Dear friends,
AI Fund, which I lead, is a venture studio that works with entrepreneurs to build companies rapidly and increase their odds of success. We’ve evaluated a lot of AI startup ideas. There’s no one-size-fits-all template for building businesses, but we’ve fine-tuned our recipes. In this and subsequent letters, I’ll share some of the patterns I’ve seen.
AI businesses differ from traditional software startups in important ways. For instance, technical feasibility isn’t always clear, product specification is complex, and data is necessary to train and test the system.
One important factor is whether a startup focuses on hard tech (sometimes called deep tech). A hard-tech company:
- Relies on advanced, better-performing technology that significantly improves the customer experience or business efficiency.
- Requires highly skilled teams that are capable of building materially better technology.
In determining whether a business requires hard tech, the key factor is whether best-in-class technology will make the difference between success and failure.
For instance, speech recognition based on deep learning was hard tech 10 years ago. Only a handful of teams were able to build highly accurate systems and put them into production at scale. Higher accuracy greatly improved the user experience, and that drove adoption. Competitors had a hard time catching up.
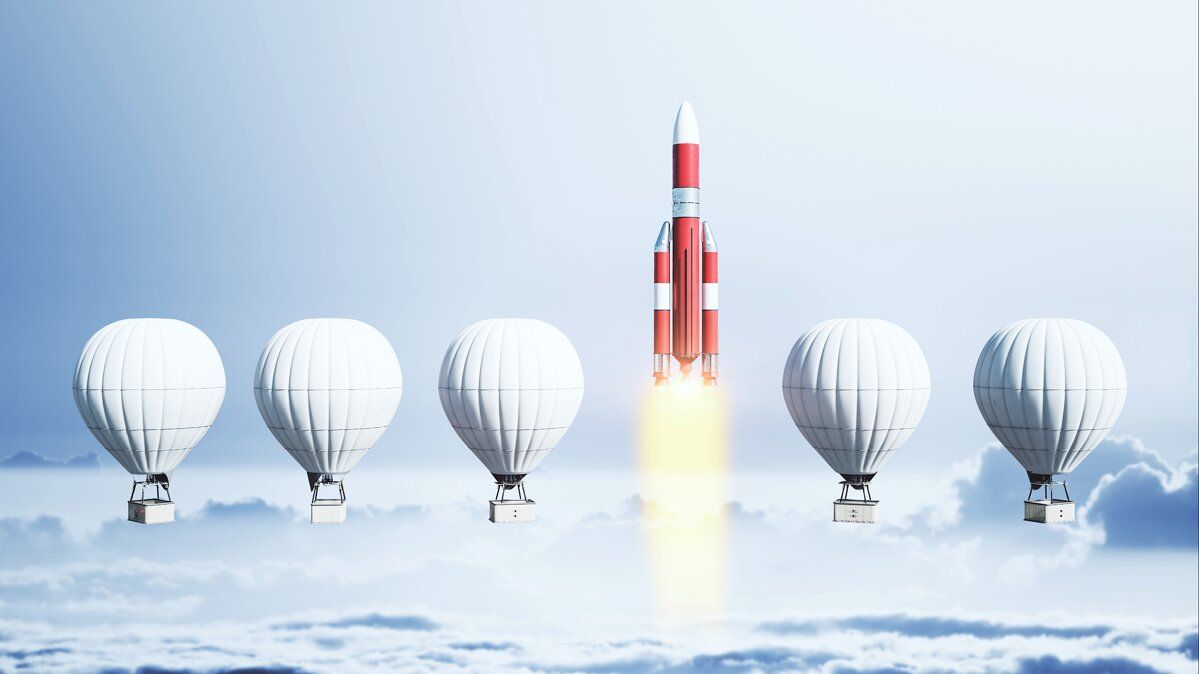
Another example is online advertising. Building a system that selects the most relevant ad within hundreds of milliseconds is very challenging. Showing better ads results in more revenue per page view. More revenue not only improves the bottom line but makes it possible to afford higher costs to acquire users (say, by paying a maker of web browsers to feature one search engine over another). This, in turn, makes it harder for rivals to compete.
