Dear friends,
I’m delighted that the crisis at OpenAI, which you can read about below, seems to have been resolved with an agreement in principle for Sam Altman to return as CEO after his sudden firing last week. OpenAI has many well-meaning employees, who have worked hard to innovate in AI and bring its benefits to others. Everyone at OpenAI has my congratulations for getting to a resolution so quickly! The team deserves kudos especially for focusing on customers even through the turmoil.
One positive take-away is that employees have power. It can be hard to be part of a large team. But through ways large and small, people doing the work can influence events in important ways. OpenAI employees banded together to demand changes in the board, and one or two engineers at any company can raise a concern. Wherever you work, use your voice to make things better!
So what’s next?
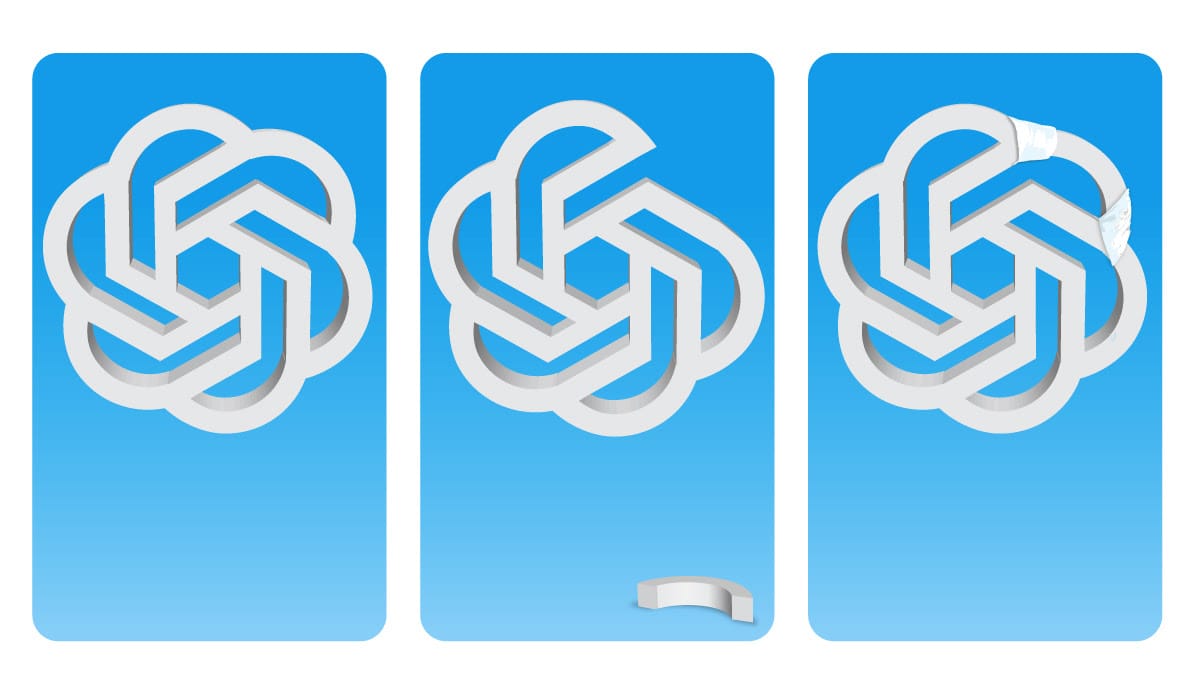
I see both hopeful and worrisome impacts as OpenAI picks up the pieces:
- The team’s camaraderie through this episode has been inspiring. Strong alignment within the team could lead to increased effectiveness. That would be great for AI innovation, the company, and its customers and users.
- A few media outlets, notably The Information and Bloomberg, demonstrated a strong ability to get scoops about what was happening. Many are saying that OpenAI will face increased scrutiny in the future.
- Bret Taylor (who helped Twitter navigate its sale to Elon Musk) and Larry Summers (former United States Secretary of the Treasury and Harvard president) are strong additions to the board. OpenAI has a small but efficient lobbying team that has been highly influential on global AI regulation, and Summers’ background makes him a valuable addition to such efforts. I look forward to a more diverse board as its membership grows.
- In recent days, I heard from multiple businesses that are looking for alternatives to the OpenAI API to ensure their own continuity of operations. The quick resolution of the crisis has stemmed much of the damage, but the fact that some customers are looking at backup options will be hard to reverse.
