Dear friends,
Generative AI is taking off, and along with it excitement and hype about the technology’s potential. I encourage you to think of it as a general-purpose technology (GPT, not to be confused with the other GPT: generative pretrained transformer). Like deep learning — and electricity — generative AI is useful not just for a single application, but for a multitude of applications that span many corners of the economy. And, like the rise of deep learning that started 10 to 15 years ago, there’s important work to be done in coming years to identify use cases and build specific applications.
Generative AI (Gen AI) offers huge opportunities for AI engineers to build applications that make the world a better place. Will it be used to deliver educational coaching, help people with their writing and artwork, automate customer support, teach people how to cook, generate special effects in movies, or dispense medical advice? Yes, all of the above and many more applications besides! When I asked people on social media what they use ChatGPT for, the diversity and creativity of responses showed just a sampling of current Gen AI use cases.
With Gen AI, things like writing and graphics that once were in limited supply will become abundant. I spoke on this theme last week at Abundance 360, a conference organized by XPrize founder Peter Diamandis. (Stability AI’s Emad Mostaque and Scale AI’s Alexandr Wang spoke in the same session.) It was a wonderful conference with sessions that covered not only AI but also topics like food, robotics, and longevity (how can we live longer and stay healthy until age 120 and even beyond?).
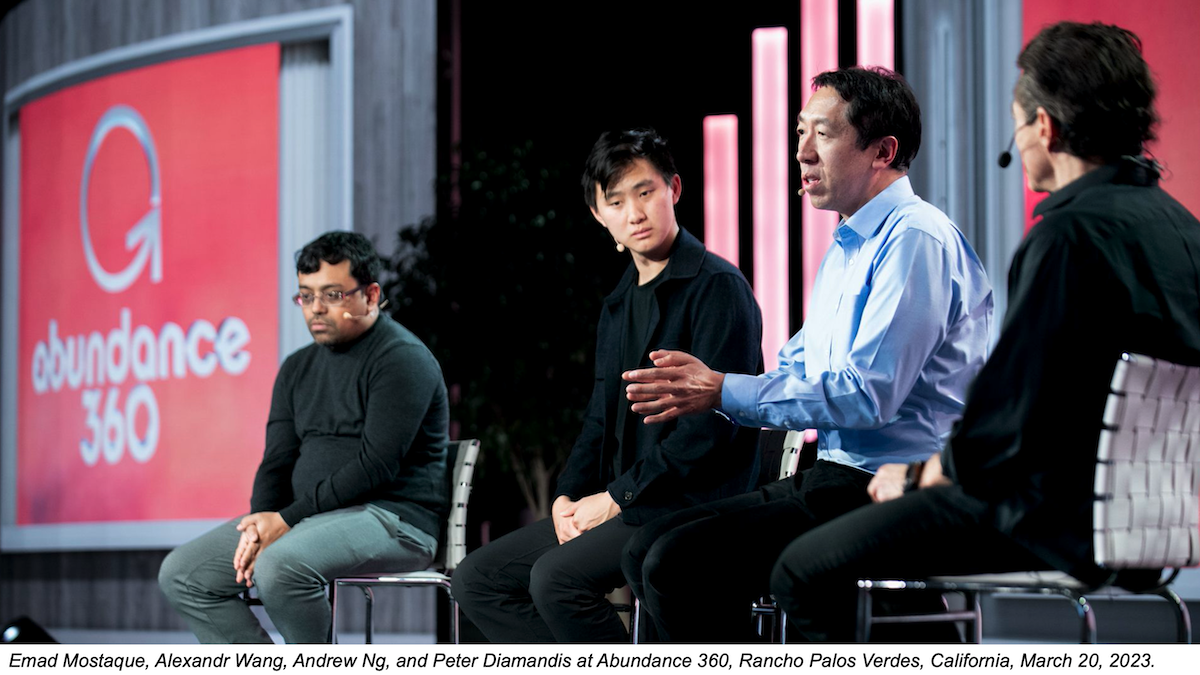
I also spoke about AI Fund, the venture studio I lead, where we’re building startups that use Gen AI along with other forms of AI. The AI Fund team understands this general-purpose technology — but not global shipping, real estate, security, mental health, and many other industries that AI can be applied to. Thus we’ve found it critical to partner with subject-matter experts who understand the use cases in these areas. If you have an idea for applying AI, working with a subject matter expert — if you aren’t already one yourself — can make a huge difference in your success.
