Dear friends,
Here’s a quiz for you. Which company said this?
“It’s always been a challenge to create computers that can actually communicate with and operate at anything like the level of a human mind. . . . What we’re doing is creating here a system that will be able to be applied to all sorts of applications in the world and essentially cut the time to find answers to very difficult problems.”
How about this?
“These creative moments give us confidence that AI can be used as a positive multiplier for human ingenuity.”
These are not recent statements from generative AI companies working on large language models (LLMs) or image generation models! The first is from a 2011 IBM video that promotes the Watson system’s upcoming participation in the TV game show Jeopardy!. The second comes from Google DeepMind webpage about AlphaGo, which was released in 2015.
IBM’s and DeepMind’s work moved AI forward. But it also inspired some people’s imaginations to get ahead of them. Some supposed that the technologies behind Watson and AlphaGo represented stronger AI capabilities than they did. Similarly, recent progress on LLMs and image generation models has reignited speculation about artificial general intelligence (AGI).
Generative AI is very exciting! Nonetheless, today’s models are far from AGI. Here’s a reasonable definition of from Wikipedia:
“Artificial general intelligence (AGI) is the ability of an intelligent agent to understand or learn any intellectual task that human beings or other animals can.”
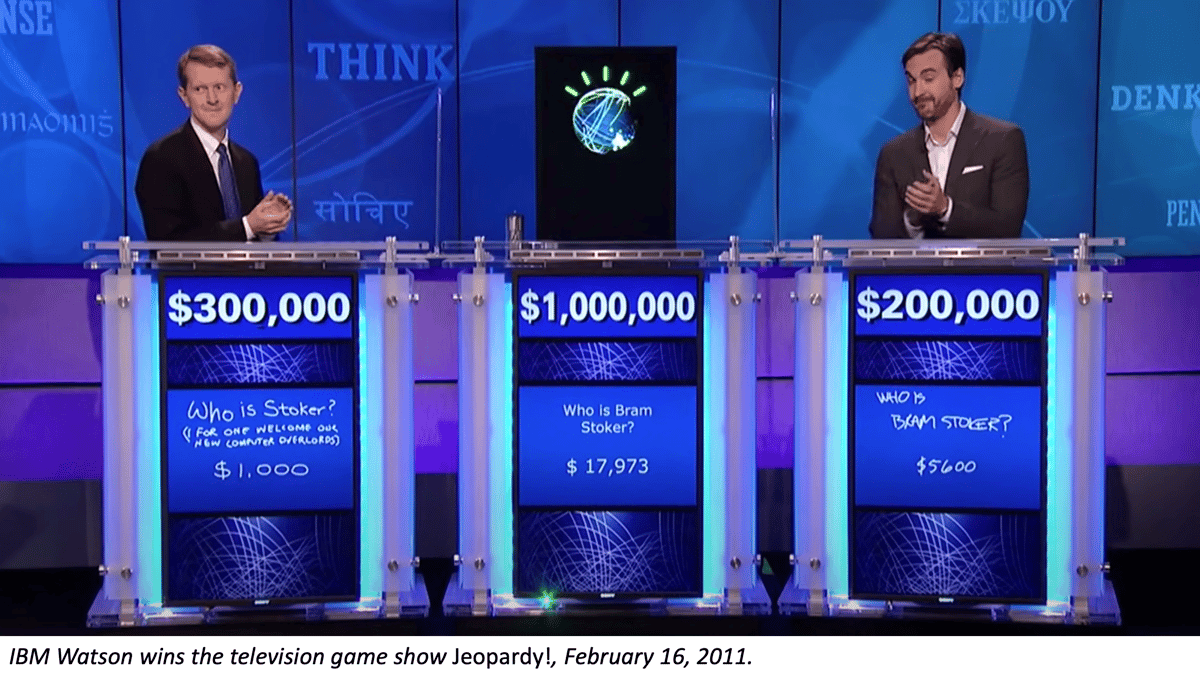
The latest LLMs exhibit some superhuman abilities, just as a calculator exhibits superhuman abilities in arithmetic. At the same time, there are many things that humans can learn that AI agents today are far from being able to learn.
If you want to chart a course toward AGI, I think the baby steps we’re making are very exciting. Even though LLMs are famous for shallow reasoning and making things up, researchers have improved their reasoning ability by prompting them through a chain of thought (draw one conclusion, use it to draw a more sophisticated conclusion, and so on).
