Dear friends,
Many laws will need to be updated to encourage beneficial AI innovations while mitigating potential harms. One example: Copyright law as it relates to generative AI is a mess! That many businesses are operating without a clear understanding of what is and isn’t legal slows down innovation. The world needs updated laws that enable AI users and developers to move forward without risking lawsuits.
Legal challenges to generative AI are on the rise, as you can read below, and the outcomes are by no means clear. I’m seeing this uncertainty slow down the adoption of generative AI in big companies, which are more sensitive to the risk of lawsuits (as opposed to startups, whose survival is often uncertain enough that they may have much higher tolerance for the risk of a lawsuit a few years hence).
Meanwhile, regulators worldwide are focusing on how to mitigate AI harm. This is an important topic, but I hope they will put equal effort into crafting copyright rules that would enable AI to benefit more people more quickly.
Here are some questions that remain unresolved in most countries:
- Is it okay for a generative AI company to train its models on data scraped from the open internet? Access to most proprietary data online is governed by terms of service, but what rules should apply when a developer accesses data from the open internet and has not entered into an explicit agreement with the website operator?
- Having trained on freely available data, is it okay for a generative AI company to stop others from training on its system’s output?
- If a generative AI company’s system generates material that is similar to existing material, is it liable for copyright infringement? How can we evaluate the allowable degree of similarity?
- Research has shown that image generators sometimes copy their training data. While the vast majority of generated content appears to be novel, if a customer (say, a media company) uses a third-party generative AI service (such as a cloud provider’s API) to create content, reproduces it, and the content subsequently turns out to infringe a copyright, who is responsible: the customer or the cloud provider?
- Is automatically generated material protected by copyright, and if so, who owns it? What if two users use the same generative AI model and end up creating similar content — will the one who went first own the copyright?
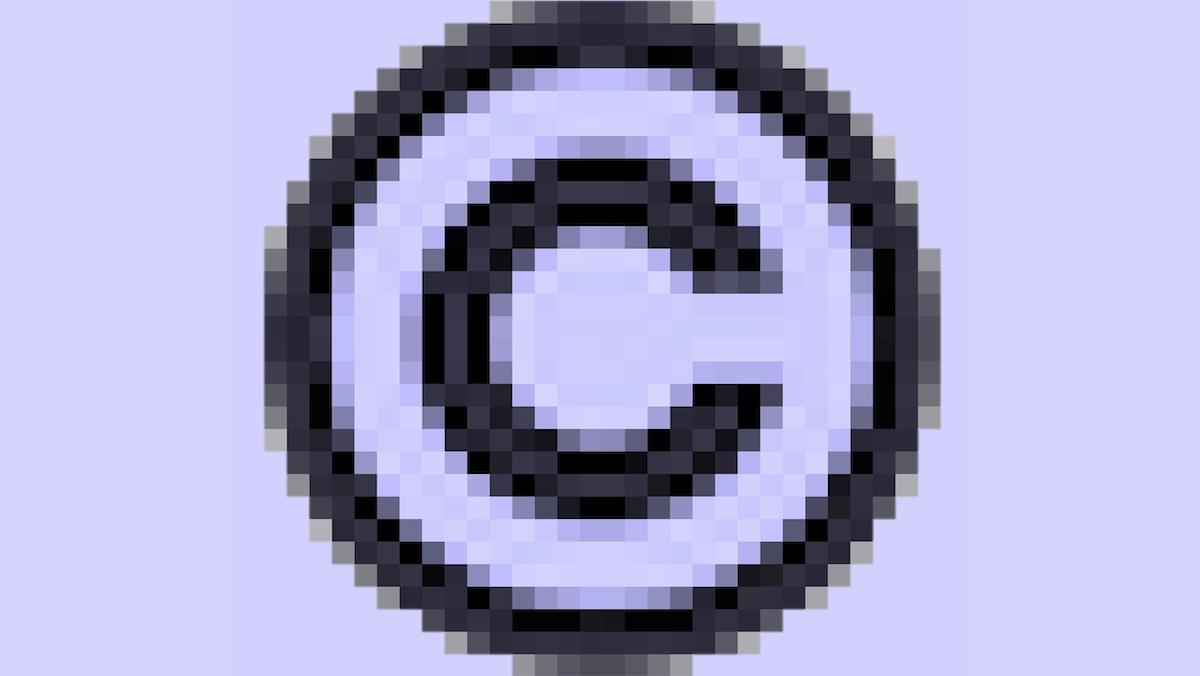
Here’s my view:
- I believe humanity is better off with permissive sharing of information. If a person can freely access and learn from information on the internet, I’d like to see AI systems allowed to do the same, and I believe this will benefit society. (Japan permits this explicitly. Interestingly, it even permits use of information that is not available on the open internet.)