What once was hard tech often becomes easier to build over time. For example, as speech recognition became commoditized, more teams were able to build useful systems. When this happens, having the best tech is much less critical to success. Other factors can have a bigger impact such as superior product design, a skilled sales team, bundling with other services, or an efficient supply chain.
I enjoy working on hard-tech businesses — and many AI Fund companies fit that description — because the quality of the technology really matters. A hard-tech company has an incentive to build the best possible team, because the finest team can significantly outperform competitors.
Of course, AI businesses that aren’t hard-tech can be very meaningful, too. There are many, many exciting applications, across all industries, yet to be built using established technology. We need developers going at these problems, too.
Keep learning!
Andrew
News
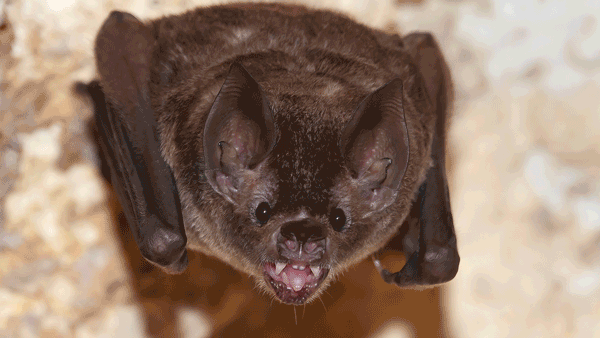
Predicting the Next Pandemic
Odds are that the next mass contagion will jump to humans from animals. But which species?
What’s new: Virus hunters are using learning algorithms to learn which animals are likely to carry microbes that pose a danger to humans, The New York Times reported.
How it works: Several systems trained on biological, ecological, and genetic data have shown promise in identifying sources of interspecies infection.
- In 2022, researchers at nearly a dozen institutions trained an ensemble of eight models to classify bat species that are likely to host coronaviruses similar to the one that causes Covid-19. The architectures included k-nearest neighbors and a gradient boosted machine. The training data included a database of bat traits and a graph dataset of 710 animal species and viruses they carry. The system identified 400 bat species as carriers of pathogens that might infect humans.
- Last year, researchers at the University of Glasgow trained a gradient boosted machine to identify animal viruses with high risk of infecting humans. The model considered the proportion of human-infecting variants in a given virus family, features of carrier species, and features of viral genomes. It identified 313 potentially dangerous animal viruses.
- Those studies build on 2015 work at Princeton and University of Georgia, where researchers trained a gradient boosted machine to classify whether a given rodent species is likely to carry pathogens that can infect humans. The data included a dataset that detailed 86 traits of rodent species and another that cataloged rodent-borne viruses known to infect humans. The model pointed to 58 species previously not considered threatening that may harbor dangerous diseases and 159 likely to carry multiple diseases that previously were believed to carry just one.
Behind the news: The AI community isn’t just working to predict future pandemics, it’s also fighting the current one.
- Covid Moonshot, a global collaboration of over 150 scientists and machine learning engineers, designed multiple antiviral drugs to target the virus that causes Covid-19. Clinical trials are expected to begin this year.
- Researchers at MIT trained a language model to predict genetic mutations that would increase the infectiousness of the virus that causes Covid-19.
- Pharmaceutical giant Pfizer accelerated development of its Covid-19 vaccine by a month by using a machine learning tool called Smart Data Query to analyze clinical trial data.
- Despite efforts to build models capable of diagnosing and prognosticating Covid-19 from medical images, a 2021 survey found that none of the proposed models was clinically useful owing to biases or flaws in methodology.
