Dear friends,
As you can read in this issue of The Batch, generative AI companies are being sued over their use of data (specifically images and code) scraped from the web to train their models. Once trained, such models can generate, on demand, images in a given artist’s style or code that executes particular tasks.
The lawsuits will answer the question of whether using publicly available data to train generative models is legal, but I see an even more important question: Is it fair? If society has a point of view on what is fair, we can work to make laws that reflect this.
To be clear, this issue is much bigger than generative AI. The fundamental question is whether AI systems should be allowed to learn from data that’s freely available to anyone with an internet connection. But the focus right now is on models that generate images and code.
Today, we routinely advise students of computer programming to read — and perhaps contribute to — open source code. Reading open source no doubt inspires individuals to write better code. No one questions whether this is fair. After all, it’s how people learn. Is it fair for a computer to do the same?
The last time I visited the Getty Museum in Los Angeles, California, I saw aspiring artists sitting on the floor and copying masterpieces on their own canvases. Copying the masters is an accepted part of learning to be an artist. By copying many paintings, students develop their own style. Artists also routinely look at other works for inspiration. Even the masters whose works are studied today learned from their predecessors. Is it fair for an AI system, similarly, to learn from paintings created by humans?
Of course, there are important differences between human learning and machine learning that bear on fairness. A machine learning model can read far more code and study far more images than a human can. It can also generate far more code or images, far more quickly and cheaply, than even the most skilled human.
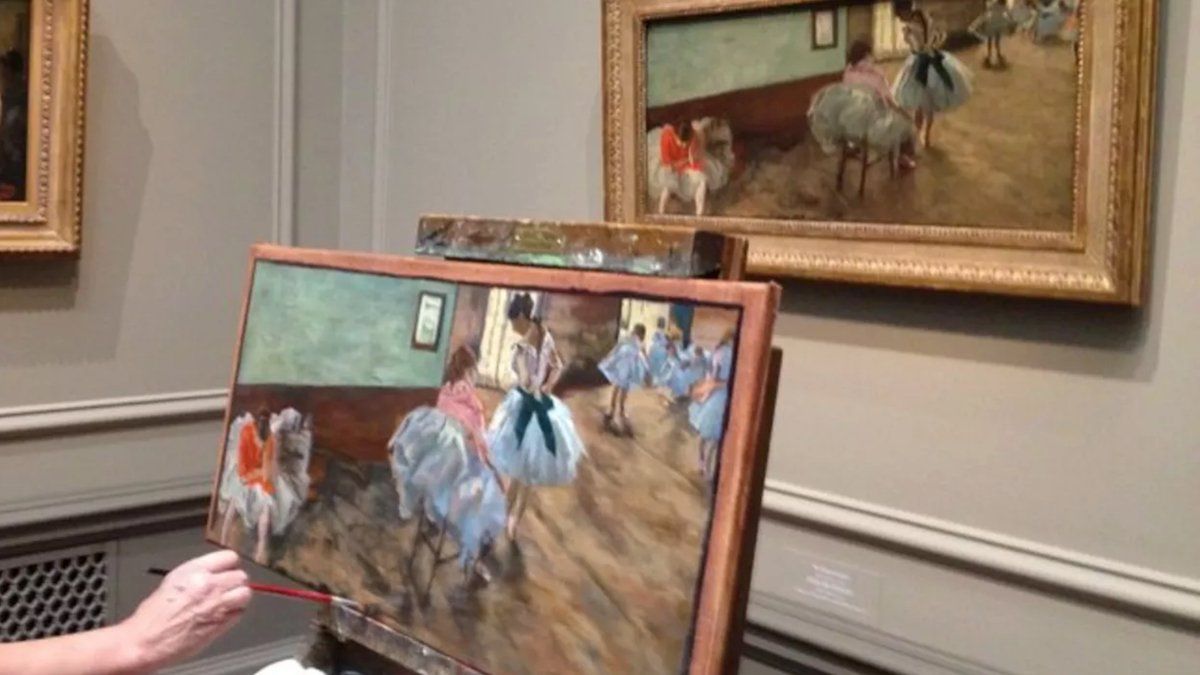
These differences raise serious issues for artists, coders, and society at large:
- Production of creative works by a machine may devalue the work of human creators.
