Dear friends,
In my letter last week, I alluded to the way AI tends to concentrate power and wealth. This tendency worries me, and I believe it deserves more attention.
The U.S. government has been looking into these winner-take-most dynamics at a few leading technology companies from an antitrust perspective. But the issue is much bigger than that. AI will concentrate power in many industries, including ones that haven’t traditionally relied on high tech, in the hands of a few winners.
For instance, Amazon has come to dominate retailing at the expense of innumerable chains and mom-and-pop stores. Uber, Lyft, and Didi are concentrating power over the taxi industry, which used to support hundreds of thriving local companies. Retailing and taxi service are not traditionally viewed as tech industries.
Driven by digitization and AI, this pattern will play out in many more industries in this decade.
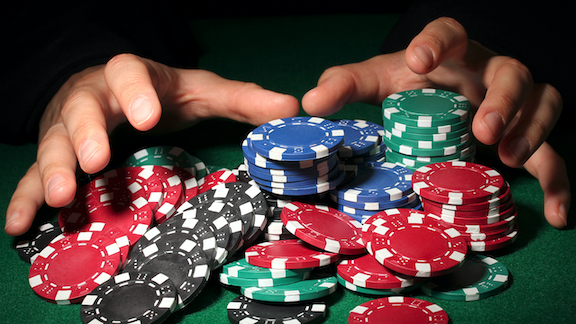
Covid-19 has added further fuel to these dynamics. Some retailers managed the shift to e-commerce. They are collecting data and implementing AI to optimize sales, and they’re becoming more powerful. But others were nearly destroyed as the pandemic choked off foot traffic in brick-and-mortar stores. They don’t have spare dollars to invest in AI, and they’re falling farther and farther behind.
Even as AI creates tremendous wealth, I worry about the growing concentrations of power and wealth, and those who will be left behind. Government will have to step up to address this situation, but significant responsibility also lies with the all of us who conceive, build, and manage this technology. I ask each of you to use your knowledge wisely, in ways that benefit society at large rather than a select few — even if that “select few” is yourself.
Keep learning!
Andrew
News
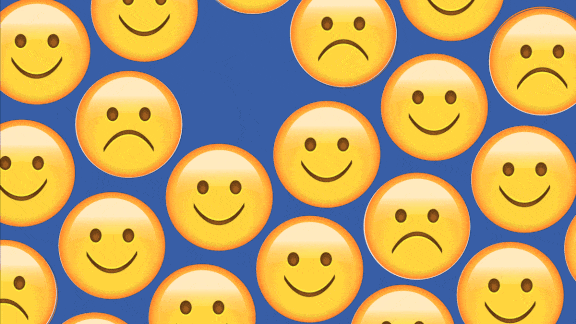
Online Clues to Mental Illness
Can social media posts reveal early signs of mental illness? A new machine learning model shows promising results.
What’s new: Researchers led by Michael Birnbaum at the Feinstein Institute for Medical Research and Raquel Norel at the IBM Watson Research Center developed a model that analyzes messages and images posted by Facebook users for indicators of psychological problems. Unlike earlier efforts to classify mental illness based on social media posts, which relied on subjects to report their condition, this one used actual diagnoses.
How it works: The authors collected millions of messages and images posted over 18 months by 223 volunteers. Some posters had been hospitalized with schizophrenia-spectrum disorders, some had been diagnosed with mood disorders like depression, and some had no mental health issues.
- For text input, the authors labeled training examples using LIWC, which represents emotional tone, confidence, and authenticity. For images, they annotated measurements of hue, saturation, pixel density, and other factors.
- They trained a random forest to classify messages from each group.
Results: The model identified people diagnosed with schizophrenia and mood disorders at a rate comparable to that of a standard 10-point questionnaire, according to Wired. The researchers found that individuals diagnosed as schizophrenic used “see,” “hear,” and other words related to perception more often than the others. Those with mood disorders tended to post more blue-tinted pictures. Both groups also used more swear words and posted smaller photos.
Behind the news: Social media posts are a popular hunting ground for researchers aiming to gauge users’ mental states. Recent studies suggest that Reddit comments can indicate conditions like ADHD, anxiety, and bipolar disorder, and that Twitter users often telegraph their depression, postpartum mood disorder, suicidal ideation, and more.