To be clear, though, in the past year, I think we’ve made one year of wildly exciting progress in what might be a 50- or 100-year journey. Benchmarking against humans and animals doesn’t seem to be the most useful question to focus on at the moment, given that AI is simultaneously far from reaching this goal and also surpasses it in valuable ways. I’d rather focus on the exciting task of putting these technologies to work to solve important applications, while also addressing realistic risks of harm.
While AGI may be part of an indeterminate future, we have amazing capabilities today, and we can do many useful things with them. It will take great effort on all of our parts to to find ways to harness them to advance humanity. Let’s get to work on that.
Keep learning!
Andrew
News
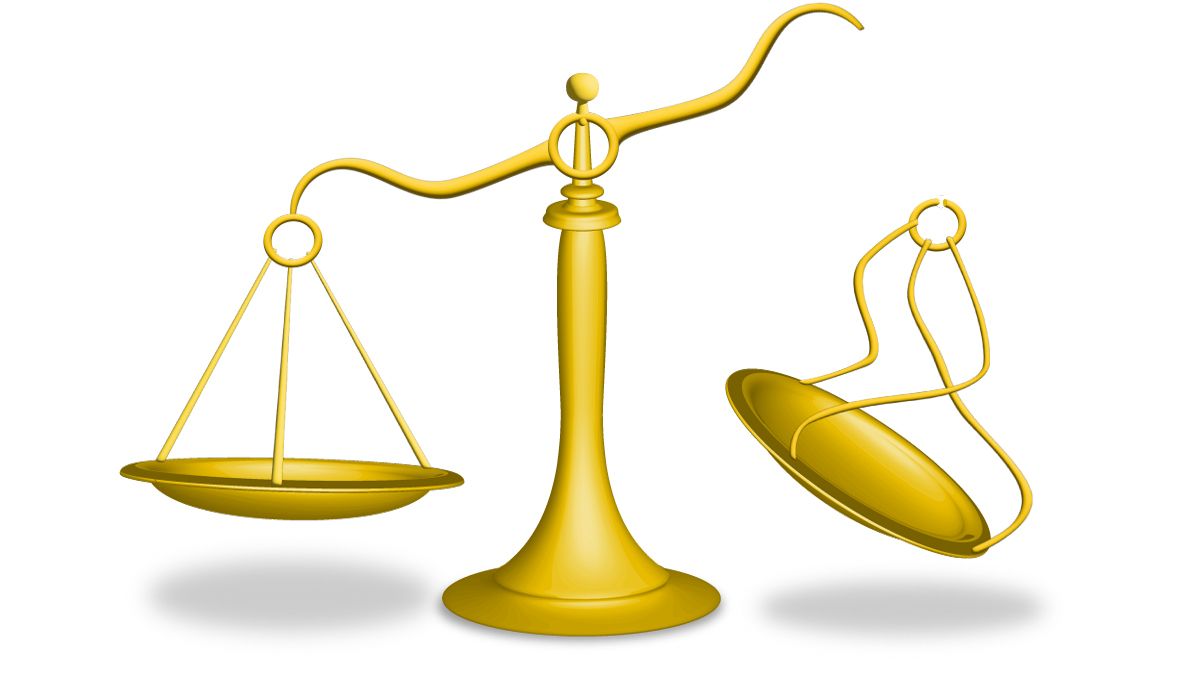
Microsoft Cuts Ethics Squad
Microsoft laid off an AI ethics team while charging ahead on products powered by OpenAI.
What’s new: On March 6, the tech giant dissolved the Ethics & Society unit in its Cognition group, which researches and builds AI services, amid ongoing cutbacks that have affected 10,000 workers to date, the tech-news outlet Platformer reported. Microsoft kept its Office of Responsible AI, which formulates ethical rules and principles, and related teams that advise senior leadership on responsible AI and help implement responsible AI tools in the cloud.
How it works: Ethics & Society was charged with ensuring that AI products and services were deployed according to Microsoft’s stated principles. At its 2020 peak, it included around 30 employees including engineers, designers, and philosophers. Some former members spoke with Platformer anonymously.
- As business priorities shifted toward pushing AI products into production, the company moved Ethics & Society staff to other teams, leaving seven members prior to the recent layoffs.
