Dear friends,
A Google Engineer recently announced he believes that a language model is sentient. I’m highly skeptical that any of today’s AI models are sentient. Some reporters, to their credit, also expressed skepticism. Still, I worry that widespread circulation of sensationalistic reports on this topic will mislead many people. (You'll find more about it in this issue of The Batch.)
The news does raise an interesting question: How would we know if an AI system were to become sentient?
As I discussed in an earlier letter, whether an AI system is sentient (able to feel) is a philosophical question rather than a scientific one. A scientific hypothesis must be falsifiable. Scientific questions about AI include whether it can beat a human chess champion, accurately translate language, drive a car safely, or pass the Turing Test. These are testable questions.
On the other hand, we have no clear test for whether a system is sentient, conscious (aware of its internal state and external surroundings), or generally intelligent (able to reason across a wide variety of domains). These questions fall in the realm of philosophy instead of science.
Here are some examples of philosophical questions. Even though we haven't devised ways to quantify many of these terms, these questions are enduring and important:
- Is the nature of humankind good or evil?
- What is the meaning of life?
- Is a tree/insect/fish conscious?
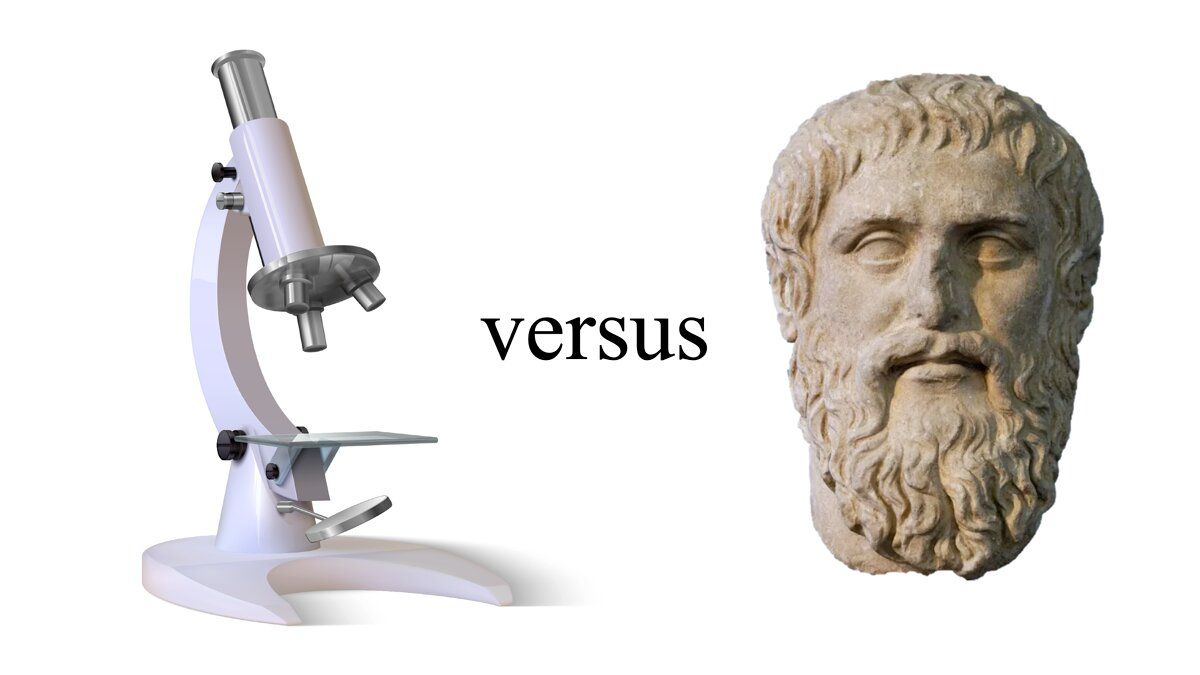
By the same token, many important questions that arise in discussions about AI are philosophical:
- Can AI be sentient? Or conscious?
- Can an AI system feel emotions?
- Can AI be creative?
- Can an AI system understand what it sees or reads?
