Dear friends,
Since the pandemic started, several friends and teammates have shared with me privately that they were not doing well emotionally. I’m grateful to each person who trusted me enough to tell me this. How about you — are you doing okay?
Last week, the Olympic gymnastic champion Simone Biles temporarily withdrew from competition because she didn’t feel mentally healthy enough to do her best and perhaps avoid a career-ending injury. She’s not alone in struggling with mental health. About 4 in 10 adults in the U.S. reported symptoms of anxiety or depression during the pandemic, according to one survey.
Once, after looking over a collaborator’s project, I said, “That’s really nice work” and got back a sad facial expression as though my collaborator was near tears. I asked if they were okay, wondering if I had said something wrong, but they paused and shook their head. After I probed gently a little more, they burst out crying and told me that my remark was the first appreciation they had received in longer than they could remember.
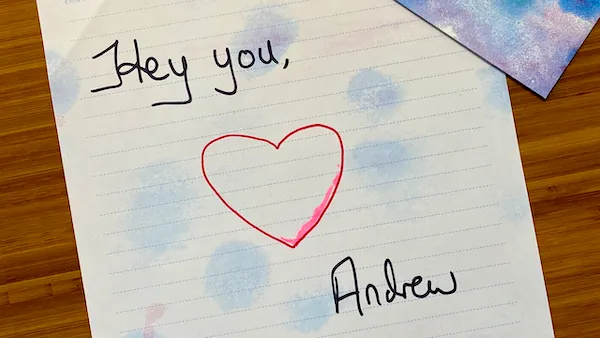
Many people outwardly look like they’re doing well, but inside they’re lonely, anxious, or uncertain about the future. If you’re feeling fine, that’s great! But if you’re among the millions who feel that something is off-balance, I sympathize, and I want you to know that I care about you and appreciate you.
As the pandemic wears on, many of us are hungry to connect with others more deeply. If this describes you, or if you want to help someone else who might feel this way, perhaps you can start by letting someone know you appreciate them or something they did. I think this will make them — and maybe you, too — feel better.
Love,
Andrew
News
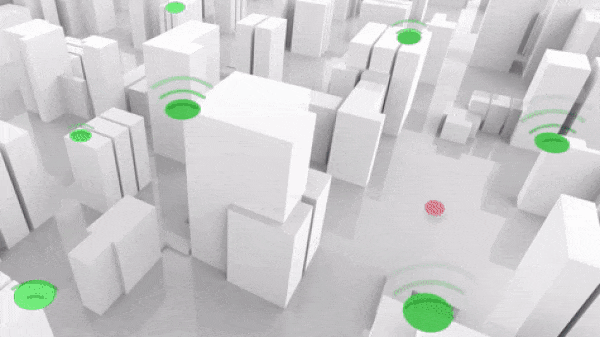
Shots in the Dark
A crime-fighting AI company altered evidence to please police, a new investigation claims — the latest in a rising chorus of criticism.
What’s new: ShotSpotter, which makes a widely used system of the same name that detects the sound of gunshots and triangulates their location, modified the system’s findings in some cases, Vice reported.
Altered output: ShotSpotter’s output and its in-house analysts’ testimony have been used as evidence in 190 criminal cases. But recent court documents reveal that analysts reclassified as gunshots sounds the system had attributed to other causes and changed the location where the system determined that gunshots had occurred.
- Last year, ShotSpotter picked up a noise around one mile from a spot in Chicago where police believed someone was murdered at the same time. The system classified it as a firecracker. Analysts later reclassified it as a gunshot and modified its location, placing the sound closer to the scene of the alleged crime. Prosecutors withdrew the ShotSpotter evidence after the defense requested that the judge examine the system’s forensic value.
- When federal agents fired at a man in Chicago in 2018, ShotSpotter recorded only two shots — those fired by cops. The police asked the company to re-examine the data manually. An analyst found five additional shots, presumably those fired by the perpetrator.
- In New York in 2016, a company analyst reclassified as gunshots a sound that the algorithm had classified as helicopter noise after being contacted by police. A judge later threw out the conviction of a man charged with shooting at police in that incident, saying ShotSpotter’s evidence was unreliable.
The response: In a statement, ShotSpotter called the Vice report “false and misleading.” The company didn’t deny that the system’s output had been altered manually but said the reporter had confused two different services: automated, real-time gunshot detection and analysis after the fact by company personnel. “Forensic analysis may uncover additional information relative to a real-time alert such as more rounds fired or an updated timing or location upon more thorough investigation,” the company said, adding that It didn’t change its system’s findings to help police.
Behind the news: Beyond allegations that ShotSpotter has manually altered automated output, researchers, judges, and police departments have challenged the technology itself.