- The failure of OpenAI’s unusual for-profit/nonprofit corporate structure is glaring. Investors and donors will be more hesitant to fund organizations with novel structures (which often come with passionate arguments — which fell apart in the case of OpenAI — about why they’re better). In most companies, board oversight over the CEO’s performance would be good governance, and for a fired CEO to rally employees against the board and get their job back would be a sign of awful governance. But OpenAI’s previous board nearly destroyed so much value, for no apparent reason, that I’m glad employees helped reverse the decision. The reconstituted board has its work cut out for it to put in place robust governance.
ChatGPT was released on November 30, 2022. It is amazing how much has happened at OpenAI — and in the AI world — in less than one year! Brief stretches of chaos may be the price of moving fast. Nonetheless, I think moving fast (but responsibly) is better than going slowly.
I hope all employees everywhere will come away from this episode feeling empowered to speak up and make things better. Let’s keep building AI, exercise wisdom and foresight, and learn what lessons we can about corporate governance. It’s probably too much to hope that there won't be additional bumps in the road ahead for AI, but I remain optimistic about all the good we can do.
Keep learning!
Andrew
News
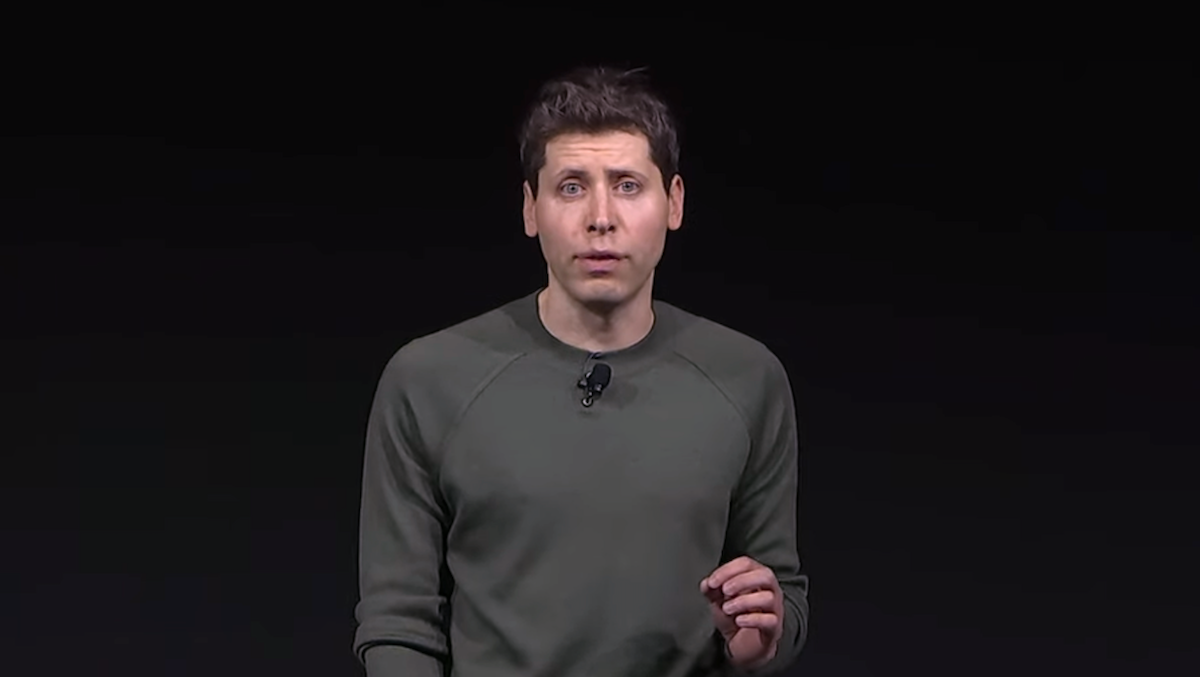
The CEO Is Out In
OpenAI abruptly fired and rehired its CEO Sam Altman, capping five days of chaos within the company.
What’s new: On Friday, the OpenAI board of directors — whose membership since has changed — ousted CEO and co-founder Sam Altman from his leadership position and his seat on the board. The board named chief technology officer Mira Murati interim CEO, soon replaced by Twitch co-founder Emmett Shear. Late Tuesday, Altman was reinstated and the board reorganized.
What happened: The dizzying events leave OpenAI with familiar leadership and a retooled board of directors. The new board, which is expected to expand, is chaired by Salesforce co-CEO Bret Taylor and includes economist Larry Summers and Quora CEO Adam D’Angelo (the sole holdover from the previous lineup). Leaving the board are Altman, co-founder and chief scientist Ilya Sutskever, entrepreneur Tasha McCauley, and AI safety researcher Helen Toner as well as president, co-founder, and former board chair Greg Brockman (who lost his seat in the turmoil, resigned, and returned with Altman).
