Dear friends,
Should we be optimistic or pessimistic about the prospects for ethical AI? I meet people who are encouraged by the progress we’ve made toward making AI more responsible and free of bias. I also see people who are dismayed by the daunting challenges we face.
Comparing things today to where they were five years ago, I find ample grounds for optimism in this area. Not long ago, we had barely defined the issues. Today we have numerous tools, publications, and conference sessions devoted to identifying bias and building systems that benefit people broadly. We’ve begun to acknowledge the social disparities that place barriers in front of talented people, and to chip away at them. Many more teams are working on these issues than ever before.
On the other hand, comparing the current state of responsible AI with where we could or should be, I understand why some people are pessimistic. AI systems often reflect pernicious social patterns. Biases infect datasets, which are used to train biased models, which are deployed without adequate auditing, which contribute to denying someone a loan, insurance policy, medical procedure, or release from prison. Far too few teams are addressing these problems effectively.
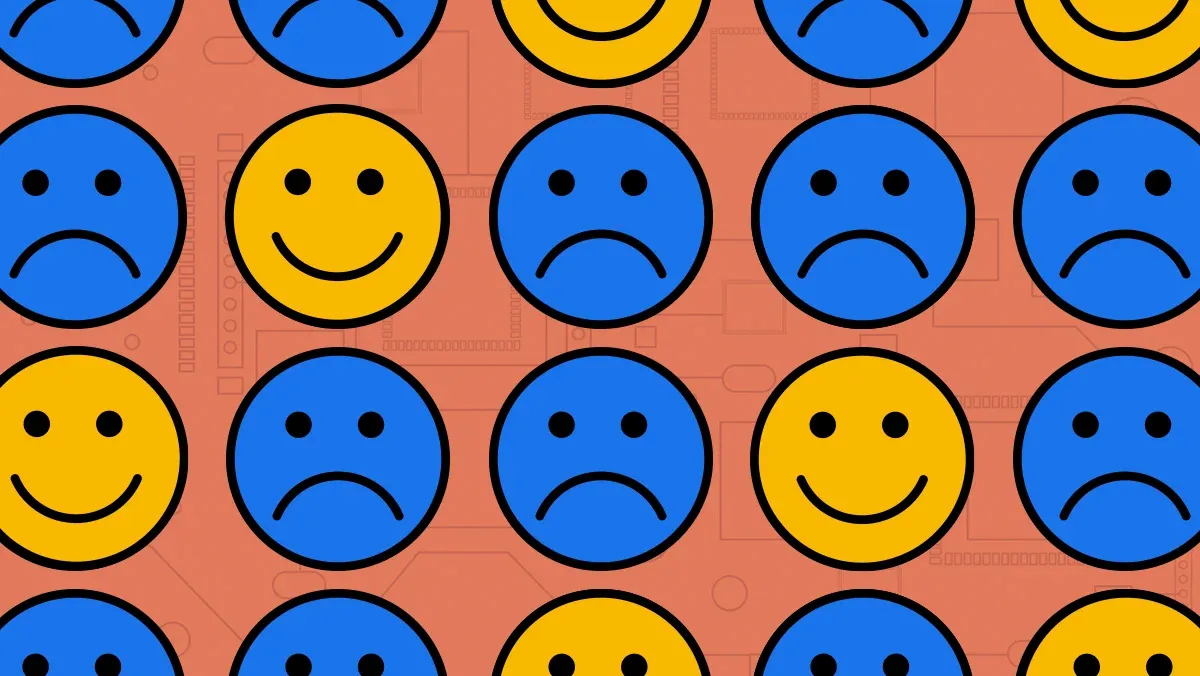
Whether one is an optimist or pessimist often depends on the frame of comparison. Do you compare where we are with how far we’ve come or how far we’ve yet to go? Beyond AI, society has made remarkable progress against racism in the last few decades. Within the past year, the Black Lives Matter movement has raised awareness of racism in the U.S. and George Floyd’s murderer was convicted. Yet the work ahead is daunting. Deeply rooted problems like racism and sexism seem nearly impossible to cure. Will we ever get past them?