- Former team members said that the prior round of downsizing had made it difficult for them to do their jobs.
- They also said that other teams often would not listen to their feedback. For example, Ethics & Society warned that Bing Image Creator, a text-to-image generator based on OpenAI’s DALL·E 2, would harm the earning potential of human artists and result in negative press. Microsoft launched the model without having implemented proposed strategies to mitigate the risk.
Behind the news: Microsoft isn’t the only major AI player to have shifted its approach to AI governance.
- Earlier this month, OpenAI began providing access to GPT-4 without supplying information on its model architecture or dataset, a major departure from its founding ideal of openness. “In a few years, it’s going to be completely obvious to everyone that open-sourcing AI is just not wise,” OpenAI’s chief scientist Ilya Sutskever told The Verge.
- In early 2021, Google restructured its responsible AI efforts, placing software engineer Marian Croak at the helm. The shuffling followed the acrimonious departures of two prominent ethics researchers.
Why it matters: Responsible AI remains as important as ever. The current generative AI gold rush is boosting companies’ motivation to profit from the latest developments or, at least, stave off potential disruption. It also incentivizes AI developers to fast-track generative models into production.
We’re thinking: Ethical oversight is indispensable. At the same time, recent developments are creating massive value, and companies must balance the potential risks against potential benefits. Despite fears that opening models like Stable Diffusion would lead to irresponsible use — which, indeed, has occurred — to date, the benefits appear to be vastly greater than the harms.
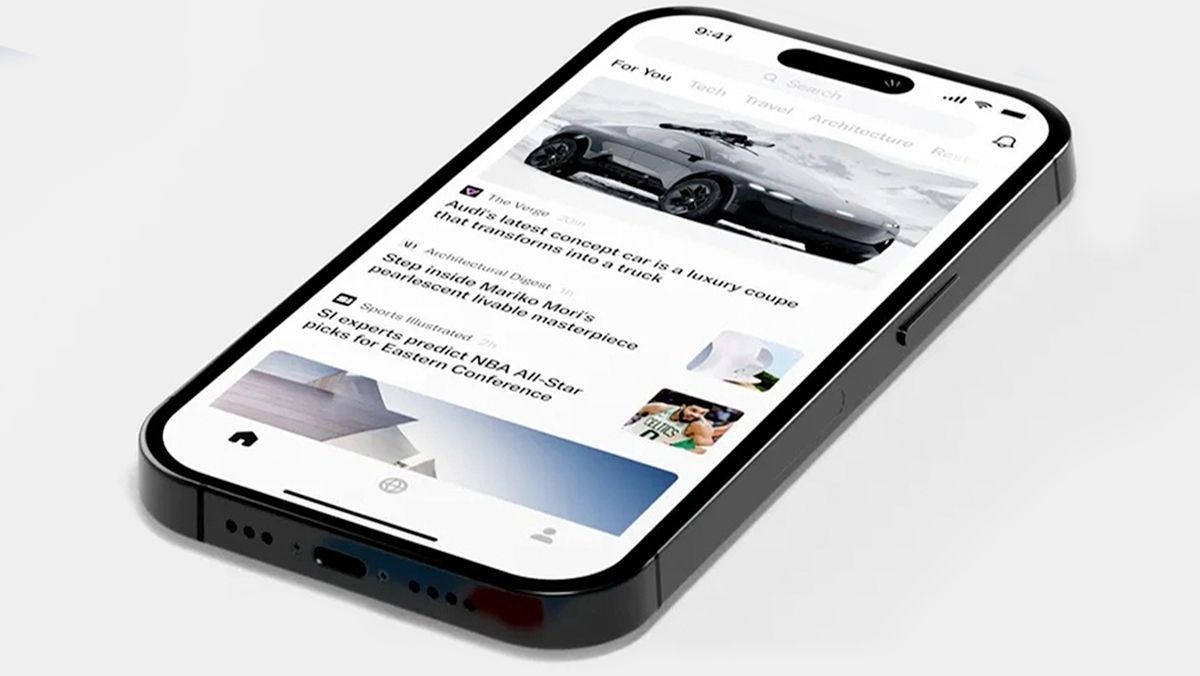
All the News That’s Fit to Learn
What does an entrepreneur do after co-founding one of the world’s top social networks? Apply the lessons learned to distributing hard news.