- Generative models can reproduce the personal style of artists whose work they were trained on without compensating those artists.
- Such models may have been trained on proprietary data that was not intended to be available on the internet (such as private images that were stolen or leaked).
On the other hand, generative models have tremendous potential value. They’re helping people who are not skilled artists to create beautiful works, spurring artists to collaborate with computers in new ways, and automating workaday tasks so humans can focus on higher-level creativity. Furthermore, advances in AI build upon one another, and progress in generative AI brings progress in other areas as well.
The upshot is that we need to make difficult tradeoffs between enabling technological progress and respecting the desire to protect creators’ livelihoods. Thoughtful regulation can play an important role. One can imagine potential regulatory frameworks such as:
- Establishing a consistent way for creators to opt out
- Mandating compensation for artists when AI systems use their data
- Allocating public funding to artists (like using tax dollars to fund public media such as the BBC)
- Setting a time limit, like copyright, after which creative works are available for AI training
What a society views as fair can change. In the United States, once it was considered fair that only certain men could vote. When society’s view on this changed, we changed the rules.
Society currently has divergent views on what is fair for AI to do. Given the bounty offered by generative AI (and other AI systems), and acknowledging the need to make sure that creators are treated fairly, I hope we find a path forward that allows AI to continue to develop quickly for the benefit of all.
Keep learning!
Andrew
News
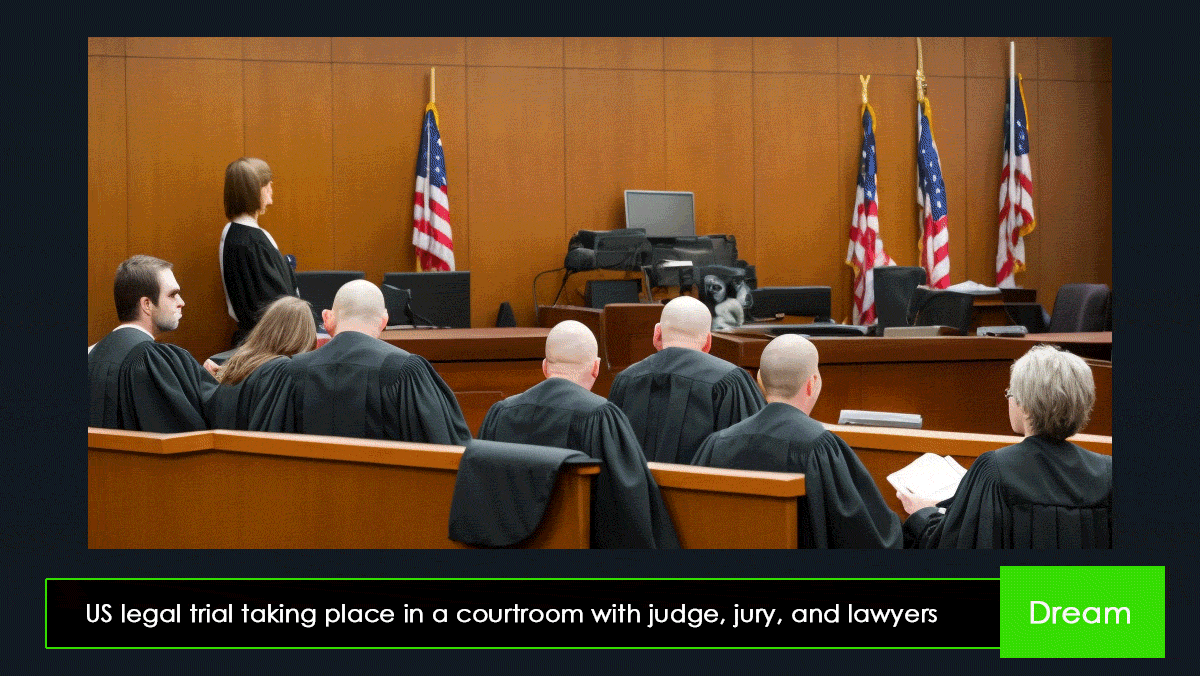
Generative AI on Trial
Models that generate text and images are raising thorny questions about the ownership of both their training data and their output.