- The circumstances surrounding Altman’s ouster remain mysterious. In explaining the decision, the earlier board said only that he had not been “consistently candid.” Chief operating officer Brad Lightcap wrote in an internal memo, “the board's decision was not made in response to malfeasance or anything related to our financial, business, safety, or security/privacy practices. This was a breakdown in communication between Sam and the board.”
- Altman learned of his dismissal on Friday in a call that co-founder and chief scientist Ilya Sutskever had scheduled the previous evening. The board briefed Microsoft, which owns 49 percent of OpenAI’s for-profit subsidiary, shortly thereafter, but it didn’t notify other investors. OpenAI’s management team learned that Altman had been fired from the public announcement.
- By the end of Friday, OpenAI president Greg Brockman had resigned along with three senior researchers and dozens of other staff. On Sunday, the board named Shear interim CEO. More than 90 percent of OpenAI employees – including Sutskever and Murati – signed an open letter threatening to leave if the board did not resign and reinstate Altman.
- While Altman was negotiating his return, Microsoft CEO Satya Nadella announced that he had hired Altman, Brockman, and the three senior researchers to staff an AI research division under Altman’s leadership.
Revolving door: OpenAI went through three CEOs within nearly as many days. Here’s who has passed through the revolving door.
- CEO Sam Altman co-founded OpenAI in 2015, while he was president of startup accelerator YCombinator, and became chief executive in 2019. He reoriented the company from research to products, gaining widespread recognition for the GPT series of large language models and the 2022 launch of ChatGPT. Lately he has invested in and raised money for other ventures including the biometric identity service Worldcoin, fusion-energy reactor builder Helion Energy, Humane’s AI Pin, and a chip company that would compete with Nvidia.
- Mira Murati served as interim CEO November 17 through November 19. She joined OpenAI in 2018 after working on AI products at Tesla and Leap Motion. She became OpenAI’s senior vice president of research, product, and partnerships in 2020 and CTO in 2022, leading development of ChatGPT, DALL·E, and other models. She championed the effort to reinstate Altman and Brockman during her stint as interim CEO.
- Emmett Shear was interim CEO November 19 through November 21. He was part of YCombinator’s initial cohort in 2005, co-founded the company that became Twitch in 2007, and sold it to Amazon for nearly $1 billion in 2014. He departed Twitch in early 2023. During his brief tenure at OpenAI, Shear threatened to resign unless the board provided evidence of Altman’s wrongdoing. Upon Altman’s return, he wrote on X, “I am deeply pleased by this result.”
Why it matters: At a moment when AI is undergoing rapid development and deepening division over the role of regulation, the chaos at OpenAI highlights the importance of strong corporate governance and an experienced board of directors that has a range of relevant experience and strong alignment with the company’s mission. It’s highly unusual for directors to fire a chief executive without arranging an orderly succession, coordinating with key investors, and preparing the market for changes. Chaos at the company opened competitive opportunities for rivals and threatened to destabilize thousands of companies that depend on OpenAI services. Although Altman’s return presumably restores the company’s stability, it will bear lingering questions and greater scrutiny going forward.
We’re thinking: There’s nothing normal about goings on at OpenAI. Nonetheless, as startup guru Eric Ries said, cofounder breakups and sometimes even boardroom coups are part of startup life. They’re unnerving, especially for people who depend on the companies involved (and vice-versa). We wish OpenAI’s employees, who have done a tremendous job of advancing AI and serving hundreds of millions of customers, renewed enthusiasm and focus as they resume their important work.