- Many generative AI companies have terms of service that prevent users from using output from their models to train other models. It seems unfair and anti-competitive to train your system on others’ data and then stop others from training their models on your system’s output.
- In the U.S., “fair use” is poorly defined. As a teacher who has had to figure out what I am and am not allowed to use in a class, I’ve long disliked the ambiguity of fair use, but generative AI makes this problem even more acute. Until now, our primary source of content has been humans, who generate content slowly, so we’ve tolerated laws that are so ambiguous that they often require a case-by-case analysis to determine if a use is fair. Now that we can automatically generate huge amounts of content, it’s time to come up with clearer criteria for what is fair. For example, if we can algorithmically determine whether generated content overlaps by a certain threshold with content in the training data, and if this is the standard for fair use, then it would unleash companies to innovate while still meeting a societally accepted standard of fairness.
If it proves too difficult to come up with an unambiguous definition of fair use, it would be useful to have “safe harbor” laws: As long as you followed certain practices in generating media, what you did would be considered non-infringing. This would be another way to clarify things for users and generative AI companies.
The tone among regulators in many countries is to seek to slow down AI’s harms. While that is important, I hope we see an equal amount of effort put into accelerating AI’s benefits. Sorting out how we should change copyright law would be a good step. Beyond that, we need to craft regulations that clarify not just what’s not okay to do — but also what is explicitly okay to do.
Keep learning!
Andrew
News
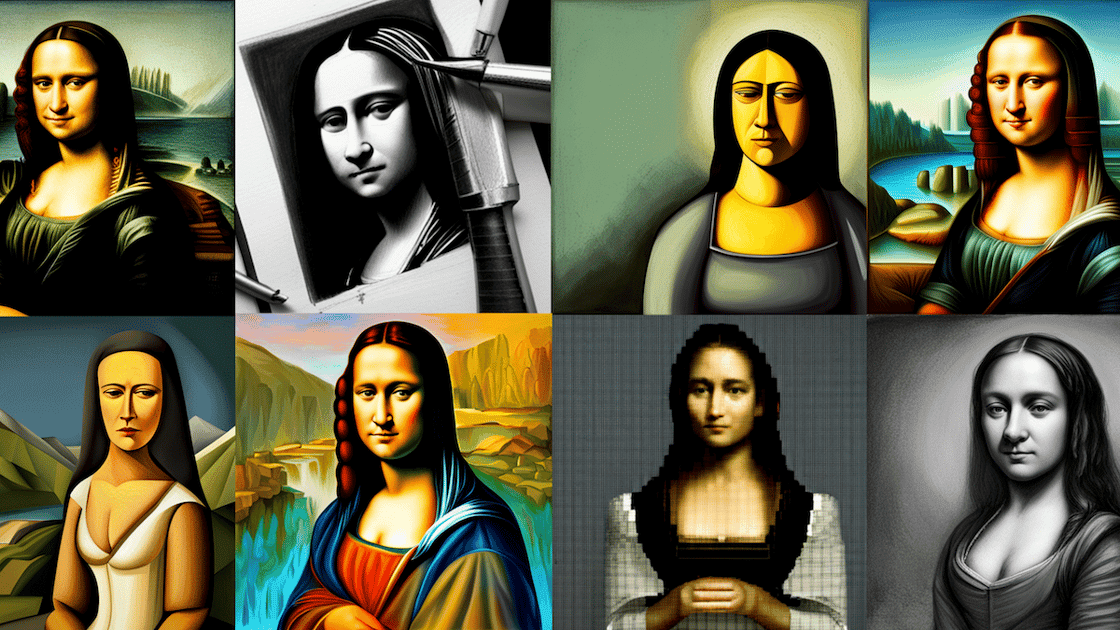
Copyright Owners Take AI to Court
AI models that generate text, images, and other types of media are increasingly under attack by owners of copyrights to material included in their training data.