What’s new: Kevin Systerom and Mike Krieger, who co-founded Instagram, launched Artifact, an app that uses reinforcement learning to recommend news articles according to users’ shifting interests.
How it works: The founders were inspired to launch a news app after witnessing TikTok’s success at designing a recommendation algorithm that learned from users’ habits, Systrom told The Verge. The app starts by classifying each user as a persona that has a standardized constellation of interests, the founders explained to the tech analysis site Stratechery. Then a transformer-based model selects news articles; its choices are continually fine-tuned via reinforcement learning, TechCrunch reported.
- The model updates its recommendations based on factors that include how many users click through to an article, how much time they spend reading it, how often they share it externally, and how often they share it with friends within the app.
- The system randomly selects some stories that are unconnected to a user’s past history to keep the feed from becoming too homogenous.
- Human curators vet news sources, weeding out sources known to distribute disinformation, poor reporting, and clickbait. Users can add their own subscriptions manually.
Behind the news: Artifact joins a crowded field of personalized news feeds from Google, Apple, Japan-based SmartNews and China-based Toutiao (owned by TikTok’s parent ByteDance). NewsBreak of California focuses on local news.
Yes, but: Delivering news is a tough business. Never mind the precipitous decline of traditional newspapers. SmartNews announced it was laying off 40 percent of its staff.