Why it matters: Ebola, HIV, swine flu — many dire human diseases evolved in animals. Using AI to identify viruses likely to cross the species barrier could give scientists a jump on whatever comes next. Medical researchers could develop vaccines and treatments ahead of time, and officials could mitigate the spread of potentially dangerous disease by managing animal populations and limiting trade in disease-carrying species.
We’re thinking: Whether an animal virus can infect a human is one question. Whether it can cause a pandemic is another. Machine learning engineers have an opportunity to help answer that one as well.
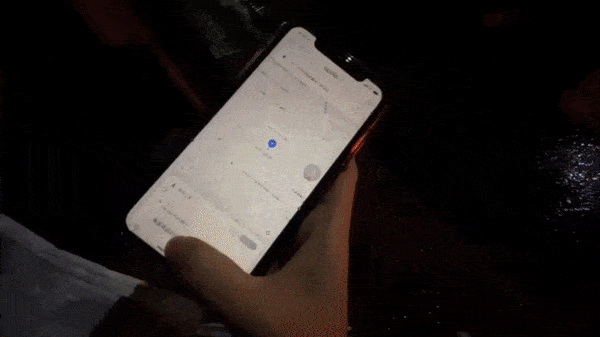
Autonomy Becomes Autonomous
In Beijing, self-driving cars are rolling without a driver behind the wheel.
What’s new: China’s capital city authorized Pony.ai and Apollo Go, a Baidu subsidiary, to deploy self-driving taxis without a human in the driver’s seat, Reuters reported. An authorization issued last November had allowed the companies to operate in the same area with safety drivers.
How it works: The robotaxis are restricted to a 23 square-mile zone in Beijing’s southeastern suburbs, home to roughly 300,000 residents. Rides are free, but both companies plan to charge in the near future.
- The cars will operate between 9 a.m. and 4 p.m. — unlike the night-time scenes depicted in the promotional clips like the one from Apollo Go shown above.
- The taxis are permitted to pick up and drop off passengers only at specific locations. This eliminates the need for customers to find cars they ordered, and for cars to find the customers who ordered them.
- Although the cars can operate without a safety driver, they must carry a human supervisor.
Behind the news: Autonomous taxis — with safety drivers — are operating in a number of Chinese cities including Shanghai and Shenzhen. All except Pony.ai have provisional licenses that require them to provide free rides. In April, Pony.ai received the country’s first authorization to charge robotaxi passengers.
Why it matters: Permission to operate autonomous vehicles in Beijing, which is home to over 20 million people, is a huge market opportunity. Permission to do it without safety drivers likely will represent a huge cost saving if the government relaxes the requirement to carry a supervisor. But the symbolism is an even bigger deal: If robotaxis can handle Beijing traffic, they may be ready for wider adoption. (Then again, gridlock isn’t the most challenging condition for an autonomous vehicle.)
We’re thinking: Safe adoption of self-driving cars still requires limitations on their range and cooperation with governments. Countries that aim to accelerate progress in this area should help entrepreneurs deploy vehicles in a relatively self-contained region and expand from there.
