Dear friends,
Activities such as writing code and solving math problems are often perceived as purely intellectual pursuits. But this ignores the fact that they involve the mental equivalent of muscle memory.
The idea of muscle memory is a powerful concept in human learning. It has helped millions of people to understand the importance of practice in learning motor tasks. However, it’s also misleading because it excludes skills that don’t involve using muscles.
I believe that a similar principle operates in learning intellectual skills. Lack of recognition of this fact has made it harder for people to appreciate the importance of practice in acquiring those skills as well.
The phenomenon of muscle memory is widely acknowledged. When you repeatedly practice balancing on a bicycle, swinging a tennis racquet, or typing without looking at the keyboard, adaptations in your brain, nervous system, and muscles eventually allow you to carry out the task without having to consciously pay attention to it.
The brain and nervous system are central to learning intellectual skills, and these parts of the body also respond to practice. Whether you’re writing code, solving math problems, or playing chess, practice makes you better at it. It leads your brain to form mental chunks that allow you to reason at a higher level. For example, a novice programmer has to think carefully about every parenthesis or colon, but with enough practice, coding common subroutines can take little conscious effort. Practice frees up your attention to focus on higher-level architectural issues.
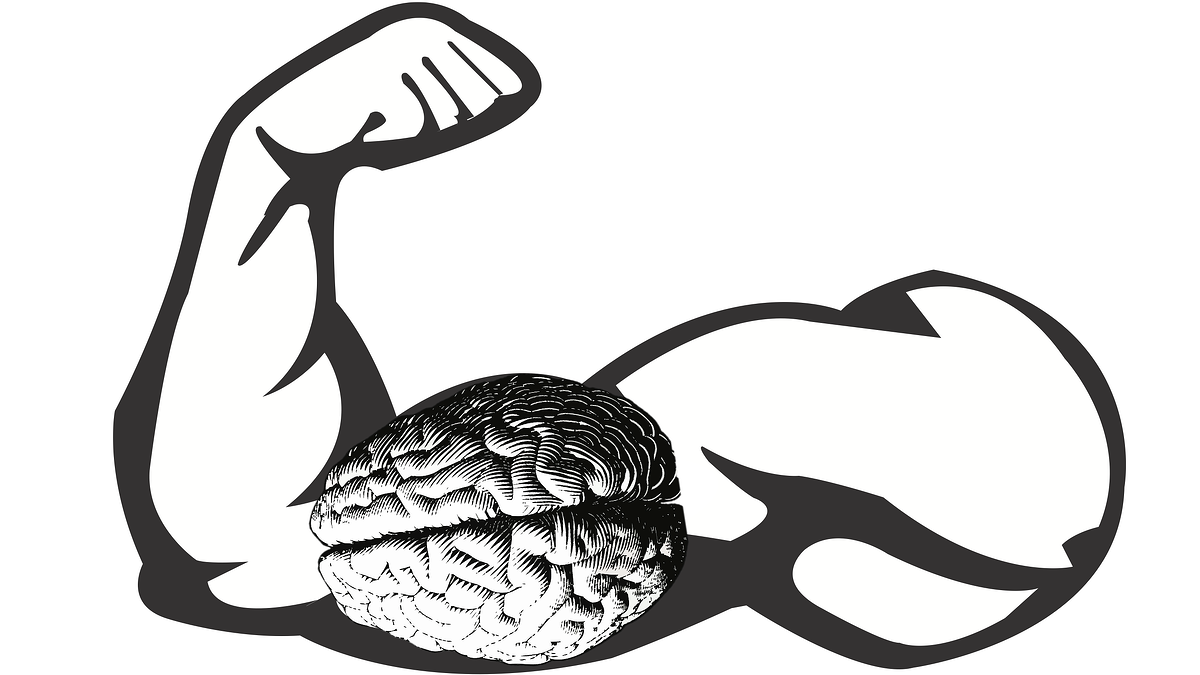
Of course, there are biological differences between learning motor skills and learning intellectual skills. For example, the former involves parts of the brain that specialize in movement. And the physical world presents somewhat different challenges each time you perform an action (for example, your bicycle hits different bumps, and an opposing tennis player returns each of your serves differently). Thus practicing motor skills automatically leads you to try out your actions in different situations, which trains your brain to adapt to different problems.