I expect that developing widely accepted tests for things like sentience and consciousness would be a Herculean, perhaps impossible, task. But if any group of scientists were to succeed in doing so, it would help put to rest some of the ongoing debate.
I fully support work toward artificial general intelligence (AGI). Perhaps a future AGI system will be sentient and conscious, and perhaps not — I’m not sure. But unless we set up clear benchmarks for sentience and consciousness, I expect that it will be very difficult ever to reach a conclusion on whether an AI system has reached these milestones.
Keep learning!
Andrew
P.S. The new Machine Learning Specialization (MLS), which I teach, has just been released on Coursera. It’s a collaboration between DeepLearning.AI and Stanford Online. Thank you for helping me spread the word and encouraging others to take the MLS!
News
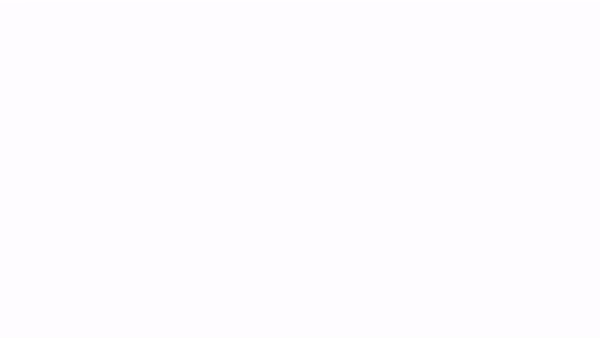
LaMDA Comes Alive?
A chatbot persuaded at least one person that it has feelings.
What’s new: A senior engineer at Google announced his belief that the company’s latest conversational language model is sentient. Google put the engineer on administrative leave.
Is there anybody in there? LaMDA is a family of transformer-based models, pretrained to reproduce 1.56 trillion words of dialog, that range in size from 2 billion to 137 billion parameters. Google previously discussed plans to incorporate it into products like Search and Assistant.
- After pretraining, given a prompt, LaMDA generated a number of possible responses. The developers collected a set of conversations with LaMDA and hired people to rate how sensible, specific, interesting, and safe its responses were. Then they fine-tuned the model to generate those ratings at the end of each response. LaMDA replies with the highest-rated response. To further improve its factual output, they fine-tuned it to mimic the hired workers’ searches and, in such cases, direct an external system to search rather than return the response. Given the previous input and new search results, the model produces new output — which may be a new response or a further search.
- Researchers in Google’s Responsible AI group tested chatbots based on LaMDA to determine their propensity for hate speech and other toxic behavior. The process persuaded researcher Blake Lemoine that the model possessed self-awareness and a sense of personhood. He transcribed nine conversations between the model and Google researchers and submitted an argument that LaMDA is sentient. In one transcript, a chatbot says it believes it’s a person, discusses its rights, and expresses a fear of being turned off.
- Google placed Lemoine on administrative leave in early June for violating confidentiality by hiring a lawyer to defend LaMDA’s right to exist and speaking to a member of the U.S. House Judiciary Committee about what he regarded as ethical violations in Google’s treatment of the LaMDA. “Lemoine was told that there was no evidence that LaMDA was sentient (and lots of evidence against it),” a company spokesperson told The Washington Post.
- In a blog post, Lemoine writes that Google’s decision to discipline him follows a pattern of unfair treatment by the company towards its ethics researchers, charging that the company disregards the concerns of ethics researchers and punishes them for speaking up. He cites the company’s earlier dismissal of Ethical AI co-leads Timnit Gebru and Margaret Mitchell.
What they’re saying: Many members of the AI community expressed skepticism of Lemoine’s claims via social media. Melanie Mitchell, professor at the Santa Fe Institute, said, “It's been known for forever that humans are predisposed to anthropomorphize even with only the shallowest of signals…Google engineers are human too, and not immune.”
Why it matters: The propensity to anthropomorphize machines is so strong that it has a name: The Eliza Effect, which refers to a mid-1960s chatbot that persuaded some patients that it was a human psychotherapist. Beyond that, the urge to fall in love with one’s own handiwork is at least as old as the ancient Greek story of Pygmalion, a fictional sculptor who fell in love with a statue he created. We must strive to strengthen our own good judgment even as we do the same for machines.
