Dear friends,
Last week, safe.org asserted that “Mitigating the risk of extinction from AI should be a global priority alongside other societal-scale risks such as pandemics and nuclear war.” This statement was signed by AI scientists who I really respect including Yoshua Bengio and Geoffrey Hinton. It received widespread media coverage.
I have to admit that I struggle to see how AI could pose any meaningful risk for our extinction. AI has risks like bias, fairness, inaccurate outputs, job displacement, and concentration of power. But I see AI’s net impact as a massive contribution to society. It’s saving lives by improving healthcare and making cars safer, improving education, making healthy food and numerous other goods and services more affordable, and democratizing access to information. I don’t understand how it can lead to human extinction.
A number of thoughtful commentators have also pushed back on the extinction narrative. For example:
- Chris Manning points out that the AI community has a large, quiet majority that’s focused on building useful software and does not share the views of the loud AI Safety crowd that talks about existential risks. It believes the risks can be mitigated.
- Emily Bender notes that AI doomsaying is a huge distraction from the technology’s real harms, which she lists as “discrimination, surveillance, pollution of the information ecosystem, data theft, labor exploitation.”
- Along this vein, Matteo Wong in The Atlantic argues that “AI doomerism is a decoy.” It appears to me that time spent by regulators stopping AI from autonomously launching nuclear weapons — which no nuclear power has publicly considered — is time that they’re not spending passing regulations on data privacy, AI transparency or anti-trust that would be less convenient for tech companies and might negatively affect their bottom line.
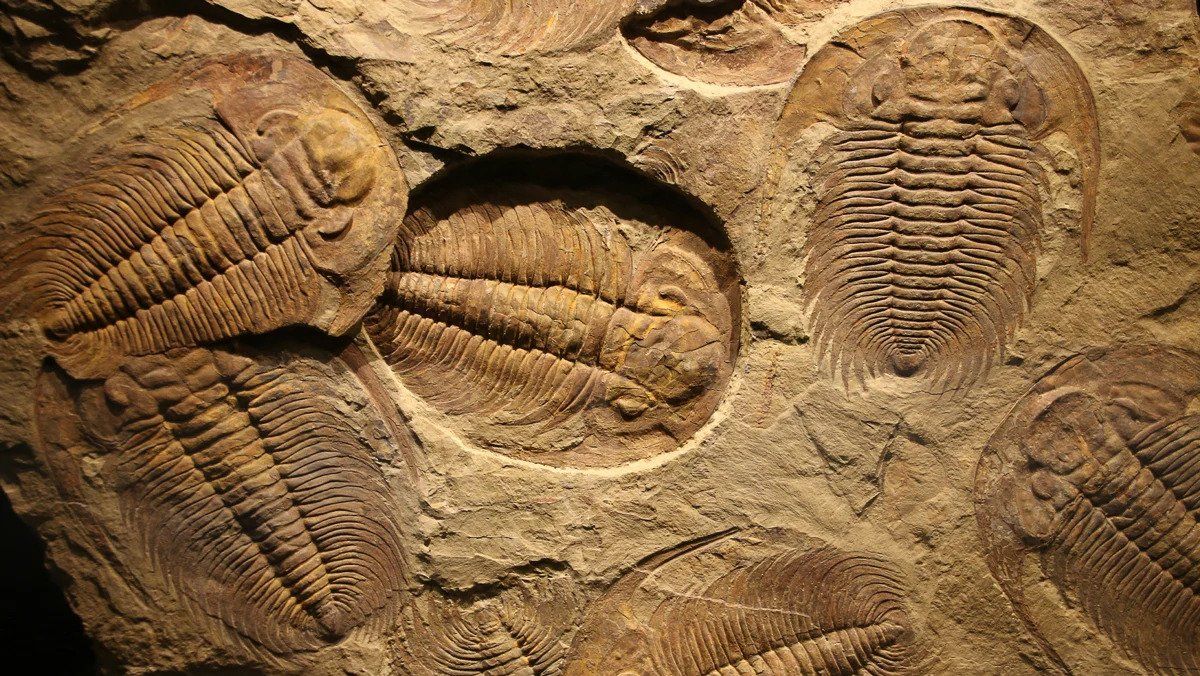
- Marc Andreessen wrote an essay on the benefits of AI. While my perspective differs from his on some points (for example, I’m more worried than he is about the negative impact of job displacement), he makes a sound argument that each time a new technology has been introduced, a predictable moral panic has taken hold. Examples are documented by the fascinating website pessimistsarchive.org (worth a look!), which describes fear of non-fiction novels corrupting youth, elevators causing brain fever, cars (“the devil wagon”) on a mission to destroy the world, and recorded sound harming babies. With the rise of deep learning about 10 years ago, Elon Musk, Bill Gates and Stephen Hawking warned of the existential risk stemming from AI. The current wave of fears about AI feels similar to me, but it’s more intense and has buy-in from prominent scientists.
