Dear friends,
The unfolding Covid-19 crisis calls for individuals and organizations to step up and contribute to the common good. I believe that the tech community has an important role to play in slowing the progress of the virus and shortening the time it takes society to recover.
- Tech businesses can offer free or reduced-cost services, as well as extra support, to healthcare providers. I’m seeing a lot of unfulfilled needs in healthcare systems that communication and visualization tools might address. I’m providing IT support to doctor friends. Many of us can help with this.
- Individuals and organizations alike can combat fake news by calling out inaccurate and ill-informed perspectives and passing along accurate, timely information. Keeping digital channels free of misinformation and open for rapid dissemination of important news is critical.
- It’s especially important to encourage the free flow of information among researchers, healthcare systems, and epidemiologists, including data that can feed analytics or AI systems.
- Help others wherever you can, especially people in greater need.
In my neighborhood, I’ve been gratified to see people volunteering on a local messaging app (Nextdoor) to shop for groceries or help out the elderly. We all need to pull together and lend a hand wherever we can.
And of course, I hope you will take care of yourselves and your family.
Stay safe,
Andrew
News
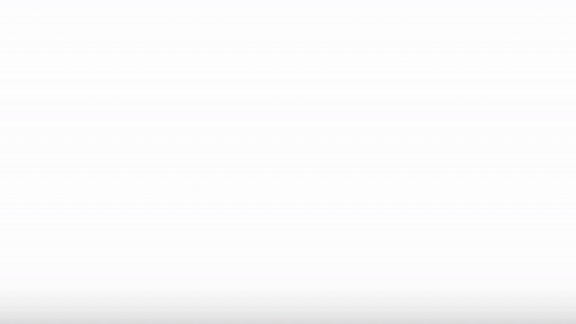
AI Takes on Coronavirus
Machine learning thrives on data, but information about the novel coronavirus and the illness it produces has been either thin or hard to access. Now researchers are pooling resources to share everything we do know.
What’s new: The White House and researchers from top U.S. AI and health institutions launched CORD-19, a free, machine-readable dataset of nearly 30,000 scholarly articles on the coronavirus. Kaggle is hosting a competition for text- and data-mining tools that sift this mountain of information for valuable insights.
Promising directions: Lack of data so far has limited AI’s usefulness in combating this outbreak, but stronger data-collection efforts could prove decisive in the next, according to MIT Technology Review. Author Will Douglas Heaven describes three areas to focus on:
- Prediction: Health surveillance companies spotted Covid-19 in late December by parsing news reports, social media, and official statements, but predicting how the epidemic will spread is harder. AI companies could do more if they were allowed access to patient records, but that would require working through thorny privacy issues. The U.S. recently finalized new rules for giving patients more control over their health data. What’s missing is an option for patients to share their data securely with researchers.
- Diagnosis: A number of tools analyze scans of patients’ lungs to detect coronavirus infections. These technologies can’t see the virus itself, however, only the damage it has caused — and by the time such damage is visible, the illness may have progressed too far to be treated easily. Small data techniques might do better, pending further research.
- Treatment: AI could accelerate discovery of new drugs and vaccines, though that will take time. DeepMind used its AlphaFold model to predict protein structures associated with the virus. If they’re verified, the information could aid efforts to develop treatments. Generative algorithms can model millions of molecules and sift through them to find potentially useful ones. More data on the disease’s evolution could accelerate that effort.
Behind the news: AI spotted the disease early, but humans still beat it to the punch. At least one Chinese doctor posted his concerns about what came to be known as Covid-19 on a WeChat group before AI health monitors issued their alerts. He later died of the virus.
Why it matters: AI has great potential to combat epidemics, and hopeful news reports bring attention to and support for the field. The community must work diligently while taking care not to encourage wildly inflated expectations and false hopes.
We’re thinking: Covid-19 isn’t the first pandemic, and sadly it won’t be the last. The AI community’s efforts to fight this virus will prove critical when the next one emerges. And there’s plenty we can do outside the medical sphere: Machine learning can help manage critical resources, coordinate responses, and optimize logistics. At this moment of international crisis, we face a common foe that is bigger than any of us, and we’re gratified to see so many AI developers eager to pitch in.