But I think there are more similarities than people generally appreciate. While watching videos of people playing tennis can help your game, you can’t learn to play tennis solely by watching videos. Neither can you learn to code solely by watching videos of coding. You have to write code, see it sometimes work and sometimes not, and use that feedback to keep improving. Like muscle memory, this kind of learning requires training the brain and nervous system through repetition, focused attention, making decisions, and taking breaks between practice sessions to consolidate learning. And, like muscle memory, it benefits from variation: When practicing an intellectual task, we need to challenge ourselves to work through a variety of situations rather than, say, repeatedly solving the same coding problem.
All of this leads me to think that we need an equivalent term for muscle memory in the intellectual domain. As knowledge work has come to play a larger economic role relative to physical labor, the ability to learn intellectual tasks has become much more important than it was when psychologists formed the idea of muscle memory around 150 years ago. This new term would help people understand that practice is as crucial to developing intellectual skills as muscular ones.
How about intellect memory? It’s not an elegant phrase, but it acknowledges this under-appreciated reality of learning.
What intellectual task do you develop intellect memory for, and can you find time in your schedule to do the necessary practice? After all, there’s no better way to learn.
Keep learning!
Andrew
News
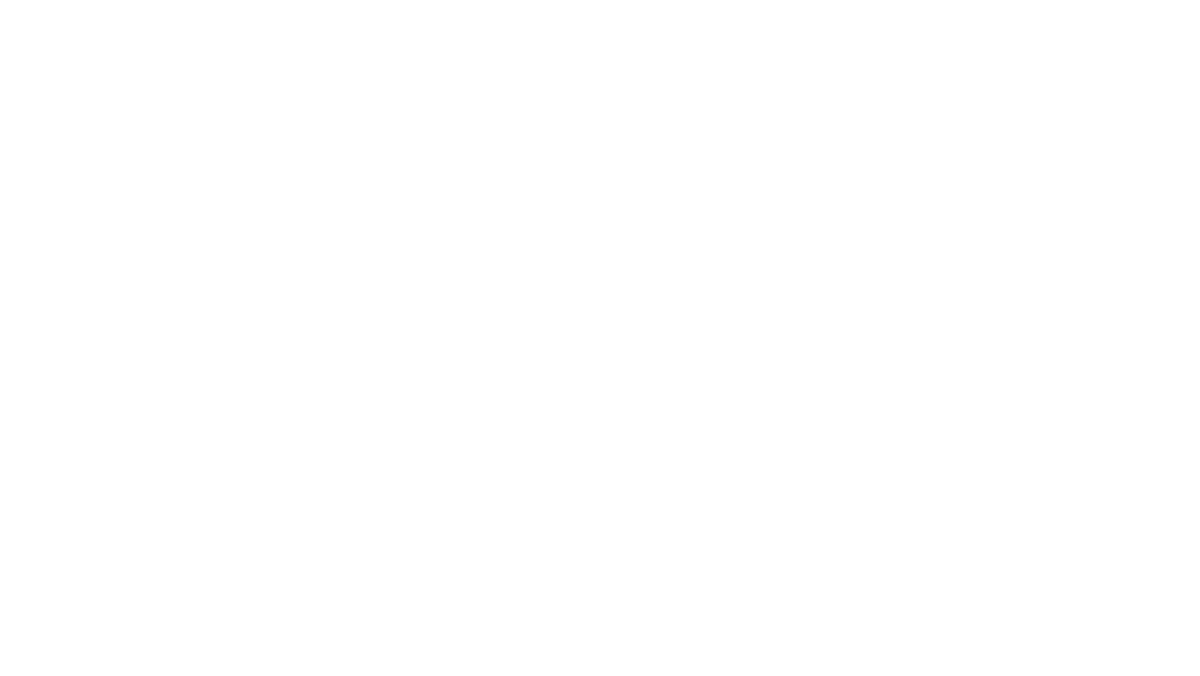
Data Scientists on Data Science
A survey of data scientists reveals a field of great opportunities but also room for improvement.
What’s new: The 2022 “State of Data Science” report from Anaconda, maker of a popular Python distribution, surveyed 3,493 students, teachers, and employees in data science, machine learning, and AI about their work and opinions of the field.
Who they surveyed: The poll reached data scientists in 133 countries (40 percent in the U.S. or Canada). 76 percent were men, 23 percent women, and 2 percent nonbinary. 80 percent had at least an undergraduate-level degree. The majority — 55 percent — worked for firms with 1,000 or fewer employees, while 15 percent worked for companies with over 10,000 employees.
