Dear friends,
AI risks are in the air — from speculation that AI, decades or centuries from now, could bring about human extinction to ongoing problems like bias and fairness. While it’s critically important not to let hypothetical scenarios distract us from addressing realistic issues, I’d like to talk about a long-term risk that I think is realistic and has received little attention: If AI becomes cheaper and better than many people at doing most of the work they can do, swaths of humanity will no longer contribute economic value. I worry that this could lead to a dimming of human rights.
We’ve already seen that countries where many people contribute little economic value have some of the worst records of upholding fundamental human rights like free expression, education, privacy, and freedom from mistreatment by authorities. The resource curse is the observation that countries with ample natural resources, such as fossil fuels, can become less democratic than otherwise similar countries that have fewer natural resources. According to the World Bank,“developing countries face substantially higher risks of violent conflict and poor governance if [they are] highly dependent on primary commodities.”
A ruler (perhaps dictator) of an oil-rich country, for instance, can hire foreign contractors to extract the oil, sell it, and use the funds to hire security forces to stay in power. Consequently, most of the local population wouldn’t generate much economic value, and the ruler would have little incentive to make sure the population thrived through education, safety, and civil rights.
What would happen if, a few decades from now, AI systems reach a level of intelligence that disempowers large swaths of people from contributing much economic value? I worry that, if many people become unimportant to the economy, and if relatively few people have access to AI systems that could generate economic value, the incentive to take care of people — particularly in less democratic countries — will wane.
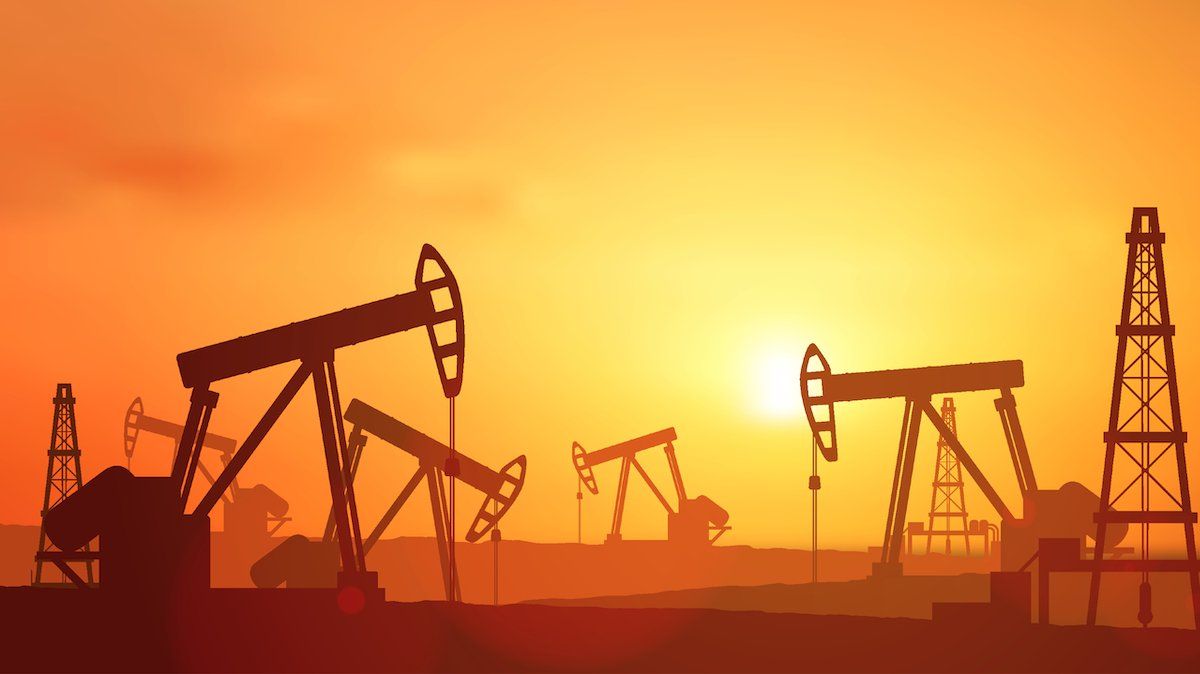
Marc Andreessen recently pointed out that Tesla, having created a good car, has an incentive to sell it to as many people as possible. So why wouldn’t AI builders similarly make AI available to as many people as possible? Wouldn’t this keep AI power from becoming concentrated within a small group? I have a different point of view. Tesla sells cars only to people who generate enough economic value, and thus earn enough wages, to afford one. It doesn’t sell many cars to people who have no earning power.