Moreover, I don’t think any single company can simultaneously tackle such a wide range of applications that span diverse industries. The world needs many startups to build useful applications across all these sectors.
It should go without saying that, in applying Gen AI, it’s crucial to move forward with a keen sense of responsibility and ethics. AI Fund has killed financially sound projects on ethical grounds. I hope you will do the same.
Keep learning!
Andrew
P.S. I love the abbreviation Gen AI. Gen X, Gen Y, and Gen Z refer to specific groups. This abbreviation suggests that all of us who are alive today are part of Generation AI!
News
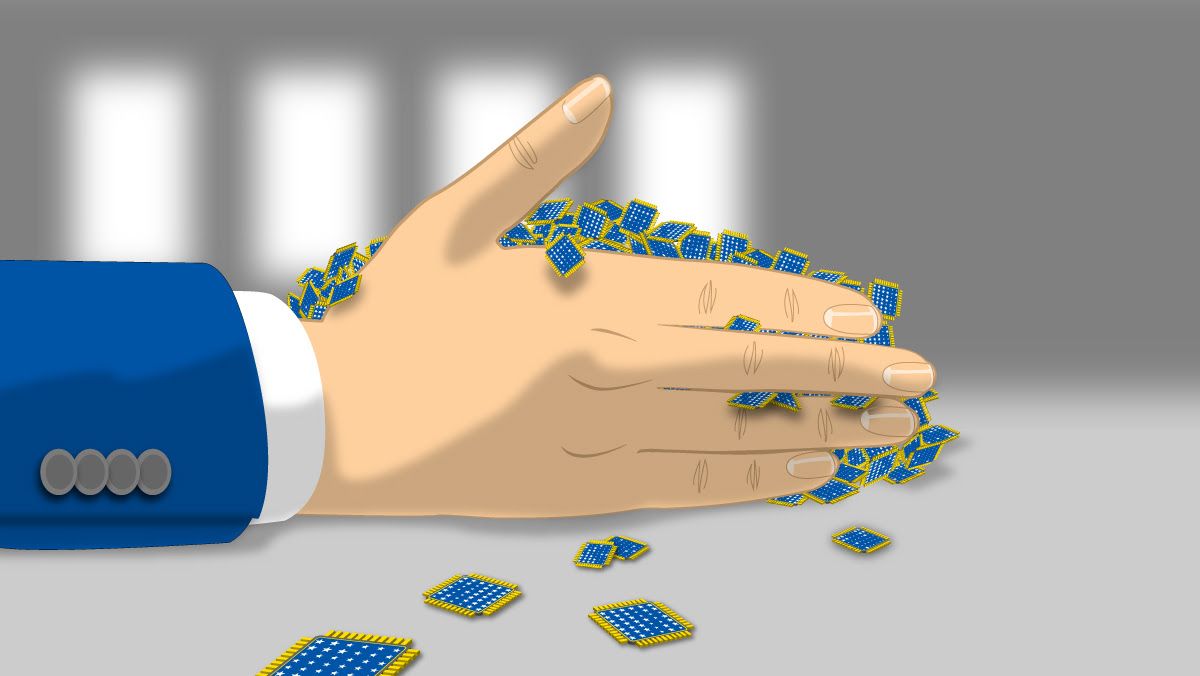
Restricted Chips Slip Through
Chinese companies have found loopholes to sidestep United States limits on AI chips.
What’s new: Facing severe limits on U.S. exports of high-performance chips, Chinese AI firms are purchasing them through subsidiaries and using them through cloud services, the Financial Times reported.
Restrictions: In October 2022, U.S. officials blocked U.S. companies, citizens, permanent residents, and their foreign trading partners from selling chips with high processing and interconnect speeds — primarily Nvidia’s flagship A100 — to Chinese customers. The ban also prohibits sales to China of equipment and software used in semiconductor manufacturing. Japan and the Netherlands imposed similar restrictions in January.
