Dear friends,
I’ve written about how to build a career in AI and focused on tips for learning technical skills, choosing projects, and sequencing projects over a career. This time, I’d like to talk about searching for a job.
A job search has a few predictable steps including selecting companies to apply to, preparing for interviews, and finally picking a job and negotiating an offer. In this letter, I’d like to focus on a framework that’s useful for many job seekers in AI, especially those who are entering AI from a different field.
If you’re considering your next job, ask yourself:
- Are you switching roles? For example, if you’re a software engineer, university student, or physicist who’s looking to become a machine learning engineer, that’s a role switch.
- Are you switching industries? For example, if you work for a healthcare company, financial services company, or a government agency and want to work for a software company, that’s a switch in industries.
A product manager at a tech startup who becomes a data scientist at the same company (or a different one) has switched roles. A marketer at a manufacturing firm who becomes a marketer in a tech company has switched industries. An analyst in a financial services company who becomes a machine learning engineer in a tech company has switched both roles and industries.
If you’re looking for your first job in AI, you’ll probably find switching either roles or industries easier than doing both at the same time. Let’s say you’re the analyst working in financial services:
- If you find a data science or machine learning job in financial services, you can continue to use your domain-specific knowledge while gaining knowledge and expertise in AI. After working in this role for a while, you’ll be better positioned to switch to a tech company (if that’s still your goal).
- Alternatively, if you become an analyst in a tech company, you can continue to use your skills as an analyst but apply them to a different industry. Being part of a tech company also makes it much easier to learn from colleagues about practical challenges of AI, key skills to be successful in AI, and so on.