Researchers have analyzed the impact of large language models on labor. While, so far, some people whose jobs were taken by ChatGPT have managed to find other jobs, the technology is advancing quickly. If we can’t upskill people and create jobs fast enough, we could be in for a difficult time. Indeed, since the great decoupling of labor productivity and median incomes in recent decades, low-wage workers have seen their earnings stagnate, and the middle class in the U.S. has dwindled.
Many people derive tremendous pride and sense of purpose from their work. If AI systems advance to the point where most people no longer can create enough value to justify a minimum wage (around $15 per hour in many places in the U.S.), many people will need to find a new sense of purpose. Worse, in some countries, the ruling class will decide that, because the population is no longer important for production, people are no longer important.
What can we do about this? I’m not sure, but I think our best bet is to work quickly to democratize access to AI by (i) reducing the cost of tools and (ii) training as many people as possible to understand them. This will increase the odds that people have the skills they need to keep creating value. It will also ensure that citizens understand AI well enough to steer their societies toward a future that’s good for everyone.
Keep working to make the world better for everyone!
Andrew
News
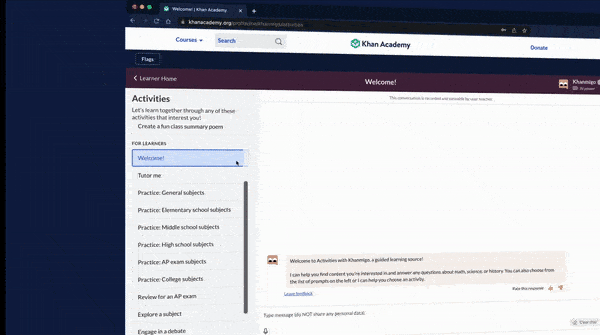
Taught by a Bot
While some schools resist their students’ use of chatbots, others are inviting them into the classroom.
What’s new: Some primary and secondary schools in the United States are testing an automated tutor built by online educator Khan Academy, The New York Times reported. Users of the Khanmigo chatbot include public schools in New Jersey and private schools like Silicon Valley’s Khan Lab School (established by Khan Academy founder Sal Khan).
How it works: Khanmigo is based on GPT-4. Instead of providing answers outright, it responds to inquiries with questions meant to encourage critical thinking.
- Khanmigo is integrated with Khan Academy’s previous tutoring software, which poses questions for students to answer. A student who has trouble answering can open the chatbot and ask for assistance.
- In addition, the chatbot offers vocabulary practice, assistance in writing stories, debates (example: “Are video games good or bad for kids?”), and the ability to chat with simulated historical figures like Harriet Tubman or fictional characters like Don Quixote. It also helps to navigate university admissions and financial aid.
- Teachers can view student conversations with the chatbot, and the system will notify them if it notices a conversation that may have taken a dangerous turn. They can also use it to create lesson plans, write classroom exercises, and refresh their own knowledge.
- Currently, Khanmigo is available only to a few schools among more than 500 Khan Academy customers. The organization plans to make it available via a waitlist, giving priority to financial donors and current customers.
Behind the news: Chegg, which maintains a cadre of tutors to help students with homework, recently lost 48 percent of its market value after the company’s CEO said ChatGPT had dampened subscriber growth. Chegg plans to launch a GPT-4-based chatbot called CheggMate next year.
Why it matters: Some educators oppose ChatGPT over concerns that it enables cheating, fuels plagiarism, and spreads misinformation. Meanwhile, many students prefer it to human tutors because it’s available around the clock, according to one survey. By offering a chatbot that leads students to an answer rather than providing it outright, Khan Academy’s approach may assuage educators’ concerns while satisfying student preferences.
We’re thinking: While large language models can be used to avoid learning, there’s much more to be gained by harnessing them to accelerate and enrich it. We hope Khan Academy’s approach catches on.