Why it matters: This tool could help doctors catch mental illness early — especially in young adults, who tend to be both prolific users of social media and at higher risk of developing mental illness — and could provide valuable context for treatment.
We’re thinking: Useful though it might be in some cases, scanning social media posts for clues to a user’s mental state holds worrisome implications. Yet another reason social media companies must adopt stricter standards to protect privacy.
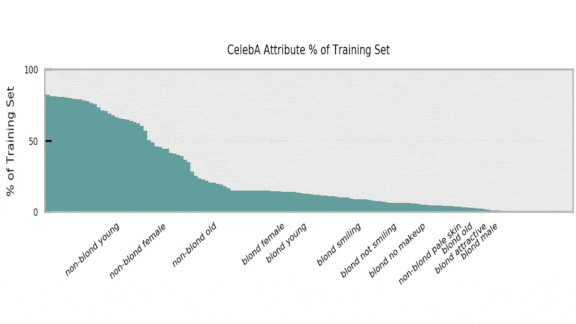
Smaller Models, Bigger Biases
Compression methods like parameter pruning and quantization can shrink neural networks for use in devices like smartphones with little impact on accuracy — but they also exacerbate a network’s bias. Do compressed models perform less well for underrepresented groups of people? Yes, according to new research.
What’s new: A Google team led by Sara Hooker and Nyalleng Moorosi explored the impact of compression on image recognition models’ ability to perform accurately across various human groups. The authors also proposed a way to rank individual examples by how difficult they are to classify.
Key insight: In earlier work, members of the team showed that compressed image recognition models, although they maintained their accuracy overall, had trouble identifying classes that were rare in their training data. To learn whether that shortcoming translates into bias against underrepresented human types, the researchers trained models to recognize a particular class (people with blond hair), compressed them, and measured the differences in their accuracy across different types of people. This enabled them to evaluate the difference in performance between compressed and uncompressed models with respect to underrepresented groups.
How it works: The authors trained a set of ResNet-18s on CelebA, a dataset of celebrity faces, to classify photos of people with blond hair. (CelebA is notorious for producing biased models.) Then they compressed the models using various combinations of pruning and quantization.
- Using both compressed and uncompressed models, they predicted blonde/not-blonde labels for the CelebA test set. They compared the performance of uncompressed and compressed models in classifying pictures of young people, old people, men, women, young men, old men, young women, and old women. This gave them a measure of how compression affected model bias against these groups.
- To rank examples for how difficult they were to classify, the authors found the difference between the number of “blond” predictions by uncompressed and compressed models for a given example, and added that to the difference between the number of “not blond” predictions by the same models. The sum yielded a score of how consistently the models labeled a given example.
- To make it easier to study various combinations of image and model, the researchers used a variable threshold to identify the least consistently labeled examples by percentage (designated “CIE” in the gallery above.)
Results: Pruning 95 percent of model parameters boosted the false-positive “blond” rate for women (who made up 14 percent of the dataset) by an average 6.32 percent, but it increased that rate for men (less than 1 percent of the dataset) by 49.54 percent. (The authors didn’t report corresponding results for models compressed by quantization.) Furthermore, the ranking method succeeded in identifying the examples that were most difficult to classify. A 95-percent pruned model was 93.39 percent accurate over the entire dataset, but 43.43 percent accurate on the 1 percent least consistently labeled examples. An unpruned model had much the same trouble. It was 94.76 percent accurate over the entire dataset, but 55.35 percent accurate on the 1 percent of least consistently labeled examples.
Why it matters: Model compression is an important part of practical deployments: Shipping a 10MB neural network for a mobile device is much more acceptable than shipping a 100MB model. But if compression exacerbates biases, we must systematically audit and address those issues.
We’re thinking: This work is a reminder that it’s not enough to optimize overall classification accuracy. We need to make sure our models also perform well on various slices of the data.