In light of these realities, I choose to be a clear-eyed optimist: grateful for progress and also realistic about the challenges ahead. I’m grateful for everyone who is making AI more responsible through frank conversation, designing responsible systems, and sharing ideas — thank you! Let’s celebrate this progress and give kudos to those who have contributed in any way, large or small. And simultaneously, let’s identify problems and work toward solutions — while treating each other with civility. As a utilitarian matter, I believe this balanced approach is the best way to make a better world.
Keep learning!
Andrew
News
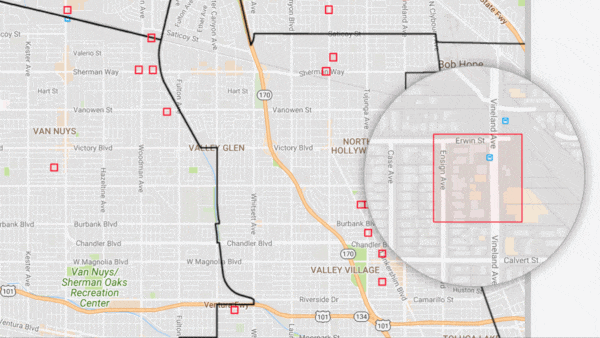
Minorities Reported
An independent investigation found evidence of racial and economic bias in a crime-prevention model used by police departments in at least nine U.S. states.
What’s new: Geolitica, a service that forecasts where crimes will occur, disproportionately targeted Black, Latino, and low-income populations, according to an analysis of leaked internal data by Gizmodo and The Markup. The reporters found the data on an unsecured police website. Geolitica, formerly called PredPol, changed its name in March.
How it works: The model predicts where crimes are likely to occur, helping police departments use allocate personnel. The company trains a separate model for each jurisdiction on two to five years of crime dates, locations, and types.
- The reporters filtered out jurisdictions with less than six months’ worth of data, leaving 5.9 million crime predictions from 38 U.S. jurisdictions between February 15, 2018 and January 30, 2021.
- They compared the output with census data that shows the geographic distribution of racial and socioeconomic groups. PredPol was more likely to predict crimes in areas with high numbers of Black and Latino residents in 84 percent of jurisdictions. It was less likely to target areas with high numbers of White residents in 74 percent of jurisdictions. The most-targeted areas included a higher proportion of lower-income households in 71 percent of jurisdictions.
- The reporters found no strong correlation between the system’s predictions and arrest rates provided by 11 police departments.
Sources of bias: Critics point to pervasive biases in the models’ training data as well as potential adverse social effects of scheduling patrols according to automated crime predictions.
- The training data was drawn from crimes reported to police. The U.S. Bureau of Justice Statistics found that only around 40 percent of violent crimes and 33 percent of property crimes were reported in 2020, leaving many possible crimes unaccounted for. Moreover, people who earned $50,000 or more reported crimes 12 percent less frequently than those who earned $25,000 or less, which would skew the dataset toward less wealthy neighborhoods.
- Because the models are trained on historical data, they learn patterns that reflect documented disparities in police practices. Black people were more likely to be arrested than White people in 90 percent of jurisdictions in the study, according to an FBI report, the authors wrote.
- Such algorithms perpetuate patrols in areas that already are heavily patrolled, leading to arrests for minor offenses that tend to receive scant attention elsewhere, critics said.
The response: Geolitica confirmed that the data used in the investigation “appeared to be” authentic, but it took issue with the analysis:
- The data was “erroneous” and “incomplete,” the company said. One jurisdiction that showed extreme disparities had misused the software, leading to extra predictions.
- The models aren’t trained on demographic, ethnic, or socioeconomic information, which “eliminates the possibility for privacy or civil rights violations seen with other intelligence-led or predictive policing models,” the company said. However, research has shown that learning algorithms can absorb biases in datasets that don’t explicitly label biased features.
Why it matters: Over 70 U.S. law enforcement jurisdictions use Geolitica’s service, and it is used in other countries as well. Yet this report is the first independent analysis of the algorithm’s performance based on internal data. Its findings underscore concerns that predictive policing systems invite violations of civil liberties, which have prompted efforts to ban such applications.
We’re thinking: Predictive policing can have a profound impact on individuals and communities. Companies that offer such high-stakes systems should audit them for fairness and share the results proactively rather than waiting for data leaks and press reports.