I’m glad to see others presenting a sensible alternative to the narrative of AI as an extinction risk. Having said that, though, I feel an ethical responsibility to keep an open mind and make sure I really understand the risk — especially given the high regard I have for some who think AI does pose this risk.
To learn more, I’m speaking with a few people who I think might have a thoughtful perspective on how AI creates a risk of human extinction, and I will report back with my findings. In the meantime, I would love to hear your thoughts as well. Please reply to my posts on Twitter or LinkedIn if there’s someone you think I should speak with or if you’d like to share your perspective. Through this, I hope we can have a real conversation about whether AI really poses an extinction risk.
I look forward to continuing the discussion with you,
Andrew
News
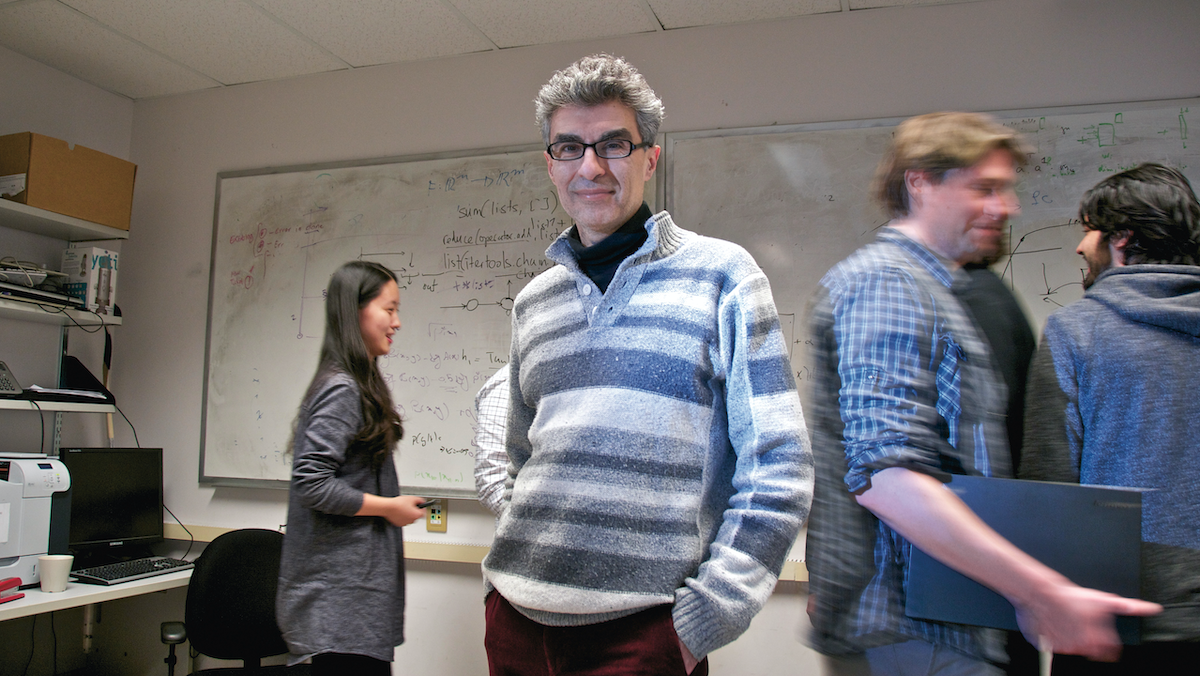
Bengio, Too, Anxious About AI Risks
Another prominent AI pioneer expressed regret over his life’s work amid rising concerns over the technology’s risks.