State of the field: Participants were asked to rate various aspects of their day-to-day work and share their hopes for the future. They expressed widespread satisfaction but expressed worries about the field’s potential for harm.
- On the job, 70 percent of respondents reported being at least moderately satisfied. Professors, instructors, and teachers reported the highest levels of job satisfaction.
- Respondents spent an average of 51 percent of their time at work preparing, cleansing, or visualizing data and 18 percent selecting and training models.
- Of those who deployed models, 60 percent deployed them on-premises, while 40 percent deployed them in the cloud.
- Most respondents preferred to program in Python, and 31 percent used it every day. 16 percent used SQL daily. Single-digit percentages were daily users of other languages including C/C++, Java, and Rust.
- Of the students surveyed, 27 percent hoped to work for a well-established startup, 23 percent for an industry giant, and 22 percent for an academic institution or research lab.
Challenges: Respondents also answered questions about challenges they face, and those faced by data science at large:
- Many of those surveyed felt their organizations could do more to support them in their work. The biggest barriers were under-investment (65 percent), insufficient access to talent (56 percent), and unrealistic expectations (43 percent).
- Students noted obstacles in finding internships (27 percent), job listings that weren’t clear about the qualifications required (20 percent), and lack of a professional network or mentoring (15 percent).
- 62 percent said their organizations were at least moderately affected by a scarcity of skilled workers. Those who were employed cited a dearth of talent in engineering (38 percent) and probability and statistics (33 percent).
- 32 percent said the biggest problem in the field was the social impact of bias, followed by data privacy (18 percent) and “advanced information warfare” (16 percent).
Behind the news: The U.S. Bureau of Labor Statistics forecasts that the number of computer and information research scientists will grow by 21 percent between 2021 and 2031 — far higher than the 5 percent average across all industries. Anecdotal evidence suggests that demand for skilled AI professionals already outstrips supply.
Why it matters: It’s great to hear that data science rates highly in both job satisfaction and market demand. The areas in which respondents expressed a desire for improvement — bias, privacy, the dearth of skilled engineers — suggest possible avenues for career development.
We’re thinking: Given that preparing, cleansing, and visualizing data takes up 51 percent of time spent on data science, and selecting and training models occupies only 18 percent, it appears that most practitioners already do data-centric AI development. They just need better principles and tools to help them do this work more efficiently!
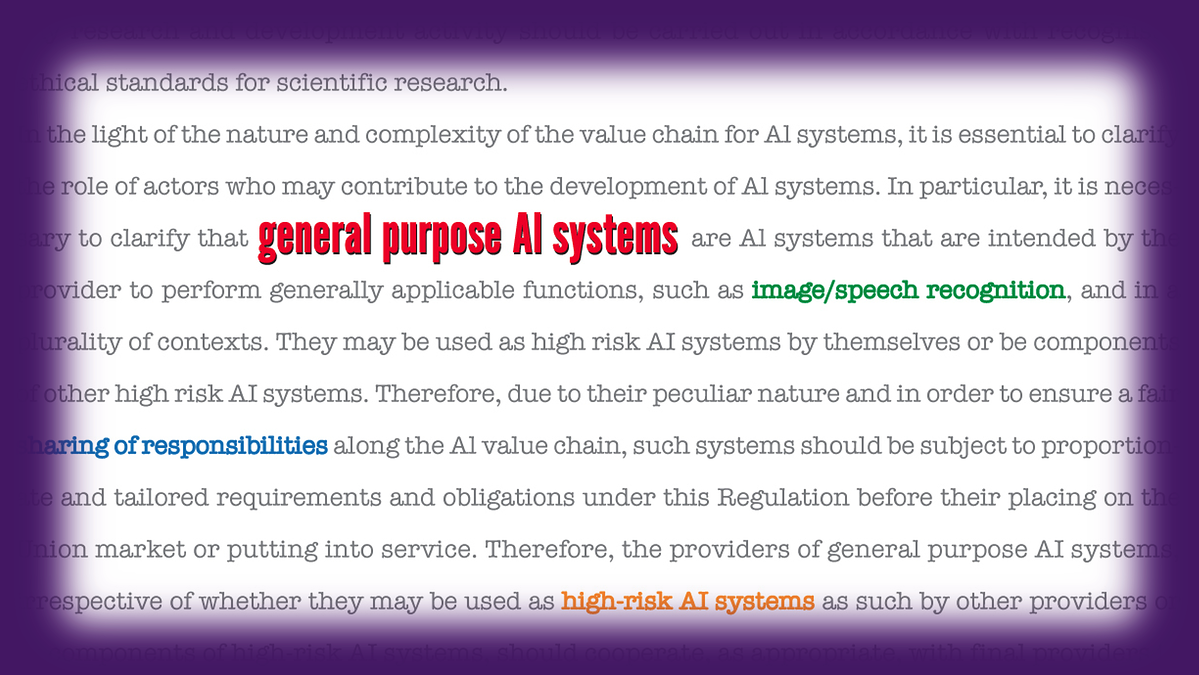
Regulating AI in Undefined Terms
A proposed European Union law that seeks to control AI is raising questions about what kinds of systems it would regulate.