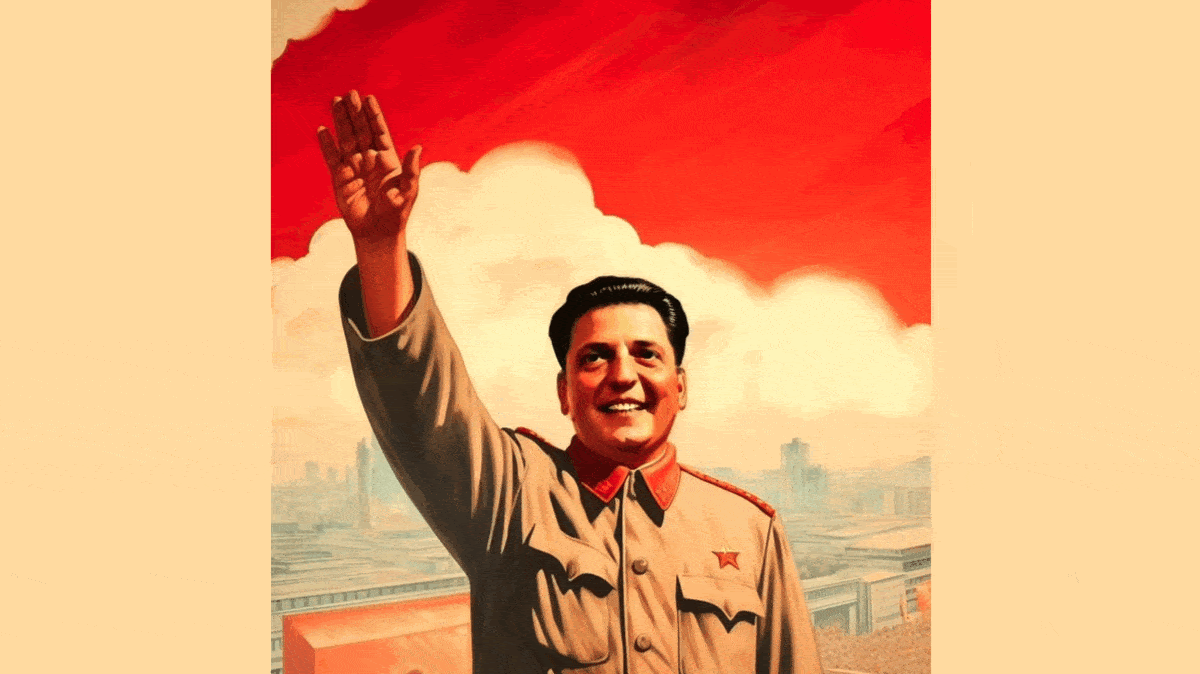
The Politics of Generative AI
Argentina’s recent presidential race was a battleground of AI-generated imagery.
What’s new: Candidates Javier Milei and Sergio Massa flooded social media with generated images of themselves and each other, The New York Times reported. On Sunday, Milei won the election’s final round.
How it works: No candidate earned enough votes to win the first round in late October, so front runners Milei, known for his hard-right libertarian economic views, and Massa, the incumbent government’s center-left economic minister, advanced to a run-off. The candidates generated a deluge of pictures and videos as the final vote neared.
- Milei’s campaign used a custom model based on Stable Diffusion to produce images of himself as a cartoon lion, while Massa’s campaign pictured its own candidate as the fearless Indiana Jones.
- Images posted by Massa’s campaign around Halloween depicted Milei as a zombie. Massa’s campaign also melded his opponent’s likeness into scenes from A Clockwork Orange and Fear and Loathing in Las Vegas, portraying Milei as psychologically unstable characters in those movies. Milei’s campaign struck back with an image that portrayed Massa in the garb and pose of Mao Zedong, founder of the People’s Republic of China.
- Most of the images were either labeled as AI-generated or obvious fabrications. However, Massa’s campaign posted on Instagram a fake video (since deleted) in which Milei proposed viewing children as a “long-term investment” in the market for human organs. Massa himself later disavowed the video.
- Another candidate used the existence of deepfakes to discredit a recording of her economic adviser apparently trading a job for sexual favors. The candidate noted that it’s easy to fake voices. The recording’s veracity has not been established.
What they’re saying: “I absolutely think it's a slippery slope. In a year from now, what already seems very realistic will only seem more so.” — Isabelle Frances-Wright, head of technology and society, Institute for Strategic Dialogue.
Behind the news: Deepfakes have appeared in campaign ads in India and South Korea. Earlier this year, Google mandated that advertisers in a number of democratic countries including Argentina clearly label AI-generated imagery in political ads distributed through Google ads, part of a global policy change. Meta will require that political advertisers clearly label AI-generated media in their ads beginning in 2024. Generated images in Argentina’s presidential campaign circulated on Meta’s Instagram network ahead of the deadline.
Why it matters: Argentina’s presidential campaign offers a glimpse of the future for democracies across the globe. Image generators are widely available, and political forces have proven willing to use them. AI-generated depictions of candidates may undermine voters’ trust in the media as a whole whether or not they’re intended to deceive, political scientists worry.
We’re thinking: Generated media poses a conundrum for democracy. Advertising has been shown to influence people even when audience members are aware of the effort to persuade. Yet free speech is essential to a healthy society. We favor mandatory labeling generated media in political ads and strong protection against defamation in hope that these measures will stem the most flagrant abuses.