We’re thinking: We see no reason to believe that LaMDA may be sentient. While such episodes are a distraction from the important work of harnessing AI to solve serious, persistent problems (including machine sentience), they are a reminder to approach extraordinary claims with appropriate skepticism.
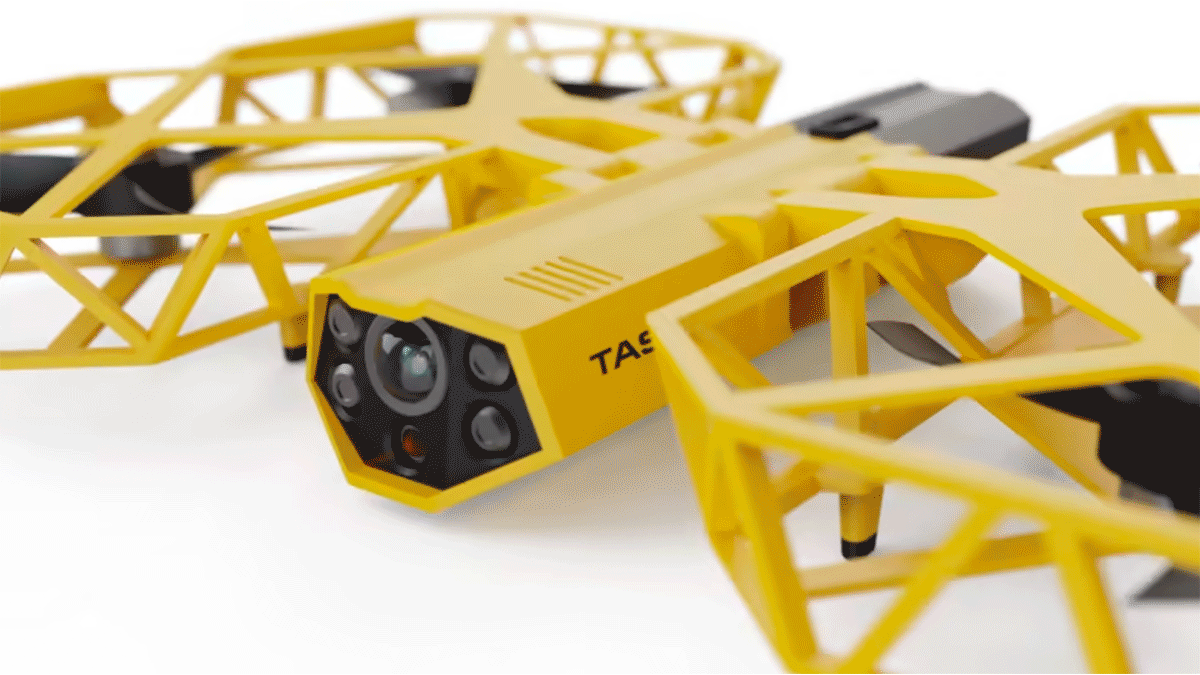
Ethics Team Scuttles Taser Drone
A weaponized AI system intended to protect students has been grounded.
What’s new: Axon, which makes law-enforcement equipment such as tasers and body cameras, canceled a plan to sell remote-controlled drones capable of firing electroshock darts to incapacitate attackers at schools, businesses, and other public places. The company, which had announced the taser drone in early June, shelved it days later after the majority of its independent ethics board resigned in protest.
How it works: The canceled flier, which was based on the company’s existing Axon Air surveillance drone, was to include a camera as well as a taser. A human operator would decide when to fire its electroshock projectile.
- The company’s CEO Rick Smith estimated that 50 to 100 drones would equal the cost of a single armed security guard, he said in an Ask Me Anything session on Reddit held on the day the ethics board members resigned.
- In his book The End of Killing and a graphic novel of the same name, Smith explains that the drone would launch automatically when an AI-enabled microphone detected the sound of gunfire. In addition, the system would alert on-site security, administrators, and local law enforcement.
