Dear friends,
Happy New Year!
Every winter holiday, I pursue a learning goal around a new topic. In between visits with family, I end up reading a lot.
About a decade ago, my holiday topic was pedagogy — I still remember lugging a heavy suitcase of books through the airport — and this helped the early days of Coursera. Last year, before Nova’s birth, I read a pile of books on child care.
This holiday, I’ve been catching up on epigenetics and the emerging science (and sometimes quackery) of anti-aging.
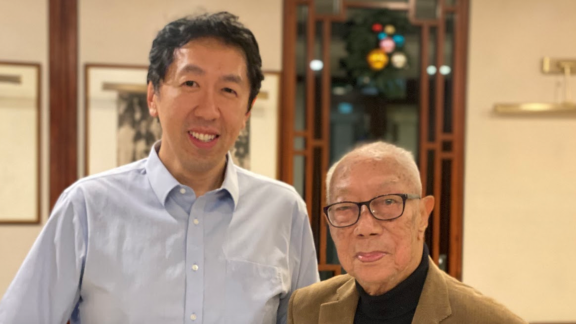
I also visited my 101-year-old grandfather. I told him what I was reading, and he said that remaining curious is the key to longevity.
If he’s right, then I think many of you will thrive well past 101!
Wishing you a wonderful 2020, with lots of curiosity, learning, and love.
Keep learning!
Andrew
High Hopes for 2020
We enter a new decade with great expectations of prosperity, as machine learning finds its place in traditional industries from manufacturing to the arts. Yet we face important questions about how to use it without causing harm through careless data collection, slipshod system design, or the limits of our ability to see around the next corner. In this special issue of The Batch, some of the brightest lights in AI express their hopes for 2020.
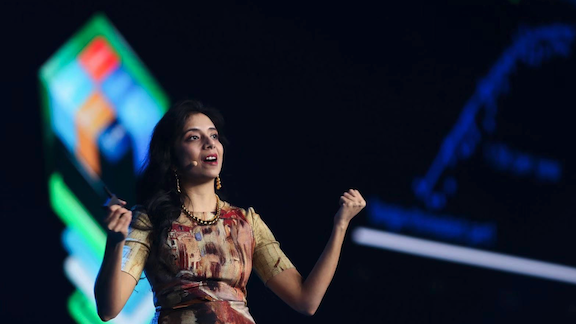
Anima Anandkumar: The Power of Simulation
We’ve had great success with supervised deep learning on labeled data. Now it’s time to explore other ways to learn: training on unlabeled data, lifelong learning, and especially letting models explore a simulated environment before transferring what they learn to the real world. In 2020, I hope to see more research in those areas.
High-fidelity simulation lets us train and test algorithms more effectively, leading to more robust and adaptive networks. Models can gain far more experience in the virtual world than is practical in the real world. We can simulate rare events that pose severe challenges but are seldom represented by ground truth.
For instance, when we’re driving a car, accidents are rare. You won’t see all the variations even if you drive hundreds of thousands of miles. If we train autonomous cars only on real-world data, they won’t learn how to manage the wide variety of conditions that contribute to accidents. But in a simulation, we can generate variation upon variation, giving the model a data distribution that better reflects real-world possibilities, so it can learn how to stay safe.
Lately, simulation has helped achieve impressive results in reinforcement learning, which is extremely data-intensive. But it’s also useful in supervised learning, when researchers may have only small amounts of real-world data. For instance, earthquakes are rare and difficult to measure. But researchers at Caltech’s seismology lab used a simple physical model to create synthetic data representing these events. Trained on synthetic data, their deep learning model achieved state-of-the-art results predicting properties of real-world earthquakes.
At Nvidia, we’ve developed powerful simulation platforms like Drive Constellation for autonomous vehicles and Isaac for robotics. These open, scalable environments enable models to act in a photorealistic virtual world, complete with highly accurate physics.
I hope that more AI scientists will come to recognize the value of training in simulated environments, as well as other techniques beyond supervised learning. That would make 2020 a year of great progress in AI.
Anima Anandkumar is director of machine learning research at Nvidia and a professor of computer science at Caltech.