If you’re considering a role switch, a startup can be an easier place to do it than a big company. While there are exceptions, startups usually don’t have enough people to do all the desired work. If you’re able to help with AI tasks — even if it’s not your official job — your work is likely to be appreciated. This lays the groundwork for a possible role switch without needing to leave the company. In contrast, in a big company, a rigid reward system is more likely to reward you for doing your job well (and your manager for supporting you in doing the job for which you were hired), but it’s not as likely to reward contributions outside your job’s scope.
After working for a while in your desired role and industry (for example, a machine learning engineer in a tech company), you’ll have a good sense of the requirements for that role in that industry at a more senior level. You’ll also have a network within that industry to help you along. So future job searches — if you choose to stick with the role and industry — likely will be easier.
When changing jobs, you’re taking a step into the unknown, particularly if you’re switching either roles or industries. One of the most underused tools for becoming more familiar with a new role and/or industry is the informational interview. I’ll share more about that in the next letter.
Keep learning,
Andrew
P.S. I’m grateful to Salwa Nur Muhammad, CEO of FourthBrain (a DeepLearning.AI affiliate), for providing some of the ideas presented in this letter.
DeepLearning.AI Exclusive
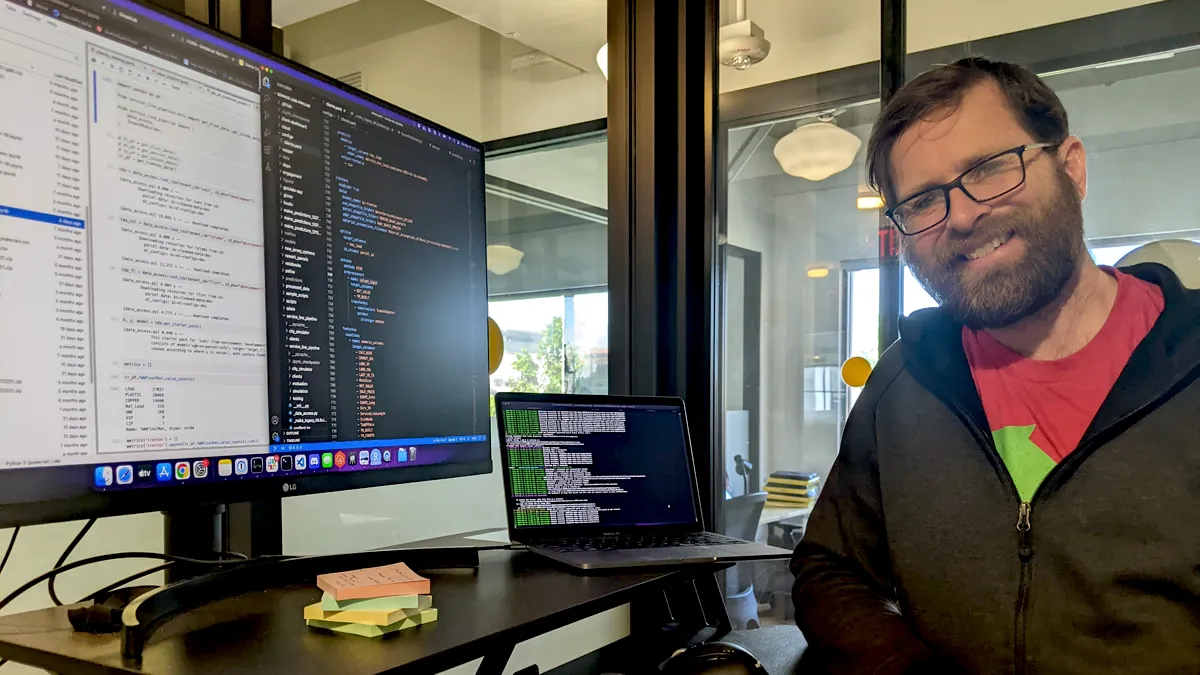
Working AI: Clean Water Warrior
Jared Webb was pursuing a PhD when a public-health crisis erupted. So he formed a company that uses AI to identify toxic water pipes. He spoke with us about solving real-world problems, switching from academia to business, and what he looks for when hiring AI talent. Read his story here
News
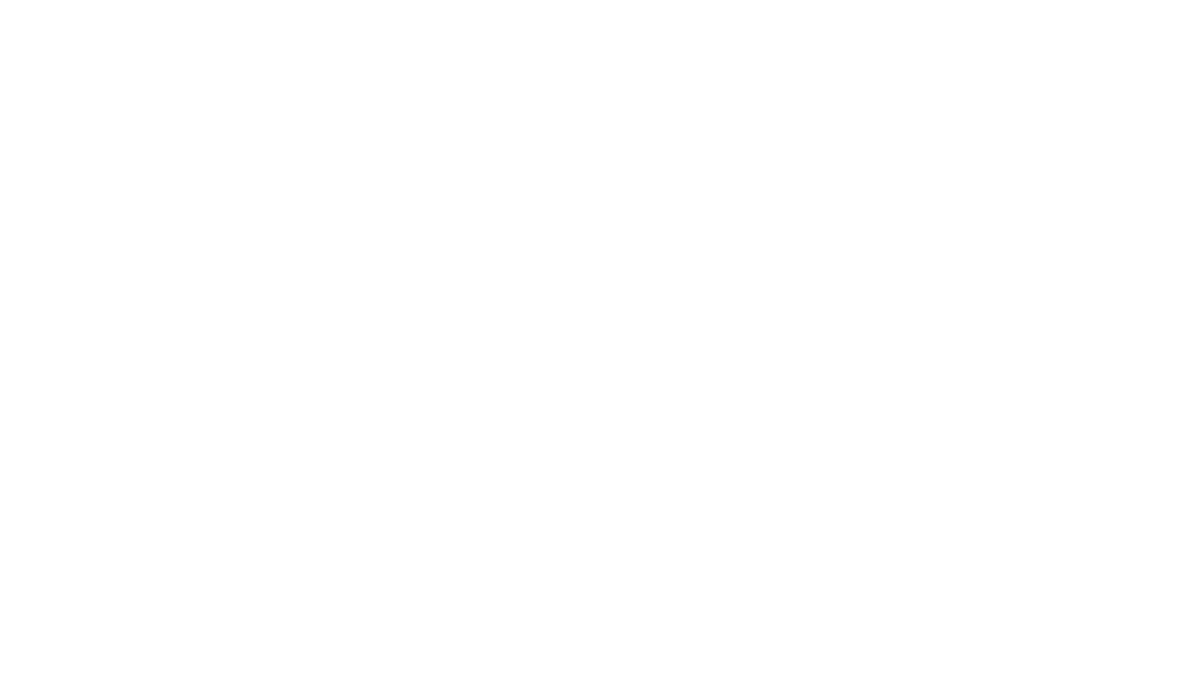
Bad Machine Learning Makes Bad Science
Misuse of machine learning by scientific researchers is causing a spate of irreproducible results.
