Dear friends,
Last week, I wrote about the U.S. Immigration and Customs Enforcement (ICE) policy that would have forced international students to leave the country if their university went fully online to manage the risk of Covid-19. This sudden change in the rules for student visas had students and institutions alike scrambling to figure out ways to comply.
Social media erupted in protest as students, parents, teachers, and administrators expressed their concerns. Harvard and MIT sued to block the policy. Attorneys general in at least 18 states brought lawsuits as well.
Yesterday, the government rescinded the policy, allowing international students to remain in the U.S. even if they take all their courses online. I am thrilled!
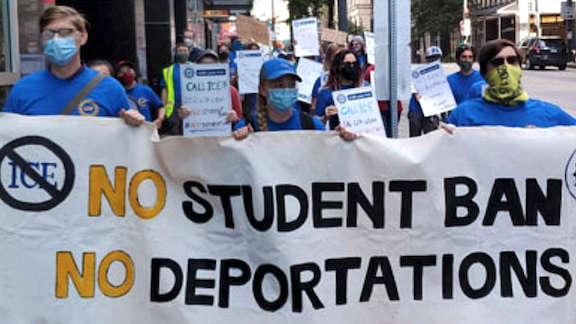
ICE’s retreat is an important reminder that our voices can make a difference. I have little doubt that the public outcry helped motivate the universities to sue and the government to backtrack.
I believe we all have a responsibility to speak out against injustice — respectfully and with cogent arguments, not “flame wars.” Even if each individual voice is just one among many, collectively we can make a huge impact.
Speaking out is especially important for the AI community as we grapple with difficult issues of bias, privacy, surveillance, and disinformation. We need every voice — including yours — to fulfill AI’s promise for the benefit of all people.
Keep learning!
Andrew
News
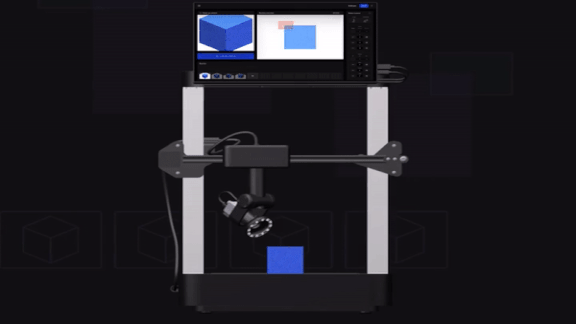
Assembly Line AI
Computer vision has been learning how to spot manufacturing flaws. The pandemic is accelerating that education.
What’s happening: Companies like Instrumental and Elementary are making AI-powered cameras that automate the spotting of damaged or badly assembled products on factory assembly lines, Wired reports. (For the record, deeplearning.ai’s sister company Landing AI is, too.)
How it works: Instrumental’s quality-control system first learns to recognize components in their ideal state and then to identify defects. It can spot faulty screws, disfigured circuit boards, and flaws in the protective coating on smartphone screens.
- Cameras along the assembly line take photos of products in the making. The manufacturer’s engineers review the images and label defects. The labeled data is used to fine-tune the system.
- Manufacturers often don’t allow outsiders direct access to their equipment, so Instrumental’s engineers typically tweak systems on-site. Amid the pandemic, though, five clients are allowing the company to monitor the assembly line remotely, making it possible to update the computer vision model on the fly.
Coming soon: Elementary plans to install robotic cameras in a U.S. Toyota plant. Workers will place a completed part beneath the camera for inspection, then press a button to indicate whether they agree with the robot’s assessment to fine-tune the model.
Behind the news: Omron, Cognex, and USS Vision have sold non-neural inspection systems for decades. Neural networks are making their way into the field as engineers develop techniques for learning what flaws look like from small numbers of examples.
Why it matters: Earlier automated inspection systems use hand-coded rules to identify specific flaws. Machine learning promises to be more adaptable and quicker to deploy. That could speed up assembly lines and cut manufacturing costs.
We’re thinking: The ability to learn from small amounts of data is the key to many applications of deep learning that are still beyond reach. We look forward to continued progress in this area.
