Dear friends,
Recent successes with large language models have brought to the surface a long-running debate within the AI community: What kinds of information do learning algorithms need in order to gain intelligence?
The vast majority of human experience is not based on language. The taste of food, the beauty of a sunrise, the touch of a loved one — such experiences are independent of language. But large language models have shown that it’s possible to capture a surprisingly rich facsimile of human experiences by consuming far more language than any human can in a lifetime.
Prior to recent advances in large language models, much of the AI community had viewed text as a very limited source of information for developing general-purpose intelligence. After all, animals evolved intelligence without language. Intelligence includes perceiving the world through sight, sound, and other senses; knowing how to move our bodies; having a common-sense understanding of physics, such as how to knock a fruit off a high tree; and being able to plan simple actions to find food, shelter, or a mate. Writing is a relatively recent invention that dates back only around 5,500 years. Spoken language arose roughly 100,000 years ago. In contrast, mammals have been around for around 200 million years.
If AI development were to follow the path of evolution, we would start by trying to build insect-level intelligence, then mouse-level intelligence, perhaps followed by dog-level, monkey-level, and finally human-level. We would focus on tasks like vision and psychomotor skills long before the ability to use language.
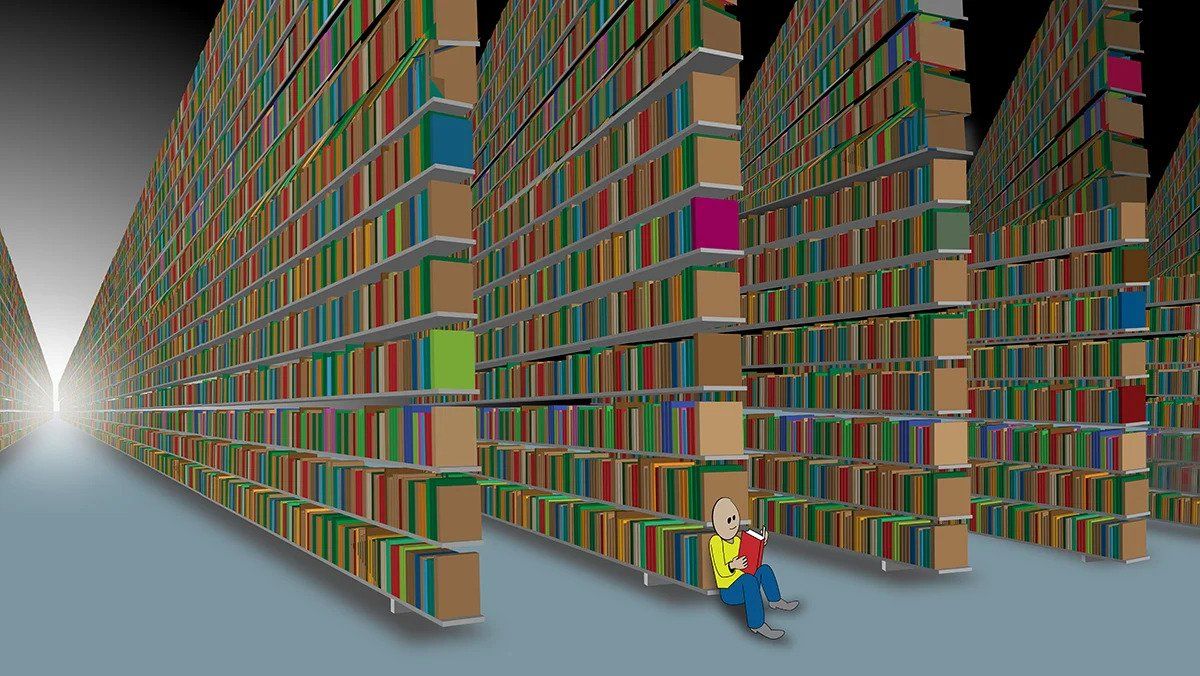
But models like ChatGPT show that language, when accessed at massive scale, overcomes many of its limitations as a source of information. Large language models can learn from more words — several orders of magnitude more! — than any individual human can.
- In a typical year, a child might hear around 10 million words (with huge variance depending on factors such as the family). So, by age 10, the child might have heard 100 million words.