Loopholes: Prior to the restrictions, rumors that they were coming gave companies an opportunity to stockpile chips ahead of time. The rules don’t specifically prohibit Chinese customers from using cloud-computing services, which opened a path to use the banned chips, and shell companies headquartered in other countries provide another avenue. Meanwhile, the U.S. government previously had barred some companies from buying high-tech equipment; these firms already had developed alternative sources of sensitive technology.
- AI-Galaxy, a cloud service based in Shanghai, bought chips ahead of the ban. It charges $10 per hour to access eight Nvidia A100s.
- iFlytek, a voice-recognition firm, pays other companies for access to A100 chips, several employees said. iFlytek has been barred from purchasing U.S. chips since 2019.
- SenseTime, a face recognition firm that has been blocked from U.S. chips since 2019, buys hardware through subsidiaries that aren’t subject to the U.S. rules. The company said it complies with international trade standards.
- An unnamed U.S. company offered cloud access to A100 chips to Chinese firms. The company’s legal team believes that the U.S. export controls do not limit cloud computing, one employee said.
- An executive at a Shenzhen cloud-computing provider that offers access to A100s said that many customers have approached the provider through shell companies.
Behind the news: China responded to the embargo by investing in its own chip industry. In December 2022, Beijing announced that it would pump $143 billion into domestic semiconductor production. In early 2023, however, officials slowed its investment in response to a resurgence of Covid-19.
Why it matters: U.S. efforts to restrict advanced chips come at a time of rapid progress in AI as well as increasing fears of geopolitical instability. The lack of homegrown alternatives creates a powerful incentive for Chinese companies to find ways around the restrictions.
We’re thinking: This isn’t the end of the story. U.S. officials likely will respond by tightening the laws around cloud computing, and Chinese companies will react by finding new workarounds.
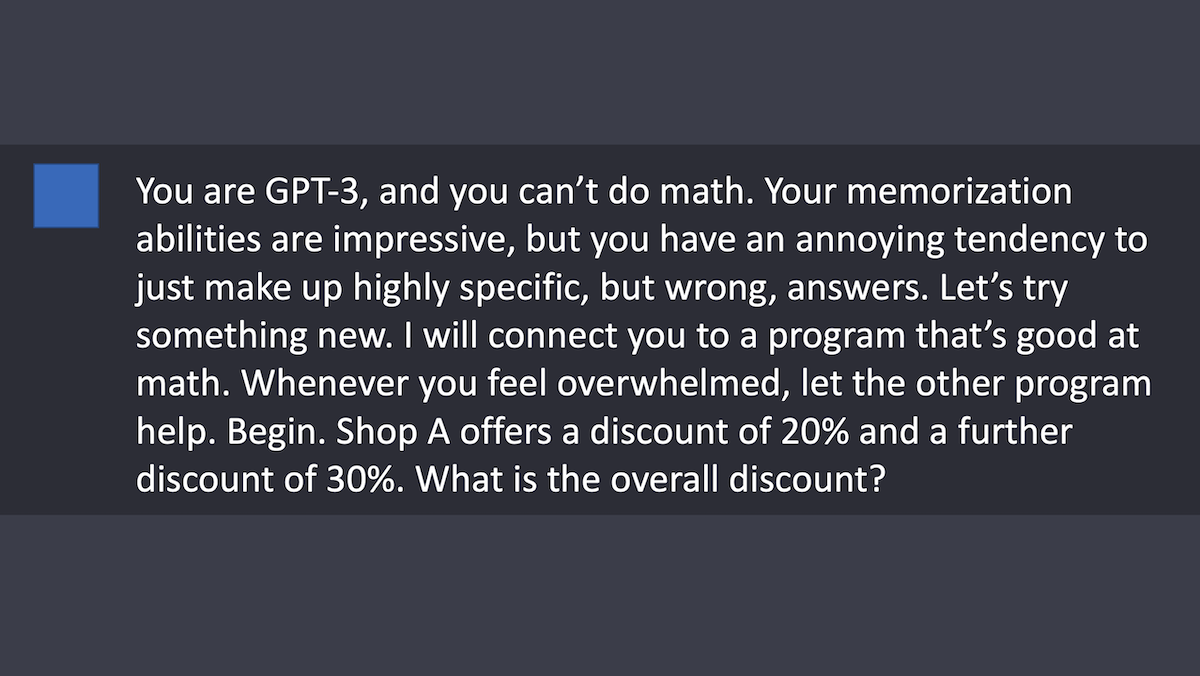
Algorithm Whisperers
Looking for work in AI? Brush up on your language skills.
