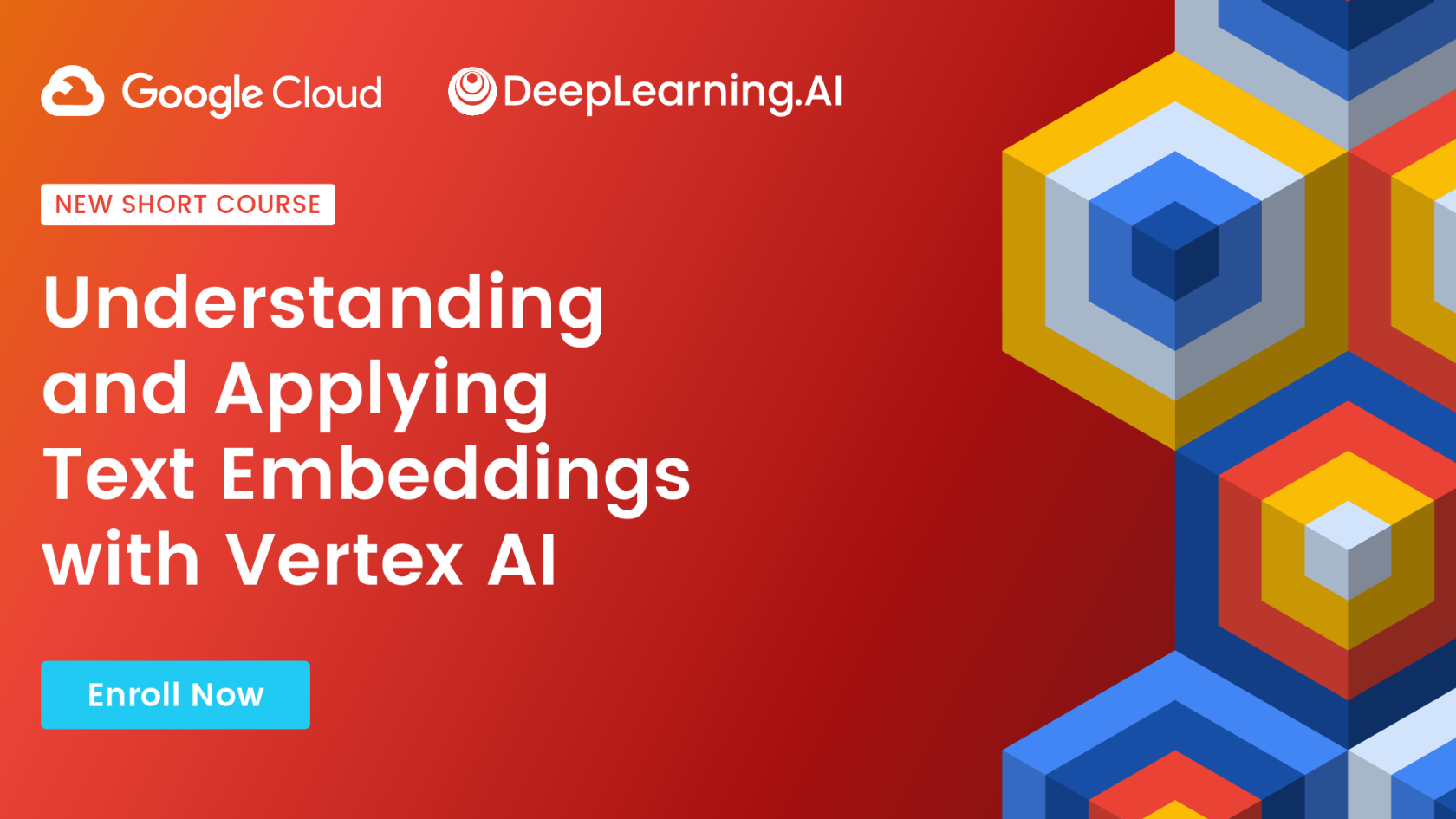
Learn how to generate and apply text embeddings in applications based on large language models! Check out our short course built in collaboration with Google Cloud, “Understanding and Applying Text Embeddings with Vertex AI.” Start learning today
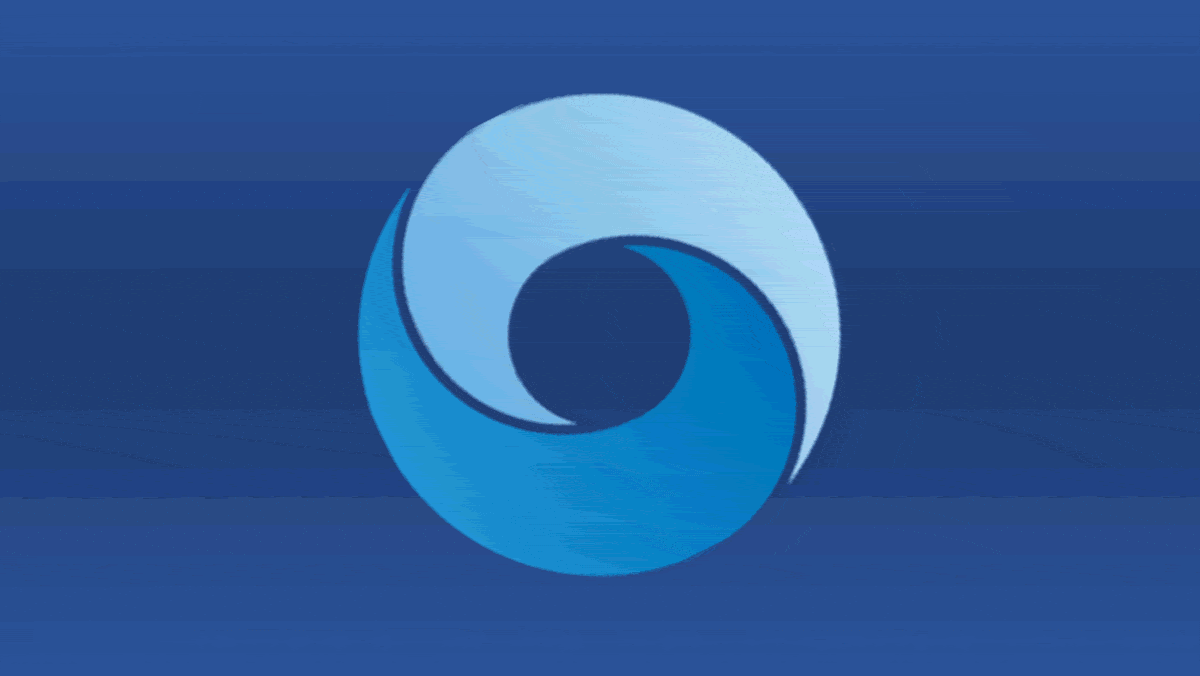
DeepMind’s Offspring Proliferate
Where spores from DeepMind scatter, startups blossom.
What’s new: Nearly 200 former employees of Google’s elite AI research lab have gone on to found or join startups, Business Insider reported.
Emerged from stealth: Venture capital firms are eager to fund projects that involve ex-DeepMinders, and alumni often benefit from angel investments by their former colleagues. While many such projects are in stealth mode, some have revealed themselves.
- Founded by DeepMind co-founder Mustafa Suleyman and former principal research scientist Karén Simonyan, Inflection AI builds conversational large language models such as the Pi chatbot. In June, the company announced a gigantic $1.3 billion funding round led by Microsoft and Nvidia.
- Mistral, co-founded by Arthur Mensch, a former DeepMind senior research scientist, seeks to build open-source language models. It secured a $113 million seed round in June, just four weeks after it was founded.
- Co-founded by ex-DeepMind senior research engineer Jonathan Godwin, Orbital Materials builds models that help develop new materials for applications such as renewable energy and carbon capture.
- Latent Labs, started by erstwhile AlphaFold team lead Simon Kohl, plans to build generative AI tools for biology.
- Brainchild of ex-DeepMind research engineers Devang Agrawal and Adam Liska, GlyphicAI is developing chatbots for business-to-business sales teams. The startup raised $5.5 million in pre-seed funding in June.
Behind the news: Acquired by Google in 2014, DeepMind has developed several high-profile innovations and popularized reinforcement learning. Earlier this year, it merged with Google Brain (which Andrew Ng started and formerly led).
- DeepMind established its reputation for cutting-edge research with AlphaGo, a reinforcement learning system that bested Go world champion Lee Sedol in 2016.