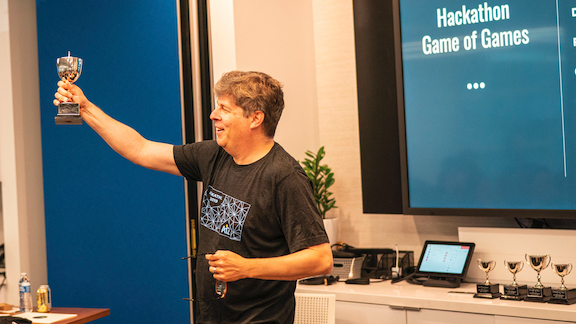
Oren Etzioni: Tools For Equality
In 2020, I hope the AI community will grapple with issues of fairness in ways that tangibly and directly benefit disadvantaged populations.
We’ve spent a lot of time talking about fairness and transparency in our algorithms, and this is essential work. But developing software tools that have a tangible impact is where the rubber meets the road. AI systems designed to improve people’s lives could help solve some of society’s major challenges.
Imagine what it’s like to use a smartphone navigation app in a wheelchair — only to encounter a stairway along the route. Even the best navigation app poses major challenges and risks if users can’t customize the route to avoid insurmountable obstacles.
Technology exists to support people with limited mobility, including AccessMap, a project of the University of Washington’s Taskar Center for Accessible Technology. But we could do so much more. Thankfully, we are living in a time when we have the means to do it at our fingertips.
Accessibility, education, homelessness, human trafficking — AI could have a major positive impact on people’s quality of life in these areas and others. So far, we’ve only scratched the surface. Let’s dig deep in the coming year.
Oren Etzioni is chief executive of the Allen Institute for AI, a professor of computer science at the University of Washington, and a partner at Madrona Venture Group.
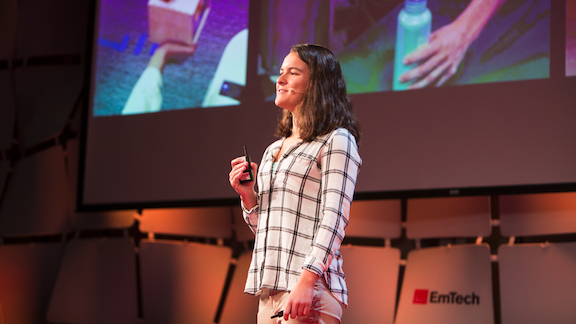
Chelsea Finn: Robots That Generalize
Many people in the AI community focus on achieving flashy results, like building an agent that can win at Go or Jeopardy. This kind of work is impressive in terms of complexity. But it’s easy to forget another important axis of intelligence: generalization, the ability to handle a variety of tasks or operate in a range of situations. In 2020, I hope to see progress on building models that generalize.
My work involves using reinforcement learning to train robots that reason about how their actions will affect their environment. For example, I’d like to train a robot to perform a variety of tasks with a variety of objects, such as packing items into a box or sweeping trash into a dustpan. This can be hard to accomplish using RL.
In supervised learning, training an image recognizer on ImageNet’s 14 million pictures tends to result in a certain degree of generalization. In reinforcement learning, a model learns by interacting with a virtual environment and collecting data as it goes. To build the level of general skill we’re accustomed to seeing in models trained on ImageNet, we need to collect an ImageNet-size dataset for each new model. That’s not practical.
If we want systems trained by reinforcement learning to generalize, we need to design agents that can learn from offline datasets, not unlike ImageNet, as they explore an environment. And we need these pre-existing datasets to grow over time to reflect changes in the world, just as ImageNet has grown from its original 1 million images.
This is starting to happen. For example, robots can figure out how to use new objects as tools by learning from a dataset of their own interactions plus demonstrations performed by humans guiding a robot’s arm. We’re figuring out how to take advantage of data from other institutions. For instance, we collected a dataset of robots interacting with objects from seven different robot platforms across four institutions.
It’s exciting to see critical mass developing around generalization in reinforcement learning. If we can master these challenges, our robots will be a step closer to behaving intelligently in the real world, rather than doing intelligent-looking things in the lab.
Chelsea Finn is an assistant professor of computer science and electrical engineering at Stanford.