What’s new: The companies that provide popular tools for generating text and images are fighting a barrage of lawsuits. TechCrunch surveyed the docket.
Legal actions: Three lawsuits are in progress:
- A group of artists filed a class-action lawsuit in a United States court against Stability AI and Midjourney, companies that provide image generators, and DeviantArt, an online community that hosts its own image generator. The lawsuit claims that the models’ ability to generate work “in the style of” a given artist infringes artists’ intellectual property rights and harms them financially.
- In a separate action, writer, programmer, and lawyer Matthew Butterick brought a class-action claim against Microsoft, OpenAI, and GitHub in a U.S. court. The plaintiff alleges that Copilot, a model that generates computer code, outputs open-source code without properly crediting its creators. Butterick is represented by the same lawyers who represent the artists who sued Stability AI, Midjourney, and DeviantArt.
- Getty Images announced its intent to sue Stability AI in a British court for using images scraped from Getty’s collection to train its models.
Defense measures: Companies are taking steps to protect themselves from legal risk.
- OpenAI asserted in a court filing that its use of open source code to train Copilot is protected by the U.S. doctrine of fair use, which allows limited reproduction of copyrighted materials for commentary, criticism, news reporting, and scholarly reports. Stability has claimed the same in the press. In 2015, a U.S. court ruled Google’s effort to digitally scan books was fair use.
- Stability AI plans to allow artists to opt out of inclusion in the dataset used to train the next version of Stable Diffusion.
- Github added a filter to Copilot that checks the program’s output against Github’s public code repository and hides output that’s too similar to existing code.
Why it matters: Companies that aim to capitalize on AI’s ability to generate text, images, code, and more raised tens of millions of dollars in 2022. Much of that value could evaporate if courts decide they must compensate sources of training data or scrap models trained using data that was obtained inappropriately.
We’re thinking: Laws that protect intellectual property haven’t yet caught up with AI. Without legal clarity, engineers have less freedom to innovate, and investors have less certainty about which approaches to support.
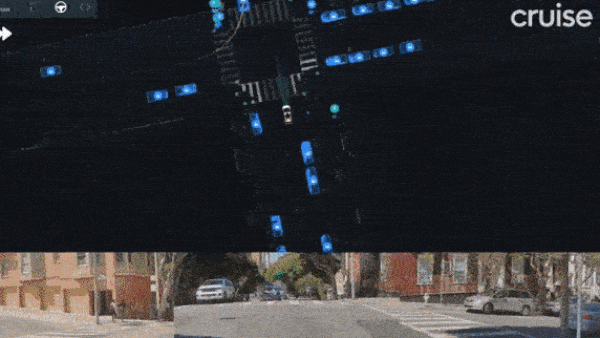
Robotaxis Face Headwinds
San Francisco officials are pushing back on self-driving taxis in the city after a deluge of public complaints.
What's new: In an open letter, the San Francisco Municipal Transportation Agency, the county Transportation Authority, and the mayor’s Office on Disability urged California officials to maintain current restrictions on self-driving cars until the operators meet certain conditions.
Pump the brakes: Cruise and Waymo are allowed to operate robotaxis in San Francisco only within limited areas and times of day. In December 2022, Cruise asked the California Public Utilities Commission to expand its range and hours of operation. In a letter rebutting the request, officials cited 92 incidents in which vehicles from Cruise or Waymo reportedly made unplanned stops between May 29 and December 31, 2022, disrupting other cars, public transportation, and bicycles. The authors recommended that the state maintain current restrictions until the operators meet certain conditions:
- Operators would be required to observe current restrictions until they demonstrate that they can operate without disrupting traffic for several months.