- If you read 24/7 for a year at a rate of 250 words per minute, you’d read about 130 million words annually.
- GPT-3 was trained on about 500,000 million words.
An individual human would need dozens of lifetimes spent doing nothing but reading to see the number of words that GPT-3 considered during its training. But the web aggregates text written for or by billions of individuals, and computers have ready access to much of it. Through this data, large language models (LLMs) capture a wealth of knowledge about the human experience. Even though an LLM has never seen a sunrise, it has read enough text about sunrises to describe persuasively what one looks like.
So, even though language is a small part of human experience, LLMs are able to learn a huge amount of information about the world. It goes to show that there are multiple paths to building intelligence, and that the path followed by evolution or human children may not be the most efficient way for an engineered system.
Seeing the entire world only through the lens of text — as rich as it turns out to be, and as valuable as systems trained on text have become — is still ultimately an impoverished world compared to the one we live in. But relying on text alone has already taken us quite far, and I expect this direction to lead to exciting progress for years to come.
Keep learning!
Andrew
DeepLearning.ai Exclusive
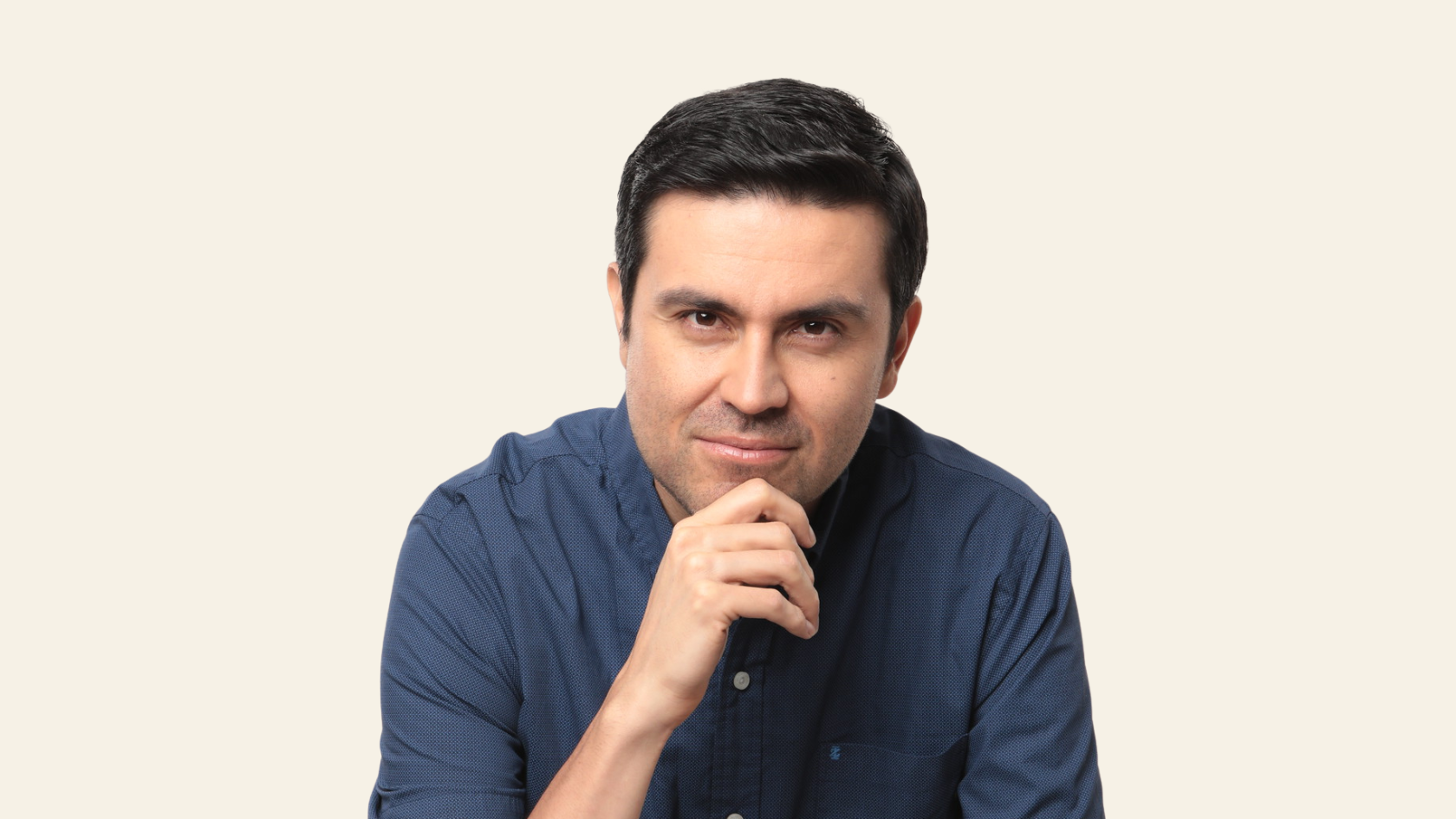
Meet Your New Math Instructor
The right teacher can make even the most intimidating subject easy. Luis Serrano knows that first-hand: He struggled with math until he started connecting concepts with real-world examples. Learn why he was the perfect person to teach the all-new Mathematics for Machine Learning and Data Science Specialization. Read more
News
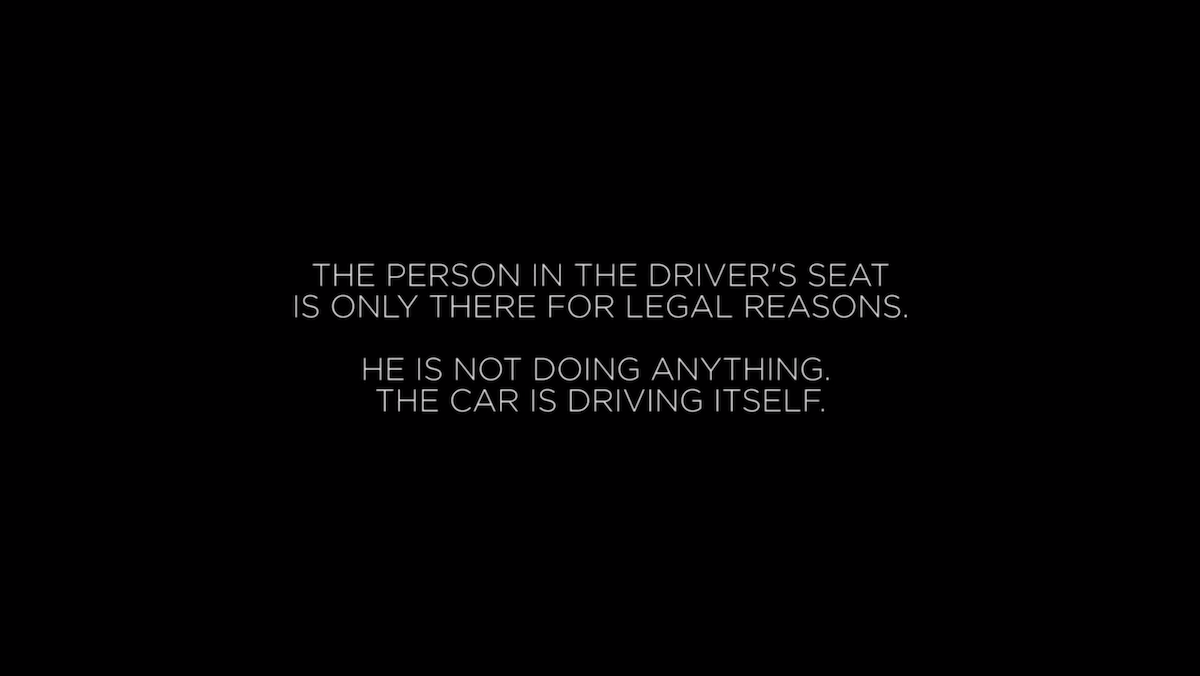
Self-Driving Deception
Tesla, whose autonomous-vehicle technology has been implicated in a number of collisions, promoted it in a way that apparently was intended to deceive.
What's new: Tesla deliberately misled the public about its vehicles’ ability to drive themselves, according to Bloomberg and other news outlets.