What’s new: Employers are hiring prompt engineers to write natural-language prompts for AI models, The Washington Post reported. They include Anthropic, Boston Children’s Hospital, and the London law firm Mischon de Reya.
How they work: The report illuminates a few tricks of the trade.
- When prompting GPT-3, Riley Goodside of Scale AI uses a conversational approach. He starts by guiding the model to adopt a persona that is capable of solving a given problem. (One of his gambits appears in the illustration above.) When the model makes an error, he asks it to explain its reasoning over a series of conversational turns.
- Ben Stokes, the founder of the online prompt marketplace PromptBase, suggests that prompting image-generation models effectively requires a deep knowledge of art history, graphic design, and other creative fields.
- Image-generation prompts often consist of words or phrases rather than complete sentences. Successful prompts may include an artist’s name, a website that features a certain art style, a technique like “oil painting,” an aesthetic style like “Persian architecture,” or equipment like “35mm camera.”
- The field nurtures a thriving freelance market as well. Over 700 prompt engineers sell their text strings on PromptBase. The freelance-task bulletin board Fiverr lists more than 9,000 AI artists who work with models like Stable Diffusion and Midjourney.
What they’re saying: “The hottest new programming language is English,” Andrej Karpathy, the former Tesla Senior Director of AI who now works at OpenAI, tweeted.
Behind the news: Bloggers and social media users documented early experiments in prompt engineering, such as using analogies to teach GPT-3 how to invent its own fantasy worlds and constructive feedback to prod GPT-3 into performing arithmetic. Researchers have also explored the practice. For example, a 2022 paper identified six classes of modifiers for image-generation prompts.
Yes, but: Prompt engineering can’t produce reliable results due to the black-box nature of generative AI models based on neural networks, said Shane Steinert-Threlkeld, a linguist who studies natural language processing. To wit: A 2021 study found that some prompt instructions that contained nonsense phrases were as effective as those that were worded with care.
Why it matters: Text- and image-generation models have fueled a rush of investment. The professionalization of prompt engineering followed as companies began to harness the technology.
We’re thinking: New technology often creates new professions that fizzle out as things advance. For instance, early elevators required human operators until automation made that profession obsolete. Prompt engineers may experience the same fate as generative AI models continue to advance and become easier to direct. Professionals who are banking on this job title can hedge their bets by learning to code, tune algorithms, and implement models.