What’s happening: Writers and artists filed a new spate of lawsuits alleging that AI companies including Alphabet, Meta, and OpenAI violated their copyrights by training generative models on their works without permission. Companies took steps to protect their interests and legislators considered the implications for intellectual property laws.
Lawsuits and reactions: The lawsuits, which are ongoing, challenge a longstanding assumption within the AI community that training machine learning models is allowed under existing copyright laws. Nonetheless, OpenAI responded by cutting deals for permission to use high-quality training data. Meanwhile, the United States Senate is examining the implications for creative people, tech companies, and legislation.
- Unnamed plaintiffs sued Alphabet claiming that Google misused photos, videos, playlists, and the like posted to social media and information shared on Google platforms to train Bard and other systems. One alleged that Google misused a book she wrote. Â The plaintiffs filed a motion for class-action status. This action echoes an earlier lawsuit against OpenAI filed in June.
- Comedian Sarah Silverman joined authors Christopher Golden and Richard Kadrey in separate lawsuits against Meta and OpenAI in a United States federal court. The plaintiffs, who are seeking class-action status, claim that the companies violated their copyrights by training LLaMA and ChatGPT, respectively, on books they wrote.
- In a similar lawsuit authors Paul Tremblay and Mona Awad allege that OpenAI violated their copyrights.
- OpenAI agreed to pay Associated Press for news articles to train its algorithms — an arrangement heralded as the first of its kind. OpenAI will have access to articles produced since 1985, and Associated Press will receive licensing fees and access to OpenAI technology. In a separate deal, OpenAI extended an earlier agreement with Shutterstock that allows it to train on the stock media licensor’s images, videos, and music for six years. In return, Shutterstock will continue to offer OpenAI’s text-to-image generation/editing models to its customers.
- A U.S. Senate subcommittee on intellectual property held its second hearing on AI’s implications for copyright. The senators met with representatives of Adobe and Stability AI as well as an artist, a law professor, and a lawyer for Universal Music Group, which takes in roughly one-third of the global revenue for recorded music.
Behind the news: The latest court actions, which focus on generated text, follow two earlier lawsuits arising from different types of output. In January, artists Sarah Anderson, Kelly McKernan, and Karla Ortiz (who spoke in the Senate hearing) sued Stability AI, Midjourney, and the online art community DeviantArt. In November, two anonymous plaintiffs sued GitHub, Microsoft, and OpenAI saying the companies trained the Copilot code generator using routines from GitHub repositories in violation with open source licenses.
Why it matters: Copyright laws in the United States and elsewhere don’t explicitly forbid use of copyrighted works to train machine learning systems. However, the technology’s growing ability to produce creative works, and do so in the styles of specific artists and writers, has focused attention on such use and raised legitimate questions about whether it’s fair. This much is clear: The latest advances in machine learning have depended on free access to large quantities of data, much of it scraped from the open internet. Lack of access to corpora such as Common Crawl, The Pile, and LAION-5B would put the brakes on progress or at least radically alter the economics of current research This would degrade AI’s current and future benefits in areas such as art, education, drug development, and manufacturing to name a few.
We’re thinking: Copyright laws are clearly out of date. We applaud legislators who are confronting this problem head-on. We hope they will craft laws that, while respecting the rights of creative people, preserve the spirit of sharing information that has enabled human intelligence and, now, digital intelligence to learn from that information for the benefit of all.
