Dear friends,
An ill-advised proposal for a 6-month pause in cutting-edge AI research got far more attention than I think it deserved. To me, this is a wake-up call that AI doomsayers have done a much better job than AI optimists at framing the narrative of progress in AI.
Most of the AI community is building systems that help and empower people, and we see every day how it is improving lives. Open AI’s ChatGPT is delivering value to hundreds of millions of users, and reportedly it’s the fastest-growing consumer application to date. This is wildly exciting, and I foresee many more products yet to be built that will help and empower people in other ways.
Yet, while most of us have been building useful systems, AI doomsayers — who forecast unlikely scenarios such as humanity losing control of runaway AI (or AGI, or even superintelligent systems) — have captured the popular imagination and stoked widespread fear.
Last week, Yann LeCun and I had an online conversation about why the proposed 6-month pause, which would temporarily suspend work on models more powerful than GPT-4, is a bad idea. You can watch the video here and read a synopsis in this article. Briefly:
- The proposal’s premises with respect to AI’s potential for harm are sensationalistic and unrealistic.
- A pause in development is unworkable— that is, unless governments intervene, which would have an even worse impact on competition and innovation.
- If it were implemented, it would (i) slow down valuable innovations and (ii) do little good, because it seems unlikely that a 6-month pause in our decades-long journey toward AGI would have much useful impact.
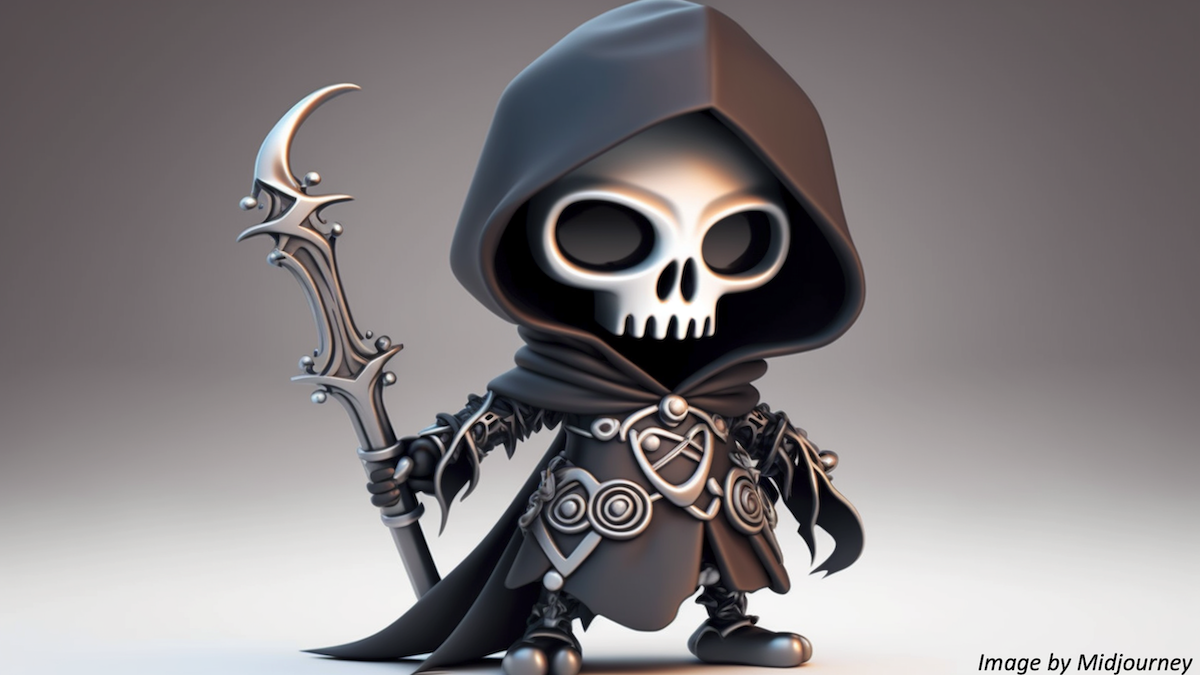
To be clear, AI has problems including bias, fairness, job displacement, and concentration of power. Our community should work, and is working, to address them. However, stoking fears about speculative risks does more harm than good:
- It distracts us from the real and present risks that we should be working on.
- It is another form of hype about AI, which misleads people to overestimate AI’s capabilities.
- It risks slowing down further progress in AI that would be very beneficial.
