Dear friends,
The rapid rise of AI has led to a rapid rise in AI jobs, and many people are building exciting careers in this field. A career is a decades-long journey, and the path is not always straightforward. Over many years, I’ve been privileged to see thousands of students as well as engineers in companies large and small navigate careers in AI. In this and the next few letters, I’d like to share a few thoughts that might be useful in charting your own course.
Three key steps of career growth are learning (to gain technical and other skills), working on projects (to deepen skills, build a portfolio, and create impact) and searching for a job. These steps stack on top of each other:
- Initially, you focus on gaining foundational technical skills.
- After having gained foundational skills, you lean into project work. During this period, you’ll probably keep learning.
- Later, you might occasionally carry out a job search. Throughout this process, you’ll probably continue to learn and work on meaningful projects.
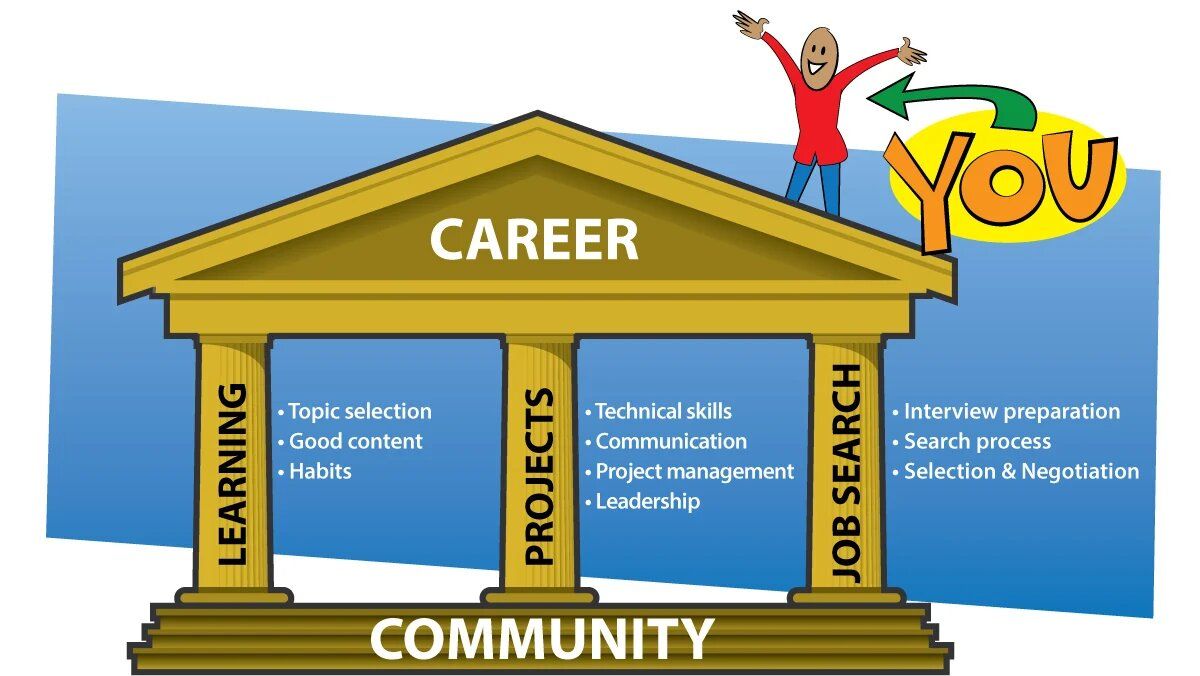
These phases apply in a wide range of professions, but AI involves unique elements. For example:
- AI is nascent, and many technologies are still evolving. While the foundations of machine learning and deep learning are maturing — and coursework is an efficient way to master them — beyond these foundations, keeping up-to-date with changing technology is more important in AI than fields that are more mature.