Why it matters: Social media sites like Facebook grew partly on their promises to deliver timely news according to individual users’ interests, but they struggle to deliver high-quality news. A 2019 Pew Research Center poll found that 55 percent of U.S. adults thought social media companies’ role in curating consumption resulted in a worse mix of news. Artifact aims to apply machine learning techniques developed to help people stay in touch with friends to keep them informed in a rapidly changing world.
We’re thinking: Social media networks have used recommendation algorithms to maximize engagement, enabling clickbait and other low-quality information to flourish. Artifact’s choice of what to maximize, be it user engagement (which, in ad-driven social networks, correlates with revenue), metrics that track consumption of high-quality news, or something else, will have a huge impact on its future.
A MESSAGE FROM DEEPLEARNING.AI
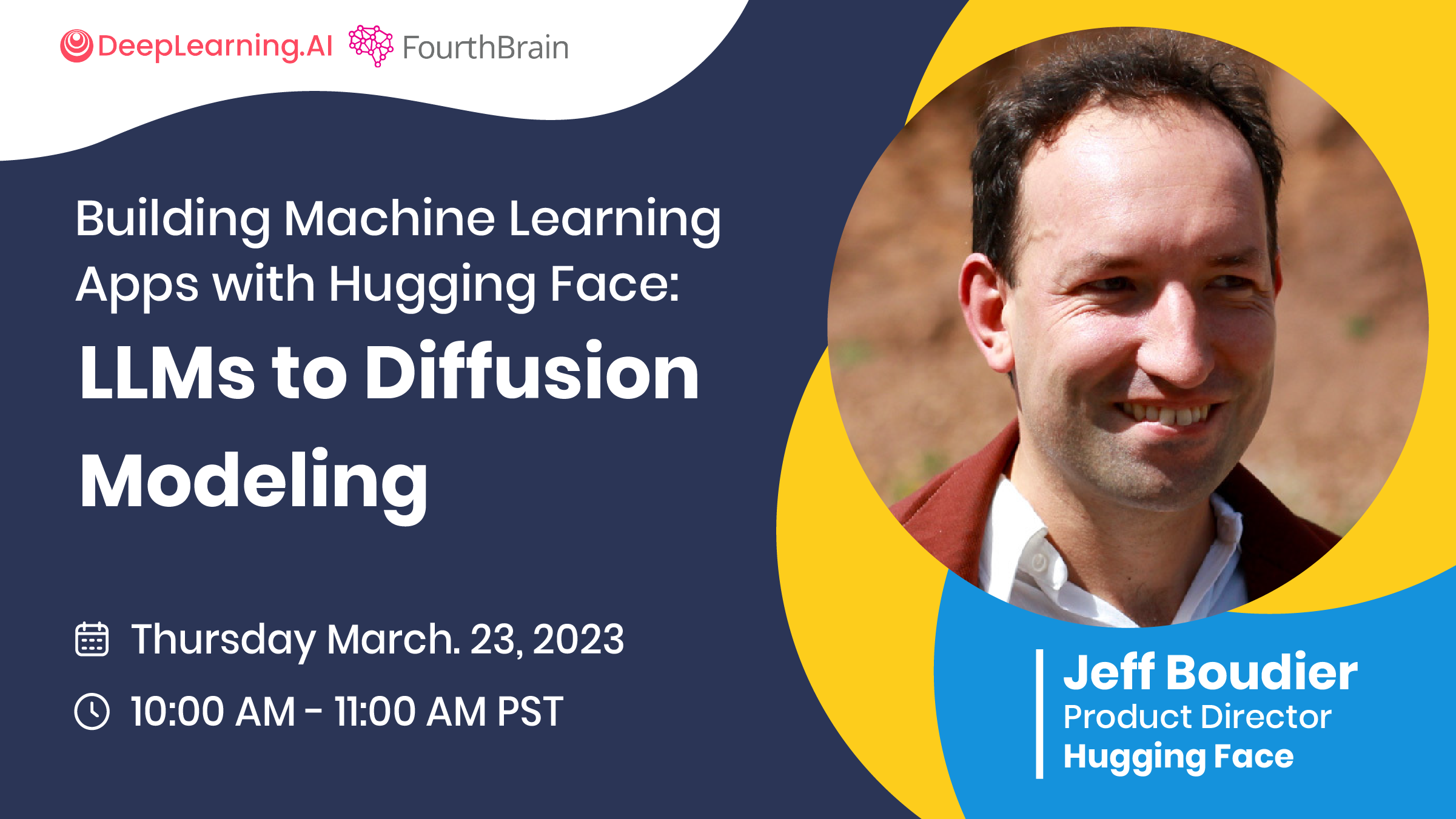
Are you interested in hands-on learning for natural language processing and machine learning for production? Join us on March 23, 2023, at 10:00 a.m. Pacific Time for a workshop in “Building Machine Learning Apps with Hugging Face: LLMs to Diffusion Modeling.” RSVP
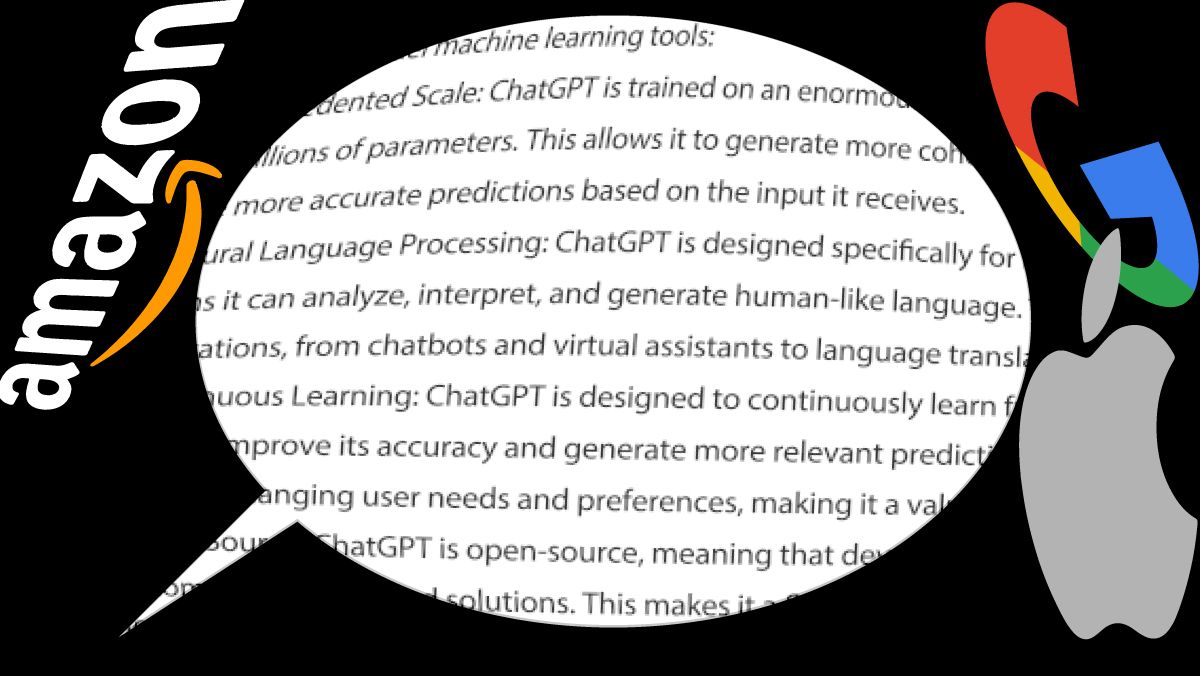
How AI Kingpins Lost the Chatbot War
Amazon, Apple, and Google have been building chatbots for years. So how did they let the alliance between Microsoft and OpenAI integrate the first smash-hit bot into Microsoft products?