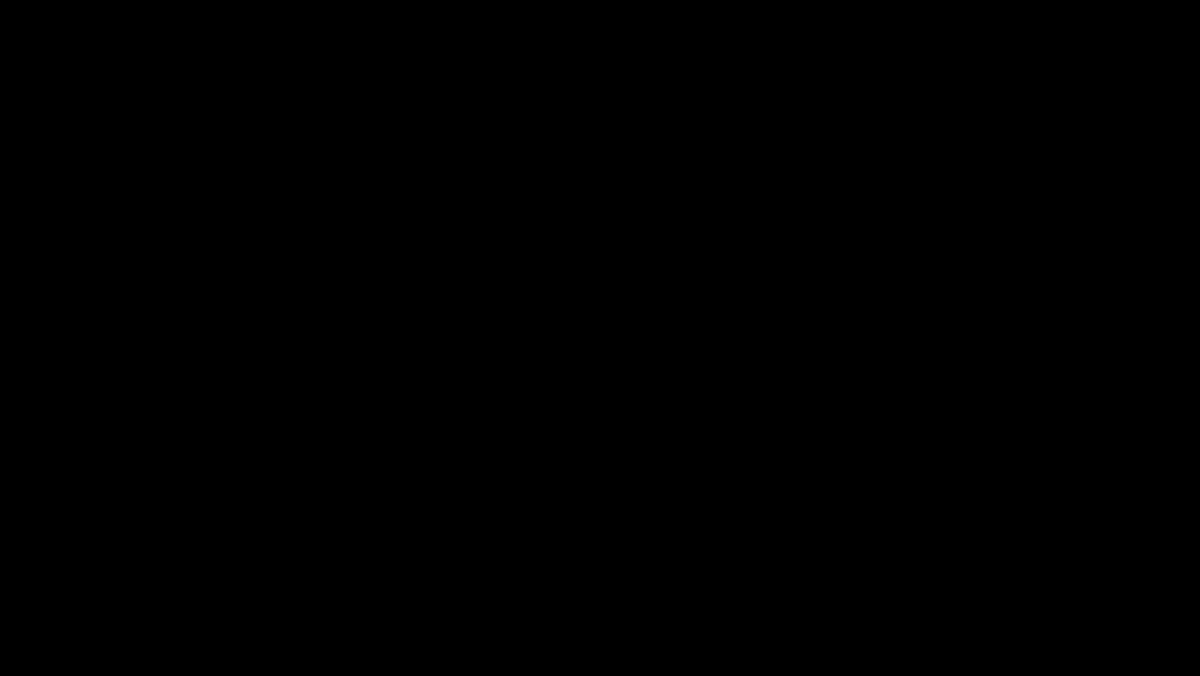
Chatbot Cage Match
A new online tool ranks chatbots by pitting them against each other in head-to-head competitions.
What’s new: Chatbot Arena allows users to prompt two large language models simultaneously and identify the one that delivers the best responses. The result is a leaderboard that includes both open source and proprietary models.
How it works: When a user enters a prompt, two separate models generate their responses side-by-side. The user can pick a winner, declare a tie, rule that both responses were bad, or continue to evaluate by entering a new prompt.
- Chatbot Arena offers two modes: battle and side-by-side. Battle mode includes both open source and proprietary models but identifies them only after a winner has been chosen. Side-by-side mode lets users select from a list of 16 open source models.
- The system aggregates these competitions and ranks models according to the metric known as Elo, which rates competitors relative to one another. Elo has no maximum or minimum score. A model that scores 100 points more than an opponent is expected to win 64 percent of matches against it, and a model that scores 200 points more is expected to win 76 percent of matches.
Who’s ahead?: As of July 19, 2023, OpenAI’s GPT-4 topped the leaderboard. Two versions of Anthropic’s Claude rank second and third. GPT-3.5-turbo holds fourth place followed by two versions of Vicuna (LLaMA fine-tuned on shared ChatGPT conversations).
Why it matters: Typical language benchmarks assess model performance quantitatively. Chatbot Arena provides a qualitative score, implemented in a way that can rank any number of models relative to one another.
We’re thinking: In a boxing match between GPT-4 and the 1960s-vintage ELIZA, we’d bet on ELIZA. After all, it used punch cards.