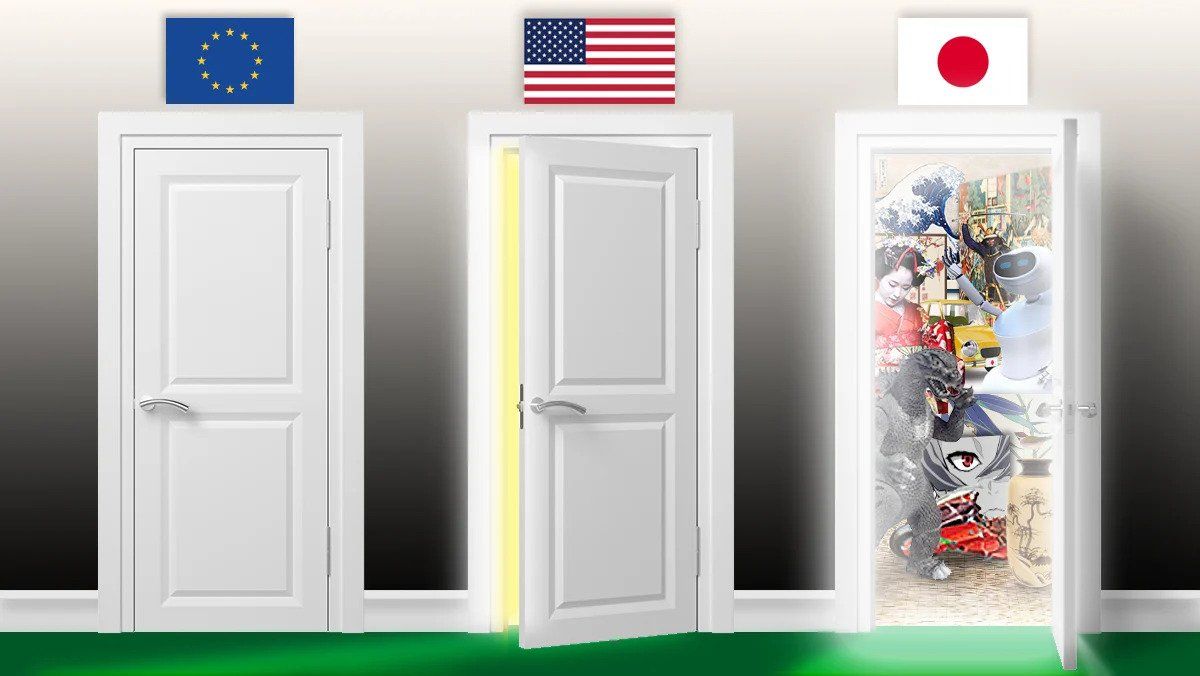
Training Data Free-For-All
Amid rising questions about the fairness and legality of using publicly available information to train AI models, Japan affirmed that machine learning engineers can use any data they find.
What’s new: A Japanese official clarified that the country’s law lets AI developers train models on works that are protected by copyright.
How it works: In testimony before Japan’s House of Representatives, cabinet minister Keiko Nagaoka explained that the law allows machine learning developers to use copyrighted works whether or not the trained model would be used commercially and regardless of its intended purpose.
- Nagaoka said the law technically prohibits developers from using copyrighted works that they had obtained illegally, but conceded that the difficulty of discerning the provenance of large quantities of data makes this limitation difficult to enforce.
- Copyright holders have no legal avenue to block use of their works for “data analysis” including AI training. However, such use is prohibited if it would cause them unreasonable harm.
- In 2018, Japan modified its Copyright Act to allow free of copyrighted works for training machine learning models as long as the purpose “is not to enjoy the thoughts or feelings expressed in the work.”
Yes, but: Politicians in minority parties have pressed the ruling party to tighten the law. Visual artists and musicians have also pushed for a revision, saying that allowing AI to train on their works without permission threatens their creative livelihoods.
Behind the news: Japan is unusual insofar as it explicitly permits AI developers to use copyrighted materials for commercial purposes.
- In the European Union, developers can use copyrighted works freely for research. The EU’s upcoming AI Act, which is expected to become law later this year, requires generative AI developers to disclose their use of copyrighted works in training.
- The United Kingdom allows developers to train machine learning models on copyrighted works for research purposes only.
- In the United States, copyright law includes a “fair use” principle that generally permits use of copyrighted works without permission as long as the use constitutes a significant change in the work and does not threaten the copyright holder’s interests. Whether or not fair use includes training machine learning models has yet to be determined and may be settled by cases currently in progress.
Why it matters: Last month, member states of the Group of Seven (G7), an informal bloc of industrialized democratic governments that includes Japan, announced a plan to craft mutually compatible regulations and standards for generative AI. Japan’s stance is at odds with that of its fellows, but that could change as the members develop a shared vision.