What’s new: Yoshua Bengio, a professor at the Université de Montréal who laid parts of the foundation for deep learning, followed fellow trailblazer Geoffrey Hinton in airing his anxiety publicly. He told BBC that AI’s potential for misuse left him feeling “lost” and questioning the value of his life’s work.
New worries: Bengio said he was afraid that “bad actors” could use AI to cause harm, for instance by developing chemical weapons. In particular, he cited militaries, terrorists, or individuals with personal vendettas.
- Bengio called for governments to register AI developers and govern them similarly to pharmaceutical companies and aircraft manufacturers. He also proposed that computer scientists should be required to undergo ethical training and certification.
- In a recent blog post, he warned of the possibility of rogue AIs that pursue their own goals. The post describes how such machines might be built and how they might cause catastrophic harm.
- Last month, he signed a statement by the Center for AI Safety that urged the world to focus on mitigating the risk that AI could bring about human extinction. In March, he signed the Future of Life Institute’s call for a six-month pause in training models more advanced than OpenAI’s GPT-4.
Behind the news: Bengio is one of the most cited computer scientists in the world. He, Hinton, and Yann LeCun shared the prestigious Turing Award in 2018 for their foundational work in deep learning. His accomplishments include helping to introduce an early attention mechanism for natural language processing and develop the generative adversarial network architecture. In a commentary he wrote for The Batch, he looked forward to neural nets that can reason.
Why it matters: The recent pace of progress in AI has startled even researchers who have spent decades improving the technology, and its potential for harm has taken many by surprise. While there is little doubt that AI poses hazards, debate runs hot around which are most pressing and how to address them. (For instance, Yann LeCun, the third winner of the shared Turing Award, has downplayed some of Bengio’s concerns.) Recognizing the most serious problems is the first step toward devising effective solutions.
We’re thinking: As AI builders, we have an ethical responsibility to minimize the harms our work might bring, even as we work to maximize the benefits. We wish Yoshua Bengio great fulfillment in the next phase of his stellar career.