What's new: Experts at a roundtable staged by the Center for Data Innovation debated the implications of limitations in the EU’s forthcoming Artificial Intelligence Act.
The controversy: The legislation is in the final stages of revision and moving toward a vote next year. As EU parliamentarians worked to finalize the proposed language, the French delegation introduced the term “general-purpose AI,” which is described as any system that can “perform generally applicable functions such as image/speech recognition, audio/video generation, pattern-detection, question-answering, translation, etc., and is able to have multiple intended and unintended purposes.” Providers of general-purpose AI would be required to assess foreseeable misuse, perform regular audits, and register their systems in an EU-wide database. The proposal has prompted worries that the term’s vagueness could hinder AI development.
The discussion: The roundtable’s participants were drawn from a variety of companies, nongovernmental organizations, and government agencies. They generally agreed that the proposed definition of general-purpose AI was too broad and vague. The consequences, they warned, could include criminalizing AI development and weakening protection against potential abuses.
- Anthony Aguirre, strategist at the Future of Life Institute, noted that “general-purpose AI” has meanings beyond those that the proposed law delineates.
- Kai Zenner, advisor to German EU parliamentarian Axel Voss, expressed concern over the law’s potential impact on open-source development. He argued that it would make anyone who worked on an open-source model legally responsible for its impact, destroying the trust essential to building such software.
- Alexandra Belias, DeepMind’s international public policy manager, recommended augmenting the definition with criteria, like the range of tasks a model can perform.
- Irene Solaiman, policy director at HuggingFace, said the proposed definition fails to account for potential future capabilities and misuses. She suggested that regulators classify AI systems according to their use cases to see where they might fit into existing laws.
- Andrea Miotti, head of policy at Conjecture, an AI research lab, suggested using terms more commonly used and better understood by the AI community, such as “foundation models.” He also said the law focused too tightly on limiting system providers rather than protecting users.
Behind the news: Initially proposed in 2021, the AI Act would sort AI systems into three risk levels. Applications with unacceptable risk, such as social-credit systems and real-time face recognition, would be banned outright. High-risk applications, such as applications that interact with biometric data, would face heightened scrutiny including a mandated risk-management system. The law would allow unfettered use of AI in applications in the lowest risk level, such as spam filters or video games.
Why it matters: The AI Act, like the EU’s General Data Protection Regulation of 2018, likely will have consequences far beyond the union’s member states. Regulators must thread the needle between overly broad wording, which risks stifling innovation and raising development costs, and narrow language that leaves openings for serious abuse.
We're thinking: The definition of AI has evolved over the years, and it has never been easy to pin down. Once, an algorithm for finding the shortest path between two nodes in a graph (the A* algorithm) was cutting-edge AI. Today many practitioners view it as a standard part of any navigation system. Given the challenge of defining general-purpose AI — never mind AI itself! — it would be more fruitful to regulate specific outcomes (such as what AI should and shouldn't do in specific applications) rather than try to control the technology itself.