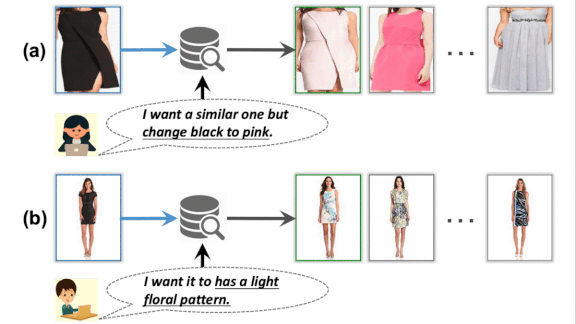
That Online Boutique, But Smarter
Why search for “a cotton dress shirt with button-down collar, breast pockets, barrel cuffs, scooped hem, and tortoise shell buttons in grey” when a photo and the words “that shirt, but grey” will do the trick? A new network understands the image-text combo. (This is the second of three papers presented by Amazon at the IEEE Conference on Computer Vision and Pattern Recognition (CVPR). We’ll cover the third one next time.)
What’s new: Online stores offer all kinds of clothing, but search engines may suggest items of a different color or style than you want. Visiolinguistic Attention Learning, developed by Yanbei Chen with researchers at Queen Mary University of London and Amazon, hones product searches based on text input from shoppers.
Key insights: If you can create a picture that approximates the ideal product, you can search for similar images. Generating realistic images is hard, but comparing extracted features is much easier.
How it works: VAL learns to modify features extracted from a product image according to text input such as “I want it to have a light floral pattern.” Then it searches for other products with features similar to the modified product features.
- VAL learned from datasets that provide an image paired with text as input, and a photo of the corresponding product as output.
- VAL contains a text encoder network and an image encoder network. The image encoder extracts image features at a few levels of detail, for instance shapes and textures.
- A pair of transformers fuses the text and image features at each level of detail.
- One transformer is a variation on self-attention transformers. It identifies relationships between image and text features, and adjusts the image features to agree with the text features.
- The second transformer learns to identify features that are unchanged in the new product and copies them without modification.
- The element-wise sum of both transformers comprises the desired product’s features. VAL compares them with features extracted from product images in its database and returns the closest matches.
Results: The researchers put VAL head-to-head against TIRG, the previous state of the art in image search with text feedback using the Fashion200K dataset of garment photos with text descriptions. VAL achieved 53.8 percent recall of the top 10 recommended products, the fraction of search results that are relevant, compared to TIRG’s 43.7 percent. VAL also outperformed TIRG on the Shoes and FashionIQ datasets.
Why it matters: VAL provides a new method for interpreting images and text together, a useful skill in areas where either one alone is ambiguous.
We’re thinking: We’ll take the blue shirt!
A MESSAGE FROM DEEPLEARNING.AI
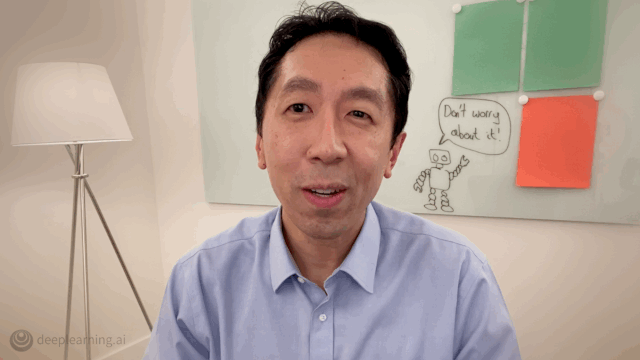
We’ve launched our much-anticipated Natural Language Processing Specialization! Courses 1 and 2 are live on Coursera. Enroll now
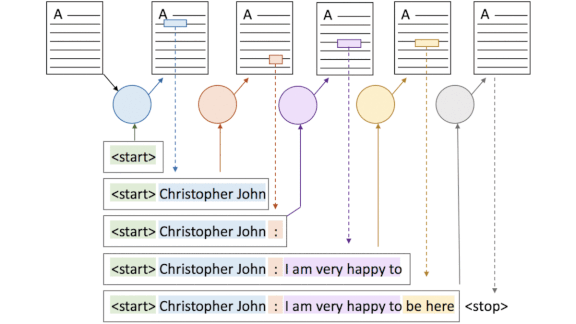
AI Makes Headlines
Which headline was written by a computer?