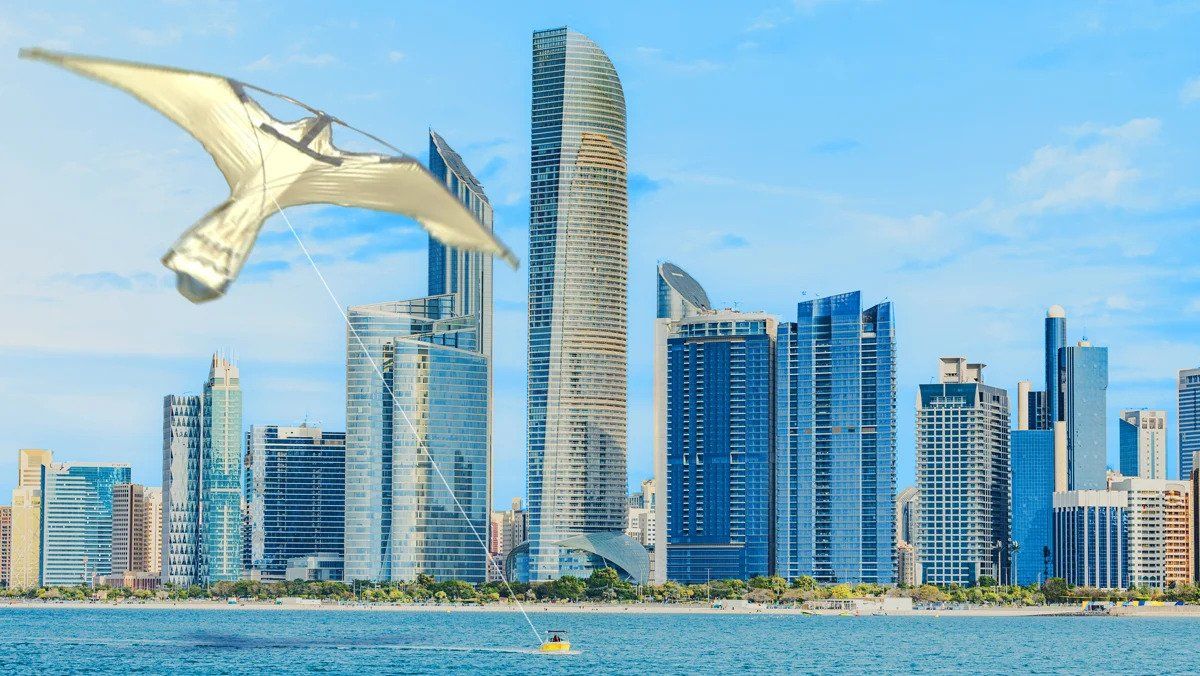
Falcon Ascends
A team in the United Arab Emirates, a seven-state federation on the Arabian Peninsula, built the latest top-performing open source large language model.
What’s new: The UAE’s Falcon edged out Meta’s LLaMA in the Hugging Face Open LLM Leaderboard to take the top spot. It’s available via the Apache 2.0 license, which is free for commercial applications. You can try it here.
How it works: Developed by Abu Dhabi’s Technology Innovation Institute (TII), Falcon is a pretrained model based on transformers. A paper is forthcoming.
- The 40-billion parameter model was pretrained to predict the next token on 1 trillion tokens of text. 820 billion tokens came from RefinedWeb, a curated subset of Common Crawl. The remainder came from books, code, academic papers, technical documents, and conversations on sites including Reddit and StackOverflow.
- The architecture is similar to OpenAI’s GPT-3 with a few differences. For instance, it uses the FlashAttention algorithm with multiquery attention, both of which cut memory requirements at inference.
- Four versions are available: the general-purpose Falcon-40B the chat-ready Falcon-40B-Instruct, the more compact Falcon-7B, and Falcon-7B-Instruct.
- Initially, TII required users whose commercial applications of Falcon earn more than $1 million to pay a 10 percent “authorization fee.” However, the developer quickly reverted to a more permissive license.
Behind the news: Open source licenses, particularly those that are free for commercial use, are enabling independent teams to build systems that are competitive with those produced by big tech companies. A recently leaked Google memo went so far as to call open source development a threat to the company’s business.
Why it matters: Amid a surge in open source language models, Falcon offers higher performance (on the four benchmarks tracked by Hugging Face) and lower training cost relative to its chief rival, LLaMA. The fact that it was built by a team in Abu Dhabi highlights the fact that AI talent is everywhere and elite skills are spreading to all parts of the globe.
We’re thinking: AI development is a global enterprise. It gives us hope that people around the world can come together to meet other common challenges.