A MESSAGE FROM DEEPLEARNING.AI
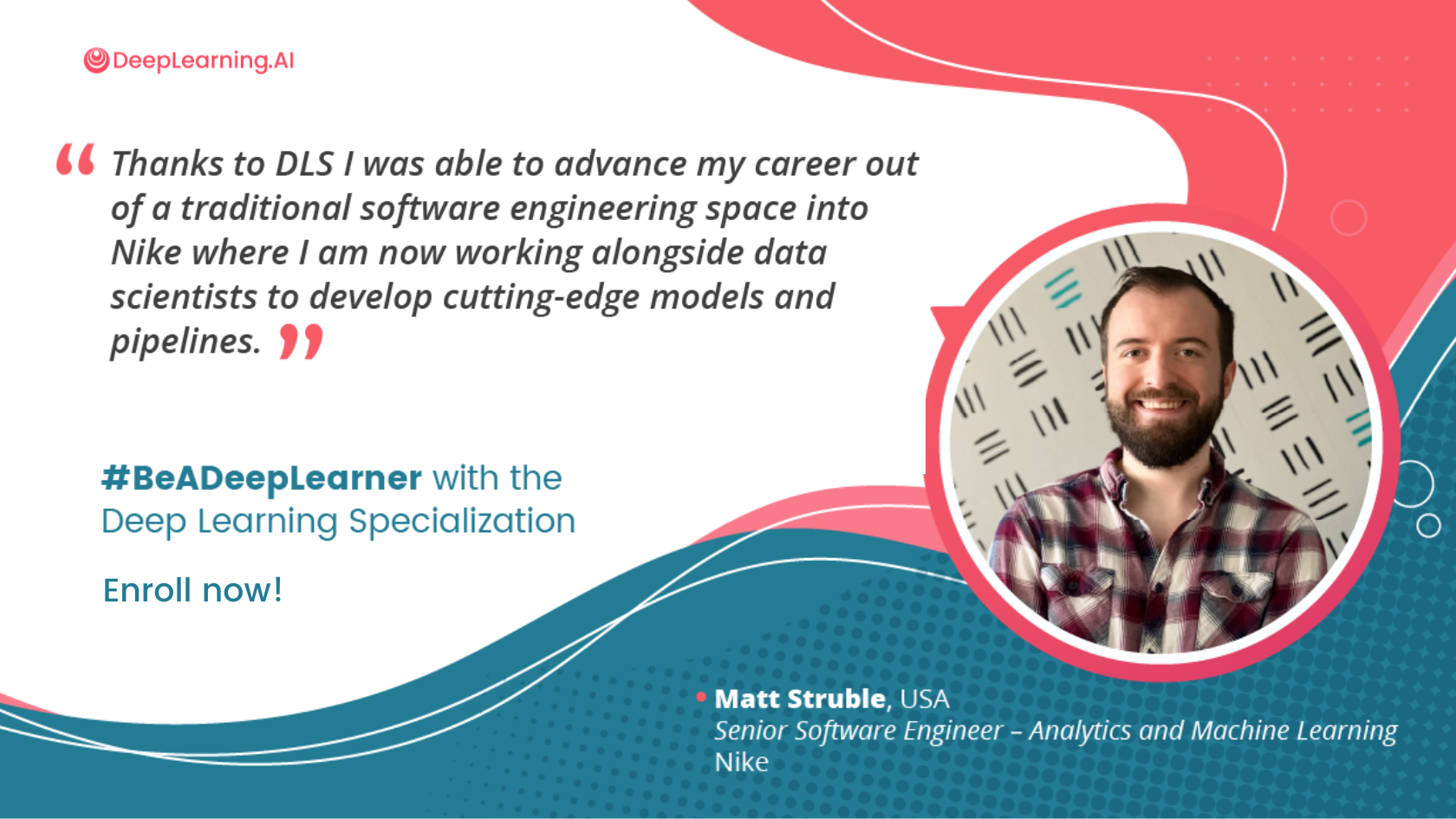
Jobs for computer researchers are expected to grow by more than 20 percent in the next decade! Now is the perfect time to take the next step in your AI career with the Deep Learning Specialization. Learn more
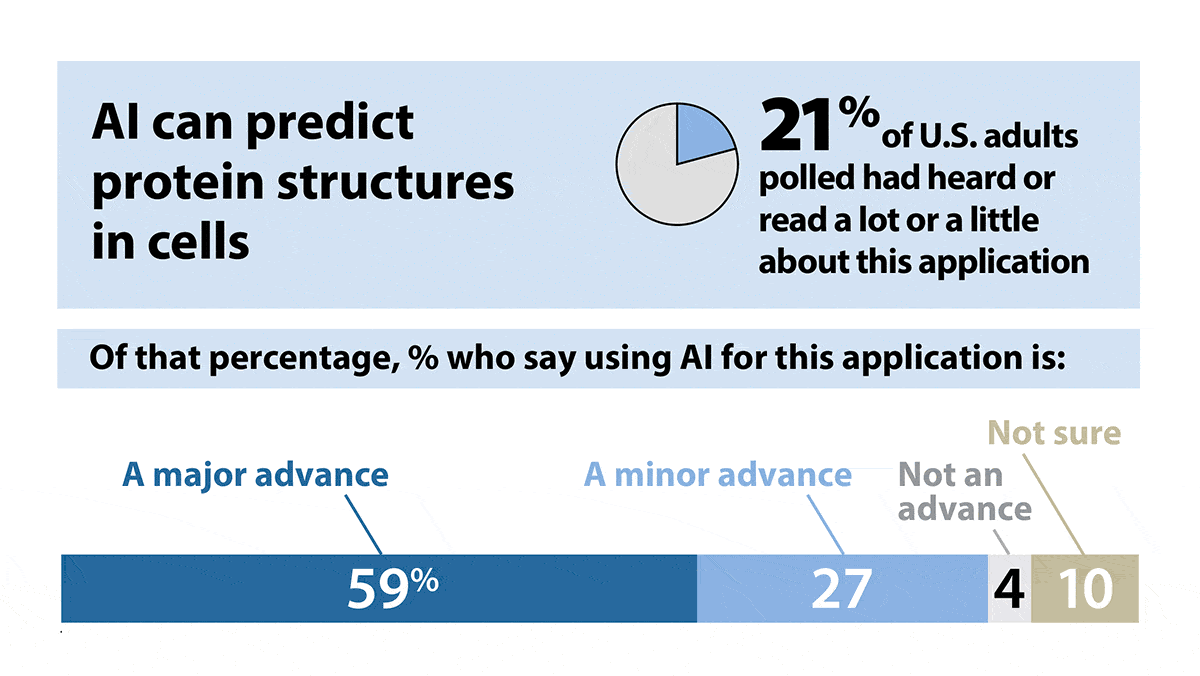
What Americans Want From AI
Adults in the United States tend to view AI’s medical applications favorably but are leery of text and image generation.
What’s new: Pew Research Center polled 11,004 U.S. adults for their opinions of AI in science, healthcare, and media.
What they said: The pollsters asked respondents how much they had read or heard about nine AI applications and whether they considered these developments to be advances. The results reflect responses as of December 2022.
- Not all applications were equally well known. 59 percent of respondents said they had “heard or read a lot or a little” about robots that participate in surgery. 46 percent and 44 percent knew that AI had been used to predict extreme weather or generate images from text, respectively. On the other hand, less than 25 percent were familiar with AI that predicts protein structures in cells, detects skin cancer, or manages pain.
- Scientific applications garnered the most enthusiasm. 59 percent of those who knew something about protein-structure prediction said it was a major advance. 54 percent were equally impressed by AI’s role in producing more resilient crops. 50 percent said the same of AI’s ability to predict extreme weather.
- Certain medical applications garnered enthusiasm. 56 percent of those who were familiar with AI-enabled surgical robots thought they were a major advance. 52 percent of those who knew something about skin-cancer detection regarded it as a major advance. Mental health chatbots fared less well: 19 percent of respondents who had heard or read a lot or a little about them said they were a major advance.
- Media applications raised the most skepticism. 31 percent of respondents who were familiar with text-to-image generation regarded it as a major advance. Of those who had encountered information about AI’s ability to generate news articles, 16 percent said it was a major advance.
Behind the news: A January 2023 survey by Monmouth University corroborates some of Pew’s findings. 35 percent of that poll’s 805 respondents had heard a lot about recent AI developments. 72 percent believed that news outlets would eventually publish AI-penned news articles. 78 percent thought this would be a bad thing.
Why it matters: As AI matures, it becomes more important to take the public’s temperature on various applications. The resulting insights can guide developers in building products that are likely to meet with public approval.
We’re thinking: The respondents’ familiarity with a given application did not correlate with their acceptance of it. While we should be responsive to what people want, part of our job is to show people the way to a future they may not yet envision — all the more reason for AI builders to follow your interests rather than the latest AI fads.