- A May report by the MacArthur Justice Center, a nonprofit public-interest legal group, found that the vast majority of police actions sparked by ShotSpotter alerts did not result in evidence of gunfire or gun crime.
- Several cities have terminated contracts with ShotSpotter after determining that the technology missed around 50 percent of gunshots or was too expensive.
- Activists are calling on Chicago to cancel its $33 million contract with the company after its system falsely alerted police to gunfire, leading to the shooting of a 13-year-old suspect.
Why it matters: ShotSpotter’ technology is deployed in over 100 U.S. cities and counties. The people who live in those places need to be able to trust criminal justice authorities, which means they must be able to trust the AI systems those authorities rely on. The incidents described in legal documents could undermine that trust — and potentially trust in other automated systems.
We’re thinking: There are good reasons for humans to analyze the output of AI systems and occasionally modify or override their conclusions. Many systems keep humans in the loop for this very reason. It’s crucial, though, that such systems be transparent and subject to ongoing, independent audits to ensure that any modifications have a sound technical basis.
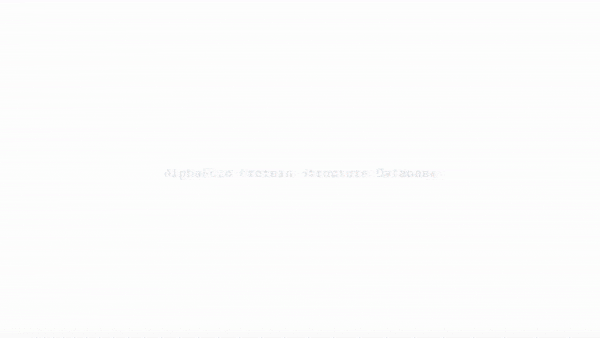
Biomedical Treasure Chest
DeepMind opened access to AlphaFold, a model that finds the shapes of proteins, and to its output so far — a potential cornucopia for biomedical research.
What’s new: The research lab, a division of Google’s parent company Alphabet, made AlphaFold freely available. It also opened databases that contain hundreds of thousands of three-dimensional protein shapes.
Shapes of things to come: Proteins are molecules made up of chains of amino acids. They perform myriad biological functions depending on the way the chain folds, and understanding their shapes can shed light on what they do and how they do it. Protein shapes are determined by the proximity of essential portions, or residues, of amino acids. AlphaFold finds likely shapes by optimizing possible structures that keep residues close to one another based on their positions and angles. For a description of how it works, see “Protein Shapes Revealed” here.
- The company published research that describes how to use AlphaFold to find the shapes of both general and human-specific proteins.
- The model has analyzed the structure of roughly 98 percent of proteins found in the human body. It has analyzed hundreds of thousands more in 20 other organisms commonly used by researchers such as e.coli, fruit flies, and soybeans.
- The company plans to release an additional 100 million protein structures by the end of 2021. Such data is published and maintained by the European Molecular Biology Laboratory.
Behind the news: Until recently, scientists had to rely on time-consuming and expensive experiments to figure out protein shapes. Those methods have yielded about 180,000 protein structures. AlphaFold debuted in 2018, when it won an annual contest for predicting protein structures. A revised version of the model won again in 2020 with an average error comparable to the width of an atom.
Why it matters: Biologists could use these tools to better understand the function of proteins within the human body and develop new treatments for some of medicine’s most vexing maladies. Researchers already are using AlphaFold data to devise treatments for maladies including Covid-19 and several common, deadly tropical diseases.
We’re thinking: We applaud DeepMind’s decision to make both its landmark model and the model’s output available for further research. We urge other companies to do the same.