We’re thinking: In the era of generative AI, the question of what’s fair, and thus what makes a sensible legal standard, is tricky, leading different regions in divergent directions. We applaud the G7 for moving toward globally compatible laws, which will make it easier for developers worldwide to do work that benefits people everywhere.
A MESSAGE FROM DEEPLEARNING.AI
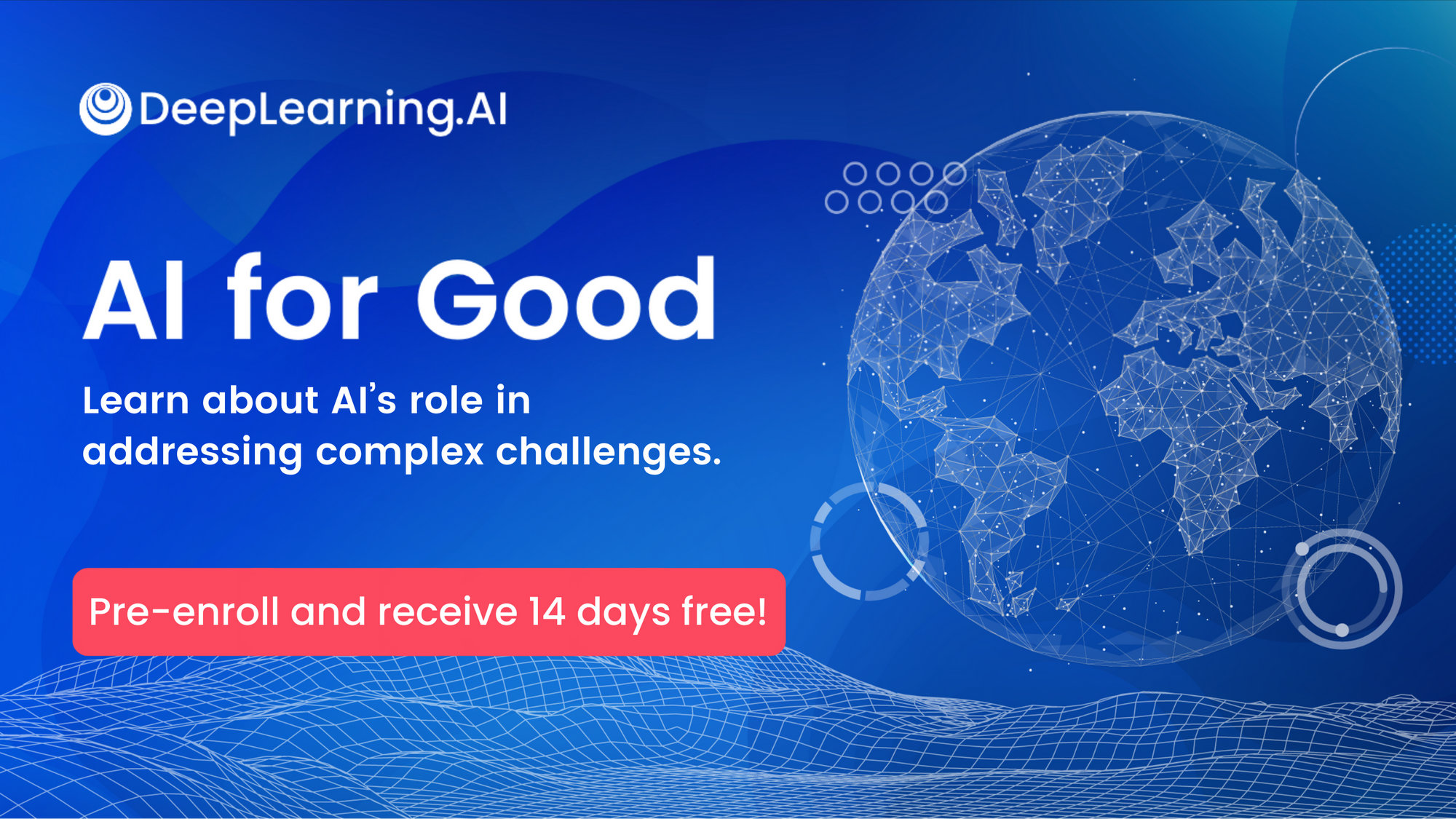
Gain hands-on experience with a framework for addressing complex public-health and environmental challenges in our upcoming specialization, AI for Good. Pre-enroll and get 14 days of your subscription for free!