A MESSAGE FROM DEEPLEARNING.AI
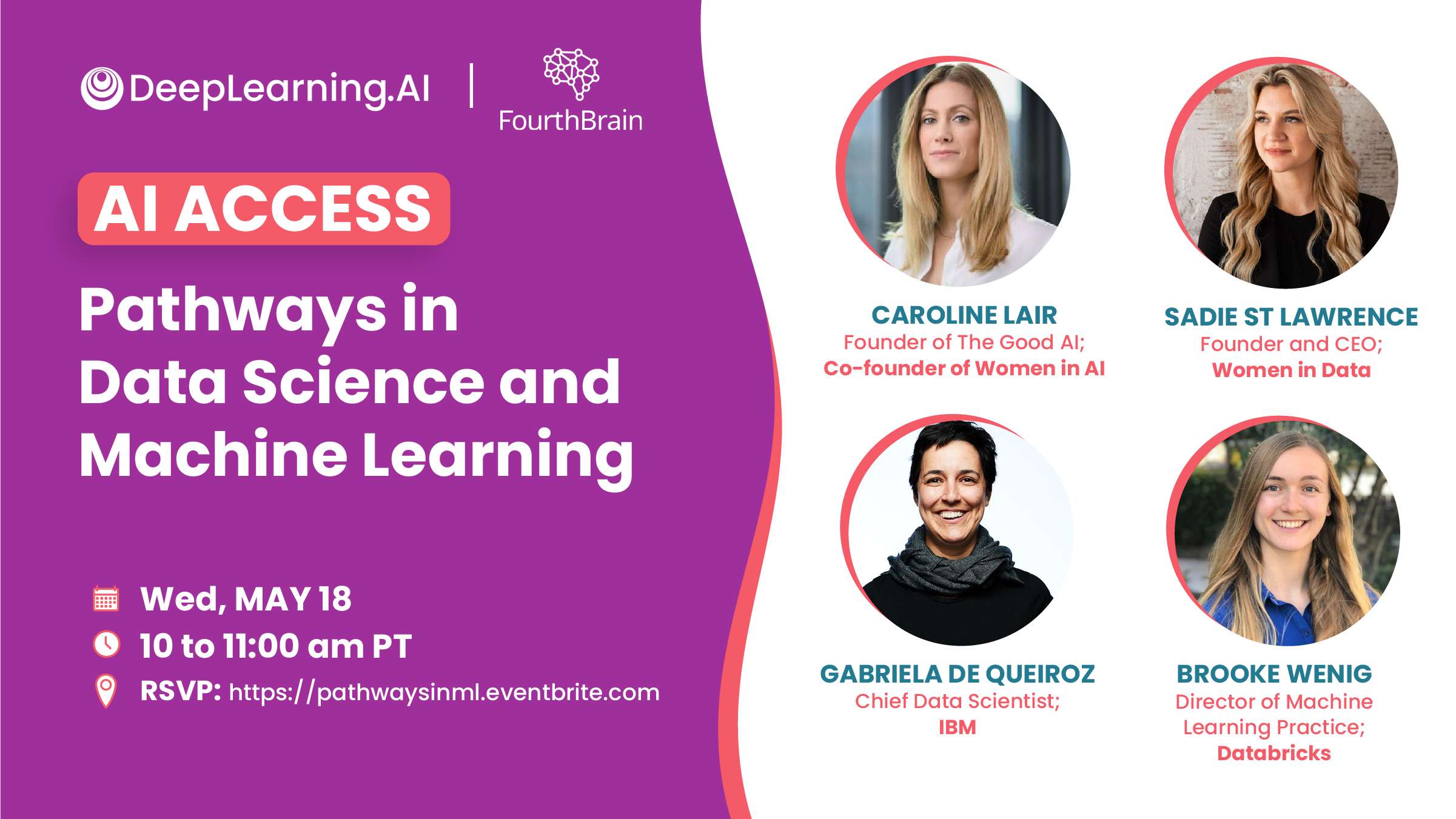
Considering a career in data science or machine learning? Join us on May 18, 2022, to hear from industry leaders about their experiences. Every story is different, and everyone’s journey is unique. Take the next step now!