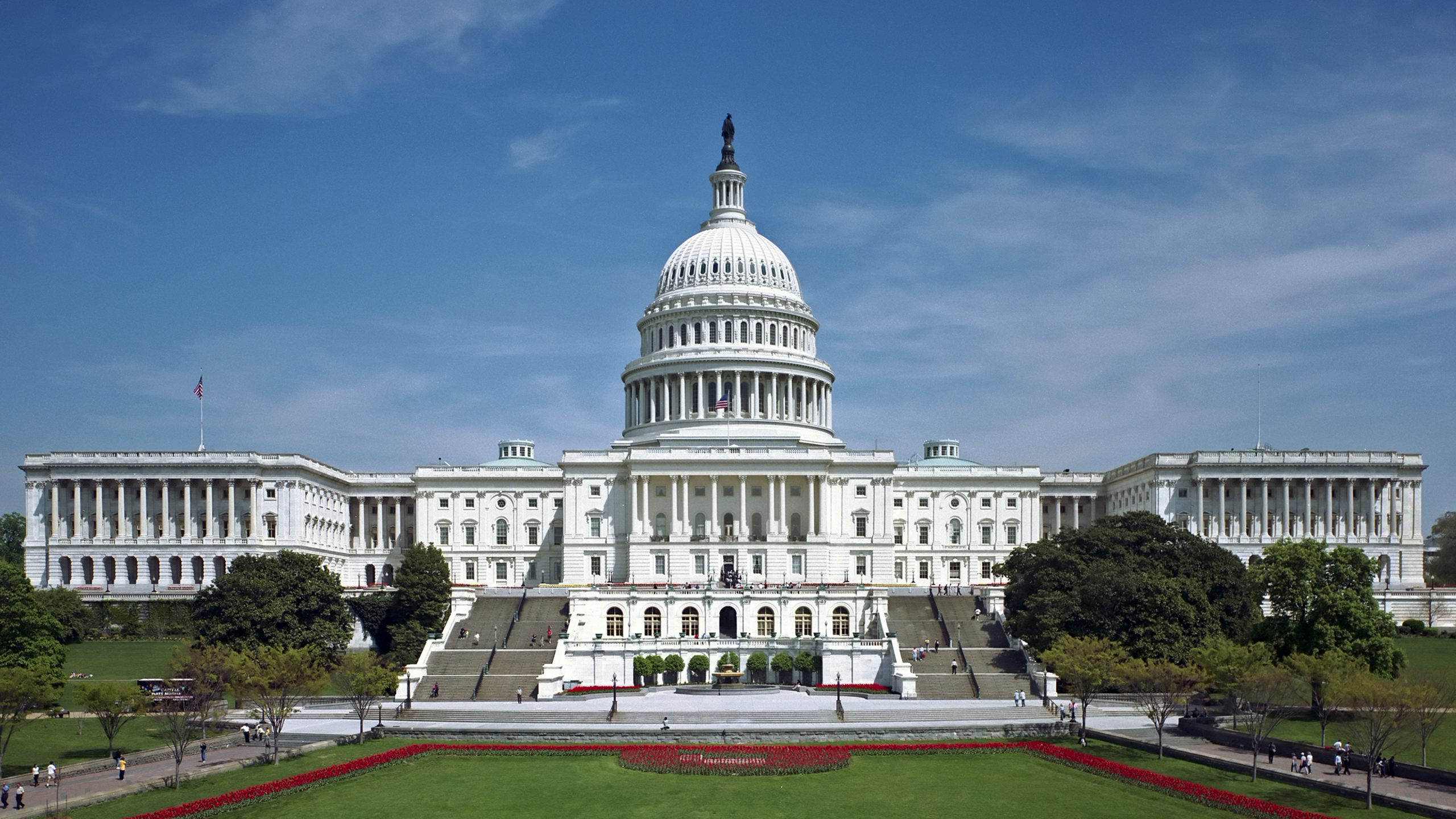
U.S. New Year’s Resolutions for AI
U.S. lawmakers authorized a slew of national programs that promote artificial intelligence research, development, and deployment, and support efforts to make sure the results are ethical and trustworthy.
What’s new: The 4,500 pages of the National Defense Authorization Act (NDAA), which primarily serves to authorize programs for the U.S. military, includes provisions that promote AI in both civilian and military agencies, and academic institutions, too.