- Project work often means working with stakeholders who lack expertise in AI. This can make it challenging to find a suitable project, estimate the project’s timeline and return on investment, and set expectations. In addition, the highly iterative nature of AI projects leads to special challenges in project management: How can you come up with a plan for building a system when you don’t know in advance how long it will take to achieve the target accuracy? Even after the system has hit the target, further iteration may be necessary to address post-deployment drift.
- While searching for a job in AI can be similar to searching for a job in other sectors, there are some differences. Many companies are still trying to figure out which AI skills they need and how to hire people who have them. Things you’ve worked on may be significantly different than anything your interviewer has seen, and you’re more likely to have to educate potential employers about some elements of your work.
Throughout these steps, a supportive community is a big help. Having a group of friends and allies who can help you — and whom you strive to help — makes the path easier. This is true whether you’re taking your first steps or you’ve been on the journey for years.
I’m excited to work with all of you to grow the global AI community, and that includes helping everyone in our community develop their careers. I’ll dive more deeply into these topics in the next few weeks.
Keep learning!
Andrew
News
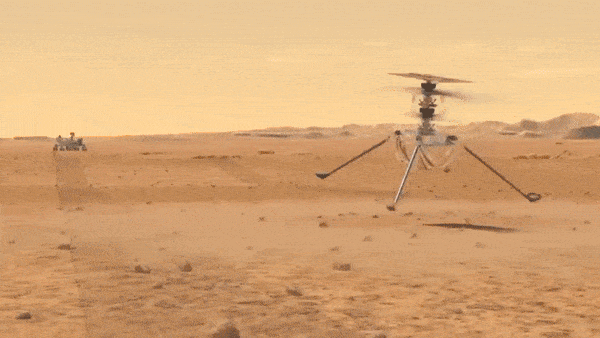
More Autonomy for Martian Drone
The United States space agency is upgrading the system that pilots its helicopter on the Red Planet.
What’s new: The National Aeronautics and Space Administration (NASA) announced that Ingenuity, a drone sent to Mars as part of its 2020 mission to Mars, will receive a new collision-avoidance algorithm, Wired reported. Ingenuity acts as a scout for the Perseverance rover as it travels from relatively flat, featureless areas to more hazardous terrain.
How it works: NASA engineers on Earth plot waypoints in a simulation. They transmit the waypoints to the rover, which relays them to the drone, where algorithms determine its path based on input from an onboard camera, altimeter, and other devices.
- An inertial measurement unit — a collection of gyroscopes and accelerometers — estimates the drone’s orientation and position during the first few seconds of flight, when dust kicked up by the rotors obscures its camera.
- When the camera can see the ground, a learning algorithm detects features in the image and classifies them as stationary or moving.
- A navigation algorithm tracks the craft’s location and velocity based on the stationary objects in view as well as its orientation and altitude.
- Engineers plan to upgrade Ingenuity with an algorithm that will detect hazards on the ground as it lands. The new software will equip the flyer to navigate an ancient river delta studded with cliffs, boulders, and sand traps.
Behind the news: Ingenuity was designed for only five flights, but has flown 29 times since its debut in April 2021. NASA hopes to extend its lifespan even further by letting it hibernate through the Martian winter. Solar energy is scarce for four months starting in July, and hibernation will enable the craft to devote its battery to keeping its electronics warm. The team plans to install the upgrade during that period.
Why it matters: Ingenuity’s evolving combination of Earthbound direction and local autonomy lays the groundwork for missions deeper into the solar system, where the delay in communications — up to 24 minutes between Earth and Mars — will be even longer. For example, the Dragonfly octocopter is scheduled to take off for Titan’s soupy atmosphere in 2027.
We’re thinking: Over-the-air software updates aren’t only for terrestrial devices!