A MESSAGE FROM DEEPLEARNING.AI
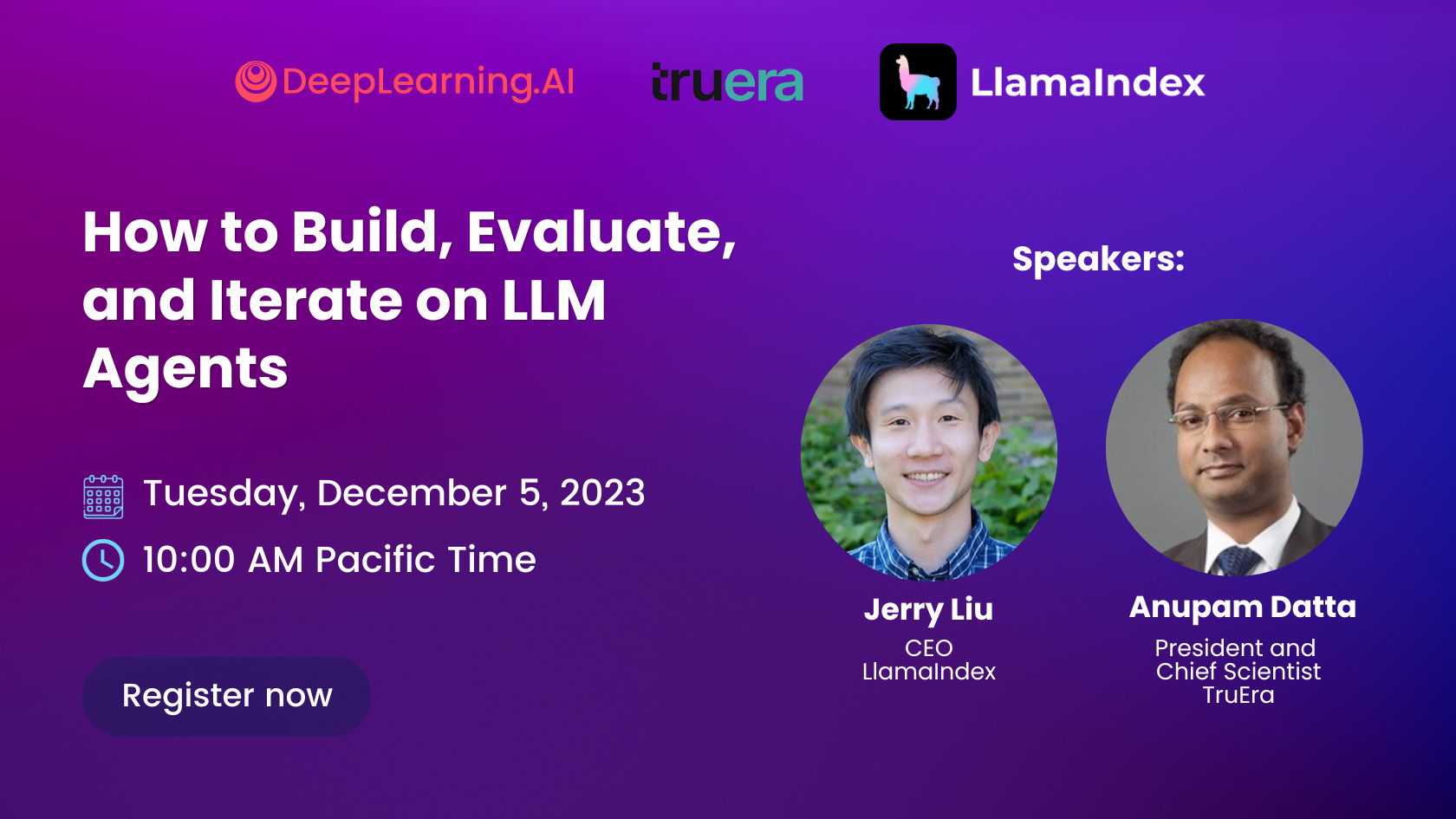
Agent applications are among the most in-demand uses of large language models (LLMs). This workshop will explore how to develop, evaluate, and iterate on LLM agents quickly and effectively. Register now
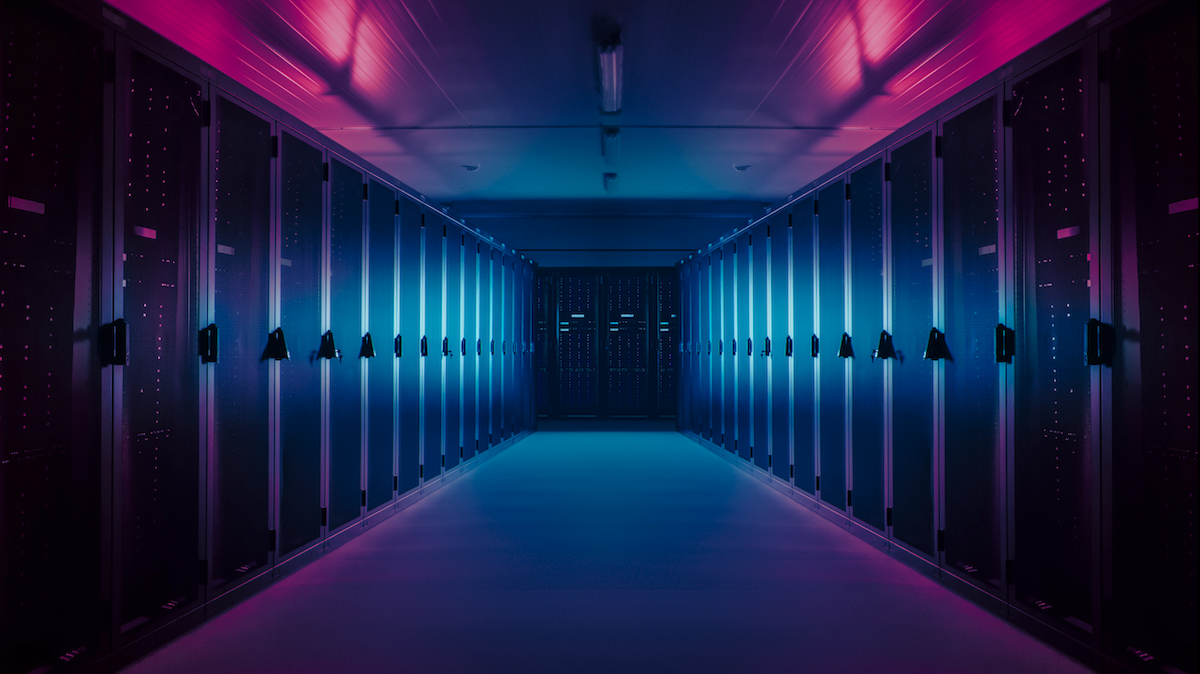
More Cloud GPUs on the Way
A new cloud-computing company promises to provide scarce AI processing power to startups and researchers.
What’s new: Voltage Park, a nonprofit north of Silicon Valley, will offer processing power from 24,000 top-of-the-line Nvidia H100 graphics processing units (GPUs) — roughly $500 million worth — at competitive prices. Rival suppliers of cloud-based GPUs are oversubscribed as the chips continue to be in short supply.
How it works: The company, which is bankrolled by cryptocurrency billionaire Jed McCaleb, plans to build data centers in Texas, Virginia, and Washington.
- Voltage Park will charge hourly rates for up to 8 dedicated GPUs. Prices start at $1.89 per hour for a single GPU. In comparison, AWS’s least expensive package offers 8 GPUs for about $43 per hour with a three-year commitment, or $98 per hour on-demand.
- Customers who need more H100s will be able to use up to 248 of the chips on a short-term lease or up to 4,088 on a year-long lease.
- The company is serving select startups including Character AI and Atomic AI. It will welcome other startups, nonprofits, and research institutions in January 2024.
Behind the news: A shortage of Nvidia’s high-end GPUs, which are optimized to process machine learning workloads, has bedeviled organizations that aim to join the generative AI boom. Businesses are scrambling to manage the demand.
- Engineers and entrepreneurs have been paying heavy premiums for the chips, if they are available at all.
- Cloud provider CoreWeave borrowed $2.3 billion to build a cluster of 45,000 Nvidia GPUs. That provider’s H100 prices start at $4.76 per hour.
- China is also facing a GPU shortage, but for a different reason: Last year, the U.S. government imposed restrictions — and recently tightened them — on sales of high-performance chips produced by U.S. companies to Chinese customers. Baidu ordered 1,600 AI chips from Huawei, a sign that homegrown alternatives may be emerging.
Why it matters: Training and serving state-of-the-art AI systems requires huge amounts of processing power. Thus AI startups are facing serious obstacles amid the scarcity of specialized hardware. Larger companies have either their own processing power or strong relationships with cloud providers. Smaller providers such as DataCrunch, Lambda Labs, and Paperspace have limited supply. As generative AI booms, organizations that can provide access to GPUs on flexible terms are likely to find takers.