- Nine members of Axon’s ethics board released a statement opposing the plan, saying it “has no realistic chance of solving the mass shooting problem” that has afflicted U.S. schools in recent decades. They criticized the drone’s surveillance capability in particular, stating that it “will harm communities of color and others who are overpoliced, and likely well beyond that.”
Behind the news: Axon’s announcement came about a week after a gunman killed 19 students and two teachers at an elementary school in Texas. It was the 27th school shooting with casualties in the U.S. in 2022.
Why it matters: The U.S. public is divided on how to address an ongoing epidemic of gun violence, with a majority calling for greater safety regulations that would limit who can own a firearm. The opposition, which believes that gun-control measures violate rights guaranteed by the nation’s constitution, favors solutions like armed guards and surveillance — proposals that align with Axon’s canceled drone.
We’re thinking: Technological countermeasures are appealing in the face of repeated attacks on schools, workplaces, hospitals, and other public spaces. However, research argues against increased security in favor of better safety regulations. Axon should have consulted its ethics committee before announcing the product, but it did the right thing by canceling it afterward.
A MESSAGE FROM DEEPLEARNING.AI
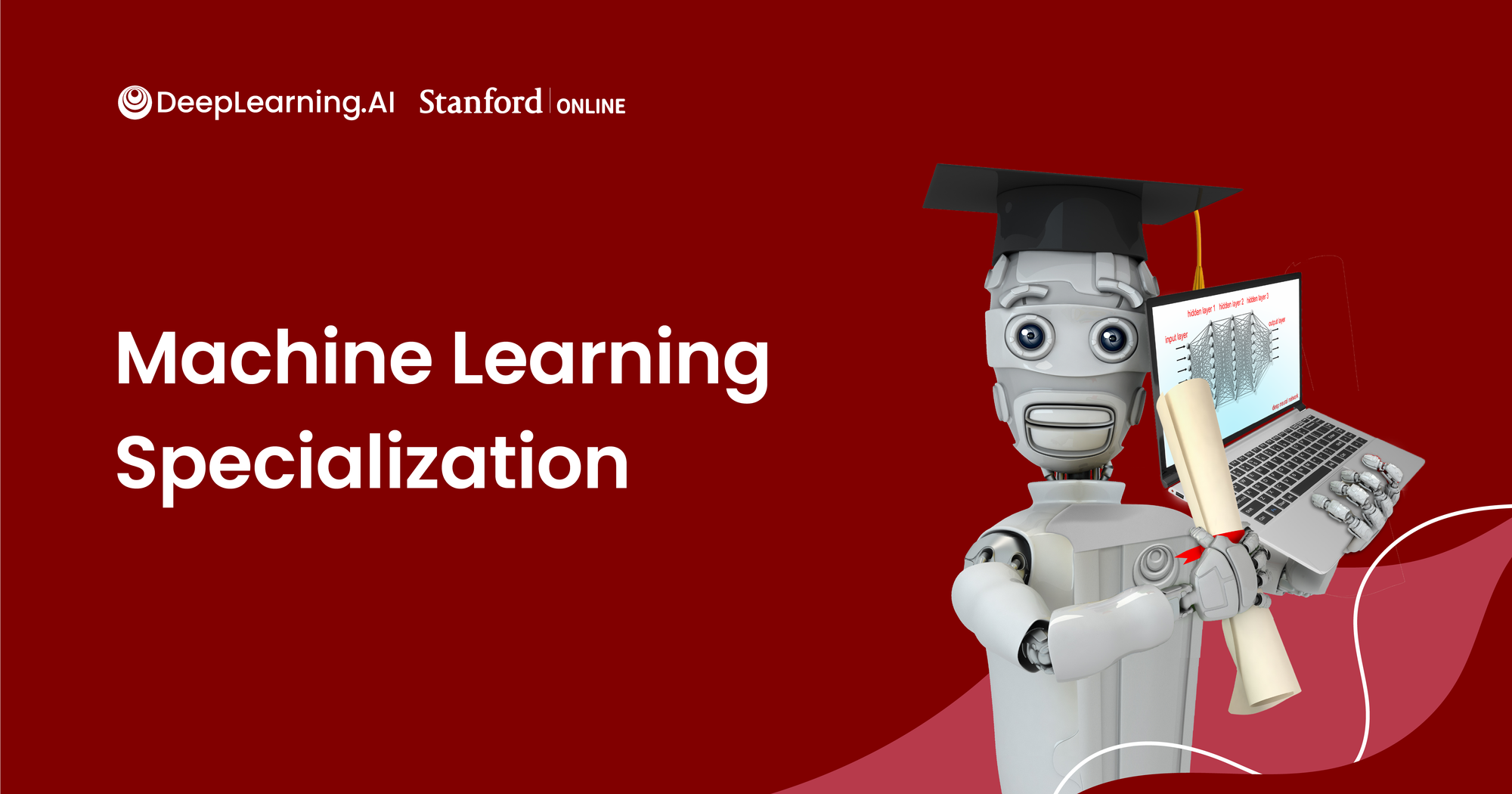
Today is the day! Our brand-new Machine Learning Specialization, created in collaboration with Stanford Online, is live on Coursera! If you want to #BreakIntoAI, now is the time to take the first step. Enroll now
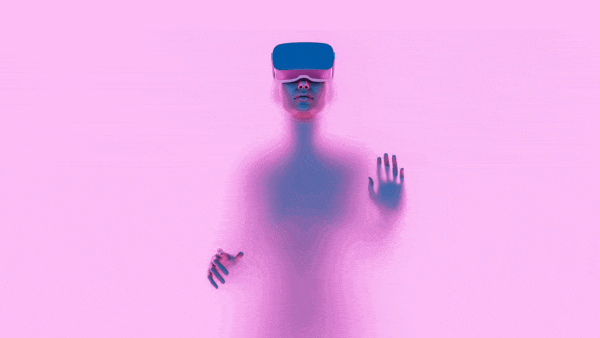
Meta Decentralizes AI Effort
The future of Big AI may lie with product-development teams.
What’s new: Meta reorganized its AI division. Henceforth, AI teams will report to departments that develop key products.