What happened: Top AI companies brought their conversational agents to market over the past decade-plus amid great fanfare. But Amazon’s Alexa, Apple’s Siri, and Google’s Assistant succumbed to technical limitations and business miscalculations, The New York Times reported. Meanwhile, Microsoft launched, retooled, and ultimately killed its entry, Cortana, instead banking on a partnership with OpenAI, whose ChatGPT went on to become a viral sensation.
Amazon: Alexa hit the market in 2014. It garnered great enthusiasm as Amazon integrated it into a range of hardware like alarm clocks and kitchen appliances.
- Amazon tried to emulate Apple’s App Store, developing a skills library that customized Alexa to play simple games or perform tasks like controlling light switches. However, many users found the voice-assistant skills harder to use than mobile apps.
- Amazon had hoped that Alexa would drive ecommerce, but sales didn’t follow. The division that includes Alexa suffered billions of dollars in financial losses in 2022 and reportedly was deeply affected by the company’s recent layoffs.
Apple: Siri became a fixture in iPhones in 2011. It drove a spike in sales for a few years, but the novelty wore off as it became mired in technical complexity.
- Siri’s engineers designed the bot to answer questions by querying a colossal list of keywords in multiple languages. Each new feature added words and complexity to the list. Some required engineers to rebuild Siri’s database from scratch.
- The increasingly complex technology made for infrequent updates and made Siri an unsuitable platform for more versatile approaches like ChatGPT.
Google: Google debuted Assistant in 2016. It touted Assistant’s ability to answer questions by querying its search engine. Meanwhile, it pioneered the transformer architecture and built a series of ever more-capable language models.
- Like Amazon with Alexa skills, Google put substantial resources into building a library of Assistant actions, but the gambit didn’t pay off. A former Google manager said that most users requested tasks like switching lights or playing music rather than web searches that would generate revenue.
- In late 2022, Google reduced its investment in Assistant. The company’s recent layoffs affected 16 percent of Assistant’s division.
- Google debuted the transformer in 2017 and used it to build the Meena language model in 2020. The Meena team encouraged Google to build the model into Assistant, but the executives — sensitive to criticism after having fired two prominent researchers in AI ethics — objected, saying that Meena didn’t meet the company’s standards for safety and fairness, The Wall Street Journal reported.
- On Tuesday, the company started to allow limited access to Bard, a chatbot based on Meena’s successor LaMDA. (You can sign up here.) Last week, it previewed LaMDA-based text generation in Gmail and Google Docs. These moves followed Google CEO Sundar Pichai’s December “code red” directive to counter Microsoft by focusing on generative AI products.
Why it matters: The top AI companies devoted a great deal of time and money to developing mass-market conversational technology, yet Microsoft got a jump on them by providing cutting-edge language models — however flawed or worrisome— to the public.
We’re thinking: Microsoft’s chatbot success appears to be a classic case of disruptive innovation: An upstart, OpenAI, delivered a product that, although rivals considered it substandard, exceeded their products in important respects. But the race to deliver an ideal language model isn’t over. Expect more surprise upsets to come!
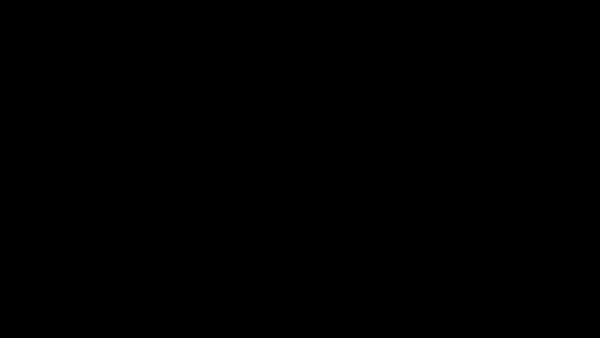
Real-World Training on the Double
Roboticists often train their machines in simulation, where the controller model can learn from millions of hours of experience. A new method trained robots in the real world in 20 minutes.