I’m disappointed that we have let AI doomsayers get this far. Their narrative hampers innovation, discourages individuals, and interferes with society’s ability to make good decisions.
Let’s help people understand that AI is empowering people even as we work to mitigate the real risks. It’s time for us all to stand up for a realistic view of this incredibly important technology.
Keep learning!
Andrew
P.S. Shoutout to University of Washington’s Emily Bender for her line-by-line analysis of how the proposal contributes to AI hype, and Princeton professor Arvind Narayanan, who explained how fears of AI-driven dangers such as misinformation often have been overblown.
News
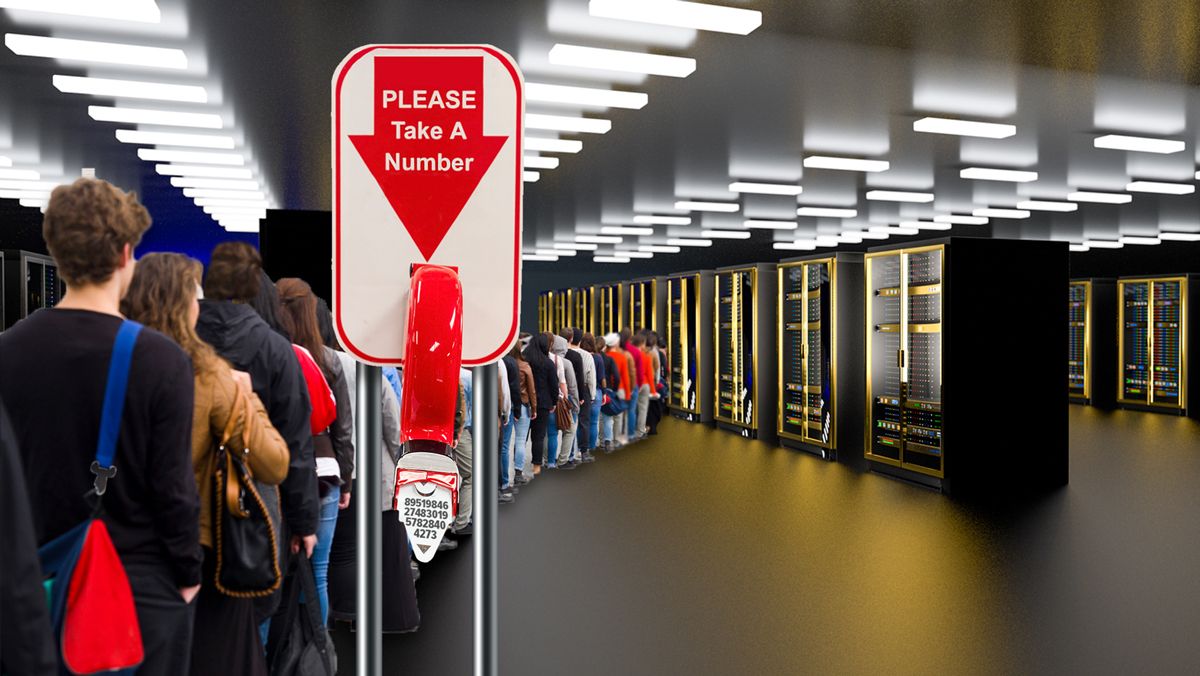
AI Startups Face Compute Shortage
Chatbot-fueled FOMO is overwhelming cloud-computing services.
What’s new: Cloud providers are struggling to meet sharply rising demand by a crowd of AI startups eager to cash in on generative AI, The Information reported.
Behind the bottleneck: The surge in demand caught Amazon Web Services, Microsoft Azure, and others off guard.
- Some cloud providers didn’t place their orders for extra AI chips early enough, while Nvidia, which manufactures the specialized GPUs that process many AI workloads, typically takes months to fulfill orders. (Google Cloud, which uses proprietary TPU chips, said it has been able to meet nearly all its customer demand.)
- Microsoft has been rationing GPU access for its internal teams. Microsoft partner OpenAI has had to slow down development.
- Electrical power is in short supply in Northern Virginia and Northern California’s Silicon Valley, two of the biggest data-center markets. The shortages have driven up cloud computing costs and further strained server capacity.
What they’re saying: Engineers and entrepreneurs shared their pain.
- Yasyf Mohamedali, engineer in residence at venture capital firm Root Ventures, said it was impossible to find servers without prepayment or an existing contact.