A MESSAGE FROM DEEPLEARNING.AI
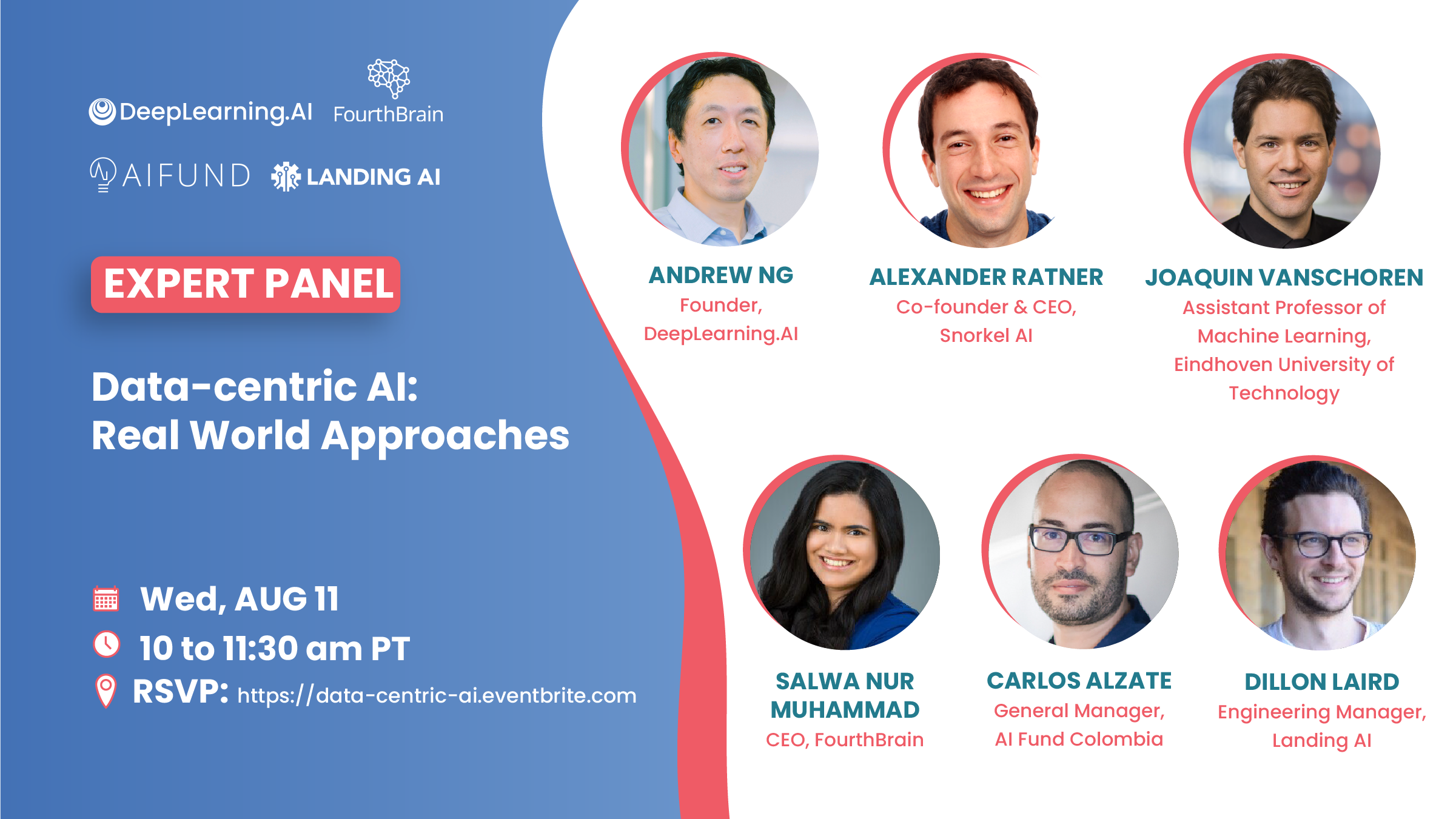
AI is undergoing a shift from model-centric to data-centric development. How can you implement a data-centric approach? Register to hear experts discuss this and other topics on August 11, 2021, at 10 A.M., Pacific time at “Data-centric AI: Real-World Approaches.”
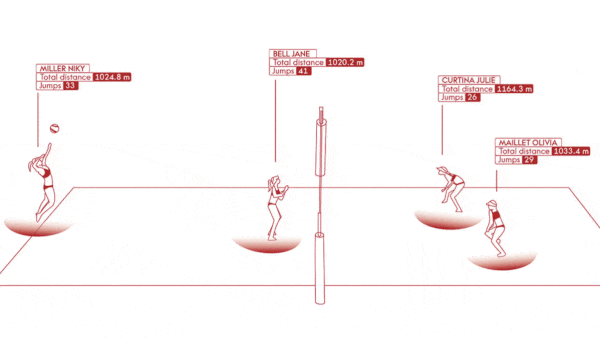
Olympic AI
Computer vision is keeping a close eye on athletes at the Summer Olympic Games in Tokyo.
What’s new: Omega Timing, a Swiss watchmaker and the Olympic Games’ official timekeeper, is providing systems that go far beyond measuring milliseconds. The company’s technology is tracking gameplay, analyzing players’ motions, and pinpointing key moments, Wired reported.
How it works: Omega Timing’s systems track a variety of Olympic sports including volleyball, swimming, and trampoline. Their output is intended primarily for coaches and athletes to review and improve performance, but it’s also available to officials and broadcasters.
- The volleyball system classifies shots such as smashes, blocks, and spikes with 99 percent accuracy by tracking changes in the ball’s direction and velocity. It integrates gyroscopic sensors embedded in players’ clothing that monitor players’ movements. If the ball flies momentarily out of the camera’s sight, it computes the likely path. The company says the system is 99 percent accurate at determining different moves.