- They would be allowed to expand their fleets only incrementally (for instance, 100 vehicles at a time) to ensure that they’re able to scale without compromising safety or operations.
- They would be required to provide data that enables officials to evaluate the impact of unplanned stops, including the number of miles traveled per vehicle, the number of unplanned stops, and their durations.
- This data would be available to the public. (Cruise currently shares limited data with the city and requires confidentiality.)
- The public would have at least 30 days to review the data and respond before the city allows an operator to expand its range or schedule.
Rearview mirror: Cruise and Waymo began operating robotaxis without safety drivers in San Francisco in 2020 and 2022 respectively. The city granted them permission to charge fares in 2022. Subsequently, Cruise vehicles clogged roads after losing their connections with the company’s servers in several incidents.
Why it matters: Self-driving cars must share the streets safely and smoothly with other forms of traffic. The reports indicate that erratic behavior by autonomous vehicles could seriously disrupt not only conventional cars but also cyclists and public transit — groups that account for nearly half of all travelers.
We're thinking: We welcome calls for greater transparency around self-driving cars. Government reports on their performance tend to leave it unclear how reliable vehicles from different providers are. Transparency is essential to developing an appropriate framework for making them part of daily life.
A MESSAGE FROM DEEPLEARNING.AI
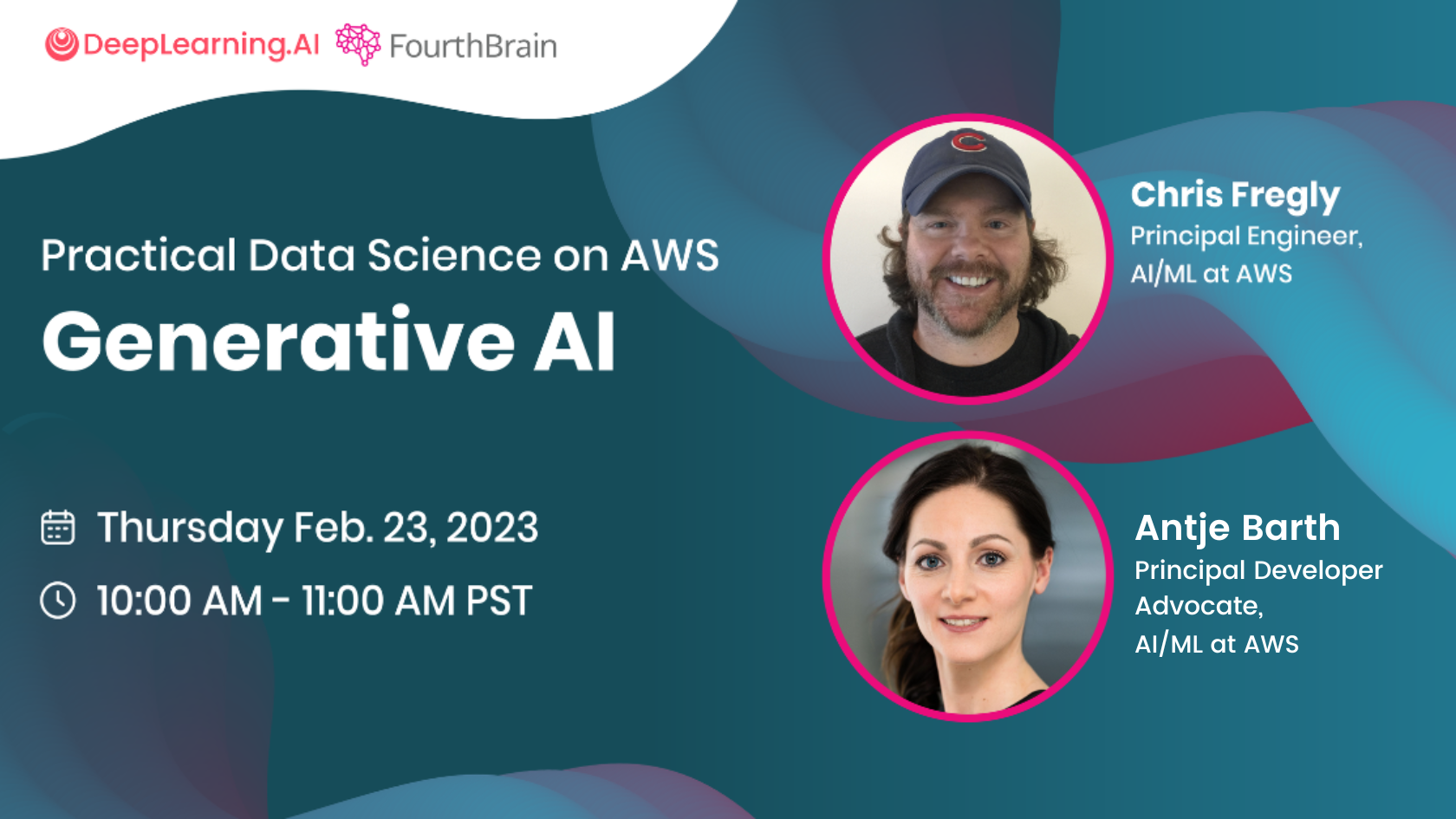
Join us for our first live workshop of the year! Learn how Amazon's CodeWhisperer generates Python and SageMaker generates images using Stable Diffusion in Practical Data Science on AWS: Generative AI. See you on Thursday, February 23, 2023, at 10:00 a.m. Pacific Time. RSVP
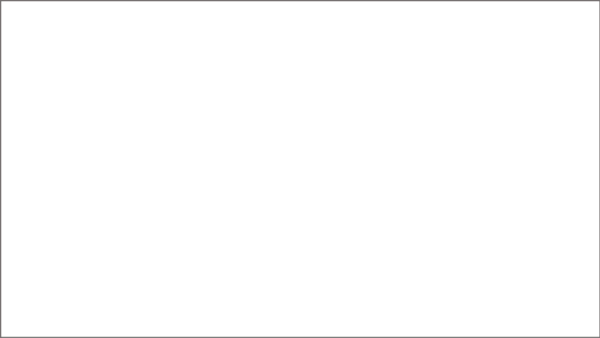
He Who Types the Prompt Calls the Tune
As AI-generated text and images capture the world’s attention, music is catching up.
What’s new: Andrea Agostinelli, Timo I. Denk, and colleagues at Google and Sorbonne Université introduced MusicLM, a system that generates music from text descriptions. You can hear its output here.