A MESSAGE FROM DEEPLEARNING.AI

Check out our course on “Generative AI with Large Language Models”! Developers hold the key to leveraging LLMs as companies embrace this transformative technology. Take this course and confidently build prototypes for your business. Enroll today
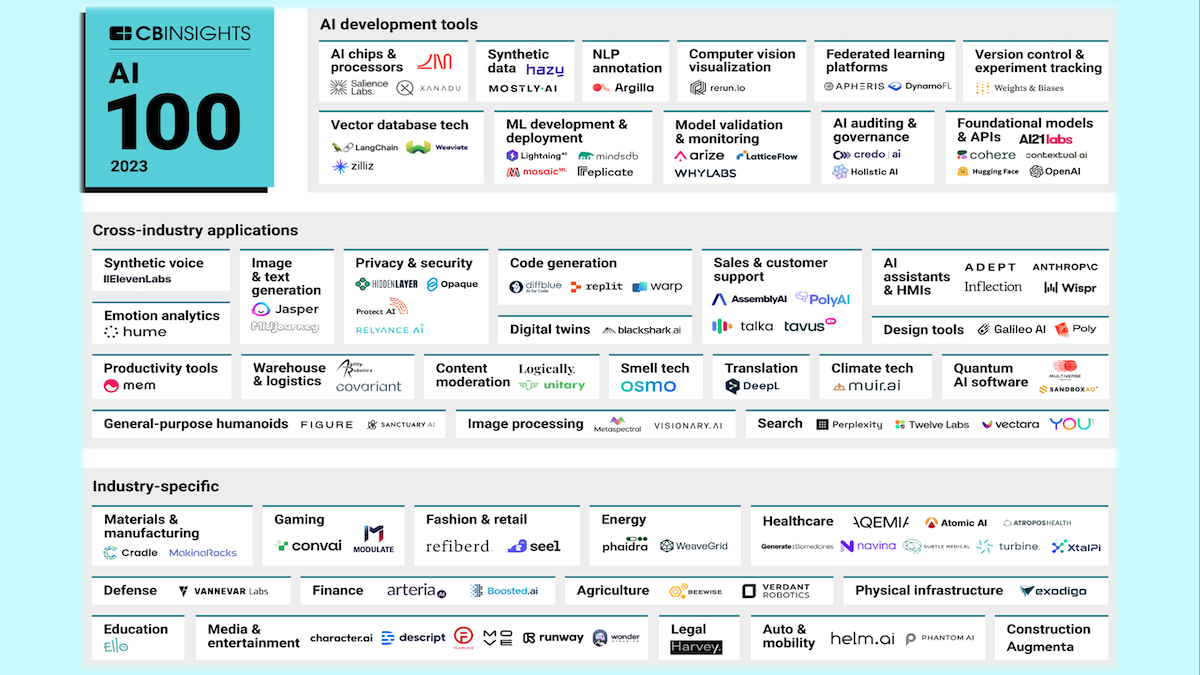
What Venture Investors Want
This year’s crop of hot startups shows that generative AI isn’t the only game in town.
What’s new: CB Insights, which tracks the tech-startup economy, released the 2023 edition of its annual AI 100, a list of 100 notable AI-powered ventures. The researchers considered 9,000 startups and selected 100 standouts based on criteria such as investors, business partners, research and development activity, and press reports.
Where the action is: The list divides roughly evenly into three categories: Startups that offer tools for AI development, those that address cross-industry functions, and those that serve a particular industry. The names of the companies are noteworthy, but the markets they serve are more telling.
- The AI tools category is dominated by ventures that specialize in foundation models and APIs (five companies including familiar names like OpenAI and Hugging Face) and machine learning development and deployment (four). AI chips, model validation/monitoring, and vector databases are represented by three companies each (including WhyLabs and Credo — both portfolio companies of AI Fund, the venture studio led by Andrew Ng).
- Among cross-industry startups, the largest concentrations are in AI assistants, privacy/security, sales/customer support, and search (four companies each). Code generation has three entries.
- The industry-focused startups concentrate in healthcare (eight companies) and media/entertainment (six). Agriculture, auto/mobility, energy, fashion/retail, finance, gaming, and materials/manufacturing are represented by two companies each.
Follow the money: Together, these startups have raised $22 billion in 223 deals since 2019. (Microsoft’s investment in OpenAI accounts for a whopping $13 billion of that total.) Half are in the very early stages.