Human in the loop: In 2016, Tesla shared a video that showed a car traveling from a household driveway to an office parking lot. Onscreen text read, “The person in the driver’s seat is only there for legal reasons. He is not doing anything. The car is driving itself.”
- Tesla CEO Elon Musk pushed engineers to falsify the video, according to internal emails obtained by Bloomberg. He said he would inform viewers that the video showed future, not current, capabilities. Instead, when the company published the video, Musk tweeted, “Tesla drives itself (no human input at all) thru urban streets to highway to streets, then finds a parking spot.”
- Testifying in a lawsuit over the fatal crash of a Tesla vehicle in 2018, the company’s head of Autopilot software said the video was partially staged, Reuters reported. “The intent of the video was not to accurately portray what was available for customers in 2016. It was to portray what was possible to build into the system,” he told the court.
- The New York Times described the making of the same video in late 2021, noting that engineers had specially mapped the route ahead of time and the vehicle crashed at least once during the shoot.
Behind the news: The United States National Highway Traffic Safety Administration (NHTSA) recently determined that a Tesla vehicle controlled by Autopilot in 2022 braked unexpectedly, leading to an eight-car pile-up. The accident occurred hours after Musk had tweeted that Autopilot was available to all North American drivers who purchased the option. (Previously it had been limited to drivers who had demonstrated safe driving.) NHTSA is investigating hundreds of complaints of Tesla vehicles braking unexpectedly.
Why it matters: Tech companies commonly promote capabilities well ahead of their capacity to deliver. In many cases, the biggest casualties are intangibles like the public’s trust and investors’ bank accounts. When it comes to self-driving cars, false promises can be deadly.
We're thinking: A company’s engineers are often the only ones who have the experience and perspective to foresee the consequences of a misleading product demo. When they do, their duty is not to keep mum but to push back.
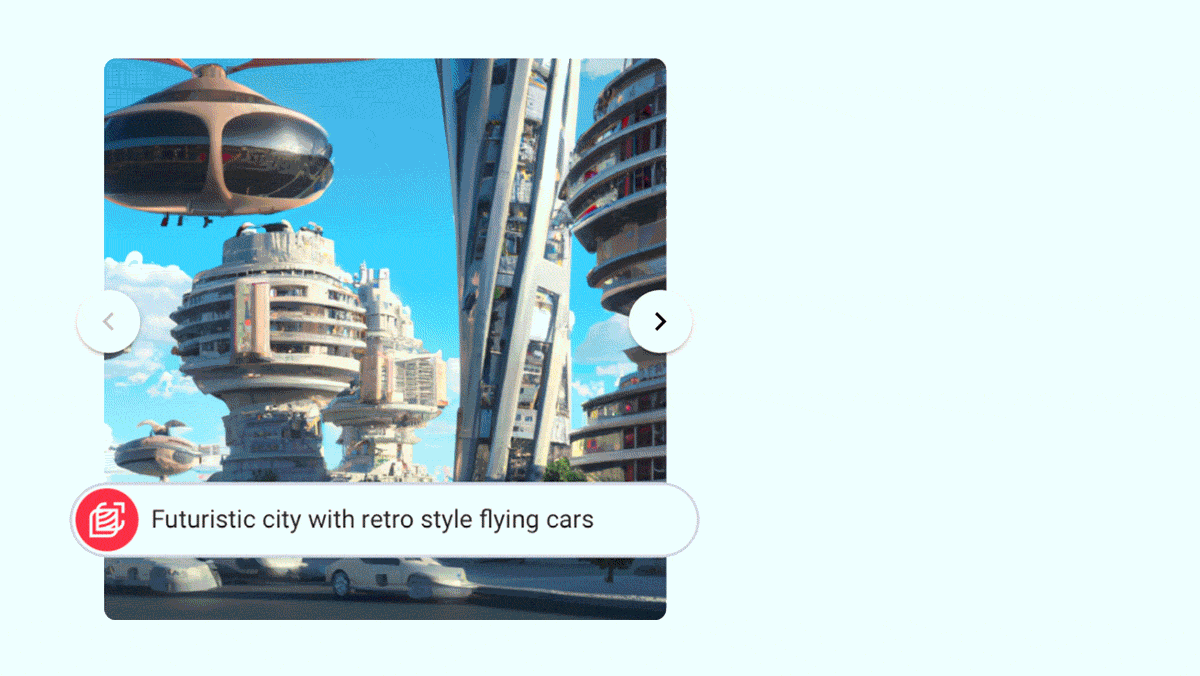
An Image Generator That Pays Artists
A top supplier of stock images will compensate artists who contribute training data to its image-generation service.