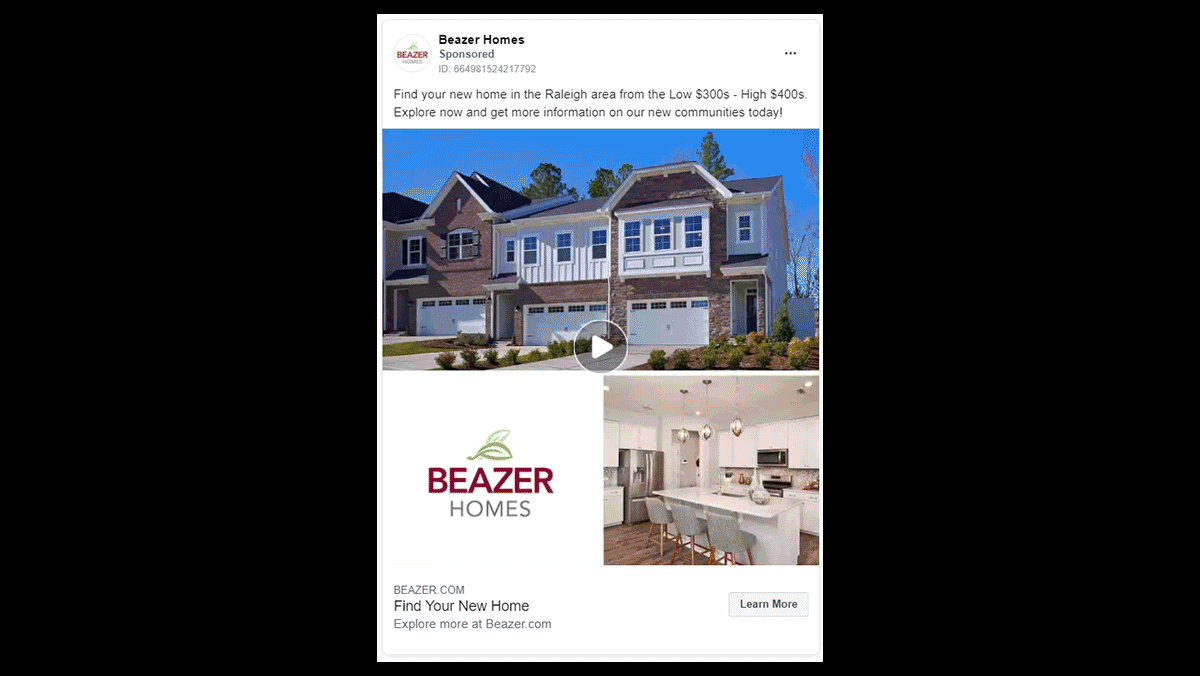
U.S. Acts Against Algorithmic Bias
Regulators are forcing Meta (formerly Facebook) to display certain advertisements more evenly across its membership.
What’s new: The United States government compelled Meta to revise its ad-placement system to deliver ads for housing to members regardless of their age, gender, or ethnicity. The company is voluntarily rebalancing its distribution of ads for credit and employment as well.
How it’s changed: The new algorithm will control ads that appear to U.S. users of Facebook, Instagram, and Messenger. Meta will roll it out by December.
- The company now allows advertisers to define the eligible audience for an ad based on variables like location, interests, and online activity but not age, sex, race, or ethnicity.
- For any given ad, the algorithm periodically monitors and corrects for differences between the actual and eligible audiences. Say, the system serves a housing ad that’s intended for park-going birdwatchers in New York City. If it ends up being viewed only by park-going, bird-watching Latina women in their 40s, the algorithm will retarget it in a way that’s more likely to reach people of other ethnic backgrounds, genders, and ages.
- It assigns a heavier weight to members of the eligible audience who have viewed more ads in the last 30 days. The settlement doesn’t explain the reason for this requirement, which appears to encourage the system to show more ads to more-active users.
- Meta will report the algorithm’s results every four months to the U.S. Justice Department and a third party nominated by the company and approved by the government.
Behind the news: The update is part of a settlement between Meta and the U.S. Justice Department, which found that the company had violated laws against discrimination in housing. Meta also agreed to terminate a different system that was intended to enforce a more even distribution of ads but was found to have the opposite effect. It will pay a fine of $115,054, the maximum penalty under the law.