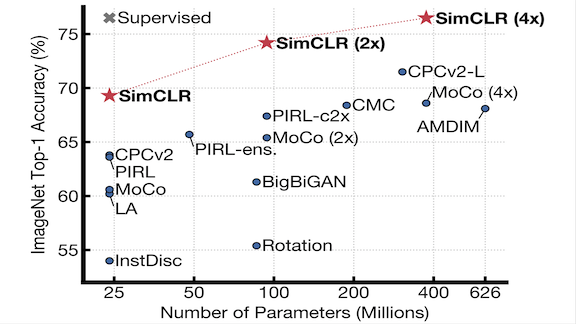
Self-Supervised Simplicity
A simple linear classifier paired with a self-supervised feature extractor outperformed a supervised deep learning model on ImageNet, according to new research.
What’s new: Ting Chen and colleagues at Google Brain devised a self-supervised training algorithm (a task that trains a model on unlabeled data to generate features helpful in performing other tasks). Simple Contrastive Learning (SimCLR) compares original and modified versions of images, so a model learns to extract feature representations that are consistent between the two.
Key insight: Images and variations produced by data-augmentation techniques such as rotation have similar features — so similar that they’re more informative than the labels such images also might share. SimCLR trains a model to extract features that are unchanged by such transformations, a technique known as contrastive learning.
How it works: Unlike other contrastive learning techniques, SimCLR can be used with any model architecture. It requires only multiple data-augmentation methods (which the researchers specify only for images, the subject of this study).
- During training, the researchers modify ImageNet examples, producing pairs that consist of an original and a variant altered by combinations of cropping, flipping, rotation, color distortion, blur, and noise.
- SimCLR trains a model to extract from each version feature vectors with minimal differences in the angles between them.
- The trained model can extract features from a labeled dataset for a downstream classifier that, in turn, learns to map the features to the labels. Alternatively, the model can be fine-tuned using labeled data, in effect using SimCLR as an unsupervised pre-training algorithm.
Results: A ResNet-50(x4) trained with SimCLR extracted features from ImageNet using all labels. A linear classifier trained on the resulting features achieved 76.5 percent top-1 accuracy, 0.1 percent better than a fully supervised ResNet-50. SimCLR achieved similar results on a variety of other image datasets.
Why it matters: Self-supervised learning schemes often rely on complicated tasks to extract features from unlabeled data. SimCLR simply extracts similar features from similar examples.
We’re thinking: This method seems like it would work well on audio data. We’re curious to see how effective it can be with text and other data types based on alphanumeric characters.
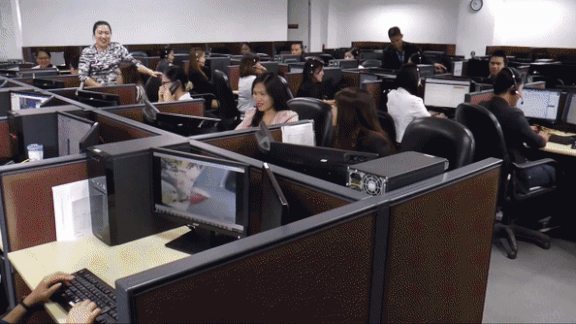
Workers Get the Jump on Automation
Businesses around the world outsource their customer service work to the Philippines. Now the Philippines is preparing its workers to outsource some tasks to AI.
What’s new: The Philippines’ booming call-center industry aims to train workers for more highly skilled jobs as AI takes over the easier ones, according to the South China Morning Post.
How it works: AI threatens half of the country’s 1.3 million outsourcing jobs, especially relatively simple work like answering simple inquiries via phone or email, according to the IT and Business Process Association of the Philippines. In response, IBPAP is launching a massive campaign to train workers in professions like data analytics and machine learning.
- The organization expects to start training 5,000 workers this year and a million by the end of 2022.
- Businesses are taking part, too. Genpact, a technical services firm that employs 6,500 Filipino workers, launched an upskilling app called Genome that connects its employees with co-workers who have skills they want to learn.
Behind the news: The Philippines has become India’s top competitor in the overseas call center business thanks in part to government-led efforts to improve technological literacy and communications infrastructure beginning in the early 1990s.
Why it matters: Outsourcing accounts for almost 10 percent of the Philippines economy, according to IBPAP. Upskilling programs cultivate workers, half of whom are women, into a garden of homegrown talent.