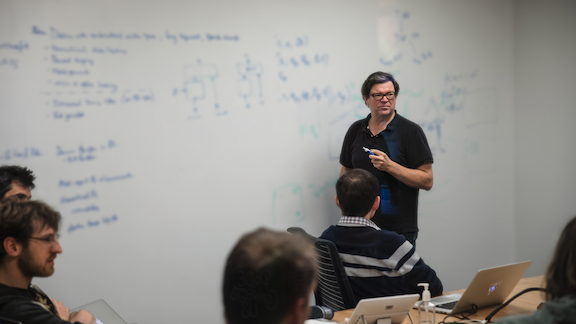
Yann LeCun: Learning From Observation
How is it that many people learn to drive a car fairly safely in 20 hours of practice, while current imitation learning algorithms take hundreds of thousands of hours, and reinforcement learning algorithms take millions of hours? Clearly we’re missing something big.
It appears that humans learn efficiently because we build a model of the world in our head. Human infants can hardly interact with the world, but over the first few months of life they absorb a huge amount of background knowledge by observation. A large part of the brain apparently is devoted to understanding the structure of the world and predicting things we can’t directly observe because they’re in the future or otherwise hidden.
This suggests that the way forward in AI is what I call self-supervised learning. It’s similar to supervised learning, but instead of training the system to map data examples to a classification, we mask some examples and ask the machine to predict the missing pieces. For instance, we might mask some frames of a video and train the machine to fill in the blanks based on the remaining frames.
This approach has been extremely successful lately in natural language understanding. Models such as BERT, RoBERTa, XLNet, and XLM are trained in a self-supervised manner to predict words missing from a text. Such systems hold records in all the major natural language benchmarks.
In 2020, I expect self-supervised methods to learn features of video and images. Could there be a similar revolution in high-dimensional continuous data like video?
One critical challenge is dealing with uncertainty. Models like BERT can’t tell if a missing word in a sentence is “cat” or “dog,” but they can produce a probability distribution vector. We don’t have a good model of probability distributions for images or video frames. But recent research is coming so close that we’re likely to find it soon.
Suddenly we’ll get really good performance predicting actions in videos with very few training samples, where it wasn’t possible before. That would make the coming year a very exciting time in AI.
Yann LeCun is vice president and chief AI scientist at Facebook and a professor of computer science at New York University.
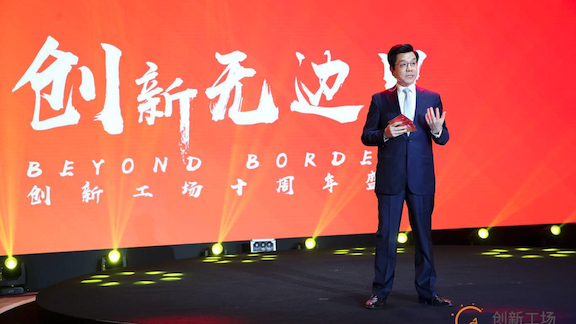
Kai-Fu Lee: AI Everywhere
Artificial intelligence has moved from the age of discovery to the age of implementation. Among our invested portfolios, primarily in China, we see flourishing applications using AI and automation in banking, finance, transportation, logistics, supermarkets, restaurants, warehouses, factories, schools, and drug discovery.
Yet, looking at the overall economy, only a small percentage of businesses is starting to use AI. There is immense room for growth.
I believe that AI will be as important as electricity in the history of mankind’s technological advancement. In the next decade or two, AI will penetrate our personal and business lives, delivering higher efficiency and more intelligent experiences. It is time for businesses, institutions, and governments to embrace AI fully and move society forward.
I am most excited about the impact of AI on healthcare and education. These two sectors are ready for AI disruption and can deploy AI for good.
We invested in a company that uses AI and big data to optimize supply chains, reducing medication shortages for over 150 million people living in rural China. We are also funding drug discovery companies that combine deep learning and generative chemistry to shorten drug discovery time by a factor of three to four.
In education, we see companies developing AI solutions to improve English pronunciation, grade exams and homework, and personalize and gamify math learning. This will free teachers from routine tasks and allow them to spend time building more inspirational and stimulating connections with up-and-coming generations of students.
I hope to see more bright entrepreneurs and businesses start using AI for good in 2020 and years to come.
Kai-Fu Lee is chairman and chief executive of Sinovation Ventures.