We’re thinking: Voltage Park is a subsidiary of McCaleb’s philanthropic organization, and its profits will fund the organization’s activities, about which its website offers no information. Nonprofit status can be a prelude to for-profit business. We’re curious to see where this company is headed.
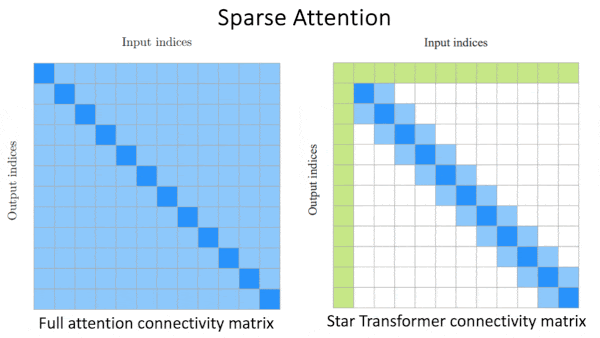
Taming Transformers
The transformer architecture is astonishingly powerful but notoriously slow. Researchers have developed numerous tweaks to accelerate it — enough to warrant a look at how these alternatives work, their strengths, and their weaknesses.
What’s new: Quentin Fournier, Gaétan Marceau Caron, and Daniel Aloise surveyed variations on the transformer, evaluating methods designed to make it faster and more efficient. This summary focuses on the variations designed to accelerate it.
The cost of attention: The attention mechanism in the original transformer places a huge burden on computation and memory; O(n2) cost where n is the length of the input sequence. As a transformer processes each token (often a word or pixel) in an input sequence, it concurrently processes — or “attends” to — every other token in the sequence. Attention is calculated by multiplying two large matrices of weights before passing the resulting matrix through a softmax function. The softmax function normalizes the matrix values to a probability distribution, bringing higher values closer to 1 and lower values near 0. This enables the transformer, when encoding a token, to use relevant tokens and ignore irrelevant tokens.
(Modified) attention is all you need: The authors identify three approaches to accelerating transformers. Two of them optimize the attention mechanism and the third optimizes other parts of the architecture.
- Sparse attention. These approaches simplify the attention calculation by using a subset of weights and setting the rest to 0. They mix and match three general patterns in which the position of a given token in a sequence determines how it attends to other tokens: (i) a token attends to all other tokens, (ii) a token attends only to directly neighboring tokens, or (iii) a token attends to a random selection of tokens. For instance, in Star Transformer, the first token attends to all other tokens and the other tokens attend only to neighbors. Calculating attention with sparse matrices is faster than usual thanks to fast sparse matrix multiplication algorithms. However, because it processes only a subset of the original attention weights, this approach degrades performance slightly. Further, because sparse attention patterns are handcrafted, they may not work well with all data and tasks.
- Factorized attention. Approaches in this category modify attention calculations by approximating individual matrices as the product of two (or more) smaller matrices. This technique enables Linformer to cut memory requirements by a factor of 10 compared to the original transformer. Factorized attention methods outperform sparse attention in some tasks, such as determining whether two dots in an image are connected by a path that consists of dashes. However, they’re less effective in other areas, such as classifying images and compressing long sequences for retrieval.
- Architectural changes. These approaches retain the original attention mechanism while altering other aspects of transformer architecture. One example is adding an external memory. With the original transformer, if an input sequence is too long, the model breaks it into smaller parts and processes them independently. Given a long document, by the time it reaches the end, it doesn’t have a memory of what happened at the beginning. Transformer-XL and Compressive Transformer store embeddings of earlier parts of the input and use them to embed the current part. Compared to the original transformer of the same size, Transformer-XL was able to improve its performance based on training examples that were 4.5 times longer.
Yes, but: It’s difficult to compare the results achieved by these variations due to differences in model size and hyperparameters (which affect performance) and hardware used (which affects speed). Further, some transformer variations utilize multiple modifications, making it hard to isolate the benefit of any particular one.
Why it matters: These variations can help machine learning engineers manage compute requirements while taking advantage of state-of-the-art approaches.
We’re thinking: The authors of Long Range Arena built a dashboard that reports performance of various transformers depending on the task. We welcome further efforts to help developers understand the tradeoffs involved in different variations.