What’s new: A recent workshop highlighted the impact of poorly designed models in medicine, security, software engineering, and other disciplines, Wired reported.
Flawed machine learning: Speakers at the Princeton University event highlighted common pitfalls that undermine reproducibility:
- Data leakage including lack of a test set, training on the test set, deciding which features to use based on those that performed well on the test set, and testing on datasets that include duplicate examples
- Drawing erroneous conclusions from insufficient data
- Applying machine learning when it’s not the best tool for the job
Behind the news: The workshop followed a recent meta-analysis by Princeton researchers that identified 329 scientific papers in which poorly implemented machine learning yielded questionable results.
Why it matters: Experienced machine learning practitioners are well aware of the pitfalls detailed by the workshop, but researchers from other disciplines may not be. When they apply machine learning in a naive way, they can generate invalid results that inherit an aura of credibility owing to machine learning’s track record of success. Such results degrade science and impinge on the willingness of more skeptical scientists to trust the efficacy of learning algorithms. Enquiries like this one will be necessary at least until machine learning becomes far more widely practiced and understood.
We’re thinking: Many AI practitioners are eager to contribute to meaningful projects. Partnering with scientists in other fields is a great way to gain experience developing effective models and educate experts in other domains about the uses and limitations of machine learning.
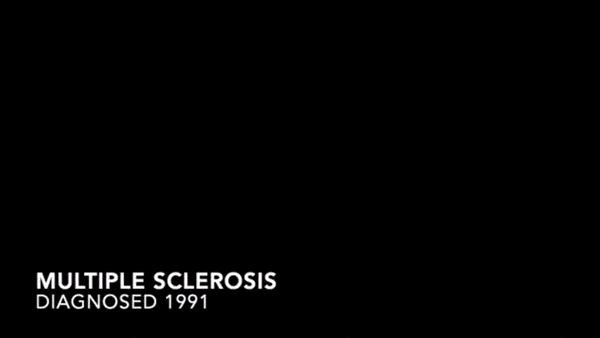
AI Hits Its Stride
A smart leg covering is helping people with mobility issues to walk.
What’s new: Neural Sleeve is a cloth-covered device that analyzes and corrects wearers’ errant leg movements. Developed by startup Cionic and product studio Fuseproject, the device is intended to help people with conditions that affect coordination of the legs, such as multiple sclerosis, cerebral palsy, spinal cord injury, and stroke.
How it works: The sleeve is fitted with electrodes that contact the wearer’s skin in the region of particular leg muscles. A machine learning model analyzes electrical impulses generated by muscles as they move and instructs the electrodes to stimulate the muscles in a way that corrects the wearer’s gait.
- The model was pretrained on muscle-motion data including examples of ideal and impaired walking, according to a patent filing. It’s fine-tuned on muscle data obtained from the specific patient who will wear it.
- The model uses input from the sleeve’s electrodes to determine the difference between the user’s motion and ideal motion.
- The model computes patterns of electrical impulses that will prod the user’s muscles toward the ideal motion and sends this information back to the sleeve’s electrodes, which deliver the specified impulses.
Behind the news: AI-enabled wearable devices have a wide variety of applications in making the world more accessible to people who are injured or otherwise disabled.
- Copenhagen-based Oticon makes hearing aids that use a neural network to identify and amplify human speech.
- Envision enhances Google Glass, a wearable augmented-reality display. The company’s technology helps blind and low-vision people interact with their surroundings by highlighting certain objects in a user’s field of view, providing audio descriptions, and reading text.
Why it matters: Some 13.7 percent of American adults who have a disability have serious trouble walking up and down stairs, according to the United States Centers for Disease Control and Prevention. Devices like Neural Sleeve may enable many of these people to move more freely and effectively.
We’re thinking: Neural Sleeve partnered with a design studio to enhance the system’s appeal to users. This sort of collaboration can be very helpful when deploying systems — especially those involved in highly personal activities like therapy — in the real world.