Key insight: Paired natural-language descriptions of music and corresponding music recordings are relatively scarce. How, then, to train a text-to-music generator? Previous work trained a model to map corresponding text and music to the same embedding. This makes it possible to train a system to regenerate music from a large corpus of recordings and then, at inference, prompt it with text.
How it works: MusicLM learned to regenerate audio clips (30 seconds at 24kHz resolution) from an undisclosed corpus that comprised 280,000 hours of recorded music. The challenge involved modeling sound in three distinct aspects: the correspondence between words and music; large-scale composition, such as a spare introduction that repeats with an added melody; and small-scale details, such as the attack and decay of a single drum beat. The team represented each aspect using a different type of token, each generated by a different pretrained system.
- Given an audio clip, MuLan (a transformer-based system) generated 12 audio-text tokens designed to represent both music and corresponding descriptions. It was pretrained on soundtracks of 44 million online music videos and their text descriptions to embed corresponding music and text to the same representation.
- Given the same audio clip, w2v-BERT generated 25 semantic tokens per second that represented large-scale composition. It was pretrained to generate masked tokens in speech and fine-tuned on 8,200 hours of music.
- Given the same audio clip, the encoder component of a SoundStream autoencoder generated 600 acoustic tokens per second, capturing small-scale details. It was pretrained to reconstruct music and speech and fine-tuned on 8,200 hours of music.
- Given the audio-text tokens, a series of transformers learned to generate semantic tokens.
- Given the semantic and audio-text tokens, a second series of transformers learned to generate acoustic tokens.
- At inference, MuLan generated audio-text tokens from an input description instead of  input music. Given the tokens from the second series of transformers, the SoundStream decoder generated a music clip.