A MESSAGE FROM OUR PARTNER
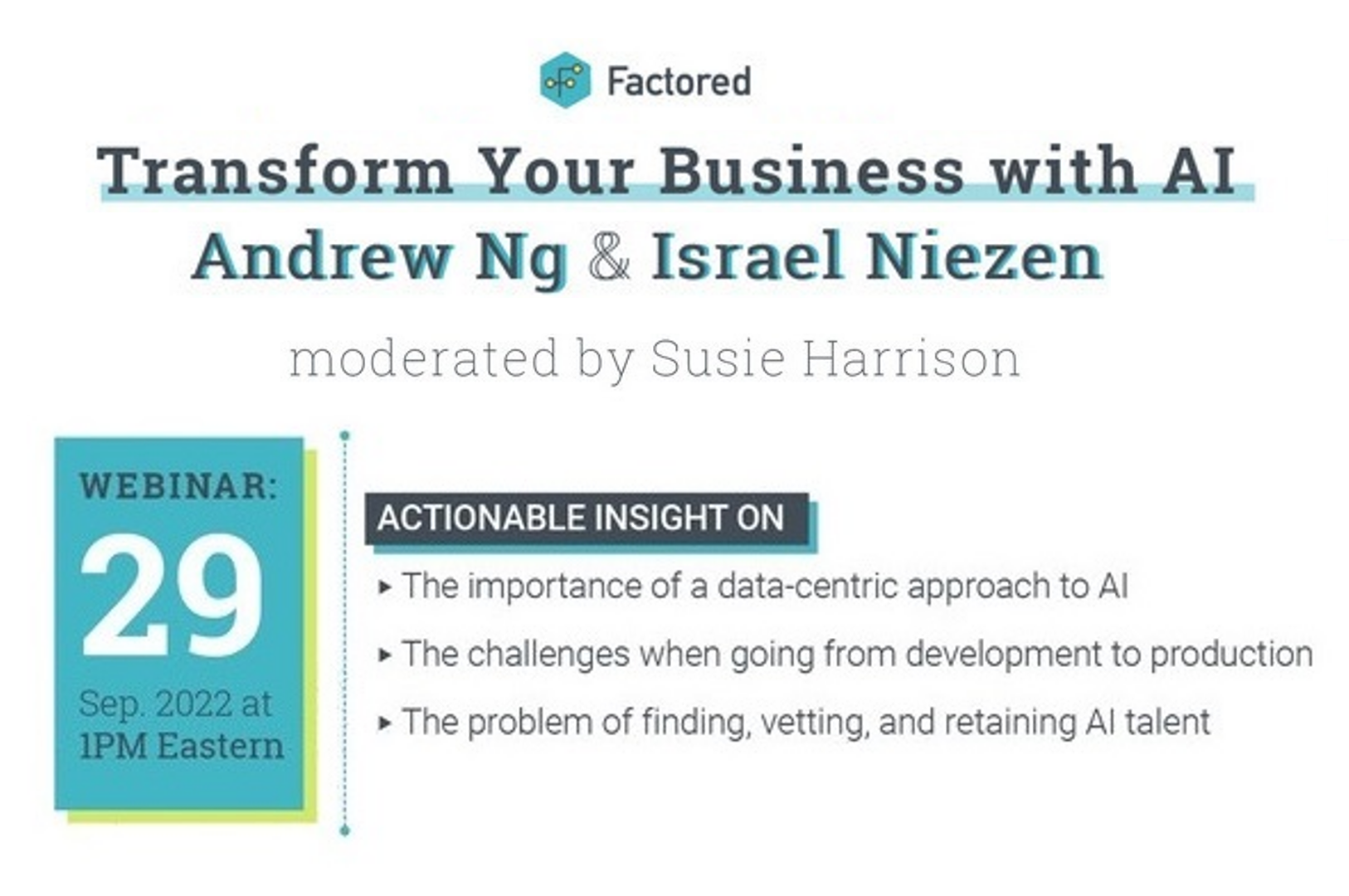
AI has undisputed business value. So why do many companies fail to realize its potential? Join Andrew Ng, Israel Niezen (co-founder of Factored), and Susie Harrison (AI editor at Informa Tech) on September 29, 2022, at 1 p.m. Eastern Time for lessons on how to make AI a profitable part of your business. Register now
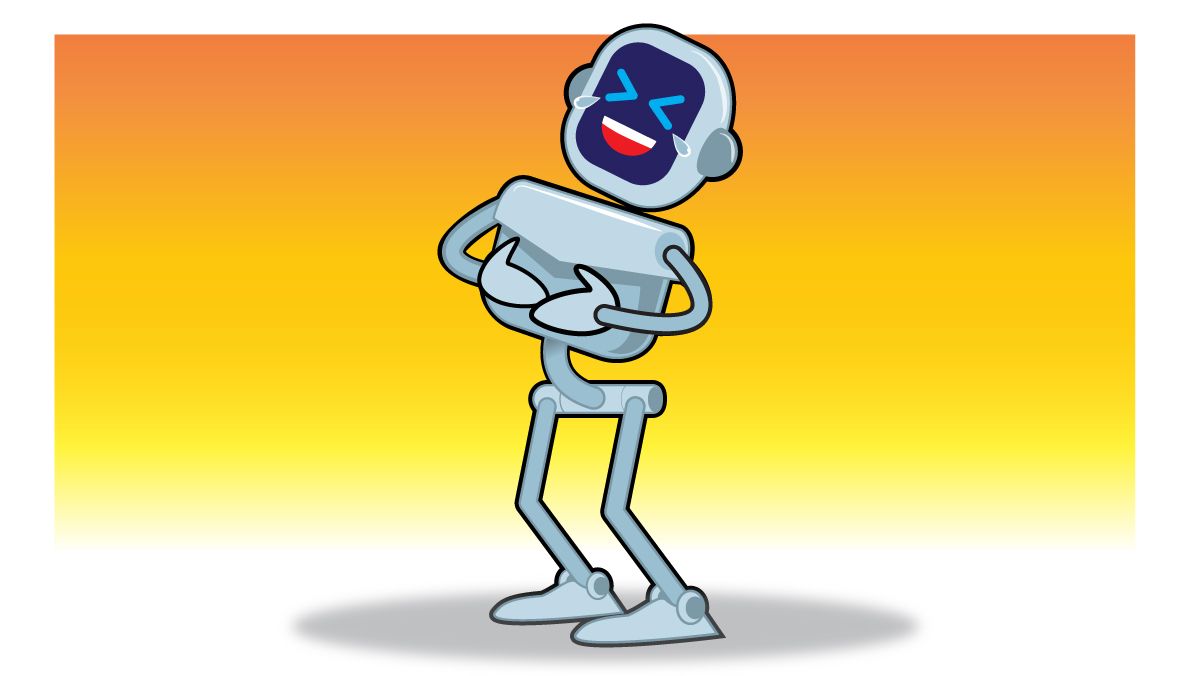
Toward Machines That LOL
Even if we manage to stop robots from taking over the world, they may still have the last laugh.
What’s new: Researchers at Kyoto University developed a series of neural networks that enable a robot engaged in spoken conversation to chortle along with its human interlocutor.
How it works: The authors built a system of three models that, depending on a user’s spoken input, emitted either a hearty hoot, a conversational chuckle, or no laugh at all. They trained all three models on recordings of speed-dating dialogs between humans and Erica, an android teleoperated by an actress, which they deemed to be rich in social laughter.
- The first model detected a conversant’s laughter. Given an utterance represented as a sequence of mel filter bank coefficients (features that describe the frequencies that make up a short audio segment), a recurrent neural network featuring BiGRUs learned to determine whether the utterance ended in a laugh.
- The second model decided when the conversant’s outburst called for a sympathetic cackle. If the utterance didn’t end in a laugh, the system didn’t generate a laughing response. If it did, the authors fed the mean and variance of the mel filter bank features, plus features that described the utterance’s lowest frequency and volume, into a logistic regression model, which learned whether or not to join in.