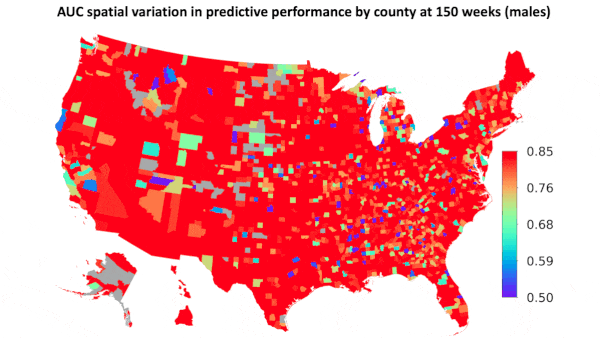
Recognizing Autism
Classical machine learning techniques could help children with autism receive treatment earlier in life.
What’s new: Researchers led by Ishanu Chattopadhyay at University of Chicago developed a system that classified autism in young children based on data collected during routine checkups.
Key insight: Autistic children have higher rates of certain conditions — such as asthma, gastrointestinal problems, and seizures — than their non-autistic peers. Incidence of these diseases could be a useful diagnostic signal.
How it works: The authors used Markov models, which predict the likelihood of a sequence of actions occurring, to feed a gradient boosting machine (an ensemble of decision trees). The dataset comprised weekly medical reports on 30 million children aged 0 to 6 years.
- The authors identified 17 disease categories — respiratory, metabolic, nutritional, and so on — that appeared in the dataset.
- They turned each child’s medical history into a time series, one for each disease category. For instance: week 1, no respiratory disease; week 2, respiratory disease; week 3, an illness in a different category; week 4, no respiratory disease.
- Using the time series, the authors trained 68 Markov models: one for each disease category for various combinations of male/female and autistic/not autistic. The models learned the likelihood that the diagnosis a given child received for each disease category occurred in the order that it actually occurred.
- Given the Markov models’ output plus additional information derived from the time series, a gradient boosting machine rendered a classification.
Results: The system’s precision — the percentage of kids it classified as autistic who actually had the condition — was 33.6 percent at 26 months. Classifying children of the same age, a questionnaire often used to diagnose children between 18 and 24 months of age achieved 14.1 percent precision. The model was able to achieve sensitivity — the percentage of children it classified correctly as autistic — as high as 90 percent, with 30 percent fewer false positives than the questionnaire at a lower sensitivity.
Why it matters: It may be important to recognize autism early. Although there’s no consensus, some experts believe that early treatment yields the best outcomes. This system appears to bring that goal somewhat closer by cutting the false-positive rate in half compared to the questionnaire. Nonetheless, it misidentified autism two-thirds of the time, and the authors caution that it, too, could lead to over-diagnosis.
We’re thinking: Data drift and concept drift, which cause learning algorithms to generalize poorly to populations beyond those represented in the training data, has stymied many healthcare applications. The authors' large 30 million-patient dataset makes us optimistic that their approach can generalize in production.