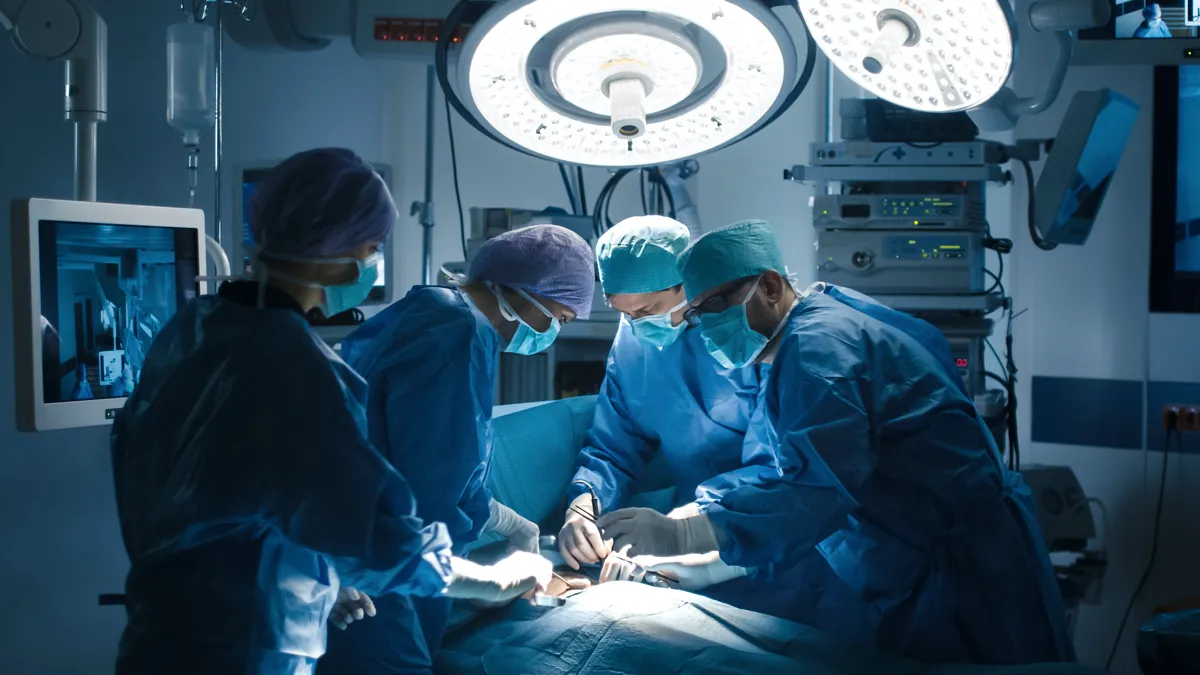
Managing Medical Uncertainty
Hospitals across the United States are relying on AI to keep patients safe.
What’s new: Doctors are using a variety of machine learning systems to assess the risk that a given patient will suffer complications, The Wall Street Journal reported.
How it works: Several facilities are using AI to identify patients who need special attention.
- Duke University Hospital uses Sepsis Watch to monitor every patient in its emergency room for acute inflammation in response to infection, which is responsible for one in three hospital deaths. Every five minutes, the system analyzes 86 variables and assigns a risk score, alerting nurses only when it passes a certain threshold.
- Kaiser Permanente deployed Advanced Alert Monitor in 21 of its hospitals after finding that it shortened hospital stays and reduced referrals to intensive care units. The system predicts whether patients will require intensive care within 12 hours based on vital signs, laboratory test results, coexisting conditions, and other factors.
- Doctors at the University of Maryland Medical System found that a machine learning model outperformed traditional methods at predicting a patient’s risk of returning within 30 days.
Behind the news: Government regulators are beginning to accept machine learning’s potential to transform healthcare.
- Earlier this month, the European Union approved for clinical use an AI system that scans chest x-rays and automatically writes reports for those with no discernable maladies.
- In October 2021, regulatory agencies in Canada, the United Kingdom, and the United States jointly issued guiding principles for the use of machine learning in medicine.
- In November 2020, the U.S. Medicare and Medicaid programs agreed to reimburse doctors who use two AI-powered tools: Viz LVO, which monitors patients for signs of a stroke, and IDx-DR, which helps diagnose a complication of diabetes that can cause blindness. Medicare and Medicaid approval often enables treatments to reach more patients in the U.S.
Why it matters: The Covid-19 pandemic has highlighted tragically underfunded and overworked healthcare workers around the globe. Automated tools could help providers make better use of limited time and resources and help them to focus their attention on the most important cases.