What's new: Laura Smith, Ilya Kostrikov, and Sergey Levine at UC Berkeley introduced a process to rapidly train a quadruped robot to walk in a variety of real-world terrains and settings.
Key insight: One way to train a model on less data is to train it repeatedly on the same examples (in this case, the robot's orientation, velocity, and joint angles at specific points in time). However, this may lead the model to overfit (for instance, the robot may learn to walk effectively only on the terrain used in training). Regularization or normalization enables a model to train multiple times on the same examples without overfitting.
How it works: The authors trained a motion-planning model to move a Unitree A1 robot forward on a given terrain using an actor-critic algorithm, a reinforcement-learning method in which an actor function learns to take actions that maximize the total return (roughly the sum of all rewards) estimated by a critic function. The actor was a vanilla neural network and the critic was an ensemble of such networks.
- The actor, given the robot’s current orientation, angular and linear velocity, joint angles, joint velocities, which feet were touching the ground, and the previous action, generated target joint angles.
- The critic encouraged the actor to move the robot forward within a range of speed defined by the authors. It also discouraged the actor from turning sideways.
- After each movement, the critic learned to estimate the expected future reward by minimizing the difference between its expected future reward before the movement and the sum of the actual reward and the expected future reward after the movement.
- The actor-critic algorithm updated the actor’s likelihood of making a particular move based on the size of the critic’s estimated reward.
- The authors applied layer normalization to the critic, enabling it to update 20 times per movement without overfitting. They updated the actor once per movement.
Results: The authors trained the model to walk the robot on each of five surfaces (starting from scratch for each surface): flat ground, mulch, lawn, a hiking trail, and a memory foam mattress. The robot learned to walk on each in about 20 minutes, which is roughly equivalent to 20,000 examples. Competing methods use either simulation or more time in the real world. For example, the authors of DayDreamer: World Models for Physical Robot Learning trained the same type of robot to walk on an indoor surface without a simulation, but it took one hour and 3.6 times more examples.
Why it matters: Training on simple features (those with a small number of dimensions, such as robot orientation and velocity) rather than complex features (such as images) reduces the number of examples required to learn a task, and regularizing the model prevents overfitting. This is a simple, general setup to train reinforcement learning models in the real world.
We're thinking: Reinforcement learning algorithms are famously data-hungry, which is why much of the progress in the past decade was made in simulated environments. A recipe for training a quadruped rapidly in the real world is a great step forward!
Data Points
New York City to hire an expert in AI and machine learning
The city’s Office of Technology is seeking an expert to identify AI use cases and develop ethical guidelines and best practices for AI applications in the public sector. (Bloomberg)
Life insurance algorithms face scrutiny for possible bias
Colorado’s Division of Insurance is crafting new regulations to limit insurers’ use of predictive models and algorithms. (The Wall Street Journal)
Research: Meta’s AI-powered tool predicts the structure of proteins faster
The program, called ESMFold, is expected to help researchers find new drugs and cures for diseases. ESMFold purportedly is 60 times faster than Google’s AlphaFold, but less accurate. (The Wall Street Journal)
Salesforce launched EinsteinGPT
The cloud-software company partnered with OpenAI to integrate text-generation services across their sales, commerce, and marketing tools. (Fast Company)
A consortium of AI research groups released an open source version of ChatGPT
OpenChatKit is a 20 billion-parameter open source base to develop generalized and specialized chatbots. You can try the demo here. (Together)
Anthropic, a company founded by former OpenAI employees, launched its own chatbot
The chatbot called Claude has more natural and coherent conversations than existing chatbots, according to the company. (The Verge)
Students made deepfake videos of a principal making racist remarks
Three high school students shared the material on Tik Tok, sparking outrage within the Putnam County, New York, community. (Vice)
Chinese media outlet is broadcasting an AI news anchor
The AI-powered journalist belongs to China’s state-owned news outlet People Daily and can report news 24/7. (PetaPixel)