A MESSAGE FROM DEEPLEARNING.AI
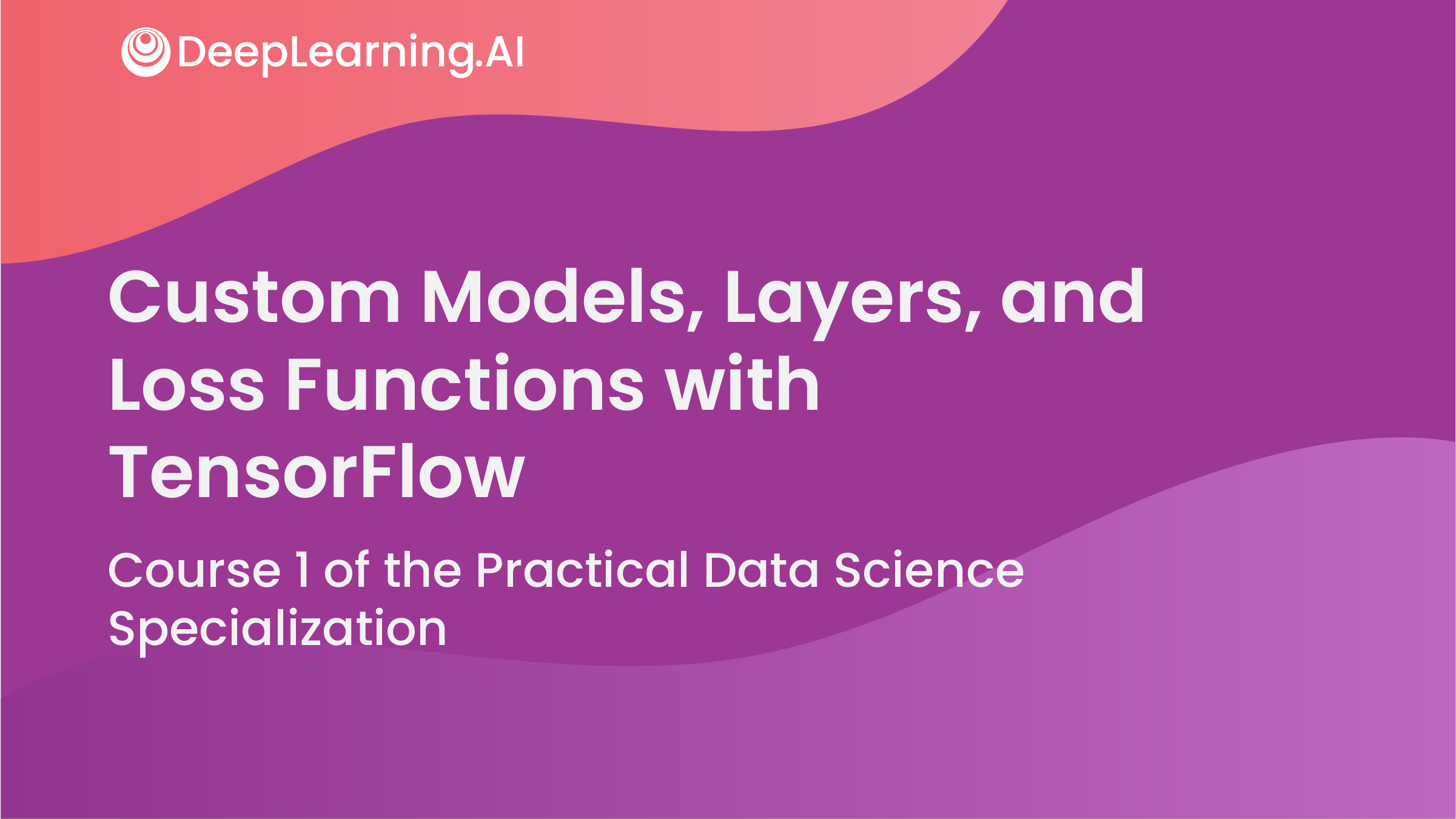
Have you checked out our Practical Data Science Specialization? This specialization will help you develop the practical skills to deploy data science projects and teach you how to overcome challenges at each step using Amazon SageMaker.

Corporate Ethics Counterbalance
One year after her acrimonious exit from Google, ethics researcher Timnit Gebru launched an independent institute to study neglected issues in AI.
What’s new: The Distributed Artificial Intelligence Research Institute (DAIR) is devoted to countering the influence of large tech companies on the research, development, and deployment of AI. The organization is funded by $3 million in grants from the Ford Foundation, MacArthur Foundation, Kapor Center, and Open Society Foundation.
How it works: DAIR is founded upon Gebru’s belief that large tech companies, with their focus on generating profit, lack the incentive to assess technology’s harms and the motivation to address them. It will present its first project this week at NeurIPS.
- Raesetje Sefala of Wits University in Johannesburg led a team to develop a geographic dataset of South African neighborhoods. It combines geographic coordinates of building footprints, household income, and over 6000 high-resolution satellite photos taken between 2006 and 2017.
- The team trained semantic segmentation models to outline neighborhoods, gauge their growth over time, and classify them as wealthy, nonwealthy, nonresidential, or vacant.
- The initial results show how policies enacted during apartheid have segregated wealthy communities from poor townships, which are often side by side.
Behind the news: Gebru was the co-lead of Google’s Ethical AI group until December 2020. The company ousted her after she refused to retract or alter a paper that criticized its BERT language model. A few months later, it fired her counterpart and established a new Responsible AI Research and Engineering group to oversee various initiatives including Ethical AI.
Why it matters: AI has the potential to remake nearly every industry as well as governments and social institutions, and the AI community broadly agrees on the need for ethical principles to guide the process. Yet the companies at the center of most research, development, and deployment have priorities that may overwhelm or sidetrack ethical considerations. Independent organizations like DAIR can call attention to the ways in which AI may harm some groups and use the technology to shed light on problems that may be overlooked by large, mainstream institutions.