What's new: Shutterstock, which launched a text-to-image generator to supplement its business in licensing images, committed to sharing revenue with contributors who permit the company to use their artwork and photographs to train its model.
How it works: The image generator is based on OpenAI’s DALL·E 2 and built in collaboration with LG AI Research.
- The developers trained the model using images (and corresponding metadata) created by artists whose work Shutterstock licenses to its customers. Contributors will be able to opt out of having their images used in future training sets.
- Shutterstock will reimburse contributors an unspecified percentage of the licensing fee for each image the model generates based on the number of their images included in the training dataset. The company offers the same deal to contributors who permit it to include their work in datasets to be licensed to third parties. Contributors will receive payment every six months.
- Users who sign up for a free account can generate up to six images per day. The company charges a fee to download and use them. Users can also upload images generated by Shutterstock’s model for licensing to other customers. The company doesn’t accept images generated by third-party image generators.
Behind the news: Rival stock-image supplier Getty banned the uploading and licensing of AI-generated art in September. Getty also recently announced its intent to sue Stability AI, developer of the Stable Diffusion image generator, claiming that the model’s training set included millions of images owned or licensed by Getty, which Stability AI used without permission.
Yes, but: Shutterstock’s revenue in 2021, the most recent year reported, was around $773 million, and image generation is likely to represent a small fraction of the revenue. Meanwhile, Image generation models like DALL·E 2 are trained on hundreds of millions of images. This suggests that individual payouts for most contributors likely will be minuscule for the foreseeable future.
Why it matters: Image generation could disrupt the business of licensing stock images. Why pay for a license when you can generate a suitable image for pennies? Shutterstock is confronting the threat proactively with a bid to own a piece of the emerging market for generated media.
We're thinking: Much of the debate over how to compensate artists for data used to train image generators has focused on what’s legal. A more important question is what’s fair. Once we hash that out, legislators can get to work updating copyright laws for a digital, AI-enabled, generative world.
A MESSAGE FROM FOURTH BRAIN
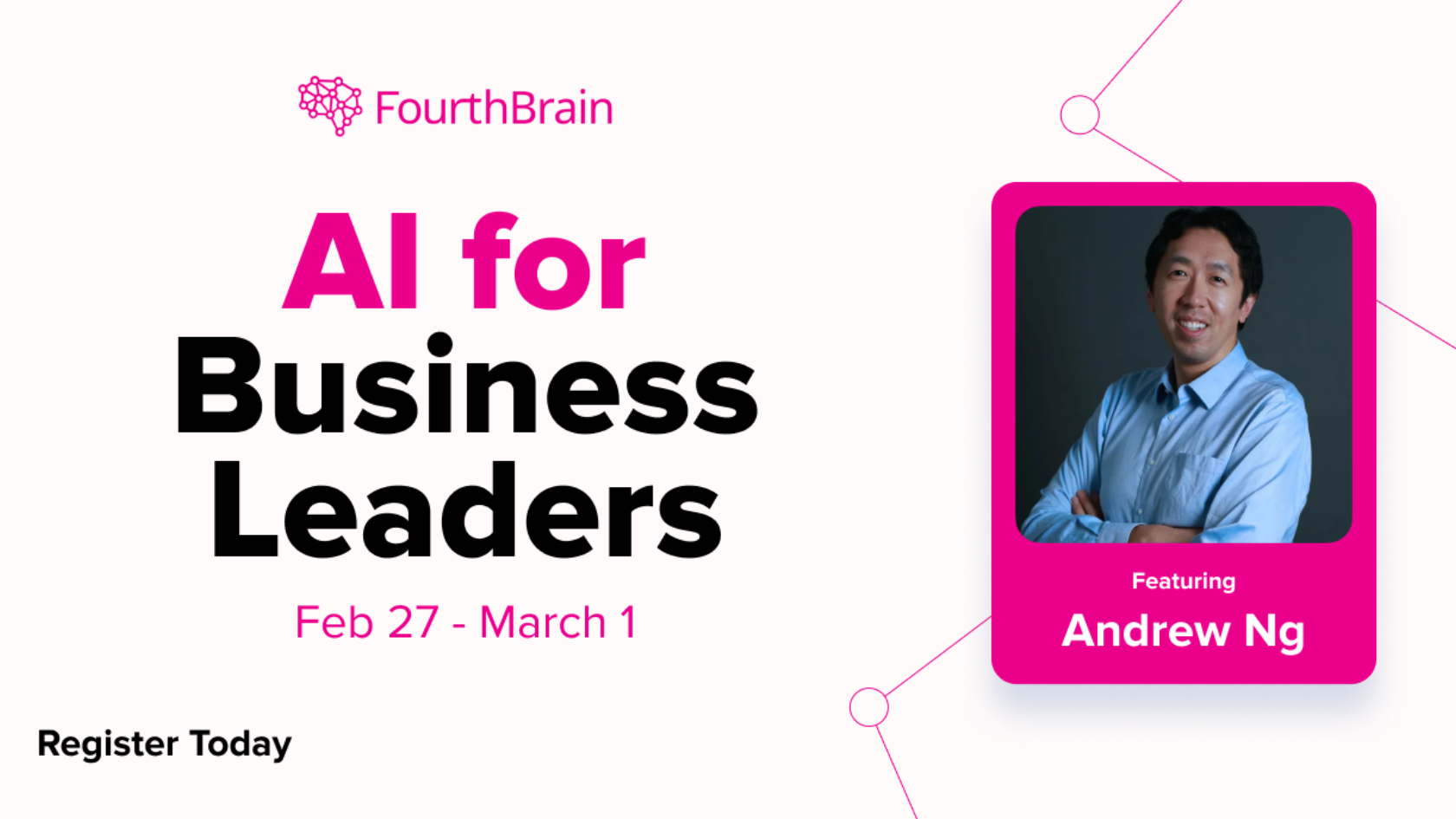
Build a practical action plan to grow your organization using AI! Join FourthBrain’s live, three-day workshop for business leaders and executives between February 27 and March 1, 2023. Register today
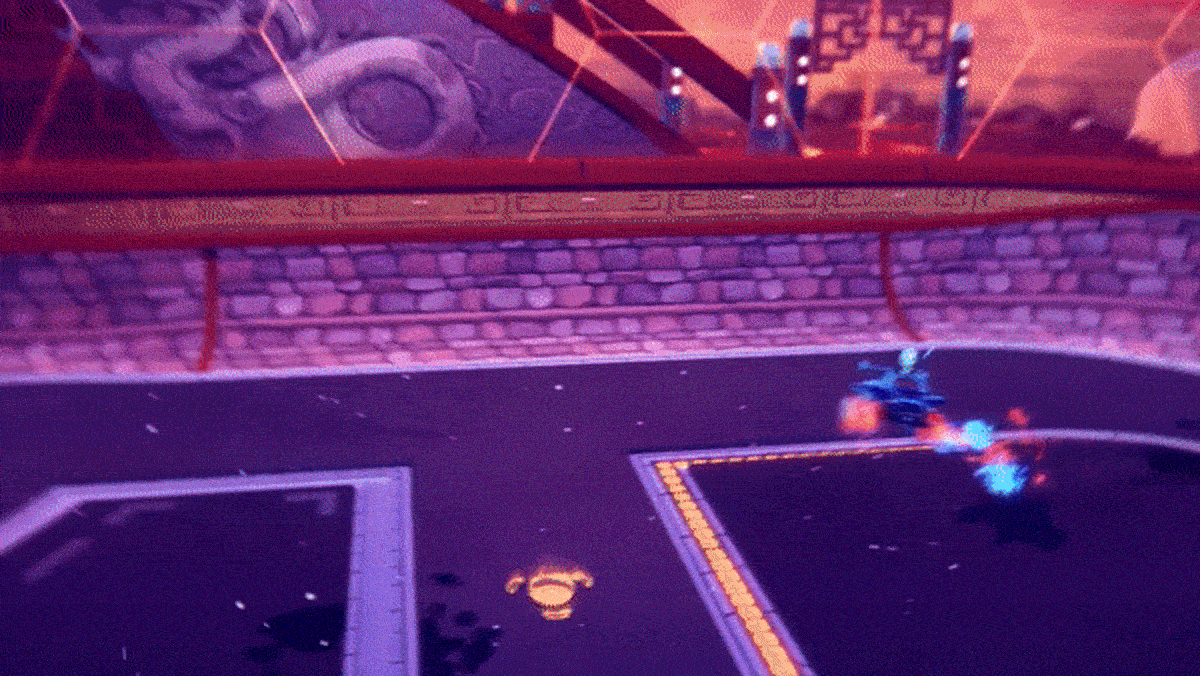
AI Cheat Bedevils Popular Esport
Reinforcement learning is powering a new generation of video game cheaters.