- The third model chose the type of laugh to use. The authors fed the same features into another logistic regression model. It learned whether to play a recording of giggles or guffaws.
Results: The authors’ system and two baselines responded to brief monologues that included laughter, while more than 30 crowdsourced workers judged naturalness and human-likeness on a scale of 1 to 7. The authors’ system achieved an average 4.01 for naturalness and 4.36 for human-likeness. One baseline, which never laughed, scored an average 3.89 for naturalness and 3.99 for human-likeness. The other, which always reacted to laughter in the monologue with a social laugh, scored an average of 3.83 for naturalness and 4.16 for human-likeness.
Behind the news: About the training corpus: The authors recorded speed-dating dialogs with Erica as part of a larger effort to elicit human-machine conversations that delved more deeply into human issues than typical text dialogs with chatbots. Built by researchers at Kyoto and Osaka Universities and Kyoto’s Advanced Telecommunications Research Institute, the feminine-styled automaton has rapped, anchored TV news, and been cast to play the lead role in a science-fiction film scheduled for release in 2025.
Why it matters: Automating laughter is no joke! Mastering when and how to laugh would be valuable in many systems that aim to integrate seamlessly with human conversation. Titters, snickers, and howls play a key role in bonding, agreement, affection, and other crucial human interactions. Laughter’s role varies in different communities, yet it can cross cultures and bring people together.