- Venture capital is flowing to generative applications. The media/entertainment category is full of them: Character.ai provides chatbots that converse in the manner of characters from history and fiction, Descript helps amateur audio and video producers automate their workflow, Flawless provides video editing tools that conform actors’ lips to revised scripts and alternative languages, Runway generates video effects and alterations, and Wonder Dynamics makes it easy to swap and manipulate characters in videos.
- Some of the most richly capitalized companies in the list focus on safe and/or responsible AI development. For instance, Anthropic, which builds AI products that emphasize safety, received $300 million from Google. Cohere, which builds language models designed to minimize harmful output, recently raised $270 million.
Why it matters: Venture funding drives a significant portion of the AI industry. That means opportunities for practitioners at both hot ventures and me-too companies that seek to cultivate similar markets. The startup scene is volatile — as the difference between this year’s and last year’s AI100 demonstrates — but each crop of new firms yields a few long-term winners.
We’re thinking: Startup trends are informative, but the options for building a career in AI are far broader. Established companies increasingly recognize their need for AI talent, and fresh research opens new applications. Let your interests lead you to opportunities that excite and inspire you.
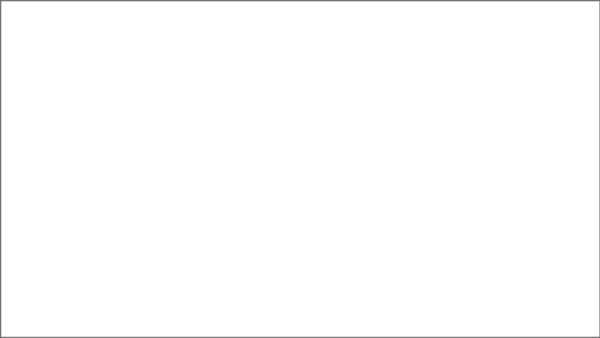
Optimizer Without Hyperparameters
During training, a neural network usually updates its weights according to an optimizer that’s tuned using hand-picked hyperparameters. New work eliminates the need for optimizer hyperparameters.
What’s new: Luke Metz, James Harrison, and colleagues at Google devised VeLO, a system designed to act as a fully tuned optimizer. It uses a neural network to compute the target network’s updates.
Key insight: Machine learning engineers typically find the best values of optimizer hyperparameters such as learning rate, learning rate schedule, and weight decay by trial and error. This can be cumbersome, since it requires training the target network repeatedly using different values. In the proposed method, a different neural network takes the target network’s gradients, weights, and current training step and outputs its weight updates — no hyperparameters needed.
How it works: At every time step in the target network’s training, an LSTM generated the weights of a vanilla neural network, which we’ll call the optimizer network. The optimizer network, in turn, updated the target network. The LSTM learned to generate the optimizer network’s weights via evolution — iteratively generating a large number of similar LSTMs with random differences, averaging them based on which ones worked best, generating new LSTMs similar to the average, and so on — rather than backpropagation.
- The authors randomly generated many (on the order of 100,000) target neural networks of various architectures — vanilla neural networks, convolutional neural networks, recurrent neural networks, transformers, and so on — to be trained on tasks that spanned image classification and text generation.
- Given an LSTM (initially with random weights), they copied and randomly modified its weights, generating an LSTM for each target network. Each LSTM generated the weights of a vanilla neural network based on statistics of the target network. These statistics included the mean and variance of its weights, exponential moving averages of the gradients over training, fraction of completed training steps, and training loss value.
- The authors trained each target network for a fixed number of steps using its optimizer network. The optimizer network took the target network’s gradients, weights, and current training step and updated each weight, one by one. Its goal was to minimize the loss function for the task at hand. Completed training yielded pairs of (LSTM, loss value).
- They generated a new LSTM by taking a weighted average (the smaller the loss, the heavier the weighting) of each weight across all LSTMs across all tasks. The authors took the new LSTM and repeated the process: They copied and randomly modified the LSTM, generated new optimizer networks, used them to train new target networks, updated the LSTM, and so on.
Results: The authors evaluated VeLO using a dataset scaled to require no more than one hour to train on a single GPU on any of 83 tasks. They applied the method to a new set of randomly generated neural network architectures. On all tasks, VeLO trained networks faster than Adam tuned to find the best learning rate — four times faster on half of the tasks. It also reached a lower loss than Adam on five out of six MLCommons tasks, which included image classification, speech recognition, text translation, and graph classification tasks.
