Dear friends,
Last week, we released a new course, ChatGPT Prompt Engineering for Developers, created in collaboration with OpenAI. This short, 1.5-hour course is taught by OpenAI’s Isa Fulford and me. This has been the fastest-growing course I’ve ever taught, with over 300,000 sign-ups in under a week. Please sign up to take it for free!
Many people have shared tips on how to use ChatGPT’s web interface, often for one-off tasks. In contrast, there has been little material on best practices for developers who want to build AI applications using API access to these hugely powerful large language models (LLMs).
LLMs have emerged as a new AI application development platform that makes it easier to build applications in robotic process automation, text processing, assistance for writing or other creative work, coaching, custom chatbots, and many other areas. This short course will help you learn what you can do with these tools and how to do it.
Say, you want to build a classifier to extract names of people from text. In the traditional machine learning approach, you would have to collect and label data, train a model, and figure out how to deploy it to get inferences. This can take weeks. But using an LLM API like OpenAI’s, you can write a prompt to extract names in minutes.
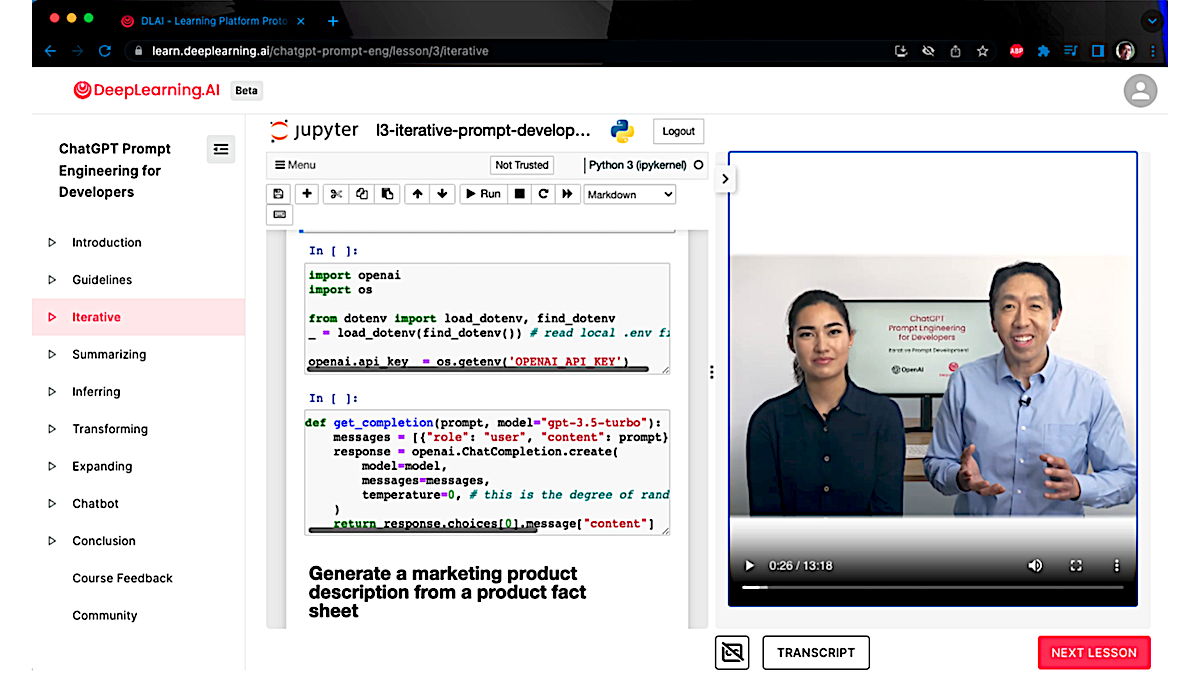
In this short course, Isa and I share best practices for prompting. We cover common use cases such as:
- Summarizing, such as taking a long text and distilling it
- Inferring, such as classifying texts or extracting keywords
- Transforming, such as translation or grammar/spelling correction
- Expanding, such as using a short prompt to generate a custom email
We also cover how to build a custom chatbot and show how to construct API calls to build a fun pizza order-taking bot.