We’re thinking: We’re glad the robots are laughing with us, not at us!
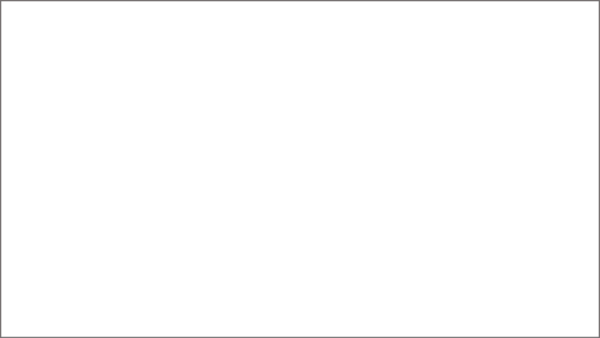
Automated Mattes for Visual Effects
An image matte is what makes it possible to take an image of a zebra in a zoo, extract the zebra, and paste it over a savannah background. Make the background (zoo) pixels transparent, leave the foreground (zebra) pixels opaque, and maintain a fringe of semitransparent pixels around the foreground (the zebra’s fur, especially its whispy mane and tail), which will combine the colors of the original foreground and the new background. Then you can meld the foreground seamlessly with any background. New work produces mattes automatically with fewer errors than previous machine learning methods.
What’s new: Guowei Chen, Yi Liu, and colleagues at Baidu introduced PP-Matting, an architecture that, given an image, estimates the transparency of pixels surrounding foreground objects to create mattes without requiring additional input.
Key insight: Previous matte-making approaches require a pre-existing three-level map, or trimap, that segments foreground, background, and semitransparent transitional regions. The previous best neural method trains one model to produce trimaps and another to extract the foreground and estimate transparency. But using two models in sequence can result in cumulative errors: If the first model produces an erroneous trimap, the second will produce an erroneous matte. Using a single model to produce both trimaps and mattes avoids such errors and thus produces more accurate output.
How it works: The authors’ model comprises a convolutional neural network (CNN) encoder that feeds into two CNN branches. They trained and tested it on Distinctions-646 and Adobe Composition-1k, datasets that contain foreground images of people, objects, or animals, each stacked atop a background image, with a transparency value for each pixel.
- One branch classified each pixel of an input image as foreground, background, or transitional area, creating a trimap. A Pyramid Pooling Module captured large- and small-scale features by scaling and processing the encoder’s output to produce representations at different scales. It concatenated these representations with the encoder’s output and fed them to the CNN, which produced the trimap. During training, the loss function encouraged the trimap to match the ground-truth trimap.
- The other branch estimated the transparency of each pixel, creating a so-called detail map. To take advantage of context from the trimap, the model combined the output of each convolutional layer in this branch with the output of each layer in the other branch using a Gated Convolutional Layer. During training, the loss function encouraged the estimated transparencies and the difference in transparency between adjacent pixels to be similar to ground truth. The loss was applied only to pixels in transitional regions.
- The model replaced the transitional areas of the trimap with the corresponding areas of the detail map, producing a final matte. During training, it reapplied the loss function in the previous step to the entire matte.
- The model used the generated matte to estimate pixel colors in the original image. It applied the generated matte to the ground-truth foreground and stacked it atop the ground-truth background. A further loss function encouraged the estimated pixel colors to match ground truth.
Results: The authors compared their model with techniques that require trimap inputs, including IndexNet (the best competing method) and Deep Image Matting. They also compared with Hierarchical Attention Matting Network (HAttMatting), a single model that doesn’t require trimap inputs but also doesn’t produce the trimaps internally. The authors’ method achieved equal or better performance on three of four metrics for both datasets. On Composition-1k, the authors’ method scored a mean squared error of 0.005, equal to IndexNet. On Distinctions-646, it achieved 0.009 mean squared error, equal to Deep Image Matting and HAttMatting.
Why it matters: The main problems with previous trimap-free approaches to matting were cumulative errors and blurred output. This work addresses cumulative errors by separating processes into different branches. It addresses image quality by feeding output from the first branch into the second to refine representations of transitional areas.
We're thinking: The ability to produce high-quality mattes without needing to produce trimaps by hand seems likely to make video effects quicker and less expensive to produce. If so, then deep learning is set to make graphics, movies, and TV — which are already amazing — even more mind-boggling!