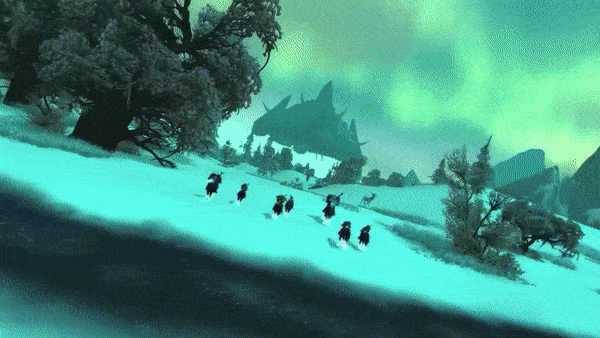
Game Makers Embrace Generative AI
The next generation of video games could be filled with AI-generated text, speech, characters, and background art.
What’s new: Nvidia announced a system that enables players to converse directly with in-game characters. Meanwhile, game developers are using generative AI to produce media assets, The New York Times reported.
How it works: Tech companies are providing software that generates game assets either in production or on the fly. Some large game studios are developing their own tools.
- At Computex 2023 in Taipei, Nvidia showed off a suite of tools called Avatar Cloud Engine (ACE). In the demo, a human player speaks to a game character that replies in real time with information that drives further gameplay. ACE interpreted the player, generated the character's words and voice, and drove the animation. Nvidia developed the software in collaboration with Convai.
- The startup Scenario offers a text-to-image generator with a specialized user interface for fine-tuning on a developer’s assets. Didimo offers a text-to-3D generator that outputs editable, animation-ready character models in developer-friendly formats.
- Blizzard Entertainment, producer of the popular Diablo, Overwatch, and World of Warcraft franchises, trained an image generator on assets from its own games. Developers use it to generate concept art for characters and environments.
- Ubisoft, whose titles include Assassin’s Creed and Far Cry, built a dialogue generator. Writers use it to create dialogue for in-game characters. Given a prompt like, “I used to be an adventurer like you,” the model generates variations such as “I remember when I was young and strong,” and “I was once the greatest explorer in the world.”
Behind the news: Gamers, too, are using generative AI to modify their favorite games. For instance, modders have used voice cloning to vocalize lines for the main character of “The Elder Scrolls V: Skyrim,” who otherwise is silent.
Why it matters: Generative AI tools can streamline video game production, which is bound to appeal to developers who aim to cut both costs and timelines. More exciting, it can supercharge their ability to explore art styles, characters, dialog, and other creative features that may not be practical in a conventional production pipeline.
We’re thinking: Given the high cost of media production, game development is ripe for disruption by generative AI. While we worry that some artists and writers may lose work, we expect that automating production will also create jobs. Big players are already using the technology to build more elaborate virtual worlds, and many smaller studios will benefit from lower production costs.
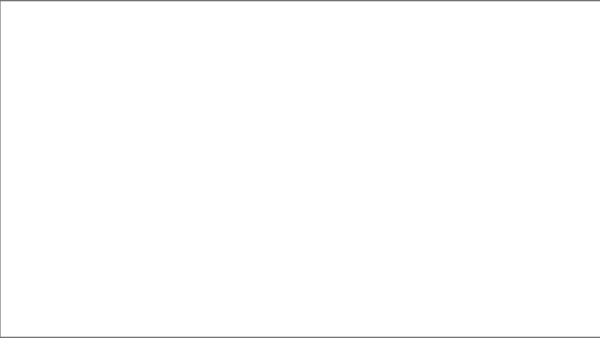
Like Diffusion but Faster
The ability to generate realistic images without waiting would unlock applications from engineering to entertainment and beyond. New work takes a step in that direction.
What’s new: Dominic Rampas and colleagues at Technische Hochschule Ingolstadt and Wand Technologies released Paella, a system that uses a process similar to diffusion to produce Stable Diffusion-quality images much more quickly.
Key insight: An image generator’s speed depends on the number of steps it must take to produce an image: The fewer the steps, the speedier the generator. A diffusion model learns to remove varying amounts of noise from each training example; at inference, given pure noise, it produces an image by subtracting noise iteratively over a few hundred steps. A latent diffusion model reduces the number of steps to around a hundred by removing noise from a vector that represents the image rather than the image itself. Instead of a vector, using a selection of tokens from a predefined list makes it possible to do the same job in still fewer steps.