In this course, we describe best practices for developing prompts. Then you can try them out yourself via the built-in Jupyter notebook (the middle portion of the image above). If you want to run the provided code, you can hit Shift-Enter all the way through the notebook to see its output. Or you can edit the code to gain hands-on practice with variations on the prompts.
Many applications that were very hard to build can now be built quickly and easily by prompting an LLM. So I hope you’ll check out the course and gain the important skill of using prompts in development. Hopefully you’ll also come away with new ideas for fun things that you want to build yourself!
Keep learning!
Andrew
News
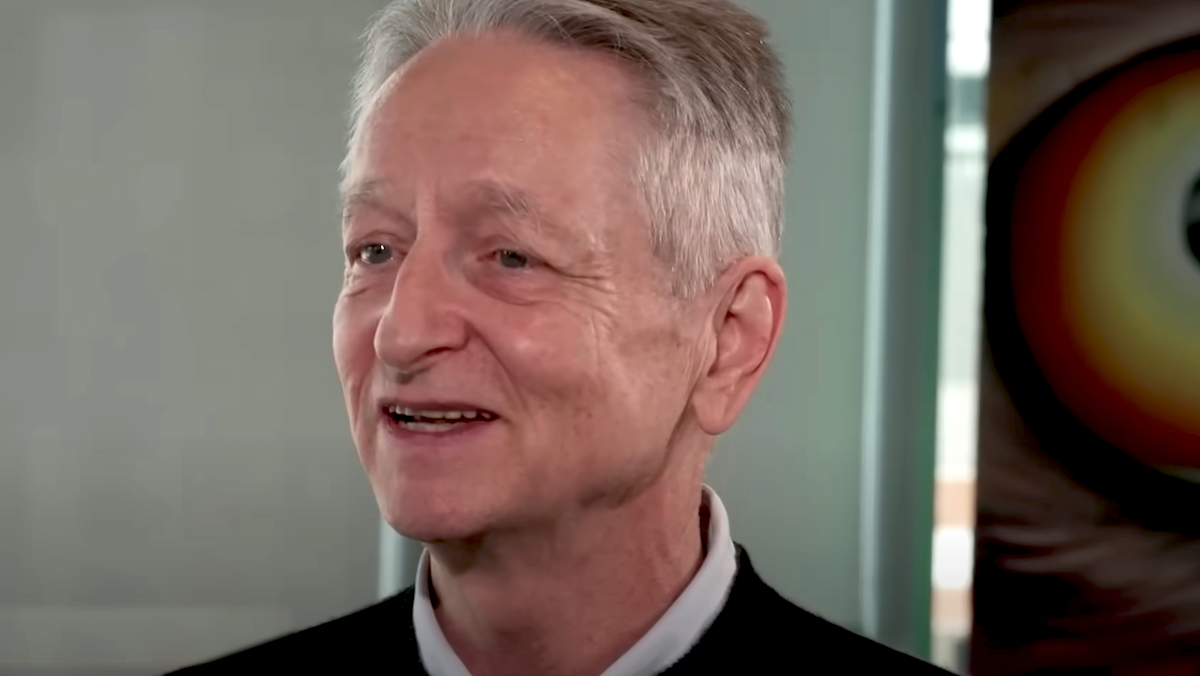
Hinton Leaves Google With Regrets
A pioneer of deep learning joined the chorus of AI insiders who worry that the technology is becoming dangerous, saying that part of him regrets his life’s work.
What’s new: Geoffrey Hinton, who has contributed to groundbreaking work on neural networks since the 1980s, stepped down from his role as a vice president and engineering fellow at Google so he could voice personal concerns about AI’s threat to society, The New York Times reported. He believes that Google has acted responsibly in its AI development, he added in a subsequent tweet.
Why he stepped down: AI models have improved faster than Hinton had expected, and the generative AI gold rush led him to believe that the financial rewards of innovating would overwhelm incentives to rein in negative effects. In addition, at 75, he has become “too old to do technical work,” he told MIT Technology Review. Instead, he will focus on philosophical matters. Among his concerns:
- Generated media could erode the average person’s ability to gauge reality.