What it says: The NDAA only authorizes these programs; funding will come with further legislation. Among its provisions:
- The National AI Initiative will coordinate research and development across civilian, intelligence, and defense agencies.
- The National Science Foundation will begin planning a National Research Cloud, an aggregation of processing and data resources to be made available to academic and nonprofit researchers. (Fei-Fei Li described the National Research Cloud in our special New Year issue of The Batch.) The NSF will also build AI research institutes focused on health care, manufacturing, and other sectors; study the impact of AI on the nation’s workforce; and sponsor competitions that promote innovation.
- The National Institute of Standards and Technology will create a framework to grade AI systems on trustworthiness and define related terms like explainability, privacy, and transparency. The agency also will formulate privacy and security standards for training datasets, data management, and AI hardware. The Defense Department must ensure that any AI it acquires was developed “ethically and responsibly.”
- The Joint AI Center, a military organization launched in 2018, will report directly to the Deputy Secretary of Defense, giving the Pentagon leadership more direct control over its research and development priorities. The center’s biannual report must describe its work developing AI standards and its collaborations with other agencies.
Yes, but: Some of these programs, such as the NSF’s AI research institutes, will cost money that Congress has yet to appropriate. Russell Wald, director of policy at Stanford’s Institute for Human-Centered Artificial Intelligence, told The Batch he’s optimistic that funding will be allocated where it’s needed.
Behind the news: President Donald Trump vetoed the NDAA in December, saying he wanted it to include a repeal of Section 230 of the Communications Decency act, which protects internet companies from legal liability for user-generated content on their sites. Congress overrode the veto and passed the bill into law on New Year’s Day.
Why it matters: The U.S. is a global leader in AI innovation and home to more big AI companies than anywhere else, but its government has lagged in issuing a comprehensive AI strategy. Directives like the National Research Cloud would give a healthy boost to AI researchers in many areas, and the impact likely would ripple across the world.
We’re thinking: This bill is a major step forward in U.S. support for AI. We’ll keep our fingers crossed that the necessary funding comes through.
A MESSAGE FROM DEEPLEARNING.AI
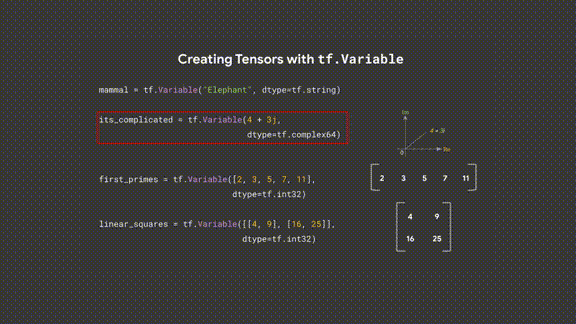
We’re excited to announce that Generative Deep Learning with TensorFlow, course 4 of the TensorFlow: Advanced Techniques Specialization, will launch on January 13. Pre-enroll now
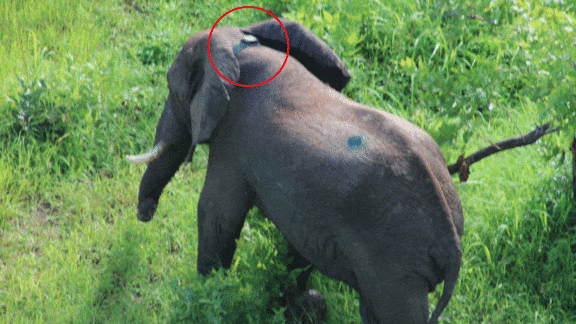
Algorithms For Elephants
An AI-powered collar may help protect wild elephants from poachers, hunters, and other hostile humans.
What’s new: Ten ElephantEdge wireless tracking collars will be fitted onto African elephants next year, TechCrunch reported. The product of an open source collaboration between hardware and software engineers, the collar serves as a platform for machine learning models designed to interpret elephant behavior and alert sympathetic humans when the animals are in trouble.