Yes, but: The authors’ approach underperformed exactly where optimizers are costliest to hand-tune, such as with models larger than 500 million parameters and those that required more than 200,000 training steps. The authors hypothesized that VeLO fails to generalize to large models and long training runs because they didn’t train it on networks that large or over that many steps.
Why it matters: VeLO accelerates model development in two ways: It eliminates the need to test hyperparameter values and speeds up the optimization itself. Compared to other optimizers, it took advantage of a wider variety of statistics about the target network’s training from moment to moment. That enabled it to compute updates that moved models closer to a good solution to the task at hand.
We’re thinking: VeLO appears to have overfit to the size of the tasks the authors chose. Comparatively simple algorithms like Adam appear to be more robust to a wider variety of networks. We look forward to VeLO-like algorithms that perform well on architectures that are larger and require more training steps.
We’re not thinking: Now neural networks are taking optimizers’ jobs!
Data Points
OECD finds that AI imperils a quarter of jobs
A survey conducted by the international organization on AI’s impact on employment revealed that three out of five workers expressed concerns about the potential loss of their job to AI within the next decade, among other findings. (Reuters)
Hollywood actors strike, partly for protection against AI
SAG-AFTRA, a trade union that represents 160,000 film and television actors, joined the Writers Guild of America (WGA) to demand pay increases and protections against AI doing their work. (Reuters)
VC automates initial meetings with startups
Connectic Ventures developed Wendal, an AI-powered tool that helps the investment firm to evaluate startup founders for potential funding. It collects information and administers a test for entrepreneurial traits, enabling the company to promise a decision within three days. . (TechCrunch)
Research: AI detectors often misclassify writing by non-native English speakers
The study revealed that GPT detectors incorrectly classify TOEFL (Test of English as a Foreign Language) essays by non-native English speakers 61.3 percent of the time. (Gizmodo)
Research: Smartwatch data reveals Parkinson’s disease
Researchers trained a machine learning model to classify Parkinson’s risk based on accelerometer data collected from wrist-worn devices. The model identified Parkinson's risk up to seven years before clinical symptoms appeared with accuracy similar to models based on combined accelerometer data, genetic screens, lifestyle descriptions, and blood chemistry. (IEEE Spectrum)
China sets new regulations on generative AI
China's Interim Measures for the Management of Generative Artificial Intelligence Services, set to take effect on August 15, requires companies that produce AI-generated content for public consumption take steps to ensure its accuracy and reliability. (The Washington Post)
Google expands Bard’s global reach
The chatbot is now available in 43 new languages and expanded its reach to more regions, including countries in the European Union, which had requested privacy features that delayed the chatbot’s release there. (Gizmodo)
Research: Google tests medical large language model
Med-PaLM, which was trained to pass medical licensing exams, is being tested by Mayo Clinic. The model generates responses to medical questions, summarizes documents, and organizes health data. Med-PaLM's answers to medical questions from consumers compared favorably with clinicians' answers, proving the effectiveness of instruction prompt tuning. (The Wall Street Journal)
Surging AI demand pushes data center operators to hike leases
Data center operators are raising prices apparently to cover increased demand for AI, which requires copious processing power and advanced chips. Data centers are struggling to expand capacity as customers consume resources faster than operators can increase them. (The Wall Street Journal)
Elon Musk officially launched AI company xAI
The billionaire announced that the goal of the new company, which initially came to light in March, is to “understand the true nature of the universe.” Team members include former employees from DeepMind, OpenAI, and Google Research. Further information about the company is yet to be released. (The Wall Street Journal)
Reporters compared Ernie and ChatGPT
Ernie, built by Baidu, tiptoed around politically sensitive topics but its connection to Baidu’s search engine enabled it to answer some questions with greater factual accuracy. OpeAI’s ChatGPT responded accurately to a wider variety of questions and more complex prompts but suffered from its September 2022 training cutoff. (The New York Times)
Federal Trade Commission investigating OpenAI over data collection
The FTC requested detailed information on OpenAI's data vetting process during model training, its methods for preventing ChatGPT from generating false claims, and how it protects data. (The Verge)