- AI models could cause massive unemployment by automating rote work, and perhaps not-so-rote work.
- Automated code generators eventually could write programs that put humans at risk.
- Hinton supports global regulation of AI but worries that it would be ineffective. Scientists probably can devise more effective safeguards than regulators, he said.
Behind the news: Hinton’s contributions to deep learning are myriad. Most notably, he helped popularize the use of backpropagation, the core algorithm for training neural networks; invented the dropout technique to avoid overfitting; and led development of AlexNet, which revolutionized image classification. In 2018, he received the Turing Award alongside Yann LeCun and Yoshua Bengio for contributions to AI.
Why it matters: Hinton’s thoughts about AI risks are exceptionally well informed. His concerns sound a note of caution for AI practitioners to evaluate the ethical dimensions of their work and stand by their principles.
We’re thinking: Geoffrey Hinton first joined Google as a summer intern (!) at Google Brain when Andrew led that team. His departure marks the end of an era. We look forward to the next phase of his career.
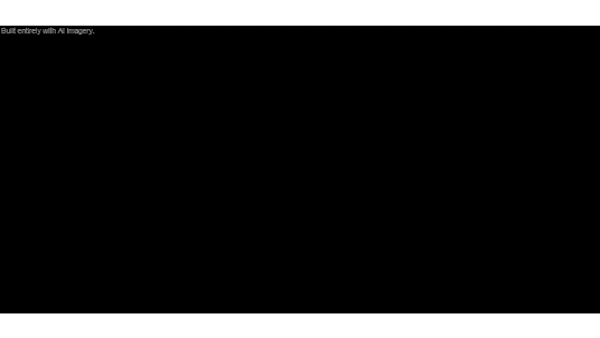
U.S. Politics Go Generative
A major political party in the United States used generated imagery in a campaign ad.
What’s new: The Republican Party released a video entirely made up of AI-generated images. The production, which attacks incumbent U.S. president Joe Biden — who leads the rival Democratic Party — marks the arrival of image generation in mainstream U.S. politics.
Fake news: The ad depicts hypothetical events that purportedly might occur if Biden were to win re-election in 2024. Voice actors read fictional news reports behind a parade of images that depict a military strike on Taipei due to worsening relations between the U.S. and China, boarded-up windows caused by economic collapse, a flood of immigrants crossing the southern border, and armed soldiers occupying San Francisco amid a spike in crime.
- The images display “Built entirely with AI imagery” in tiny type in the upper left-hand corner.
- An anonymous source familiar with the production told Vice that a generative model produced the images and human writers penned its script.
Behind the news: Generative AI previously infiltrated politics in other parts of the world.
- In 2022, both major candidates in South Korea’s presidential election created AI-generated likenesses of themselves answering voters’ questions.
- In 2020, in a regional election, an Indian political party altered a video of its candidate so his lips would match recordings of the speech translated into other languages.
- In 2019, a video of Gabon’s president Ali Bongo delivering a speech triggered a failed coup attempt after rumors spread that the video was deepfaked.
Why it matters: Political campaigns are on the lookout for ways to get more bang for their buck, and using text-to-image generators may be irresistible. In this case, the producers used fake — but realistic — imagery to stand in for reality. Despite the small-type disclaimer, the images make a visceral impression that fictional events are real, subverting the electorate's reliance on an accurate view of reality to decide which candidates to support. The power of such propaganda is likely to grow as generative video improves.
We’re thinking: This use of generated images as propaganda isn’t limited to political jockeying. Amnesty International recently tweeted — and sensibly deleted — a stirring image of a protester detained by Colombian police bearing the fine print, “Illustrations produced by artificial intelligence.” Organizations that seek to inform their audiences about real-world conditions counteract their own interests when they illustrate those conditions using fake images.