A MESSAGE FROM DEEPLEARNING.AI
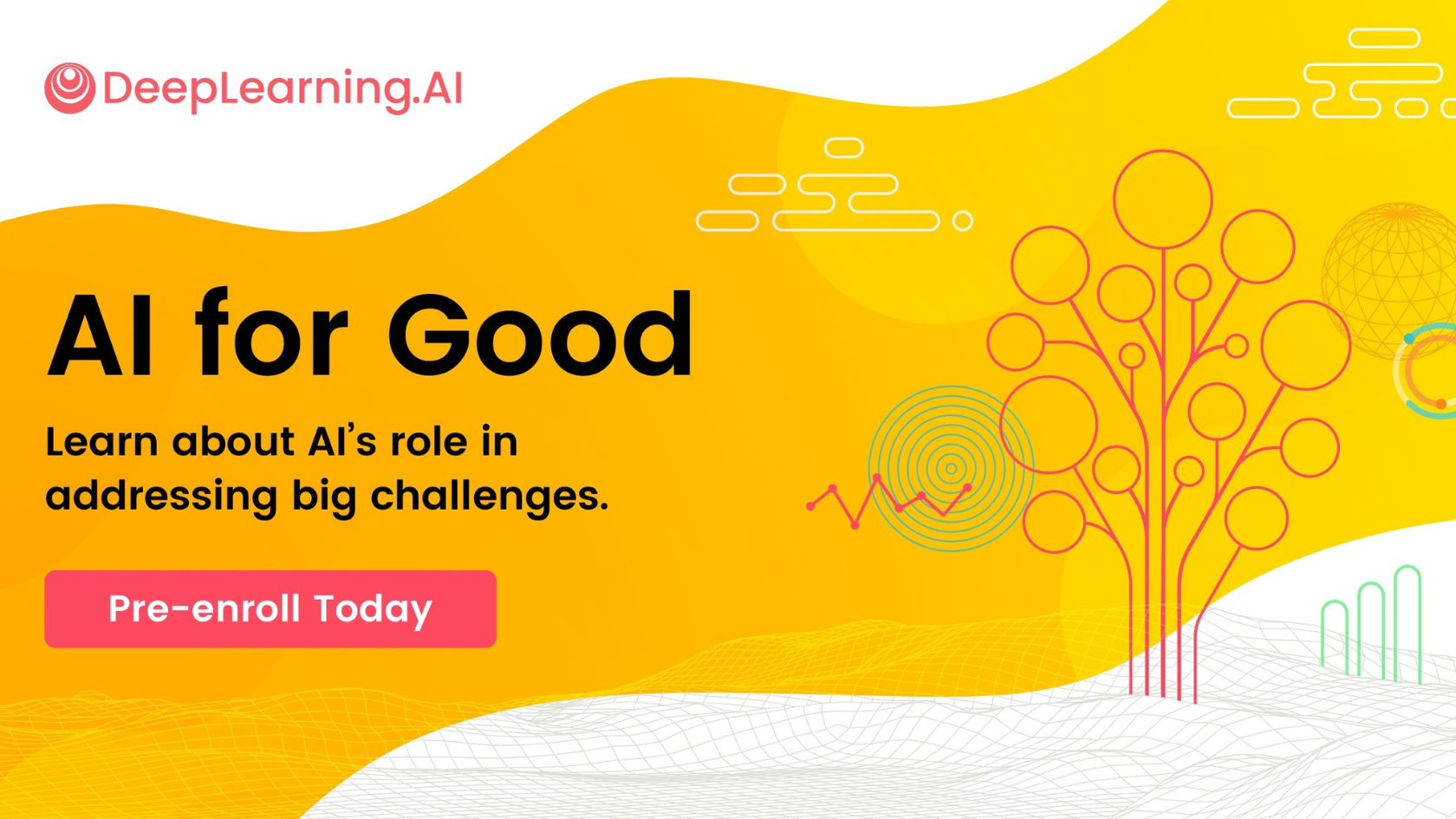
Are you ready to use AI in projects that can have a positive impact on public health, climate change, and disaster management? Pre-enroll now and get 14 days of your subscription for free!