A: FIFA to Decide on 2022 World Cup in March
B: Decision in March on 48-team 2022 World Cup, Says Infantino
What’s new: Researchers at Primer, an AI-driven document analysis company, introduced an automatic headline generator. In an appealing twist, some articles that human publishers had tried to tart up with clickbait — for instance, You’ll Never Guess Which U.S. Counties Grew the Fastest — the model gave a sensible, informative headline: MacKenzie County in North Dakota Had Highest Population Growth in Entire U.S.
How it works: A headline is a very short document summary. Summarizers come in two flavors. Extractive models use only sentences or phrases from the text itself, building summaries that are closely related to the source but may be narrow or off-point. Abstractive models create new text based on an independent dictionary, synthesizing fresh but potentially confused summaries. Primer developed a hybrid model that generates abstractive headlines using vocabulary found in the document.
- The authors fine-tuned a Bert Question-Answer model from Hugging Face on 1.5 million news story/headline pairs drawn from sources including the New York Times, BBC, and CBC.
- The model frames headline generation as a series of question-answer tasks. The question is the beginning of the headline and the answer is the passage that makes up the next part. The model iterates this process sequentially through the document.
- The researchers also adapted the model to create bullet-point summaries of news articles, financial reports, and even movie plots — though not perfect. For instance, it declared that the character named Maverick in the 1986 Tom Cruise hit Top Gun enters a romantic relationship with his co-pilot Goose, rather than his instructor, per the actual plot.
Results: Human evaluators each read 100 news stories and graded two accompanying headlines, one written by a person and the other by the model. The computer-generated headlines scored slightly better overall. The model performed best on short-form journalism but stumbled on longer articles, probably because key information in longer items is more spread out.
Behind the News: Earlier headline generation methods mostly use an encoder-decoder to produce abstractive results. Unlike the new model, the encoder-decoder approach can generate any possible headline but risks poor grammar, factual inaccuracy, and general incoherence.
Why it matters: Imagine a world without clickbait!
We’re wondering: The computer wrote option A. Did you guess correctly?
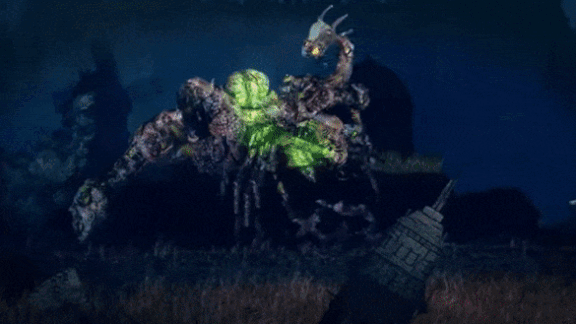
Monsters in Motion
How do you control a video game that generates a host of unique monsters for every match? With machine learning, naturally.
What’s new: The otherworldly creatures in Source of Madness learn how to target players through reinforcement learning, the developers told The Batch.
How it works: Players battle an infestation of fiends in a procedurally generated, side-scrolling wasteland.
- At the start of each level, the game uses non-neural computation to slap together a menagerie of unique monsters, each an assemblage of spidery legs, fireball-spitting tentacles, and bulbous carapaces. The monsters become more powerful as the game progresses.
- The endless variety of monsters makes traditional game-control techniques impractical. Instead, a feed-forward network trained on a sandbox simulation of the game receives a reward for a monster’s every step toward a player.
- The reinforcement learning environment comes from Unity, which makes 3D software development tools.
- The game’s developer, Carry Castle, is still fine-tuning it. The release date hasn’t been set, but you can request a test version here.
Behind the news: Most commercial titles use rules-based systems to control non-player characters. But some games have had success experimenting with neural networks.
- Supreme Commander 2, a war game similar to Starcraft, uses neural networks to decide whether the computer’s land, airborne, and naval units will fight or flee.
- The racing series Forza trains networks to imitate a human player’s style, such as how they take corners or how quickly they brake. These agents compete against other humans to earn points for the one they mimic.
Why it matters: Machine learning is infiltrating games as developers seek to build virtual worlds as variable and surprising as the real one.
We’re thinking: To all monsters, we say: keep learning!