Why it matters: AI technology is largely unregulated in the U.S. But that doesn’t mean the federal government has no jurisdiction over it, especially when it migrates into highly regulated sectors. Facebook once hosted ads for credit cards that excluded younger people, job postings that excluded women, and housing ads that excluded people by race. Regulators who oversee civil rights didn’t settle for mere changes in Meta’s advertising guidelines and ultimately forced it to alter the algorithm itself.
We’re thinking: Meta’s periodic reports will provide some evidence whether or not regulation can mitigate algorithmic bias. Still, we wonder whether regulators can craft effective rules. Data can be sliced in a variety of ways, and it can be very difficult to detect bias against a particular group within a slice. For example, a system that appears not to discriminate by gender on average may do so, say, within a particular type of town or when handling a certain sort of housing. Given the slow progress of legislation and the rapid development of technology, we worry that regulators will always trail the companies they regulate.
A MESSAGE FROM DEEPLEARNING.AI
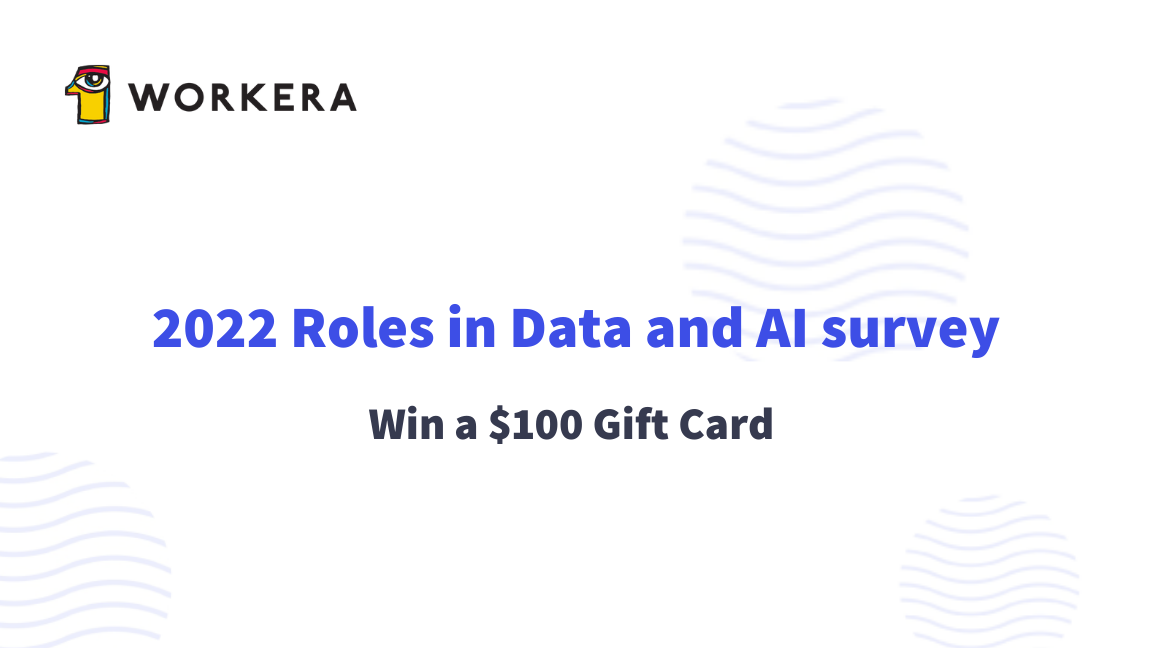
How important is querying databases to your role? Do you use Python or R? Take the Workera 2022 Roles in Data and AI survey and help us upskill the data and AI workforce. Tell us about your role and get a chance to win a $100 gift card!