- In 2018, the lab astonished the biomedical community with AlphaFold, a model that finds the structures of proteins — a capability that could lead to discovery of new medicines and other biologically active compounds. The lab spun out a startup, Isomorphic, to capitalize on the achievement.
- DeepMind also has contributed important work in AI-based fluid dynamics and energy forecasting.
Why it matters: Tech giants are magnets for AI talent, and top employees gain valuable practical and market experience. Yet many come to feel confined by conditions within an established company. Former DeepMinders who formed their own companies cited their desire to follow currents of deep learning, such as generative AI, that their former employer doesn’t emphasize and their need for flexibility to pursue goals that didn’t necessarily revolve around machine learning.
We’re thinking: While high-profile associations often attract capital and attention, great ideas can come from anywhere. They seldom happen overnight; usually, they’re the end result of a long incubation period spent honing them through experimentation and feedback. Start small and develop your intuition, skills, and credibility. That’s how pretty much everyone started who ended up having a huge impact!
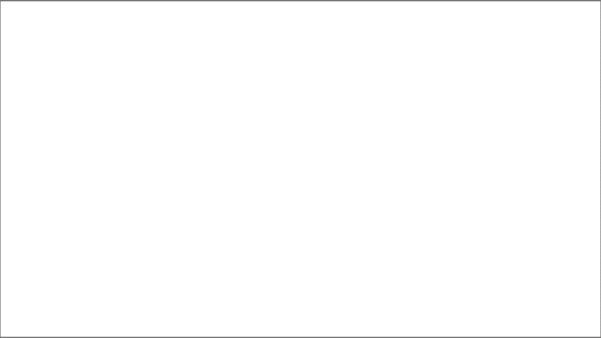
Different Media, Similar Embeddings
The ability of OpenAI’s CLIP to produce similar embeddings of a text phrase and a matching image (such as “a photo of a cat” and a photo of a cat) opened up applications like classifying images according to labels that weren’t in the training set. A new model extends this capability to seven data types.
What’s new: Rohit Girdhar, Alaaeldin El-Nouby, Ishan Misra, and colleagues at Meta developed ImageBind, a system that produces similar embeddings of text phrases, audio clips, images, videos, thermal images, depth images, and Inertial Measurement Unit (IMU) readings (which include accelerometer and gyroscope measurements).
Key insight: One challenge to learning multimodal embeddings is access to training data that includes matched pairs of all data types involved. For instance, matched image-text pairs, image-depth pairs, and image-thermal pairs are readily available, but pairings of text-thermal, text-depth, and so on are not. Learning to produce similar embeddings given pairings of one media type (in this case images) with other media types will transfer to pairings of pairings of that type with further types. There’s no need for specific training for each pairing.
How it works: ImageBind uses a separate transformer to embed each media type with one exception: The transformer that processes images handles video as well by treating a video as a two-frame image (sampled from the video).
- The training data comprised matched pairs of video-audio clips from YouTube, image-depth scenes shot by a depth camera, image-thermal pictures of street scenes at night, and video-IMU shot from a first-person point of view.
- Instead of training image and text encoders from scratch, the authors adopted the encoders from OpenCLIP, which is pretrained on billions of image-text pairs.
- The transformers learned via a contrastive loss function. Given an image (or video) and its match in another data type, the loss encouraged them to produce similar embeddings. Given an image (or video) and an example that didn’t match, it encouraged them to produce dissimilar embeddings.
Results: The authors use a method similar to CLIP to classify data using ImageBind. For example, using the Clotho test set of roughly 1,000 audio and text descriptions, ImageBind compared the embedding of a description with the embedding of every audio clip and returned the most similar audio clip. ImageBind returned the correct audio clip 6 percent of the time, whereas AVFIC, which learned using pairs of audio and text, returned the correct audio clip 3 percent of the time. However, ImageBind did not match supervised learning. ARNLQ, a supervised model, returned the correct audio 12.6 percent of the time.
Why it matters: The authors’ approach acts as an upgrade for models that generate similar embeddings for examples that have similar meanings in different media: To enhance the model’s repertoire with a new data type (say, audio), simply fine-tune it on relevant paired data (such as image, audio).
We’re thinking: ImageBind shows that machine learning models don’t need to learn from all pairs of data types to produce similar embeddings among various data types. Still, we can’t help but wonder how much its performance would improve if it did learn from other pairings, like (text, audio).