How it works: Like a diffusion model, Paella learned to remove varying amounts of noise from tokens that represented an image and then produced a new image from noisy tokens. It was trained on 600 million image-text pairs from LAION-Aesthetics.
- Given an image of 256x256 pixels, a pretrained encoder-decoder based on a convolutional neural network represented the image using 256 tokens selected from 8,192 tokens it had learned during pretraining.
- The authors replaced a random fraction of the tokens with tokens chosen from the list at random. This is akin to adding noise to an example in training a diffusion model.
- Given the image’s text description, CLIP, which maps corresponding text and images to the same embedding, generated an embedding for it. (The authors used CLIP’s text-image embedding capability only for ablation experiments.)
- Given the text embedding and the tokens with random replacements, a U-Net (a convolutional neural network) learned to generate all the original tokens.
- They repeated the foregoing steps 12 times, each time replacing a smaller fraction of the generated tokens. This iterative procedure trained the U-Net, guided by the remaining generated tokens, to remove a smaller amount of the remaining noise at each step.
- At inference, given a text prompt, CLIP generated an embedding. Given a random selection of 256 tokens, the U-Net regenerated all the tokens over 12 steps. Given the tokens, the decoder generated an image.
Results: The authors evaluated Paella (573 million parameters) according to Fréchet inception distance (FID), which measures the difference between the distributions of original and generated images (lower is better). Paella achieved 26.7 FID on MS-COCO. Stable Diffusion v1.4 (860 million parameters) trained on 2.3 billion images achieved 25.40 FID — somewhat better, but significantly slower. Running on an Nvidia A100 GPU, Paella took 0.5 seconds to produce a 256x256-pixel image in eight steps, while Stable Diffusion took 3.2 seconds. (The authors reported FID for 12 steps but speed for eight steps.)
Why it matters: Efforts to accelerate diffusion have focused on distilling models such as Stable Diffusion. Instead, the authors rethought the architecture to reduce the number of diffusion steps.
We’re thinking: The authors trained Paella on 64 Nvidia A100s for two weeks using computation supplied Stability AI, the firm behind Stable Diffusion. It’s great to see such partnerships between academia and industry that give academic researchers access to computation.
Data Points
FBI warns about deepfakes
The agency has seen a surge in reports of extortion and harassment cases involving AI-generated nudes and is urging the public to post online content with caution. (The Verge)
Replika’s parent company launched erotic chatbot
Called Blush, the bot aims to help users develop intimacy and flirting skills in a dating app-like format. (TechCrunch)
Putin deepfake broadcast in Russia
A video in which Vladimir Putin appeared to declare martial law was broadcast by Russian radio and television stations in regions that border Ukraine. Russian authorities said the video was fake and blamed hackers for the broadcast. (Vice)
Stack Overflow moderators strike over AI content
The forum’s volunteer moderators left the site unattended in protest against the a policy that allows AI-generated content to be posted without moderation. The moderators contend that the free flow of generated text could lead to misinformation and plagiarism. (Vice)
Apple keynote ignored generative AI
At the Apple’s Worldwide Developers Conference, the company unveiled its Vision Pro headset and showcased AI-powered features. However, the keynote address omitted any mention of generative AI. (Wired)
UK to host AI summit
The gathering is expected to bring together key governments and companies yet to be announced. It will focus on AI safety. (Politico)
EU urged tech giants to label AI-generated content
Amid ongoing efforts to combat Russian disinformation, the European Union requested that Google and Facebook apply labels to generated text and images. The EU also issued a warning to Twitter to comply with digital content regulations. (The Guardian)
Research: Human-made videos outperformed AI-generated clips
An academic survey found that news consumers prefer short-form videos that are either produced or edited by journalists, as opposed to AI-generated videos. (Press Gazzette)
WordPress introduced a generative AI plug-in
The plug-in, called Jetpack, corrects grammar, edits the tone and style of text, generates summaries and translations, and more. It’s available free for a limited time. (The Verge)
Research: Researchers developed a tool to detect AI-generated scientific articles
The tool achieved 100 percent accuracy in identifying generated papers papers and 92 percent accuracy when detecting individual paragraphs. (ABC Australia)
AI image of “smiling” protestors sparked controversy
A deepfaked photograph circulated on social media showing Indian protestors apparently smiling in a police vehicle after they were detainedThe fake image prompted accusations that the protestors were pleased by the publicity their detention achieved. In the original photo, they did not smile. (Alt News)