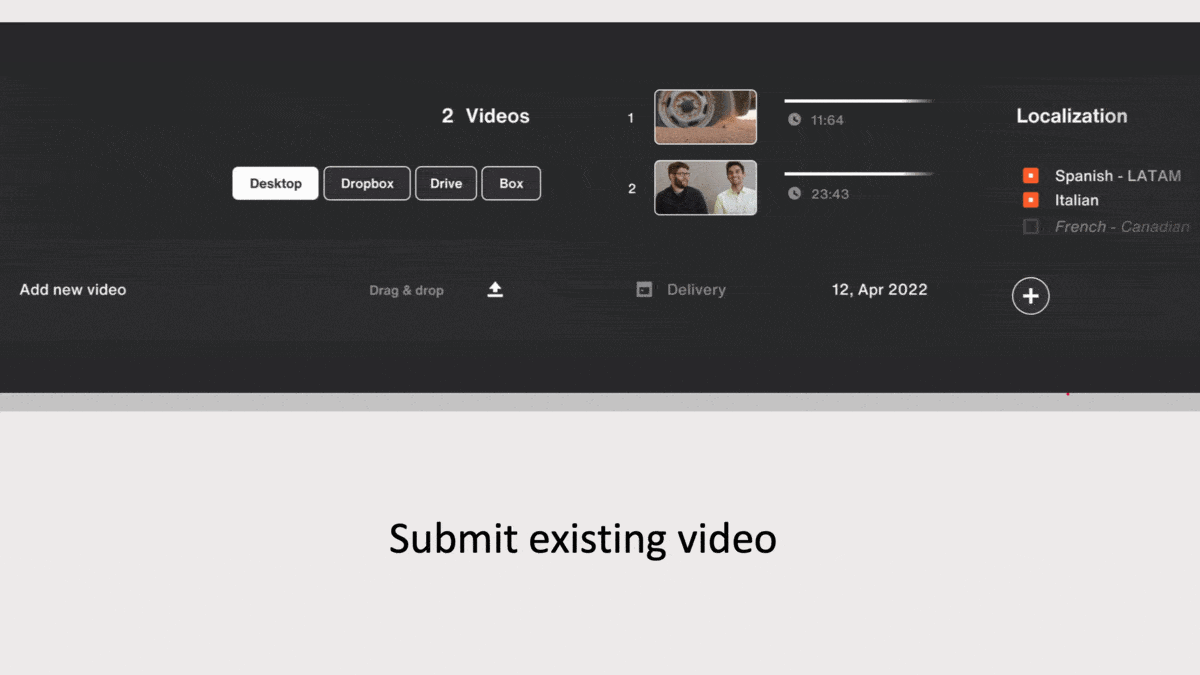
Speaking Your Language
A startup that automatically translates video voice overs into different languages is ready for its big break.
What’s new: London-based Papercup offers a voice translation service that combines algorithmic translation and voice synthesis with human-in-the-loop quality control. A recent funding round suggests that investors have a measure of confidence in the company’s approach.
How it works: Video producers can upload clips and specify an output language such as English, Mandarin, Italian, Latin American Spanish, or Brazilian Portuguese. They can choose among synthesized voices that represent a range of gender and age, and tweak the voice’s pitch and character and alter its emotional expression as “happy,” “sad,” “angry,” and the like.
- Algorithms convert speech into text and translate it into the target language.
- A text-to-speech generator renders the voice over in the new language. It was trained on a combination of third-party and proprietary data.
- A native speaker of the output language checks the result and edits it manually if necessary.
Yes, but: Keeping in a human in the loop to oversee an operation as sensitive as language translation makes good sense. However, current technology can take this automation a good deal further. For instance, Papercup offers a selection of voices rather than generating a facsimile of the original voice in a new language. It doesn’t conform video of the speaker’s mouth to new languages — the mouth continues to form words in one language while the synthesized voice intones another. Nor does it demix and remix vocal tracks that are accompanied by background music or other sounds.