A MESSAGE FROM DEEPLEARNING.AI
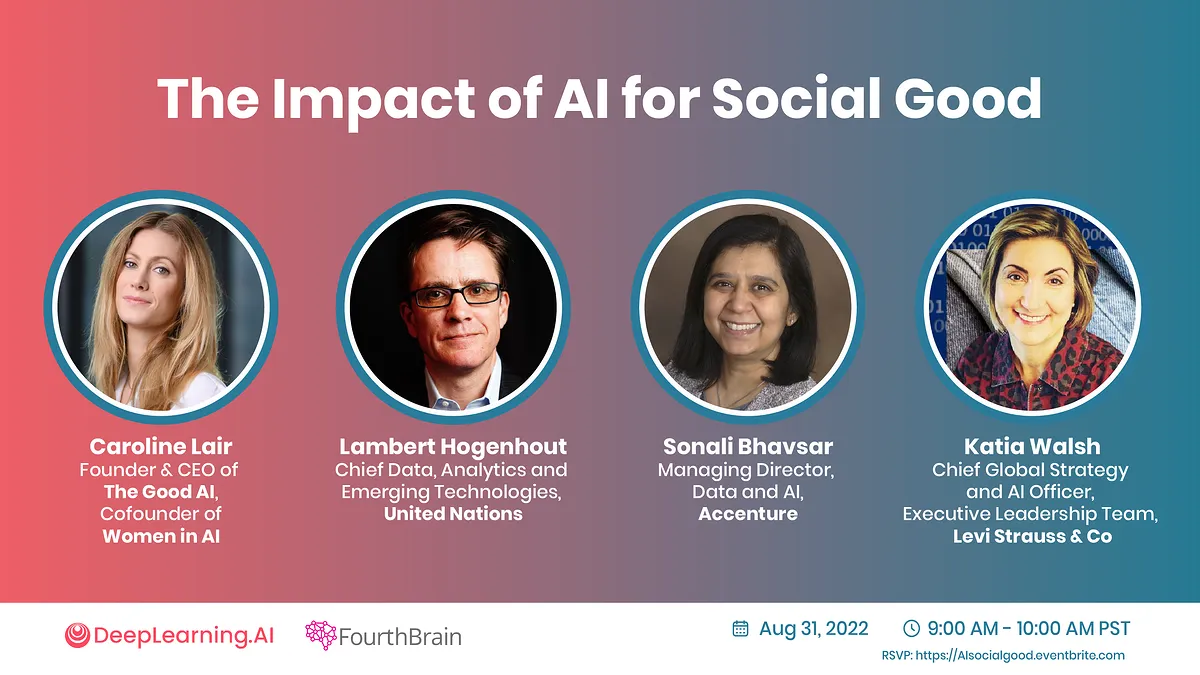
Are you ready to use AI for social good? Advances in AI offer opportunities to tackle important environmental, public health and socioeconomic issues. Learn how! Join us on August 31, 2022, at 9:00 a.m. Pacific Time for “The Impact of AI for Social Good.” RSVP
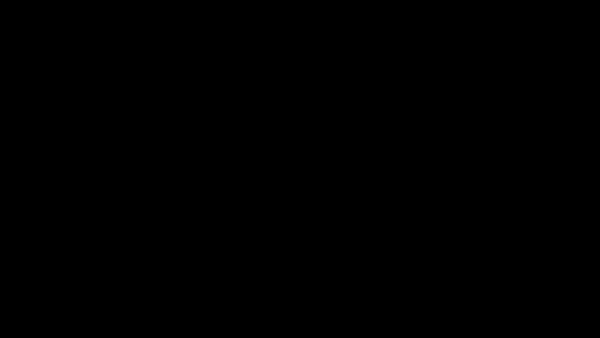
Deepfakes Against Profanity
Deepfake technology enabled a feature film to reach a broader audience.
What’s new: Fall, a thriller about two friends who climb a 2,000-foot tower only to find themselves trapped at the top, originally included over 30 instances of a certain offensive word. The filmmakers deepfaked the picture to clean up the language, enabling the film to earn a rating that welcomes younger viewers, Variety reported.
How it works: Director and co-writer Scott Mann re-recorded the film’s actors reciting more family-friendly versions of the troublesome word. Then he used a generative adversarial network to regenerate the actors’ lip motions to match the revised dialog.