We’re thinking: Technical upskilling is a great way to transform outsourcing spokes into innovation hubs. AI is still young enough that there’s room for many such hubs around the world.
A MESSAGE FROM DEEPLEARNING.AI
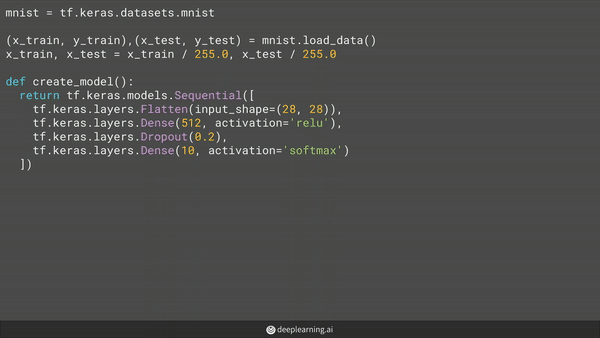
Explore federated learning and learn how to retrain deployed models while maintaining user privacy. Take the new course on advanced deployment scenarios in TensorFlow. Enroll now
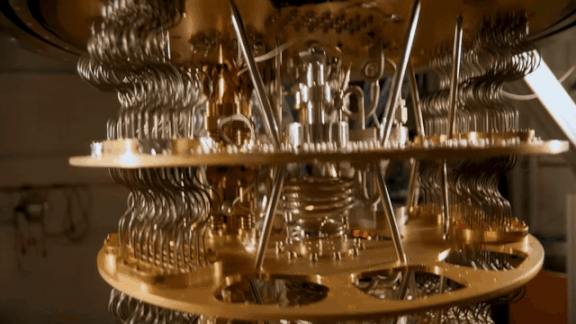
Quantum Leap
Quantum computing has made great strides in recent years, though it still faces significant challenges. If and when it gets here, machine learning may be ready for it.
What’s new: TensorFlow Quantum is a platform for building, training, and deploying neural networks on quantum processors. It was developed by Alphabet, Volkswagen, and the University of Waterloo.
How it works: The software works with quantum hardware like Google’s Sycamore computer, which has 54 qubits. Each qubit processes multiple calculations at once, theoretically enabling such systems to vastly outperform conventional CPUs.
- TFQ marries TensorFlow with Cirq, a library that makes it easier to map machine learning algorithms to quantum circuitry. Researchers can use Cirq to prototype quantum neural networks built with layers of quantum circuits, and then embed their models within a TensorFlow graph.
- The framework introduces two new concepts: quantum tensors and quantum layers. Quantum tensors are like normal tensors, but they store quantum superpositions (like storing many tensors at once, or storing a batch of tensors). Quantum layers operate on quantum tensors.
- Not all operations are supported, but those that are get a quantum speedup. You can mix quantum tensors and quantum layers with normal tensors and layers, but the conversions between quantum tensors and regular tensors are slow.
- Like the usual TensorFlow, the quantum version works with CPUs, GPUs, and TPUs, while adding QPUs to the mix.
Why it matters: Imagine that, instead of living one life, you could live billions of lives simultaneously, and at the end, you would have learned from all of them. Quantum speedups can be enormous for operations in which a single quantum computer can outperform the fastest supercomputer (that is, millions of classical computers working together). Machine learning could be one of those operations.
Yes, but: It may be a while before quantum computing is practical outside of research labs. Among other challenges, quantum systems are so sensitive that the noise they generate can derail their calculations.
Behind the news: Last year, Google claimed that Sycamore had achieved so-called quantum supremacy by performing a calculation that it deemed impractical for a classical supercomputer. IBM challenged the claim by solving the problem using conventional technology. The two tech giants, which are vying for leadership in the field, remain at loggerheads.
We’re thinking: Tech giants are always on the lookout for disruptions that may threaten their business. By creating tools for developers, they’re positioning themselves for a quantum future whether or not it arrives. Meanwhile, machine learning engineers have a shiny new toy to play with!