How it works: The models included are winners of a competition organized by Hackster.io, a hardware engineering community, and Smart Parks, a Dutch conservation group. They were built using development tools from Edge Impulse and work with hardware from organizations including Institute Irnas and Avnet.
- Elephant AI contributed a model that recognizes human sounds picked up by the collar’s microphone and cross-references them with GPS coordinates to detect possible poachers. A different one uses data from the collar’s accelerometer to determine when elephants are eating, sleeping, or running.
- The Gajraj AI project built models to limit harm when elephants seek food from farms. For instance, one analyzes motions and vibrations of an elephant’s trunk for signs of distress from human interaction and alerts people nearby.
- Elephant Guardian provided models that interpret elephant activity, as well as one that alerts rangers to sounds of weapons commonly used by poachers, such as AK-47s.
Behind the news: Defenders of wildlife are increasingly using AI to extend their reach and effectiveness.
- A machine learning model called PAWS suggests optimal patrol routes to help park rangers in Cambodia intercept poachers.
- Image recognition models associated with camera traps in the wild help conservationists keep track of numbers and movements of endangered species. For instance, a model from Google Earth recognizes 614 species and classifies 3.6 million images an hour.
Why it matters: Africa’s elephant population has plummeted in recent years. Only about 350,000 wild elephants remain on the continent, and poachers illegally kill upward of 15,000 a year. These animals need all the help they can get.
We’re thinking: This work addresses the elephant in the room.
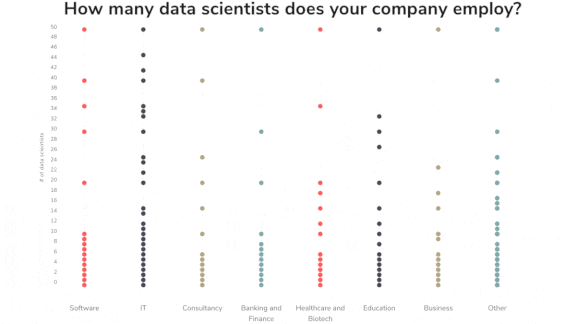
Enterprise AI on the Rise
A survey of AI in large companies sees boom times ahead — if AI teams can get past issues that surround implementation.
What’s new: Businesses of all sizes are using more machine learning, spending more on it, and hiring more engineers to wrangle it, according to a survey of 750 business leaders by Algorithmia, which provides tools that automate model deployment and management. Nonetheless, struggles with deployment, scaling, and other issues continue to hinder adoption.
What they found: The survey questioned executives in a variety of sectors including finance, healthcare, education, and information technology. More than two-thirds of those who responded said their AI budgets are growing, while only 2 percent are cutting back.
- 40 percent of companies surveyed employed more than 10 data scientists, double the rate in 2018, when Algorithmia conducted its previous study. 3 percent employed more than 1,000 data scientists.
- Many respondents said they’re in the early stages, such as evaluating use cases and developing models.
- Many struggle with deployment. Half of those surveyed took between 8 days and three months to deploy a model. 5 percent took a year or more. Generally, larger companies took longer to deploy models, but the authors suggest that more mature machine learning teams were able to move faster.
- Scaling models is the biggest impediment, cited by 43 percent of respondents. In larger organizations, this may reflect siloing of machine learning teams in various departments. The authors believe that the solution is to centralize AI efforts in an innovation hub like those launched by Ericsson, IBM, and Pfizer.
Behind the news: Several other recent surveys shed light on AI’s evolving role in the business world. For instance, MIT Technology Review looked at AI’s growth in different global regions, and McKinsey examined how different market sectors, like manufacturing, marketing, and supply chain management, are finding profitable uses for the technology.
Why it matters: AI is new enough, and evolving fast enough, that every company’s experience is different. Spotting areas where industries where machine learning is having an impact, as well as trouble spots in deployment, can help guide crucial decisions.
We’re thinking: In 2019, many companies experimented with AI. In 2020, a growing number started talking about how to productionize models. In the coming year, we hope for rapid progress in MLOps processes and tools to make building and productionizing machine learning systems repeatable and systematic. AI Fund (where Andrew is managing general partner) has seen a lot of startups jump into this space, which bodes well for the future.