We’re thinking: Many countries face a demographic cliff: The population of younger people is falling precipitously, while the number of elders is growing. It seems likely that AI will be instrumental in helping doctors care for an aging population with a rising life expectancy.
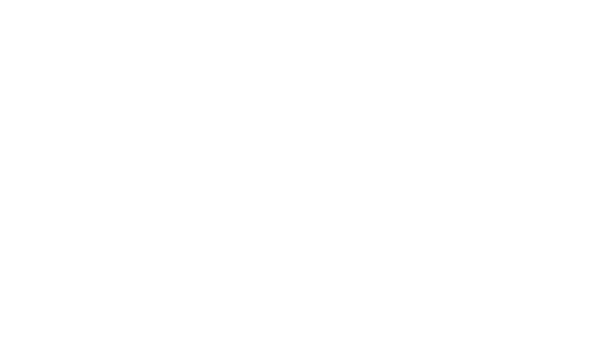
Less Data for Vision Transformers
Vision Transformer (ViT) outperformed convolutional neural networks in image classification, but it required more training data. New work enabled ViT and its variants to outperform other architectures with less training data.
What’s new: Seung Hoon Lee, Seunghyun Lee, and Byung Cheol Song at Inha University proposed two tweaks to transformer-based vision architectures.
Key insight: ViT and its variants divide input images into smaller patches, generates a representation — that is, a token — of each patch, and applies self-attention to track the relationships between each pair of tokens. Dividing an image can obscure the relationships between its parts, so adding a margin of overlap around each patch can help the attention layers learn these relationships. Moreover, an attention layer may fail to distinguish sufficiently between strong and weak relationships among patches, which interferes with learning. For instance, it may weight the relationship between a background patch and a foreground patch only slightly lower than that between two foreground patches. Enabling the attention layers to learn to adjust such values should boost the trained model’s performance.
How it works: Starting with a collection of transformer-based image classifiers, the authors built modified versions that implemented two novel techniques. The models included ViT, T2T, CaiT, PiT, and Swin. They were trained on datasets of 50,000 to 100,000 images (CIFAR-10, CIFAR-100, Tiny-ImageNet, and SVHN) as well as the standard ImageNet training set of 1,281 million images.
- The first modification (shifted patch tokenization, or SPT) created overlap between adjacent patches. Given an image, the model produced four copies, then shifted each copy diagonally in a different direction by half the length of a patch. It divided the image into patches and concatenated the corresponding patches. Given the concatenated patches, it created a representation.
- The second modification (locality self-attention, or LSA) altered the self-attention mechanism. Given the matrix computed by the dot-product between the patches (typically the first step in self-attention), the model masked the diagonal. That is, it set to negative infinity every value that represented the strength of relationships between corresponding patches, causing the model to ignore relationships between them. It also rescaled the matrix using a learned parameter, so the model increased the weight of the closest relationships while decreasing the others.
Results: The alterations boosted the top-1 accuracy of all models on all datasets. They improved the accuracy of PiT and CaiT by 4.01 percent and 3.43 percent on CIFAR100, and the accuracy of ViT and Swin by 4.00 percent and 4.08 percent on Tiny-ImageNet. They improved the ImageNet accuracy of ViT, PiT, and Swin by 1.60 percent, 1.44 percent, and 1.06 percent respectively.
Yes, but: The authors also applied their alterations to the convolutional architectures ResNet and EfficientNet. Only CaiT and Swin surpassed them on CIFAR100 and SVHN. Only CaiT beat them on Tiny-ImageNet. No transformer beat ResNet’s performance on CIFAR10, though all the modified transformers except ViT beat ResNet on the same task.
Why it matters: The authors’ approach makes transformers more practical for visual tasks in which training data is limited.
We’re thinking: Transformers are making great strides in computer vision. Will they supplant convolutional neural networks? Stay tuned!