We’re thinking: Gebru has uncovered important issues in AI and driven the community toward solutions. We support her ongoing effort to promote ethics in technology.
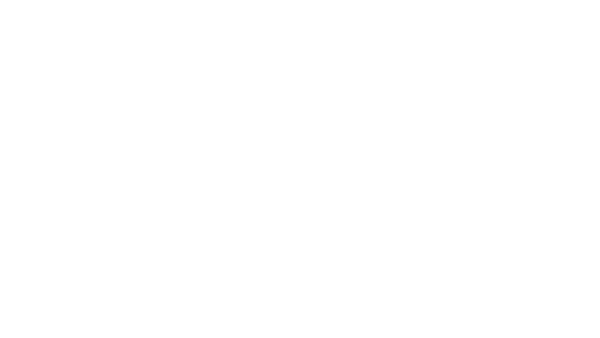
Reinforcement Learning Transformed
Transformers have matched or exceeded earlier architectures in language modeling and image classification. New work shows they can achieve state-of-the-art results in some reinforcement learning tasks as well.
What’s new: Lili Chen and Kevin Lu at UC Berkeley with colleagues at Berkeley, Facebook, and Google developed Decision Transformer, which models decisions and their outcomes.
Key insight: A transformer learns from sequences, and a reinforcement learning task can be modeled as a repeating sequence of state, action, and reward. Given such a sequence, a transformer can learn to predict the next action (essentially recasting the reinforcement learning task as a supervised learning task). But this approach introduces a problem: If the transformer chooses the next action based on earlier rewards, it won’t learn to take actions that, though they may bring negligible rewards on their own, lay a foundation for winning higher rewards in the future. The solution is to tweak the reward part of the sequence. Instead of showing the model the reward for previous actions, the authors provided the sum of rewards remaining to be earned by completing the task. This way, the model took actions likely to reach that sum.
How it works: The researchers trained a generative pretrained transformer (GPT) on recorded matches of three types of games: Atari games with a fixed set of actions, OpenAI Gym games that require continuous control, and Key-to-Door. Winning Key-to-Door requires learning to pick up a key, which brings no reward, and using it to open a door and receive a reward.
- The transformer generated a representation of each input token using a convolutional layer for visual inputs (Key-to-Door and Atari screens) and a linear layer for other types of input (actions, rewards, and, in OpenAI games, state).
- During training, it received tokens for up to 50 reward-state-action triplets. For instance, in the classic Atari game Pong, the sum of all rewards for completing the task might be 100. The first action might yield 10 points, so the sum in the next triplet would fall to 90; the state would be the screen image, and the action might describe moving the paddle to a new position. In Key-to-Door, the sum of all rewards for completing the task remained 1 throughout the game (the reward for unlocking the door at the very end); the state was the screen; and the action might be a move in a certain direction.
- At inference, instead of receiving the sum of rewards remaining to be earned, the model received a total desired reward — the reward the authors wanted the model to receive by the end of the game. Given an initial total desired reward and the state of the game, the model generated the next action. Then the researchers reduced the total desired reward by the amount received for performing the action, and so on.
- For all games except Key-to-Door, the total desired reward exceeded the greatest sum of rewards for that game in the training set. This encouraged the model to maximize the total reward.
Results: The authors compared Decision Transformer with the previous state-of-the-art method, Conservative Q-Learning (CQL).They normalized scores of Atari and OpenAI Gym games to make 0 on par with random actions and 100 on par with a human expert. In Atari games, the authors’ approach did worse, earning an average score of 98 versus CQL’s 107. However, it excelled in the more complex games. In OpenAI Gym, averaged 75 versus CQL’s 64. In Key-to-Door, it succeeded 71.8 percent of the time versus CQL’s 13.1 percent.
Why it matters: How to deal with actions that bring a low reward in the present but contribute to greater benefits in the future is a classic issue in reinforcement learning. Decision Transformer learned to solve that problem via self-attention during training.
We’re thinking: It’s hard to imagine using this approach for online reinforcement learning, as the sum of future rewards would be unknown during training. That said, it wouldn’t be difficult to run a few experiments, train offline, and repeat.