Why it matters: Automated voice over translation is yet another task in which machines are vying to edge out human workers. On one hand, automation can make translation available to producers on a tight budget, dramatically extending their reach to new markets and use cases. On the other hand, we worry that performing artists will lose work to such systems and support efforts to protect their livelihoods.
We’re thinking: Earlier this week, Nando de Freitas — DeepMind research director, Oxford professor, and former officemate of Andrew Ng’s — urged us on Twitter to translate the newly updated Machine Learning Specialization into every language. We're working with Coursera’s global translator community to create subtitles, but we're always eager to have options.
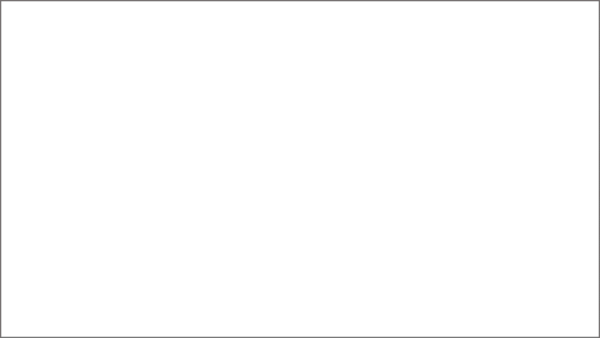
A Transformer for Graphs
Transformers can learn a lot from sequential data like words in a book, but they’ve shown limited ability to learn from data in the form of a graph. A new transformer variant gives graphs due attention.
What's new: Vijay Prakash Dwivedi and Xavier Bresson at Nanyang Technological University devised Graph Transformer (GT), a transformer layer designed to process graph data. Stacking GT layers provides a transformer-based alternative to typical graph neural networks, which process data in the form of nodes and edges that connect them, such as customers connected to products they’ve purchased or atoms connected to one another in a molecule.
Key insight: Previous work applied transformers to graph data by dedicating a token to each node and computing self-attention between every pair. This method encodes both local relationships, such as which nodes are neighbors (given a hyperparameter that defines the neighborhood within a number of degrees of separation), and global information, such as a node’s distance from non-neighboring nodes. However, this approach is prohibitively expensive for large graphs, since the computation required for self-attention grows quadratically with the size of the input. Applying attention only to neighboring nodes captures crucial local information while cutting the computational burden. Meanwhile, a positional vector that represents each node’s relative distance from all other nodes can capture global information in a compute-efficient way.
How it works: The authors built three models, each of which comprised embedding layers, 10 GT layers (including self-attention and fully connected layers) followed by a vanilla neural network. They trained each model on a different task: two-class classification of synthetic data, six-class classification of synthetic data, and a regression task that estimated the solubility of various compounds that contain zinc.
- Given a graph, the embedding layers generated an embedding and positional vector for each node. Using a contrastive approach, it generated similar positional vectors for nearby nodes and dissimilar positional vectors for distant nodes. It added the embedding and positional vector to form a node representation.
- The GT layer honed each node representation by applying self-attention between it and its neighbors. Then it passed the node representation to the fully connected layer.
- The model executed these steps through 10 layers and delivered the final representations to the vanilla neural network, which performed classification or regression.
Results: The authors’ model achieved 73.17 percent accuracy and 84.81 percent accuracy on the two- and six-class classification tasks, respectively. A baseline GAT graph neural network, which applied attention across neighboring node representations, achieved 70.58 percent accuracy and 78.27 percent accuracy respectively. On the regression task, the authors’ model achieved mean absolute error (MAE) of 0.226 compared to GAT’s 0.384 (lower is better). However, it slightly underperformed the state-of-the-art Gated Graph ConvNet in all three tasks.
Why it matters: Transformers have proven their value in processing text, images, and other data types. This work makes them more useful with graphs. Although the Graph Transformer model fell short of the best graph neural network, this work establishes a strong baseline for further work in this area.
We're thinking: Pretrained and fine-tuned transformers handily outperform trained convolutional neural networks. Would fine-tuning a Graph Transformer model yield similarly outstanding results?