- A pose estimator tracks gymnasts’ motions as they twist and flip on the trampoline. It also detects how precisely they land at the end of their routines.
- An image recognition system watches water events, measuring the distance between swimmers, their speed, and the number of strokes each one takes.
Behind the news: Omega Timing has measured Olympic performance since 1932. It introduced photo-finish cameras at the 1948 Olympiad in London. Its systems are certified by the Swiss Federal Institute of Metrology.
Why it matters: Technology that helps athletes examine their performance in minute detail could give them a major edge in competition. It offers the rest of us a finer appreciation of their accomplishments.
We’re thinking: For this year’s games, the International Olympic Committee added to the schedule competitive skateboarding, surfing, and climbing. Next time, how about a data-centric AI competition?
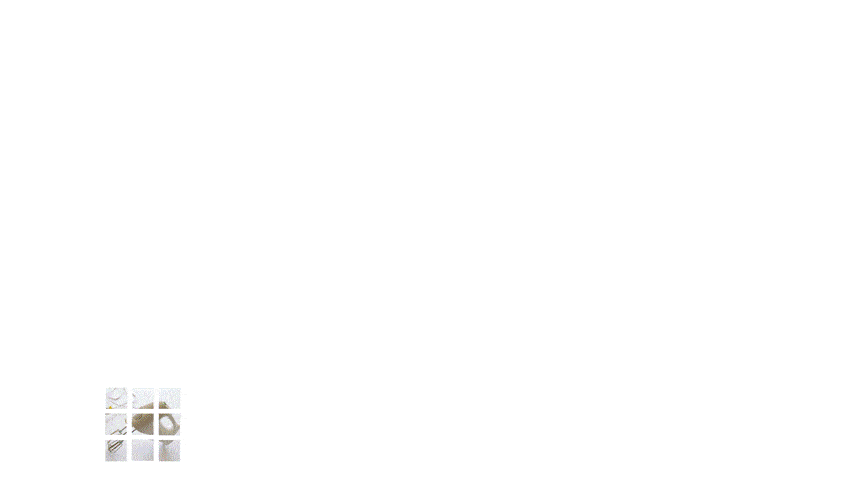
Revenge of the Perceptrons
Why use a complex model when a simple one will do? New work shows that the simplest multilayer neural network, with a small twist, can perform some tasks as well as today’s most sophisticated architectures.
What’s new: Ilya Tolstikhin, Neil Houlsby, Alexander Kolesnikov, Lucas Beyer, and a team at Google Brain revisited multilayer perceptrons (MLPs, also known as vanilla neural networks). They built MLP-Mixer, a no-frills model that approaches state-of-the-art performance in ImageNet classification.
Key insight: Convolutional neural networks excel at processing images because they’re designed to discern spatial relationships, and pixels that are nearby one another in an image tend to be more related than pixels that are far apart. MLPs have no such bias, so they tend to learn interpixel relationships that exist in the training set and don’t hold in real life. By modifying MLPs to process and compare images across patches rather than individual pixels, MLP-Mixer enables this basic architecture to learn useful image features.
How it works: The authors pretrained MLP-Mixer for image classification using ImageNet-21k, which contains 21,000 classes, and fine-tuned it on the 1,000-class ImageNet.
- Given an image divided into patches, MLP-Mixer uses an initial linear layer to generate 1,024 representations of each patch. MLP-Mixer stacks the representations in a matrix, so each row contains all representations of one patch, and each column contains one representation of every patch.
- MLP-Mixer is made of a series of mixer layers, each of which contains two MLPs, each made up of two fully connected layers. Given a matrix, a mixer layer uses one MLP to mix representations within columns (which the authors call token mixing) and another to mix representations within rows (which the authors call channel mixing). This process renders a new matrix to be passed along to the next mixer layer.
- A softmax layer renders a classification.
Results: An MLP-Mixer with 16 mixer layers classified ImageNet with 84.15 percent accuracy. That’s comparable to the state-of-the-art 85.8 percent accuracy achieved by a 50-layer HaloNet, a ResNet-like architecture with self-attention.
Yes, but: MLP-Mixer matched state-of-the-art performance only when pretrained on a sufficiently large dataset. Pretrained on 10 percent of JFT300M and fine-tuned on ImageNet, it achieved 54 percent accuracy on ImageNet, while a ResNet-based BiT trained the same way achieved 67 percent accuracy.
Why it matters: MLPs are the simplest building blocks of deep learning, yet this work shows they can match the best-performing architectures for image classification.
We’re thinking: If simple neural nets work as well as more complex ones for computer vision, maybe it’s time to rethink architectural approaches in other areas, too.