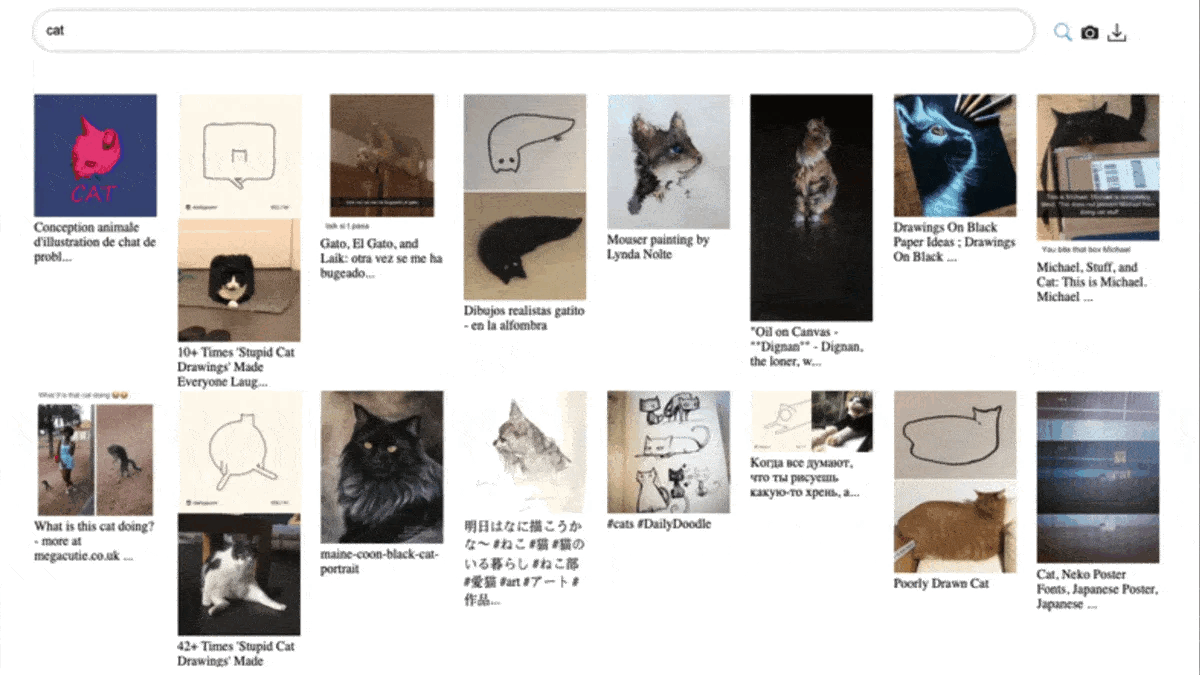
LAION Roars
The largest dataset for training text-to-image generators was assembled by volunteers for roughly $10,000. Now it’s implicated in fights over whether copyrighted works can be used for training.
What’s new: Christoph Schuhmann, a German high school teacher who helped found the Large-scale Artificial Intelligence Open Network (LAION), told Bloomberg how a cadre of outsiders came together to ensure that large tech companies aren’t the only ones with access to large quantities of training data. The nonprofit group’s datasets — notably LAION-5B (5 billion text-image pairs) — have been used to train Stability AI’s Stable Diffusion, Google’s Imagen, and other text-to-image models.
Volunteer work: Schuhmann and two co-founders met on a Discord server for AI enthusiasts. Catalyzed by the launch of OpenAI’s DALL•E in January 2021, they decided to build their own image dataset. They established a separate Discord server in March 2021, which continues to act as LAION’s nerve center.
- The group used a Python script to trawl through raw HTML in the Common Crawl dataset to identify images paired with alt text. They used OpenAI’s CLIP to calculate a similarity score between a linked image and its corresponding text and selected pairs with sufficiently high scores.
- They probed image hosting sites like Pinterest and DeviantArt, ecommerce services like Shopify, cloud services like Amazon Web Services, thumbnails from YouTube, photos from U.S. government websites, and images from news sites. The team did not filter out objectionable content.