Results: The authors fed 1,000 text descriptions from a text-music dataset (released with the paper) to MusicLM and two other recent text-to-music models, Riffusion and Mubert. Listeners judged which clip — including the music in the dataset, which was produced by professional musicians — best matched a given caption. They judged MusicLM to have created the best match 30.0 percent of the time, Riffusion 15.2 percent of the time, and Mubert 9.3 percent of the time. They judged the ground-truth, human-created music to be the best fit 45.4 percent of the time.
Yes, but: The listeners didn’t evaluate the generated clips based on how musically satisfying they were, just how well they matched the corresponding text.
Why it matters: Rather than relying on a single embedding, the authors combined three embeddings that represent an audio clip with increasing degrees of specificity. This approach, which is analogous to a human writer’s tendency to start with a concept, sketch an outline, and fill in the words, may be useful in other applications that require a computer to generate detailed, dynamic, long-form output.
We’re thinking: MusicLM’s output sounds more coherent than that of previous music generators, but it’s hard to judge musical values that unfold over time from brief clips. That said, its shows an impressive ability to interpret the diverse emotional language found in descriptions of painter Jacques-Louis David’s triumphant “Napoleon Crossing the Alps” and Edvard Munch’s harrowing “The Scream.”
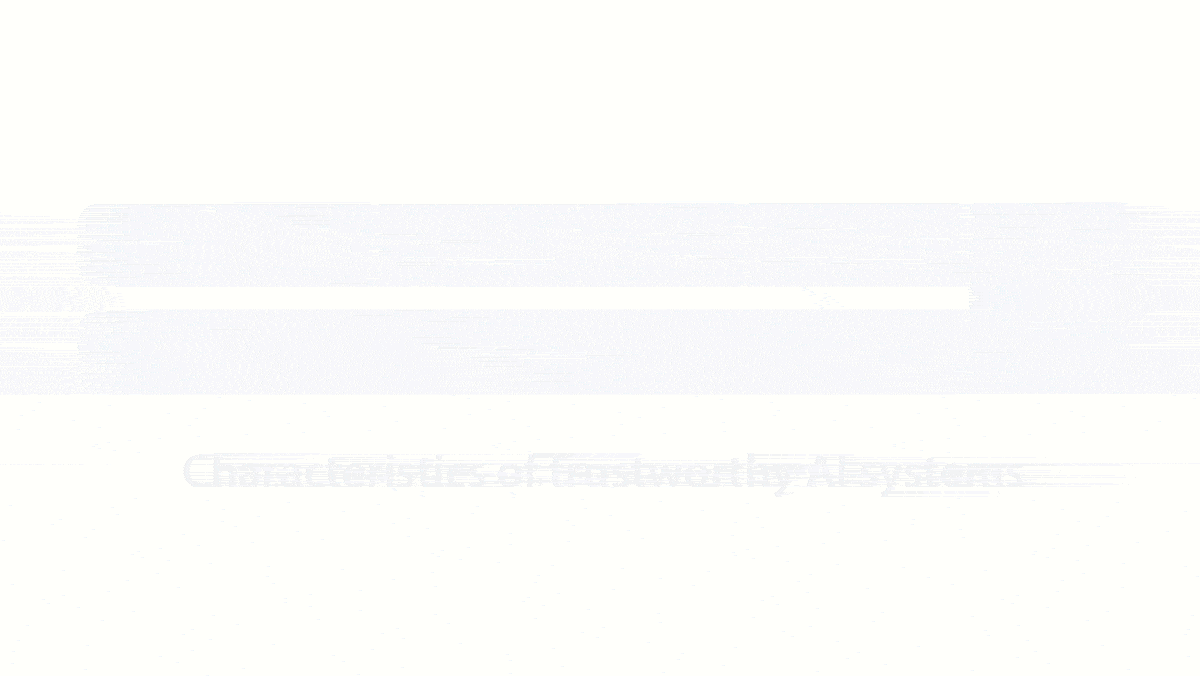
Guidelines for Managing AI Risk
The United States government published guidelines designed to help organizations limit harm from AI.