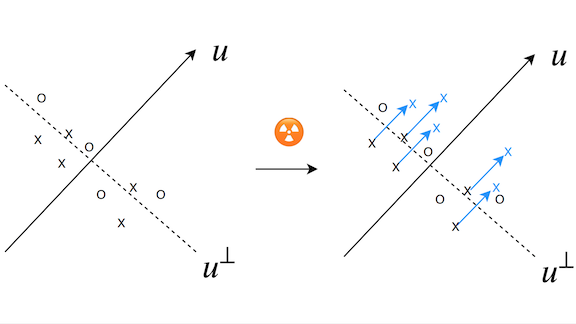
X Marks the Dataset
Which dataset was used to train a given model? A new method makes it possible to see traces of the training corpus in a model’s output.
What’s new: Alexandre Sablayrolles and colleagues at Facebook and France’s National Institute for Research in Computer Science and Automation adulterated training data with imperceptible signals. Decisions made by models trained on this so-called radioactive data showed signs of the altered corpus.
Key insight: Changes in training data can affect the loss in a trained model’s decisions. A small, consistent alteration in the training data affects the loss in a predictable way.
How it works: The researchers replaced a portion of images in a training corpus with marked images. After training a model on the whole dataset, they compared the model’s loss on small subsets of marked and unmarked images.
- Consider a two-dimensional classification task, as illustrated above. Displacing the features of examples in one class by a constant amount shifts the decision boundary. This change acts as a fingerprint for the altered dataset.
- Radioactive data extends this intuition to higher dimensions. The algorithm randomly chooses a direction to shift extracted features of each class. Then it learns how to modify input images most efficiently to produce the same shifts.
- There are several ways to identify training on radioactive data, depending on the model. A simple one is to compare the model’s loss for a given class on radioactive and unaltered data. A model trained on radioactive data has a lower loss value on radioactive images because the model recognizes the added structure.
Results: The researchers marked 1 percent of Imagenet and trained a Resnet-18 on the entire dataset. The model’s loss on subsets of radioactive and normal data differed by a statistically significant amount, confirming that the model had been trained on a portion of marked data, while accuracy declined by only 0.1 percent compared to training on standard Imagenet. Different architectures and datasets yielded similar results.
Why it matters: As neural networks become enmeshed more deeply in a variety of fields, it becomes more helpful to know how they were trained — say, to understand bias or track use of proprietary datasets. This technique, though nascent, offers a potential path to that goal.
We’re thinking: Beyond identifying training sets, radioactive data may offer a method to enforce data privacy by making it possible to identify models trained from improperly obtained private data.
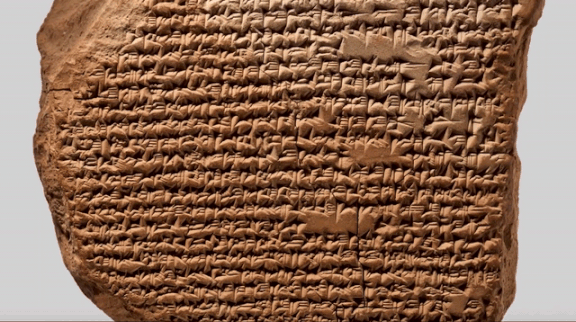
The King’s Moleskine
Machine learning promises to streamline handling of tomorrow’s bureaucratic drudgery — and, it turns out, that of 2,500 years ago.
What’s new: Computer vision is helping researchers at the University of Chicago translate a massive collection of ancient records inscribed on clay tablets.
How it works: Persian scribes around 500 BCE produced thousands of documents now collected in the Persepolis Fortification Archive. Researchers have been translating the cuneiform characters for decades. Now they hope to speed up the job with help from DeepScribe, a model built by computer scientist Sanjay Krishnan.
- The university began capturing digital images of the tablets in 2002. Students hand-labeled 100,000 symbols.
- DeepScribe was trained using 6,000 annotated images. It deciphered the test set with 80 percent accuracy.
- The researchers hope to build a generalized version that can decipher other ancient languages.
Behind the news: The archive mostly contains records of government purchases, sales, and transport of food, helping scholars develop a detailed understanding of life in the First Persian Empire. University of Chicago archaeologists found the tablets in 1933 near the palace sites of early Persian kings. They returned the artifacts to Iran in 2019.
Why it matters: DeepScribe’s current accuracy is good enough to automate translation of repetitive words and phrases, freeing up human attention for more specialized work like translating place names or deciphering particular words in context. The researchers also believe the model could be useful for filling in gaps on tablets where text has worn away or is indecipherable.
We’re thinking: These tablets hold an important lesson for all of us during tax season: Never throw away your receipts.