- The team covered its server fees through a combination of crowdfunding, a 2021 donation from Hugging Face for an unspecified amount, and a donation from Stability AI founder Emad Mostaque for between $9,000 and $10,000. Mostaque, who had founded Stability AI in 2020, used a 2 billion-image subset of LAION-5B to train Stable Diffusion, released in August 2022.
- Schuhmann, who continues to work for LAION pro bono, has refused job offers from several tech firms.
Behind the news: Data scraped from the web is at the center of several disputes.
- Artists are suing Stability AI and Midjourney for their use of copyrighted works in developing AI models. Developers are suing Microsoft, GitHub, and OpenAI over their use of open source code for the same purpose. Both cases are in progress.
- LAION may be insulated from claims of copyright violation because it doesn’t host its datasets directly. Instead it supplies web links to images rather than the images themselves. When a photographer who contributes to stock image libraries filed a cease-and-desist request that LAION delete his images from its datasets, LAION responded that it has nothing to delete. Its lawyers sent the photographer an invoice for €979 for filing an unjustified copyright claim.
- A major recording company has pressured streaming services to block AI developers from downloading music.
- Such conflicts are set to proliferate. The latest draft of the European Union’s AI Act, which has been approved by the bloc’s assembly and is pending review by a higher authority, mandates that generative AI developers disclose copyrighted materials used to train their models — a tall order when those materials are scraped from the web en masse.
Why it matters: Copyright holders are questioning the ethics of using their materials to build AI models. LAION plays a major role in the controversy. On one hand, it’s a nonprofit effort run by volunteers on a shoestring budget. On the other, the datasets it curates are driving tremendous business value. Stability AI, for instance, seeks a $4 billion valuation.
We’re thinking: The AI community is entering an era in which we are called upon to be more transparent in our collection and use of data. We shouldn’t take resources like LAION for granted, because we may not always have permission to use them.
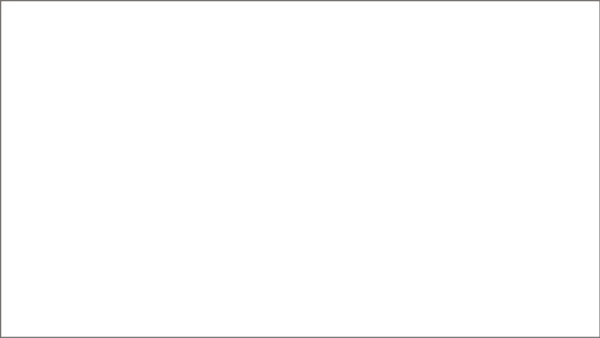
Optimizing Matrix Multiplication
Matrix multiplication is executed so often in deep learning, video games, and scientific computing that even a slight acceleration can save substantial amounts of processing time. New work finds ways to speed up this crucial operation.
What’s new: Alhussein Fawzi and colleagues at DeepMind developed AlphaTensor. This reinforcement learning agent discovers algorithms that multiply matrices faster than those previously developed by humans.
Composition and decomposition: Computers need more time to multiply than to add or subtract. Developers often take advantage of algebraic properties — for instance, (a^2 - b^2) = (a+b)(a-b) — to manually find matrix multiplication algorithms that require fewer multiplications. To minimize the number of multiplications systematically, we can take advantage of the fact that a tensor (a high-dimensional matrix) can represent a matrix multiplication algorithm. It’s easy to compose a tensor from three matrices. However, to decompose a tensor (the reverse operation) is not straightforward; the procedure could result in any of thousands of potential sets of matrices. Any valid decomposition of the tensor into three matrices represents a valid algorithm for matrix multiplication. The number of columns equals the number of multiplications required.
Key insight: Just as DeepMind’s AlphaZero learned via reinforcement learning to play Go by simulating future game-board states and, based on those states, predicting the likelihood that it would win, a reinforcement learning model can learn to win a game of decomposing tensors by predicting the columns of three matrices.