- Naveen Rao, CEO of startup MosaicML, said customers who had committed to multi-year spending had better luck gaining access to large blocks of servers.
- Some startups are turning to smaller cloud providers like RunPod, Lambda Labs, Crusoe Energy, and CoreWeave.However, even these firms are struggling to meet demand, said Stephen Balaban, CEO and co-founder of Lambda Labs.
- Even customers that get access to cloud servers often lack sufficient capacity, said Johnny Dallas, founder and CEO of Zeet, which automates management of cloud services.
Behind the news: China is facing its own chip shortage — and finding ways to address it. That situation, though, is a result of United States trade sanctions rather than a surge in demand.
Why it matters: Startups that serve a market with generated text or pictures are white-hot, but even the most promising ventures can’t do without servers to build, test, and deploy their models. The winners will need not only a great product but also ready access to computation.
We’re thinking: Our hearts go out to everyone who is trying to build AI products in these unpredictable times. We trust that the supply of compute will catch up in due course and that the current run of AI-fueled growth will continue for the foreseeable future.
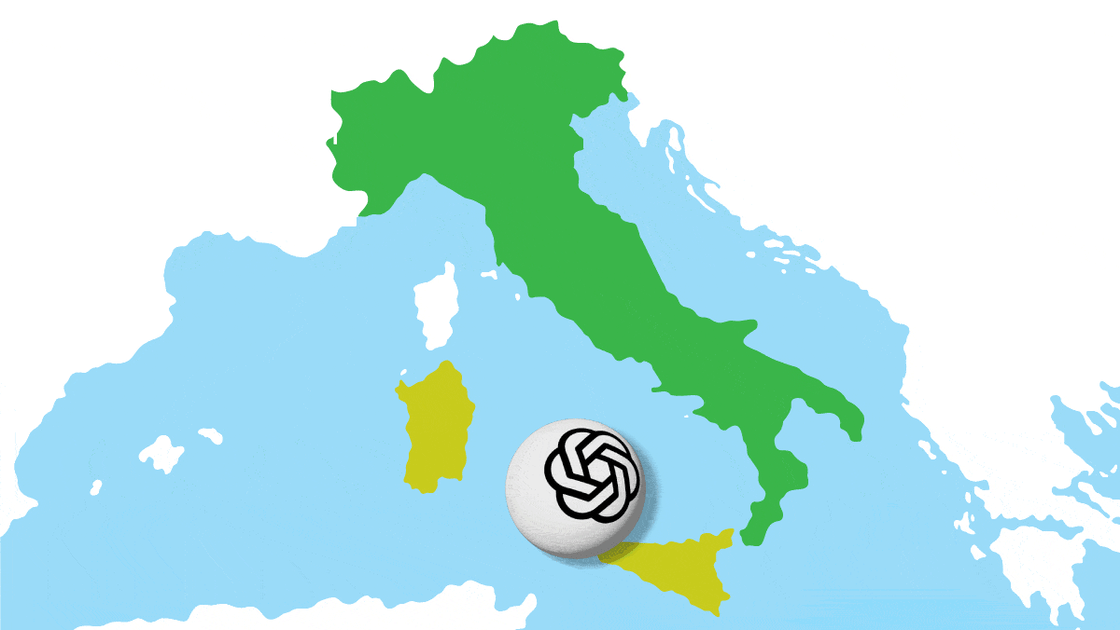
Italy Boots ChatGPT
Italy blocked ChatGPT after determining that it violates European Union laws.
What’s new: The Guarantor for the Protection of Personal Data suspended access to ChatGPT for 20 days after saying that OpenAI enables underage children to use the chatbot, distributes misinformation about people, and collects personal data used to train its models without proper authority.
The ruling: The Guarantor, which enforces the rules in Italy, banned ChatGPT for 20 days citing four concerns: The chatbot doesn’t prevent children under 13 from using it, the chatbot can provide inaccurate information about individuals, OpenAI did not inform individuals that the firm was collecting data that could be used to identify them, and OpenAI did not meet the EU privacy law’s guidelines for collecting personal data.
- The Guarantor gave OpenAI 20 days to respond with a plan that would address these issues. Failure to comply would have resulted in a fine worth 4 percent of OpenAI’s global revenue, which the company expects to exceed $200 million.
- On April 8, OpenAI submitted an unspecified plan. OpenAI CEO Sam Altman previously tweeted that he believed ChatGPT did comply with EU law.