What’s new: Players of Rocket League, a video game that ranks among the world’s most popular esports, are getting trounced by cheaters who use AI models originally developed to train contestants, PC Gamer reported.
The game: Rocket League’s rules are similar to football (known as soccer in the United States): Players aim to force a ball into their opponent’s goal at the other end of an arena — except, rather than kicking the ball, they push it with a race car. Doing so, however, requires mastering the game’s idiosyncratic physics. Players can drive up the arena’s walls, turbo-boost across the pitch, and launch their car into the air.
How it works: The cheat takes advantage of a bot known as Nexto. Developed by AI-savvy players as a training tool, Nexto and similar bots typically include hard-coded restrictions against being used in competitive online play. However, someone customized the bot, enabling it to circumvent the restriction, one of Nexto’s developers revealed in a discussion on Reddit.
- Nexto was trained using RLGym, an API that allows bot-makers to treat Rocket League as a simulation environment for reinforcement learning.
- Its reward function examined physics parameters within the game such as the velocity of the user’s car, its distance to the ball, and where it touches the ball during a pass or shot.
- Nexto learned by playing against itself in approximately 250,000 hours (roughly 29 years 24/7) worth of gameplay, typically playing many accelerated games simultaneously. The developers estimate that its performance matches that of the top 1 percent of players.
- Nexto’s developers are working on a new bot that can learn from gameplay against human players. They plan not to distribute it beyond their core group to prevent cheaters from exploiting it.
- Rocket League developer Psyonix has banned players it determined cheated with bots including Nexto.
Behind the news: Despite reinforcement learning’s ability to master classic games like go and video games like StarCraft II, news of AI-powered cheats has been scant. The developers of Userviz, a cheatbot for first-person shooters that automatically aimed and fired on enemies detected by a YOLO implementation, deleted access to the app after receiving legal notice from video game publisher Activision.
Why it matters: Video games are big business. Rampant cheating could impact a game’s sales by ruining the experience for casual players. Cheating can also tarnish the reputation of games that, like Rocket League, are played professionally, where top players stand to win millions of dollars.
We’re thinking: While we condemn cheating, we applaud anyone who is so motivated to improve their gaming skill that they develop reinforcement learning models to compete against!
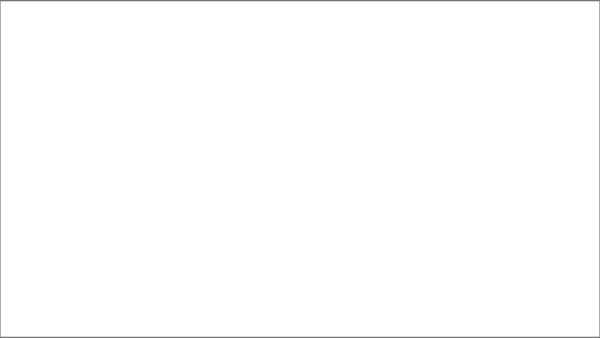
Language Models Defy Logic
Who would disagree that, if all people are mortal and Socrates is a person, Socrates must be mortal? GPT-3, for one. Recent work shows that bigger language models are not necessarily better when it comes to logical reasoning.