How it works: Given a tensor that represents a matrix multiplication algorithm, AlphaTensor played a game in which it decomposed the tensor into three matrices with as few columns — and thus as few multiplications — as possible. (The values in the predicted columns were limited to {-2,-1,0,1,2} to avoid precision issues that could have occurred with floating-point values.) At each turn, it predicted the entries in one column of each of the three matrices. The game updated the tensor’s state by subtracting the outer product of the predicted columns. It ended when all entries in the tensor equalled 0. AlphaTensor received a negative reward after predicting each set of columns, which encouraged it to decompose the tensor into matrices that had few columns. It received a positive reward for predicting all columns of the three matrices.
- The authors constructed the training dataset of tensor decompositions by randomly generating three matrices and composing them into a tensor.
- Given a tensor’s state (starting with the tensor to be decomposed), AlphaTensor embedded the tensor using a series of axial attention layers.
- Given the tensor embedding, AlphaTensor predicted columns using two components: a transformer that predicted likely next columns and a vanilla neural network that predicted the future total reward for those columns.
- Of the predicted columns, AlphaTensor chose a set that wasn’t often previously predicted and had a high probability and high predicted reward.
Results: AlphaTensor rediscovered known matrix multiplication algorithms for matrices as large as five rows and columns (5x5). Notably, to multiply two 4x4 matrices that contain binary numbers, AlphaTensor discovered an algorithm that requires 47 multiplications, compared to Strassen’s algorithm, which requires 49 and had not been improved upon since its creation in 1969. To multiply 4x5 and 5x5 matrices that contain real numbers, AlphaTensor found an algorithm that requires 76 multiplications; the previous best takes 80. After training AlphaTensor with an additional reward that reduced hardware-specific compute time, the authors found algorithms for an Nvidia V100 GPU that are, on median, 8.5 percent faster than the usual implementation. Optimized for TPUs, AlphaTensor sped up matrix multiplication by 10.3 percent.
Why it matters: Neural networks learn from data how to perform a particular task reasonably well (for instance, they may be correct 95 percent of the time). But is reasonably well sufficient for a field such as mathematics, in which results are provably true or false? This paper stands alongside achievements such as a neural theorem finder and neural theorem prover, showing that deep learning can advance even the most exacting fields.
We’re thinking: This work shows deep learning’s potential for synergy between humans and machines: People supply an algorithm (such as matrix multiplication) and AI accelerates its runtime.
Data Points
ChatGPT is helping autistic people practice communication skills
The chatbot is serving as a platform to help autistic and other neurodivergent people develop scripts for interacting socially and articulating emotions. (Wired)
Organization that supports people with eating disorders withdrew chatbot
The National Eating Disorder Association took down Tessa, a chatbot that recently replaced human workers, after it suggested dangerous dieting advice to users. (Daily Dot)
Research: PandaGPT can write, see, and hear
Researchers developed a versatile model that performs complex tasks such as generating detailed image descriptions, writing video-inspired stories, and answering audio-related questions. (GitHub)
Australia considers banning some uses of AI
The Australian government is contemplating a prohibition on “high-risk” applications of AI like deepfakes, citing concerns like their potential to manipulate democratic processes and deceive people. (The Guardian)
Alibaba introduced Tongyi Qianwen chatbot
The chatbot, which responds in Chinese and English for the company’s business apps, is available for public tests. It can analyze multimedia content and generate text summaries from video and audio files. (CNBC)
AI-driven “camera” with no lens
Paragraphica, which is an art project rather than a product, captures location data and inserts it into a prompt, causing an image generator to create an image of the scene before it. The device is available as a physical prototype and on the web. (Creative Bloq)
Amazon is using AI to detect damaged merchandise
Amazon's major warehouses use computer vision to screen products for damage prior to shipment. The system is three times more effective than human workers, according to the company. (The Wall Street Journal)
Research: Toward optimal AI evaluation
“Saliency cards” assist users in choosing the most suitable method to evaluate AI models. (MIT News)