A MESSAGE FROM DEEPLEARNING.AI
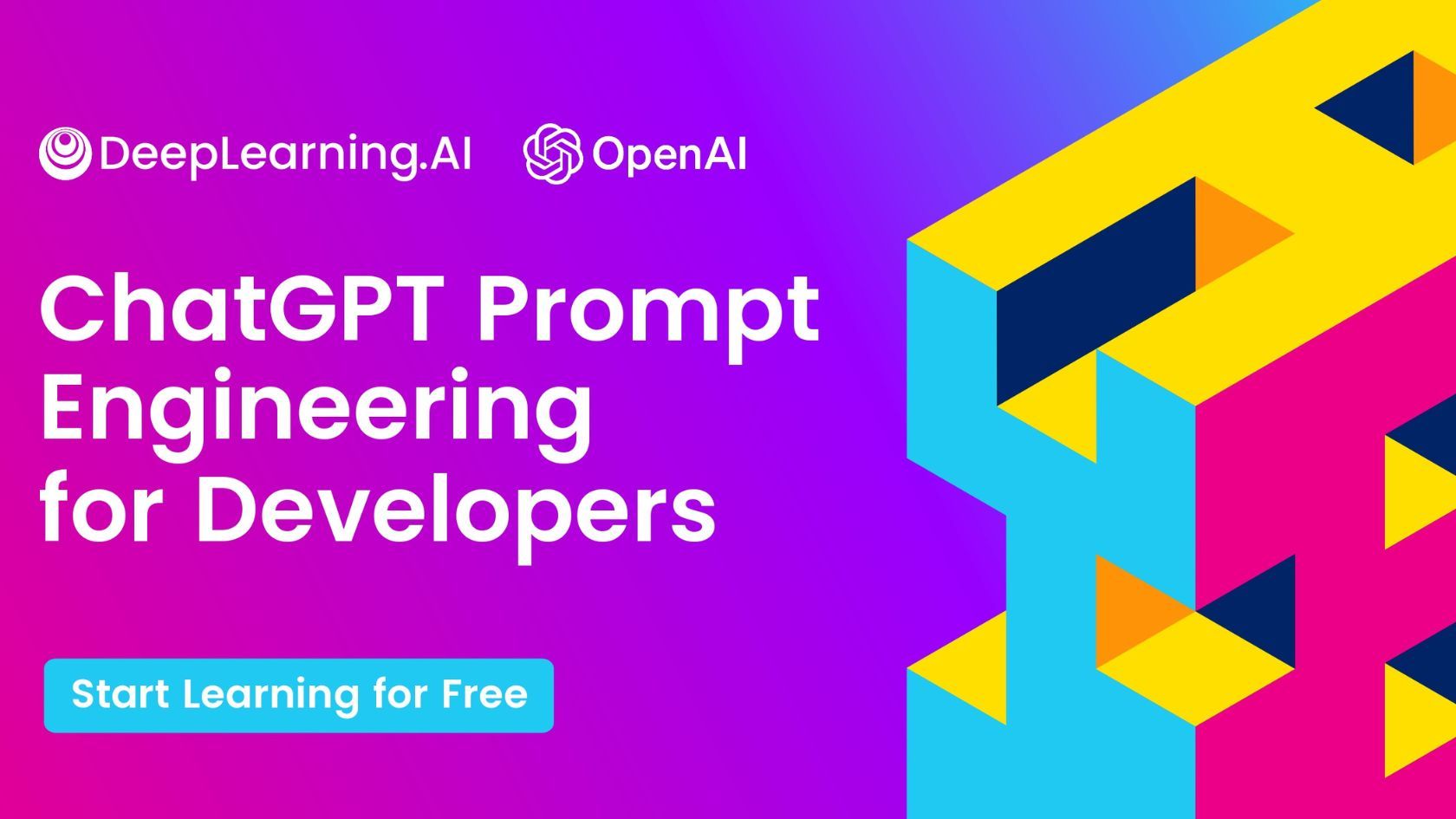
Learn new use cases for large language models and improve your ChatGPT API skills in our one-hour course, “ChatGPT Prompt Engineering for Developers.” Sign up for free
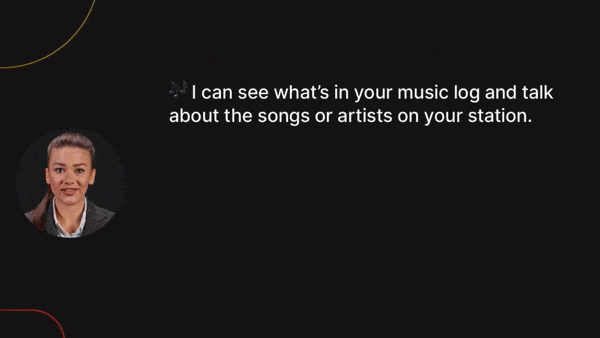
Radio Stations Test AI DJs
A language model will stand in for radio disk jockeys.