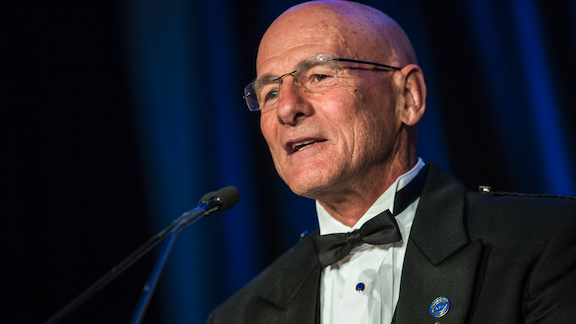
David Patterson: Faster Training and Inference
Billions of dollars invested to create novel AI hardware will bear their early fruit in 2020.
Google unleashed a financial avalanche with its tensor processing unit in 2017. The past year saw specialized AI processors from Alibaba, Cerebras, Graphcore, Habana, and Intel, with many others in the pipeline. These new chips will find their way slowly into research labs and data centers. I hope the AI community will embrace the best of them, pushing the field toward better models and more valuable applications.
How can machine learning engineers know whether a newfangled alternative performs better than the conventional CPU-plus-GPUs combo?
Computer architecture is graded on a curve rather than an absolute scale. To account for differing computer sizes, we normalize performance by price, power, or numbers of chips. Competitors select a set of representative programs to serve as a benchmark. Averaging scores across many of these programs is more likely to reflect real performance than scores on any single one.
MLPerf is a recent benchmark for machine learning created by representatives from more than 50 companies and nine universities. It includes programs, data sets, and ground rules for testing both inference and training, specifying important details like the accuracy target and valid hyperparameter values. New versions occur every three months (alternating inference and training) to keep up with rapid advances in machine learning.
Not every product can win a fair comparison, so some marketing departments may sidestep MLPerf, saying some version of, “Our customers don’t care about the programs in MLPerf.” But don’t be fooled. First, MLPerf welcomes new programs, so if a given workload isn’t in MLPerf, it can be added. Second, competitors check MLPerf results for fairness to ensure apples-to-apples comparisons.
Caveat emptor. Ask to see MLPerf scores!
David Patterson is a professor of computer science at UC Berkeley.
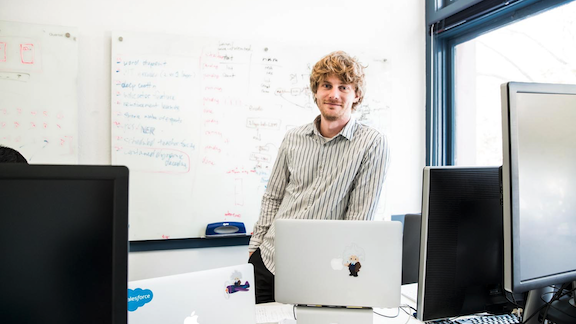
Richard Socher: Boiling the Information Ocean
Ignorance is a choice in the Internet age. Virtually all of human knowledge is available for the cost of typing a few words into a search box.
But managing the deluge of facts, opinions, and perspectives remains a challenge. It can be hard to know what information you’ll find in a lengthy document until you’ve read it, and knowing whether any particular statement is true is very difficult.
Automatic summarization can do a lot to solve these problems. This is one of the most important, yet least solved, tasks in natural language processing. In 2020, summarization will take important steps forward, and the improvement will change the way we consume information.
The Salesforce Research team recently took a close look at the field and published a paper that evaluates the strengths and weaknesses of current approaches. We found that the datasets used to train summarizers are deeply flawed. The metric used to measure their performance is deeply flawed. Consequently, the resulting models are deeply flawed.
We’re working on solutions to these problems. For instance, researchers evaluate summarization performance using the ROUGE score, which measures overlap in words between source documents, automated summaries, and human-written summaries. It turns out that summarizers based on neural networks can make mistakes and still earn high ROUGE scores. A model can confuse the names of a crime’s perpetrator and its victim, for example. ROUGE measures the fact that the names appear in both generated and human-made summaries without taking the error into account.
We introduced a model that makes it easy to examine factual consistency between source documents and summaries. We also proposed a metric to evaluate summarizers for factual consistency. Ranking summarizers according to this metric in addition to ROUGE will help researchers develop better models, and that will speed progress in other areas, such as maintaining logical coherence throughout a long summary.