Behind the news: Privacy regulators in Europe and the United States have their eyes on AI.
- Earlier this year, the same Italian regulator deemed the AI chatbot Replika a threat to emotionally vulnerable individuals. The regulator ordered the developer to cease processing Italian users’ data or face a €20 million fine.
- In 2022, British, French, Greek, and Italian authorities issued concurrent fines to Clearview AI, which provides face recognition services to law enforcement agencies, and ordered the company to delete personal data that described their citizens.
- In 2022, U.S. regulators ruled that Kurbo, a weight-loss app, violated a 1998 law that restricts collection of personal data from children under 13. The developer paid a fine, destroyed data, and disabled the app.
Yes, but: Not everyone in the Italian government agrees with the ruling. Matteo Salvini, one of the country’s two deputy prime ministers, criticized it as excessive.
Why it matters: A national (or international) ban on ChatGPT could have major implications for large language models, which rely on sprawling datasets and routinely output misinformation. It could also harm European innovation by blocking access to the latest technology. And it’s not just Italy: French, German, and Irish regulators reportedly are considering similar actions. Belgian regulators went a step further and called for an EU-wide discussion of data violations related to ChatGPT.
We’re thinking: Some of the regulators’ concerns may stem from a lack of transparency into how OpenAI trains its models. A more open approach might alleviate some fears.
A MESSAGE FROM AIFUND
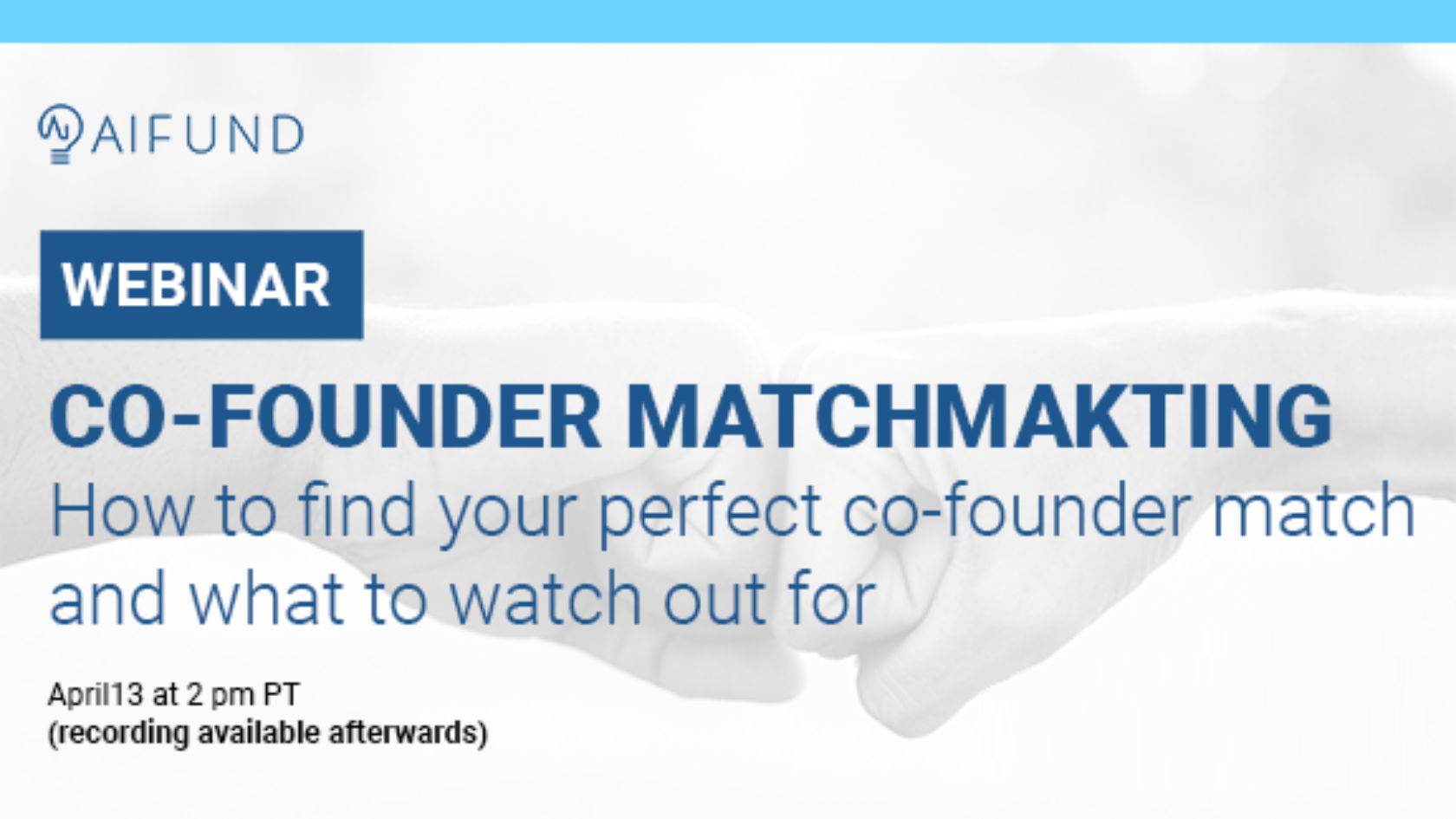
The core of many successful companies is a strong co-founder partnership. Join us on April 13, 2023, at 2:00 p.m. Pacific Time to learn how to find your perfect co-founder. Register now
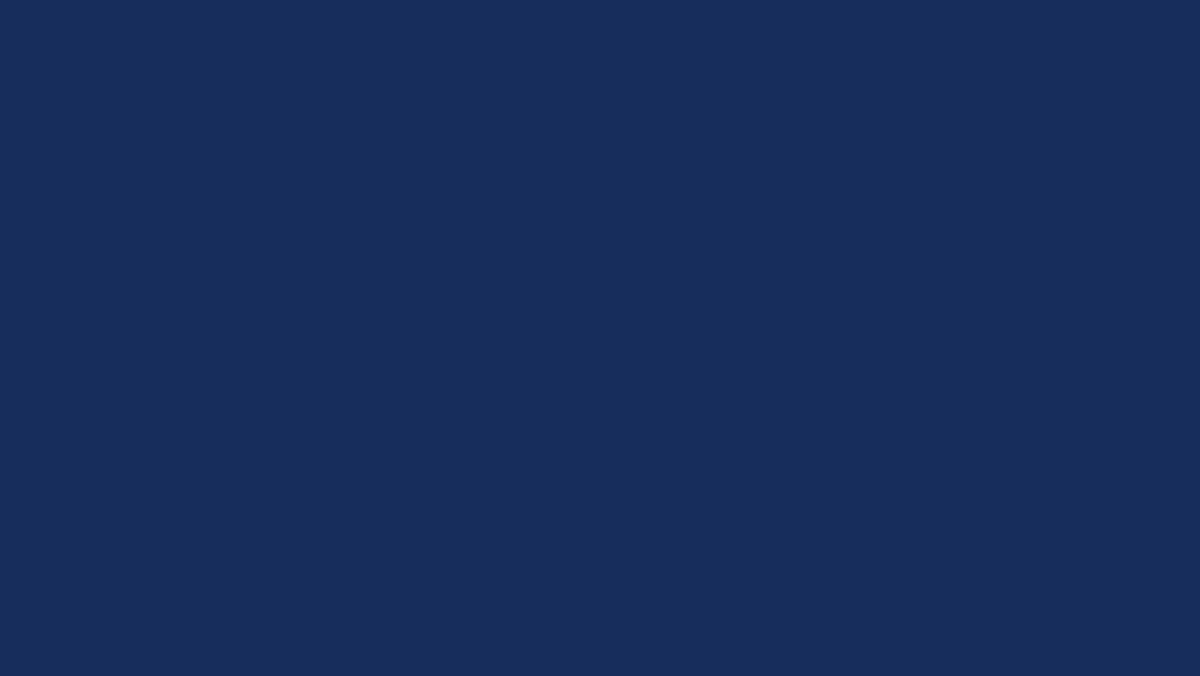
AI Trends Tracked
Stanford’s sixth annual AI Index takes stock of a rapidly growing field.
What’s new: The sprawling, 386-page report from the Institute for Human-Centered AI presents the past year’s developments in AI based on a wide variety of sources including benchmarks, papers, market research, job listings, and polls. (You can find info about earlier editions here.)
Reasons for celebration: The report highlights several positive trends:
- In 2022, 50 percent of companies reported that they had adopted AI in at least one business unit or function. This figure has fluctuated between around 50 and 60 percent since 2018. 63 percent of companies that have adopted AI reported an increase in revenue, and 37 percent reported lower costs.