What’s new: RadioGPT generates radio shows tailored for local markets. The system, which is undergoing tests across North America, is a product of Futuri, a company based in Cleveland, Ohio, that focuses on digital audience engagement.
How it works: Futuri’s proprietary Topic Pulse system determines trending topics in a radio station’s local market, and OpenAI’s GPT-4 generates a script. An unspecified model vocalizes the script using between one and three voices. Customers can choose a preset voice or clone their own.
- RadioGPT plays songs from a user-selected list. It punctuates the presentation with a chatty AI-generated voice that delivers factoids about songs or artists. It can also generate weather, traffic, and brief news reports.
- Listeners who download an app can send voice memos, and the automated DJ can incorporate them into its script. For instance, the DJ can play back listeners’ voices as though they were calling in and use their locations to call out specific areas within reach of the station’s signal.
- Alpha Media, which owns 207 radio stations in the United States, and Rogers Sports & Media, which owns 55 stations in Canada, will beta test the technology beginning in April, Axios reported.
Behind the news: Fully automated media programs are gaining momentum as AI models make it easy to produce endless amounts of text and audio.
- Music-streaming service Spotify recently launched its own automated DJ, which similarly spices up custom playlists with factoids and observations about the user’s listening habits.
- In January, a never-ending, AI-generated comedy based on the sitcom Seinfeld debuted on Twitch. It used GPT-3 to generate scripts, Microsoft Azure to simulate voices, and the Unity game engine to generate the scenes.
- In October, Dubai-based Play.ht launched a podcast that features simulations of famous — sometimes deceased — people. So far, the show has featured ersatz voices of Oprah Winfrey, Terence McKenna with Alan Watts, and Lex Friedman with Richard Feynman.
Why it matters: Many radio stations already are highly automated and rely for news on syndicated programming. AI-generated DJs that localize news and listener interactions can give them programming customized to their markets and may help them compete with streaming services.
We’re thinking: RadioGPT fits generative AI into a traditional radio workflow. Ultimately, we suspect, this tech will remake the medium in more fundamental ways.
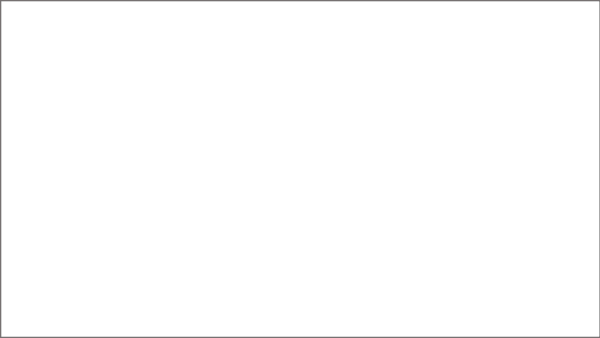
For Better Answers, Generate Reference Text
If you want a model to answer questions correctly, then enriching the input with reference text retrieved from the web is a reliable way to increase the accuracy of its output. But the web isn’t necessarily the best source of reference text.
What's new: Wenhao Yu at University of Notre Dame and colleagues at Microsoft and University of Southern California used a pretrained language model to generate reference text. They fed that material, along with a question, to a second pretrained language model that answered more accurately than a comparable model that was able to retrieve relevant text from the web.
Key insight: Given a question, documents retrieved from the web, even if they’re relevant, often contain information that doesn’t help to answer it. For instance, considering the question “How tall is Mount Everest?,” the Wikipedia page on Mount Everest contains the answer but also a lot of confusing information such as elevations attained in various attempts to reach the summit and irrelevant information that might distract the model. A language model pretrained on web pages can generate a document that draws on the web but focuses on the question at hand. When fed to a separate language model along with the question, this model-generated reference text can make it easier for that model to answer questions correctly.