This kind of development gives me confidence that 2020 will be a great time for summarization, and for NLP in general. The progress I expect to see in the coming year will help people not only to cope with the ceaseless flood of new information, but also to embrace AI’s great potential to make a better world.
Richard Socher is chief scientist at Salesforce.
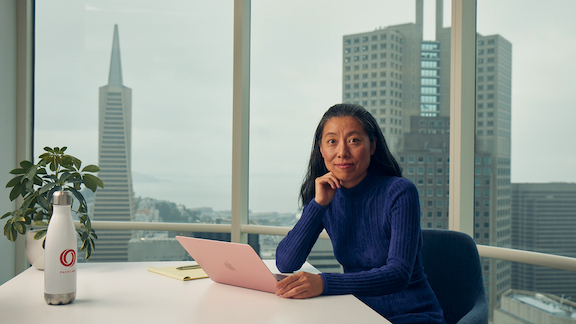
Dawn Song: Taking Responsibility for Data
Datasets are critical to AI and machine learning, and they are becoming a key driver of the economy. Collection of sensitive data is increasing rapidly, covering almost every aspect of people’s lives. In its current form, this data collection puts both individuals and businesses at risk. I hope that 2020 will be the year when we build the foundation for a responsible data economy.
Today, users have almost no control over how data they generate are used. All kinds of data are shared and sold, including fine-grained locations, medical prescriptions, gene sequences, and DMV registrations. This activity often puts personal privacy and sometimes even national security at risk. As individuals become more aware of these issues, they are losing trust in the services they use.
At the same time, businesses and researchers face numerous challenges in taking advantage of data. First, large scale data breaches continue to plague businesses. Second, with Europe’s General Data Protection Regulation, California’s Consumer Privacy Act, and similar laws, it is becoming more difficult and expensive for businesses to comply with privacy regulations. Third, valuable data are siloed, impeding technical progress. For example, easier use of medical data across institutions for machine learning could lead to improvements in healthcare for everyone.
Changing this broken system into a responsible data economy requires creating new technologies, regulations, and business models. These should aim to provide trustworthy protection and control to data owners (both individuals and businesses) through secure computation, the ability to audit, and machine learning that maintains data privacy. Secure computation can be provided by secure hardware (such as Intel SGX and Keystone Enclave) and cryptographic techniques. Those computations can be made auditable by tying encrypted storage and computation to a distributed ledger.
Greater challenges remain on the machine learning side. In 2020, we can expand on current efforts in differentially private data analytics and machine learning, building scalable systems for practical deployment with large, heterogeneous datasets. Further research and deployment of federated learning also will be important for certain use cases. Finally, advances in robust learning from limited and noisy data could help enable a long tail of ML use cases without compromising privacy.
We are building parts of this vision at Oasis Labs, but there is much more to be done. I hope this year that technologists, businesses, regulators, and the AI community will join us in building the foundation for a truly responsible data economy.
Dawn Song is chief executive and co-founder of Oasis Labs and a professor of computer science and electrical engineering at UC Berkeley.
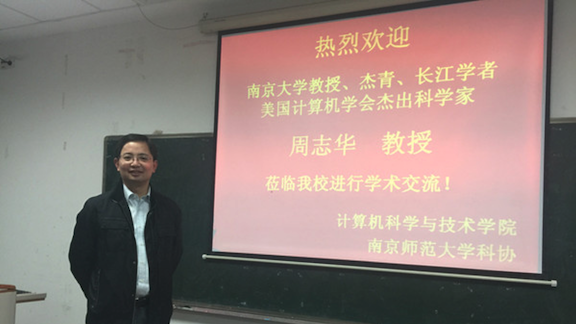
Zhi-Hua Zhou: Fresh Methods, Clear Guidelines
I have three hopes for 2020:
- Hope that advanced machine learning techniques beyond deep neural networks can emerge. Neural networks have been studied and applied by many researchers, engineers, and practitioners for a long time. Other machine learning techniques offer relatively unexplored spaces for technical innovation.
- Hope that AI can come into more fields and bring more positive changes to people’s everyday lives.
- Hope for more thinking and discussion about what AI researchers, engineers, and practitioners must do to prevent wrong developments or misuses of AI techniques.
Zhi-Hua Zhou is a professor of computer science and artificial intelligence at Nanjing University.