- Built by London-based Flawless AI, where Mann is co-CEO, the system combined an image of the actor’s face from the original film with estimated lip motion based on the re-recorded words. The company developed it to alter lip motion in movies whose dialog was dubbed into foreign language.
- The process of revising the off-color language added two weeks to the film’s post-production schedule.
- Following the revisions, the Motion Picture Association changed the film’s rating from R, which requires audience members under 17 years old to be accompanied by an adult, to PG-13, which is open to all ages.
Behind the news: Neural networks are increasingly common in the edit suite.
- Director Peter Jackson used neural networks to isolate dialogue in footage of the Beatles for his 2021 documentary Get Back.
- In the 2021 biopic Roadrunner, filmmaker Morgan Neville synthesized the voice of the deceased celebrity chef Anthony Bourdain. The generated voice recited quotations from emails the chef wrote before his death.
- The English-language release of the 2020 Polish film Mistrz (The Champion) used a neural network from Tel Aviv-based Adapt Entertainment to adjust actors’ lips to dubbed audio.
Why it matters: Fall’s distributor Lionsgate determined that the movie would make more money if it was aimed at a younger audience. However, reshooting the offending scenes might have taken months and cost millions of dollars. AI offered a relatively affordable solution.
We’re thinking: The global popularity of shows like Squid Game, in which the original dialog is Korean, and La Casa de Papel, in which the actors speak Spanish, suggest that dialog replacement could be a blockbuster AI application.
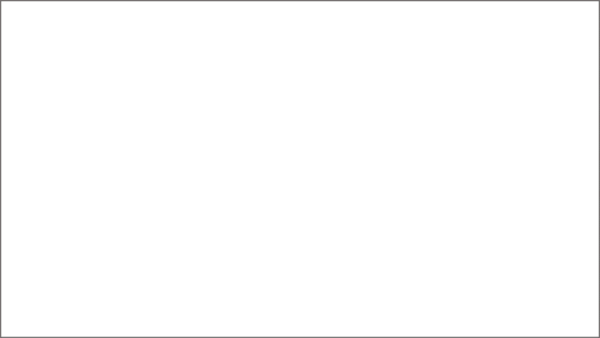
Ensemble Models Simplified
Why build an ensemble of models when you can average their weights?
What’s new: A model whose weights were the mean of an ensemble of fine-tuned models performed as well as the ensemble and better than its best-performing constituent. Mitchell Wortsman led colleagues at University of Washington, Tel Aviv University, Columbia University, Google, and Meta to build this so-called model soup.
Key insight: When fine-tuning a given architecture, it’s common to try many combinations of hyperparameters, collect the resulting models into an ensemble, and combine their results by, say, voting or taking an average. However, the computation and memory requirements increase with each model in the ensemble. Averaging the fine-tuned weights might achieve similar performance without the need to run several models at inference.
How it works: Â The authors investigated model soups based on 72 pre-trained CLIP models that were fine-tuned on ImageNet.
- The authors fine-tuned the models by varying hyperparameters including data augmentations, learning rates, lengths of training, label smoothing (which tempers a model’s response to noisy labels by adding noise), and weight decay (which helps models generalize by encouraging weights to be closer to zero during training).
- They sorted the fine-tuned models according to their accuracy on the validation set.
- Starting with the best-performing model, they averaged its weights with those of the next-best performer. If performance improved, they kept the averaged weights; otherwise, they kept the previous weights. They repeated this process for all fine-tuned models.
Results: The authors’ model achieved 81.03 percent accuracy on ImageNet, while an ensemble of the 72 fine-tuned models achieved 81.19 percent and the single best-performing model achieved 80.38 percent. Testing the ability to generalize to a number of shifted distributions of ImageNet, the authors’ model achieved 50.75 percent average accuracy, the ensemble 50.77 percent, and the best model 47.83 percent.
Why it matters: When training models, it’s common to discard weaker models or build an ensemble. The model-soup method puts that effort into better performance without costing computation or memory at inference.
We're thinking: Averaging weights across various numbers of training steps increased performance in prior work. It's good to find that this method extends to different training runs.