- Scientists used AI to make important breakthroughs. Among them: controlling plasma for nuclear fusion, improving algorithms for matrix multiplication, designing novel antibodies for drug discovery, and improving the power efficiency of chips.
- Researchers express increasing interest in AI ethics. The 2022 Conference on Fairness, Accountability, and Transparency received 772 papers, more than double the previous year’s submissions.
- While training large AI models continues to have an outsized carbon footprint, evidence suggests that this could change. In 2022, training BLOOM emitted as much carbon dioxide as an average human would in four years. In 2020, training GPT-3, which is around the same size, emitted more than an average human would in 91 years.
Causes for concern: Not everything in the report is rosy:
- Global private-sector investment in AI declined to $92 billion in 2022 from around $125 billion in 2021.
- Questionable uses of AI are on the rise. A clearinghouse of such incidents recorded 260 incidents in 2022, 26 times higher than it had recorded a decade earlier. A few notable incidents include: A deepfaked video of Ukrainian president Voldymyr Zelenskyy calling for Ukrainians to surrender to Russia, U.S. prisons using AI to transcribe prisoners’ phone calls, and a gang-tracking tool criticized for having a racial bias.
- The field continues to struggle with gender diversity. Women receive 22 percent of new North American bachelor’s degrees in computer science, up from 10 percent a decade ago.
Behind the news: The new report surveyed the AI’s recent past, but other measures indicate the near-term future. An April study by investment bank Goldman Sachs found that generative AI could boost the global productivity by 7 percent in the coming decade as it automates tasks that affect 300 million full-time jobs. Meanwhile, at the startup incubator Y Combinator, AI is at the heart of 34 percent of newly formed companies — the highest number on record.
Why it matters: The AI Index offers a sober yet exciting summary of AI’s march into all areas of society. Immense opportunities and grave challenges alike lie ahead.
We’re thinking: Focusing on 2022, this report doesn’t reflect the staggering impact of generative AI — a reminder of the extraordinary pace of development as well as AI’s potential in areas well beyond the current buzz.
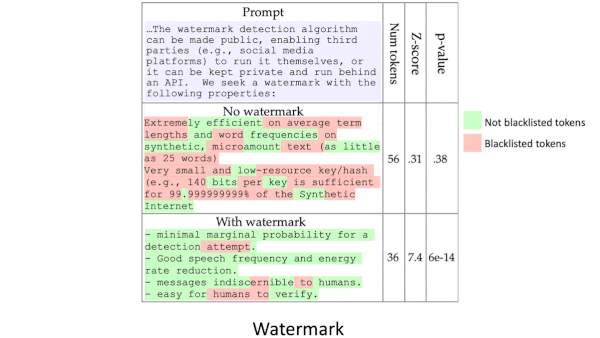
Detecting Generated Text: Three Methods
How can you tell when you’re reading machine-generated text? Three recent papers proposed solutions: Watermarking, classification, and a statistical method.
Watermark: John Kirchenbauer, Jonas Geiping, and colleagues at University of Maryland applied a digital watermark, invisible to humans but detectable by an algorithm, to generated text. Their method adjusted the way in which the model chose which word would come next.
- To watermark text, when each new word was generated, the authors hashed the previous word to seed a random number generator. They used the random number generator to assign 20 percent of the model’s vocabulary to a blacklist. Then they reduced the probability that those words would appear in the output.
- Given a text, the authors compared the number of blacklisted words to the number expected in an output of the same length without watermarking. They considered the watermark to be present if the comparison passed a certain threshold.
- Given watermarked text from a pretrained OPT-1.3B and a random selection of news text from C4, they detected 99.6 percent of watermarked text. Watermarking had little impact on the character of the text according to average perplexity (a measure of how easy it is to predict the text). Watermarked text scored 1.210 average perplexity while unwatermarked text scored 1.195 average perplexity.
- This approach can detect text generated by any model that implements the watermarking procedure. Attackers may be able to defeat it by paraphrasing generated text or by swapping in blacklisted words.
Classifier: Sandra Mitrovic, Davide Andreoletti, and Omran Ayoub at University of Southern Switzerland and University of Applied Sciences and Arts of Southern Switzerland trained a model to classify text generated by ChatGPT.
- The authors fine-tuned a pre-trained DistilBERT to classify text using human-written restaurant reviews, reviews generated by ChatGPT using prompts such as “please write me a 3-line review for a bad restaurant,” and ChatGPT paraphrases of human-written reviews.