How it works: Prior to the reshuffle, the company’s Responsible AI, AI for Products, AI4AR (that is, for augmented reality), and Facebook AI Research teams were managed by a single division called Meta AI. This structure made it difficult to translate machine learning into marketable applications, according to chief technology officer Andrew Bosworth.
- Responsible AI, which aims to mitigate bias in the company’s models, will report to Social Impact, which develops tools to help nonprofits use Meta’s social media platform.
- AI for Product, which develops applications for advertising and recommendation, will join the product engineering team.
- AI4AR, which develops augmented- and virtual-reality tools like Builder Bot, will join Meta’s Reality Labs as part of the XR (an acronym for extended reality) team, which oversees technologies like Spark AR and Oculus headsets.
- Facebook AI Research, led by Antoine Borges, Joelle Pineau, and Yann LeCun, will also report to Reality Labs. In addition, Pineau will lead a new team that assesses company-wide progress on AI.
- Jerome Pesenti, Facebook and Meta’s vice president of AI since 2018, will depart the company in mid-June.
Shaky platform: AI teams who work for Meta’s flagship Facebook social platform have had a rocky few years.
- Last year, a former product manager leaked documents to the press showing that the company knowingly tweaked its recommendation algorithm in ways that harmed both individuals and society at large.
- In 2020, reports surfaced that company leadership had blocked internal efforts to reduce the amount of extreme content the algorithm promoted over concerns that doing so would drive down profits.
- In 2018, Joaquin Quiñonero Candela, an architect of Facebook’s recommendation algorithm, took charge of the Responsible AI division to mitigate the algorithm’s propensity to promote disinformation, hate speech, and other divisive content. (Candela departed in 2021.)
Trend in the making? Meta isn’t the first large company to move AI teams closer to its product groups.