What’s new: Researchers tested the ability of language models to determine whether a statement follows a set of premises. Simeng Han led the project with collaborators at Yale University, University of Illinois, Iowa City West High School, University of Washington, University of Hong Kong, Penn State University, Meta, and Salesforce.
Key insight: Previous efforts to test logical reasoning in language models were based on datasets that contained limited numbers of words (roughly between 100 and 1,000), premises (up to five per example), and logical structures (less than 50). A more diverse dataset would make a better test.
How it works: The authors assembled FOLIO, a dataset of over 1,400 examples of real-world logical reasoning that uses more than 4,350 words, up to eight premises, and 76 distinct logical structures. They challenged a variety of models to classify whether the relationship between a set of premises and an example conclusion was true, false, or unknown.
- The authors asked human annotators to generate logical stories of premises and a conclusion. They verified the logic using an automated program.
- They tested BERT and RoBERTa, two of the most popular language encoders, by appending two fully connected layers and fine-tuning the models on 70 percent of the dataset.
- They tested Codex, GPT-3, GPT-NeoX-20B, and OPT in 13- and 66-billion parameter variations. They prompted the models with eight labeled examples. Then the model classified an unlabelled example.
Results: A fine-tuned RoBERTa-large (340 million parameters) accurately labeled 62.11 percent of FOLIO’s test examples, while a fine-tuned BERT-large of the same size achieved 59.03 percent accuracy. The probability of predicting the correct answer at random was 33.33 percent. Given eight labeled logic stories as input, Codex (of unknown size) achieved 56.04 percent accuracy, while GPT-3 (175 billion parameters) achieved 43.44 percent.
Why it matters: Language models can solve simple logic puzzles, but their performance is inconsistent and depends a great deal on the prompt they’re given. This work offers a more rigorous benchmark for tracking progress in the field.
We’re thinking: The recently unveiled ChatGPT has wowed many users, but its ability to solve logic problems varies wildly with the prompt. It’s not clear whether some of the outputs shared on social media represented its best — or most embarrassing — results. A systematic study like this would be welcome and important.
Data Points:
Research: Google devised a next-generation AI music generator, but the company has yet to release it.
MusicLM can generate coherent songs from complex descriptions. Google is keeping it under wraps while it sorts out ethical and legal challenges. (TechCrunch)
Research: A large language model generated functional protein sequences.
Researchers trained a language model called ProGen to produce synthetic enzymes, a type of globular protein, and some of them worked as well as those found in nature. (Vice)
Professors are navigating the pros and cons of ChatGPT in education.
An educational dilemma takes shape: Should text generators be banned or embraced? (The Wall Street Journal)
Academic publisher Springer Nature announced guidelines for the use of text generators.
The world’s largest academic publishing company established ground rules for using large language models ethically to produce scientific papers. (The Verge)
A long-running controversy over which species laid a prehistoric eggs has finally come to an end.
A machine learning model helped scientists confirm that eggshells found in the 1980s belonged to a giant, extinct bird called Genyornis. (The Conversation)
Research: The exporting of surveillance technology to countries experiencing political unrest may have negative effects.
China’s exports of AI technology used for state surveillance have the potential to reinforce and give rise to more autocratic countries. (Brookings)
Researchers unveiled the mystery of a Renaissance painting.
A face recognition system found a painting attributed to an unknown artist is likely to be a Raphael masterpiece. (BBC)
A plan to replace a lawyer with an AI legal assistant in court fell apart.
DoNotPay, a startup that offers AI-powered legal services, intended to deploy its chatbot to represent a defendant in a U.S. court, but state prosecutors threatened the company’s CEO with possible prosecution and jail time. (NPR)
BuzzFeed will use OpenAI’s services to enhance its content.
This digital media company plans to use automated methods to personalize content for its audiences. (The Wall Street Journal)
Research: A startup is exploring new smells with the help of AI.
Google spinout Osmo aims to create the next generation of aromatic molecules for everyday products. (Wired)
Research: ALS patient communicated 62 words per minute using a brain impant, breaking previous record.
A brain-computer interface (BCI) decoded speech in an ALS patient 3.4 times faster than the prior record for any kind of BCI. (MIT Technology Review)