How it works: The authors used a pretrained InstructGPT (175 billion parameters) to generate reference text related to questions in trivia question-answer datasets such as TriviaQA. They generated answers using FiD (3 billion parameters), which they had fine-tuned on the dataset plus the reference text. (A given question may have more than one valid answer.)
- InstructGPT generated reference text for each question in the dataset based upon a prompt such as, “Generate a background document to answer the given question,” followed by the question.
- The authors embedded each question-reference pair using GPT-3 and clustered the embeddings via k-means.
- At inference, the system randomly selected five question-reference pairs from each cluster — think of them as guide questions and answers.
- For each cluster, given an input question (such as, "What type of music did Mozart compose?") and the question-reference pairs, InstructGPT generated a document — information related to the question.
- Given the question and documents, FiD generated an answer. (Valid answers to the Mozart question include, "classical music," "opera," and "ballet.")
Results: The authors evaluated their fine-tuned FiD on TriviaQA according to the percentage of answers that exactly matched one of a list of correct answers. Provided with generated documents, FiD answered 71.6 percent of the questions correctly compared to 66.3 percent for FiD fine-tuned on TriviaQA and provided with text retrieved from Wikipedia using DPR.
Yes, but: The authors’ approach performed best (74.3 percent) when it had access to both Wikipedia and the generated documents. While generated documents may be better than retrieved documents alone, they worked best together.
Why it matters: Good reference text substantially improves a language model’s question-answering ability. While a relevant Wikipedia entry is helpful, a document that’s directly related to the question is better — even if that document is a product of text generation.
We're thinking: Your teachers were right — Wikipedia isn’t the best source.
Data Points
Nvidia introduces NeMo Guardrails, a software designed to regulate chatbots
The open source software can help developers ensure that AI-powered text generators remain on topic and don't take unauthorized actions. (Bloomberg)
The Washington Post analyzed popular web-scale text dataset
The news outlet, with help from Allen Institute for AI, categorized the contents of Google's C4 dataset, scraped from 15 million websites. C4 has been used to train large language models including Google’s T5 and Facebook’s LLaMA. (The Washington Post)
OpenAI introduced new ChatGPT privacy featureChatGPT users can opt to turn off their chat histories. This prevents OpenAI from using that data to train the company's models. (The Seattle Times)
AI-generated product reviews are flooding the web
Fake product reviews have proliferated on sites like Yelp and Amazon. They’re easy to identify because they contain the phrase “as an AI language model,” exposing a new wave of spam. (The Verge)
U.S. federal agencies issued a joint statement to battle AI bias and discrimination
Four law enforcement agencies outlined a commitment to enforce responsible use of AI in areas such as lending and housing. (The Wall Street Journal)
EU lawmakers agreed on a draft of the AI Act
Under the proposal, companies that develop generative AI tools would have to disclose the use of copyrighted material in their systems. EU member states must negotiate on details before the bill becomes law. (VentureBeat)
Apple preparing an AI-powered health coaching service
The service will motivate users and suggest exercise, healthy eating, and sleep habits based on their Apple Watch data. (Bloomberg)
PriceWaterhouseCoopers plans to invest $1 billion over three years in generative AI
Microsoft and OpenAI will help the accounting and consulting giant to automate its tax, audit, and consulting services. The investment will go into recruiting, training, and acquisitions. (The Wall Street Journal)
Elon Musk’s AI Company Comes into Focus
The Tesla CEO has hired researchers from DeepMind and Twitter. (The New York Times)
Inside Microsoft’s Development of Bing’s Chat Capabilities
Reporters told the story of how Microsoft worked with OpenAI to build Prometeus, the code name for the wedding of Bing search and the GPT-4 language model. (Wired)
Runway launches generative video mobile app
The app gives users access to Gen-1, Runway’s video generator. It enables them to transform videos using text prompts, image, or style prompts. (TechCrunch)
Inside Meta’s Effort to Innovate in AIMeta’s ambitions in AI were weighed down by an initiative to design its own AI chip, insiders said. (Reuters)