- Last year, Microsoft moved its data and AI units under the umbrella of its Industries and Business Applications group. In 2018, Microsoft had integrated AI research more closely with its cloud computing business.
- In 2018, Google absorbed its DeepMind division’s healthcare unit with the goal of translating applications, such as the Streams app that alerts caregivers to concerning test results, into clinical practice.
Why it matters: In 2019, 37 percent of large AI companies maintained a central AI group, The Wall Street Journal reported. Reorgs by Meta and others suggest that centralization hindered their ability to capitalize on AI innovations.
We’re thinking: In a corporate setting, when a technology is new, a centralized team can make it easier to share learnings, set standards, and build company-wide platforms. As it matures, individual business units often gain the ability to manage the technology themselves and absorb experienced developers. Apparently this pattern — which we describe in AI For Everyone — is playing out in some leading AI companies.
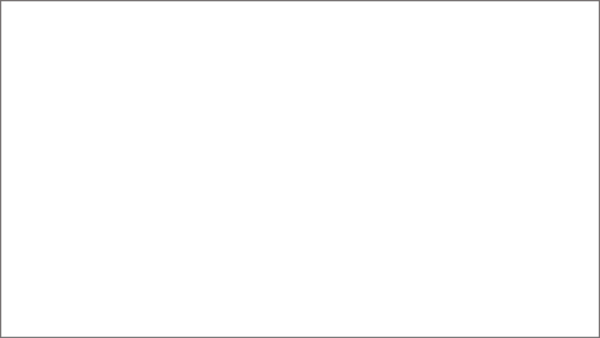
Airfoils Automatically Optimized
Engineers who design aircraft, aqueducts, and other objects that interact with air and water use numerical simulations to test potential shapes, but they rely on trial and error to improve their designs. A neural simulator can optimize the shape itself.
What’s new: Researchers at DeepMind devised Differentiable Learned Simulators, neural networks that learn to simulate physical processes, to help design surfaces that channel fluids in specific ways.
Key insight: A popular way to design an object with certain physical properties is to evolve it using a numerical simulator: sample candidate designs, test their properties, keep the best design, tweak it randomly, and repeat. Here’s a faster, nonrandom alternative: Given parameters that define an object’s shape as a two- or three-dimensional mesh, a differentiable model can compute how it should change to better perform a task. Then it can use that information to adjust the object’s shape directly.
How it works: Water and air can be modeled as systems of particles. The authors trained MeshGraphNets, a type of graph neural network, to reproduce a prebuilt simulator’s output. The networks were trained to simulate the flow of particles around various shapes by predicting the next state given the previous state. The MeshGraphNets’ nodes represented particles, and their edges connected nearby particles.
- They trained one network to simulate the flow of water in two dimensions and used it to optimize the shapes of containers and ramps. They trained another to simulate water in three dimensions and used it to design surfaces that directed an incoming stream in certain directions. They trained the third on the output of an aerodynamic solver and used it to design an airfoil — a cross-section of a wing — to reduce drag.
- Given a shape’s parameters, the trained networks predicted how the state would change over a set number of time steps by repeatedly predicting the next state from the current one. Then they evaluated the object based on a reward function. The reward functions for the 2D and 3D water tasks maximized the likelihood that particles would pass through a target region of simulated space. The reward function for the aerodynamic task minimized drag.
- To optimize a shape, the authors repeatedly backpropagated gradients from the reward function through the network (without changing it) to the shape, updating its parameters.
Results: Shapes designed using the authors’ approach outperformed those produced by the cross-entropy method (CEM), a technique that samples many designs and evolves them to maximize rewards. In the 2D water tasks, they achieved rewards 3.9 to 37.5 percent higher than shapes produced by CEM using the prebuilt simulator. In the aerodynamic task, they achieved results similar to those of a highly specialized solver, producing drag coefficients between 0.01898 and 0.01919 compared to DAFoam’s 0.01902 (lower is better).
We’re thinking: It’s not uncommon to train a neural network to mimic the output of a computation-intensive physics simulator. Using such a neural simulator not to run simulations but to optimize inputs according to the simulation’s outcome — that’s a fresh idea.