What's new: The National Institute for Standards and Technology, which recommends technological standards in a variety of industries, released the initial version of its AI Risk Management Framework.
What it says: The framework outlines principles for defining classes of potential harm, building trustworthy systems, and defending against AI-related risks as they emerge.
- Broad categories of AI-related risk include harm to people (by, say, causing medical distress or undermining civil liberties), harm to organizations (such as security breach or financial loss), and harm to ecosystems (both natural and artificial; for example, global financial networks).
- Trustworthy AI systems are validated, privacy-enhanced, secure, explainable, fair, and accountable. Validated AI systems are accurate, reliable, and generalized to data and settings beyond their training. Privacy-enhanced systems protect the anonymity and confidentiality of people and their data.
- Organizations can manage emerging capabilities by mapping risks that arise from a system’s intended uses, measuring risks, handling risks based on their projected impact, and, above all, cultivating a culture of transparency around mitigating risk.
- NIST plans to evaluate the framework on an ongoing basis and will release an update in a few months.
Behind the news: NIST’s framework, created in response to a 2021 order from Congress, incorporates feedback from over 240 organizations. It’s backed by corporations including IBM and Microsoft, lobbyists such as the U.S. Chamber of Commerce, nonprofits like the National Science Foundation, and think tanks like the Future of Life Institute.
Why it matters: A 2019 paper counted 84 efforts to codify best practices for managing AI risks. NIST’s effort marks a step away from this jigsaw-puzzle approach and toward guidelines that have broad support and thus are more likely to be implemented.
We're thinking: A framework like this is necessarily general, and different organizations will implement it very differently. For example, reliability in healthcare is very different from reliability in an app that customizes selfies, leading to different approaches to monitoring AI systems. It will take disciplined effort to translate these high-level ideas into specific practices — but it’s likely to head off tremendous trouble down the line.
Data Points
Students weighed in on the use of ChatGPT in education
High schoolers gave their opinions on the AI-powered chatbot that has educators divided. (The New York Times)
Economic uncertainty is giving AI a boost in the corporate world
Companies are increasing their use of AI and automation tools in pursuit of cost-effective growth strategies. (The Wall Street Journal)
Microsoft plans to position itself at the forefront of AI technology with the help of ChatGPT
The tech giant is looking to turn OpenAI’s technology into a big business. (The Wall Street Journal)
An AI art generator was credited as a co-author on a book
Zarya of the Dawn became the first comic book illustrated by AI-generated images to obtain copyright, but this milestone is sparking a debate over ownership. (The Wall Street Journal)
Baidu plans to release a competitor to ChatGPT is planned to be released in March
The Chinese tech company is developing a new AI-powered chatbot to bolster China’s leadership in emerging technologies. (The Wall Street Journal)
Fact-checking organizations are using AI to stop misinformation
Disinformation produced by AI spreads too fast for human fact-checkers to keep up, but the technology may help uphold the truth. (Wired)
China faces new restrictions on the export of chip manufacturing technology
New export controls from Japan and The Netherlands aim to prevent China from shoring up its military and AI capabilities. (The Verge)
More startups are jumping on the AI bandwagon to persuade investors and clients
Companies are mentioning AI in their press releases and pitch decks. They aim to generate more interest from venture firms and customers, but it may not be that simple. (Bloomberg)
Architectures are using AI art to evoke their design ambitions
Midjourney, a text-to-image model, is helping architects produce photorealistic renderings of proposed buildings and structures. (Bloomberg)
New Netflix film contains AI-generated artwork
Citing a labor shortage in the anime industry, the streaming-video company announced that an image generator produced background images for Dog and Boy, a three-minute animated film. (Ars Technica)
OpenAI, the company behind ChatGPT, launched a tool to detect AI-generated writing, but it’s far from perfect
The independent AI lab recently released a classifier to help educational institutions identify AI-generated submissions by students. However, the company warned that it’s not reliable yet. (The Wall Street Journal)