- The trained classifier differentiated human-written from ChatGPT-generated reviews with 98 percent accuracy. It discerned ChatGPT paraphrases with 79 percent accuracy.
- Applying this approach on a broad scale would require training classifiers on different sorts of text and output from different text generators. Like other neural networks, the classifier is vulnerable to adversarial attacks in which small alterations to the input change the output classification.
Likelihood of generation: Eric Mitchell and colleagues at Stanford University developed DetectGPT, a method that detects generated text by relying on statistical differences between rewordings of machine-generated text and rewordings of human written text — no training data required.
- Language models tend to assign much higher likelihood to text they generate than to rewordings of it. In contrast, the authors found little difference in likelihood between human-generated text and machine-generated rewrites. Thus, a model’s assessment of the difference in likelihood between initial and reworded versions of text reveals whether or not the model generated it.
- The authors reworded text passages from a model and humans 100 times by masking 15 percent of the words and letting T5 fill in the blanks. Given an initial and reworded passage, the model calculated the difference in likelihood sentence by sentence. The text was deemed model-generated if the average drop in likelihood exceeded an empirically determined threshold.
- They used their method to detect the output of five text generators including GPT-3. They drew prompts and human examples from PubMedQA and other datasets. Their approach detected text generated by GPT-3 with .84 AUC (a measure of true versus false positives in which 1 is a perfect score).
- DetectGPT requires no additional models, datasets, or training and works on the output of any text generator. However, it requires access to the text generator’s output probabilities. Models like ChatGPT, BingChat, and YouChat that are available only via an API do not provide such access.
We’re thinking: Independent reporting on technology designed to detect generated text finds that it frequently delivers false positives, which can lead to unfair accusations of cheating, as well as false negatives. Watermarking can work from a technical perspective, but competitive pressure is likely to disincentivize AI providers to offer it. So, for now, at least, it seems as though we will have to adapt to the inability to distinguish between human- and machine-generated text.
Data Points
Research: Google released new details about its AI supercomputers
Google described the design and performance of its AI supercomputers used in over 90 percent of the company's AI training. The company claims that its supercomputers, which include up to 4,000 Tensor Processing Unit (TPU) chips, are up to 1.7 times faster and 1.9 times more power-efficient than systems based on Nvidia A100 chips. (Reuters)
New York prepares for the first AI Fashion Week
AI Fashion Week is set to launch later this month. The week will showcase collections from emerging designers. The event aims to promote AI as a tool for fashion design and support designers who work with this technology. (Vogue Business)
AI triage tool could speed up treatment for shrapnel injuries in Ukraine
Ukrainian scientists are using AI to analyze CT scans of shrapnel wounds. The team is using artificial mock-ups of wounds to train models to determine the material type, location, and urgency of removal. (New Scientist)
The success of large language models unsettles researchers in other areas of natural language processing
Models such as GPT-4 dominate so many subspecialties in natural language processing that researchers are concerned about the value of their work. Lack of public information about the precise nature of GPT-4’s technology intensifies the discomfort. (IEEE)
Research: Bloomberg launched a language model designed for finance
The data and media company described a large-scale generative AI model trained on financial language. The model, trained on a 700-billion token dataset, outperformed similar open models while maintaining competitive performance on general-purpose benchmarks. (Bloomberg)
Google to enhance its search with conversational AI
Google CEO Sundar Pichai announced plans to add conversational AI features to the search engine, which could change the traditional link-based experience. Google is testing several new search products that let users interact with its large language models. (The Wall Street Journal)
Bing chatbot gears up to show more ads
Microsoft announced that it will be introducing more ads to its Bing chatbot. While details are vague, the ads are set to appear within the chat experience and will be similar to those featured in traditional search results. Microsoft will share ad revenue with content owners. (The Verge)
Microsoft and Google take risks in the generative AI race
Despite internal concerns that their language models might generate falsehoods and exhibit other undesirable behavior, both tech giants are taking greater risks to build and release chatbots. (The New York Times)
Google provides coding tools from AI startup Replit
Developers on Google cloud will have access to Replit's suite of AI features for writing code, called Ghostwriter, while users of Replit will have access to Google’s cloud-computing infrastructure. The partnership aims to compete with Microsoft's GitHub and OpenAI by suggesting code blocks, completing programs, and answering developer questions. (Bloomberg)