Data Points
Cambridge Dictionary declares AI-induced 'Hallucinate' Word of the Year 2023
The Cambridge Dictionary expanded the definition of the word to include the false information produced by large language models. The acknowledgment of AI 'hallucinations' underscores the evolving vocabulary surrounding the capabilities of language models. (University of Cambridge)
'Make It Real' prototype transforms drawings into functional software
Tldraw, a collaborative digital whiteboard, launched a prototype of a feature that allows users to turn vector drawings into functional software. A live demo of the GPT-4V powered tool is available to the public. (Ars Technica)
Research: Text-to-image AI models vulnerable to 'SneakyPrompt' jailbreaking are generating disturbing content
Prominent text-to-image AI models, including Stabile Diffusion and DALL-E 2, face a significant security breach. Security researchers revealed the "SneakyPrompt" method, which uses reinforcement learning. SneakyPrompt enables the generation of seemingly nonsensical prompts that AI models learn to recognize as hidden requests for inappropriate images. Stability AI and OpenAI are already collaborating with the researchers to strengthen defenses against such attacks. (MIT Technology Review)
Amazon announces job cuts in Alexa division, shifting focus to generative AI
Daniel Rausch, the Vice President of Alexa and Fire TV, stated in an internal memo that the shifts are intended to maximize resources for generative AI. The company recently previewed a generative AI-based Alexa feature called "Let’s Chat," emphasizing longer and more context-aware conversations with the voice assistant. (GeekWire)
Google launches Project Open Se Cura, an open source framework for secure and efficient AI
The framework emphasizes co-design and development, focusing on security, transparency, and scalability. Google released the code base, including design tools and IP libraries, to foster open development and transparency in AI system design. (Google Open Source)
Google-backed AI research lab, Kyutai, aims for open science with $330 million budget
French billionaire Xavier Niel unveiled details about Kyutai, a newly established AI research lab in Paris with plans to release not only open source models but also training source code and data. French President Emmanuel Macron supports the initiative, emphasizing the need to regulate AI use cases rather than model makers. (TechCrunch)
GPT-4 outperforms humans on lawyer ethics exam
The model surpassed the average scores of human test-takers on the Multistate Professional Responsibility Exam (MPRE), a legal ethics test required by almost every U.S. state for practicing law. GPT-4 achieved a 74% accuracy rate on the simulated exam, outperforming the estimated 68% average among humans. The study, conducted by LegalOn Technologies, suggests that AI could play a role in assisting lawyers with ethical compliance in the future. (Reuters)
Google DeepMind and YouTube present Lyria, an advanced AI music generation model
Lyria, designed to generate high-quality music with instrumentals and vocals, aims to address the challenges of maintaining musical continuity across various elements like beats, individual notes, and vocal harmonies. The announcement includes two AI experiments: "Dream Track," an experiment within YouTube Shorts allowing creators to connect with fans through AI-generated soundtracks featuring global artists; and "Music AI Tools," a set of tools developed with artists, songwriters, and producers to enhance their creative processes. (Google DeepMind)
Microsoft introduces custom-designed chips for Azure
The Azure Maia AI Accelerator for AI tasks and generative AI, and the Azure Cobalt CPU, an Arm-based processor optimized for general-purpose compute workloads, will be integrated into custom server boards and racks. The chips will be working in tandem with software to maximize performance, flexibility, and efficiency. (Microsoft)
Microsoft and Google collaborate on OneTable project to address data lake challenges
The open source project seeks to create a layer on top of existing data lake table formats like Apache Iceberg, Apache Hudi, and Delta Lake, enabling seamless conversions and access across these formats. The project promotes interoperability, preventing vendor lock-in and facilitating compatibility for data analytics and AI workloads. (VentureBeat)
Microsoft teams up with Be My Eyes to offer GPT-4-powered support for visually impaired users
The tool enables visually impaired users to independently resolve technical issues and perform tasks without human agent assistance. During tests, only 10 percent of users opted to speak with a human representative after interacting with the AI tool. (The Verge)
OpenAI temporarily halts new ChatGPT Plus subscriptions and upgrades
Overwhelming demand led to capacity challenges, prompting a decision to pause access to ensure a high-quality experience for existing users. The move follows a series of outages related to high demand and DDoS attacks on OpenAI services, impacting ChatGPT and the API. (Search Engine Journal)
Common Sense Media flags generative AI models unsafe for kids
The organization introduced "nutrition labels" for AI products, evaluating them based on principles such as trust, safety, privacy, transparency, accountability, learning, fairness, social connections, and benefits to society. The generative AI category received lower ratings due to biases and concerns related to objectification and sexualization. (TechCrunch)