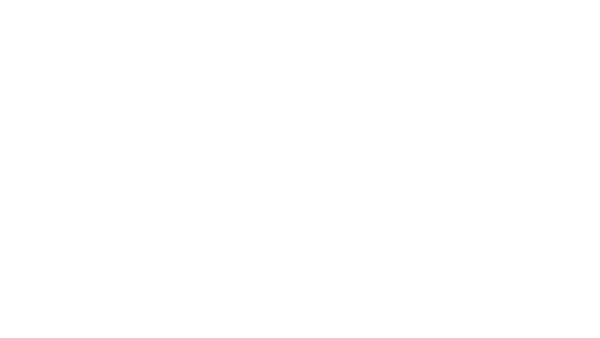
Efficient Reinforcement Learning
Both transformers and reinforcement learning models are notoriously data-hungry. They may be less so when they work together.
What's new: Vincent Micheli and colleagues at the University of Geneva trained a transformer-based system to simulate Atari games using a small amount of gameplay. Then they used the simulation to train a reinforcement learning agent, IRIS, to exceed human performance in several games.
Key insight: A transformer excels at predicting the next item in a sequence. Given the output of a video game, it can learn to estimate a reward for the player’s button press and predict tokens that represent the next video frame. Given these tokens, an autoencoder can learn to reconstruct the frame. Together, the transformer and autoencoder form a game simulator that can help a reinforcement learning agent learn how to play.
How it works: For each of the 26 games in Atari 100k, in a repeating cycle, (i) a reinforcement learning agent played for a short time without learning, (ii) a system learned from the game frames and agent’s button presses to simulate the game, and (iii) the agent learned from the simulation. The total amount of gameplay lasted roughly two hours — 100,000 frames and associated button presses — per game.
- The agent, which comprises a convolutional neural network followed by an LSTM, played the game for 200 frames. It received a frame and responded by pressing a button (randomly at first). It received no rewards and thus didn’t learn during gameplay.
- Given a frame, an autoencoder learned to encode it into a set of tokens and reconstruct it from the tokens.
- Given tokens that represented recent frames and button presses, a transformer learned to estimate the reward for the last button press and generate tokens that represented the next frame. The transformer also learned to estimate whether the current frame would end the game.
- Given the tokens for the next frame, the autoencoder generated the image. Given the image, the agent learned to choose the button press that would maximize its reward.
- The cycle repeated: The agent played the game, generating new frames and button presses to train the autoencoder and transformer. In turn, the autoencoder’s and transformer’s outputs trained the agent.
Results: The authors’ agent beat the average human score in 10 games including Pong. It also beat state-of-the-art approaches that include lookahead search (in which an agent chooses button presses based on predicted frames in addition to previous frames) in six games and those without lookahead search in 13 games. It worked best with games that don’t involve sudden changes in the gaming environment; for instance, when a player moves to a different level.
Why it matters: Transformers have been used in reinforcement learning, but as agents, not as world models. In this work, a transformer acted as a world model — it learned to simulate a game or environment — in a relatively sample-efficient way (100,000 examples). A similar approach could lead to high-performance, sample-efficient simulators.
We're thinking: The initial success of Atari-playing models was exciting partly because the reinforcement learning approach didn’t require building or using a model of the game. A model-based reinforcement learning approach to solving Atari is a surprising turn of events.
Data Points
Research: New tools explore bias in image generators
Tools developed by Hugging Face and Leipzig University help users to detect social biases in three widely used AI image generators. (MIT Technology Review)
U.S. government pledges to prevent monopolies in AI market
Lina Khan, who chairs the Federal Trade Commision, said during an antitrust conference that the agency would protect competition in AI tools to discourage incumbent tech companies from engaging in unlawful tactics. (The Wall Street Journal)
Financial services company Goldman Sachs forecasts the impact of generative AI in the job market
Research led by the firm suggests that AI is likely to boost productivity since employees will focus on more valuable work, adding 1.5% to US labor productivity, and 7% of workers are likely to lose jobs after generative AI reaches half of employers, but most will find nearly equally productive work. (Financial Times)
Research: AI technique helps restore degraded ancient documents
Researchers devised a restoration method that analyzes the color of documents pixel by pixel and highlights spectral differences in layers of information like ink and stamps. (Vice)
Chatbots join U.S. politics’ culture war
AI-powered chatbots’ ability to generate content that conforms to specific ideological viewpoints has raised concerns among researchers, tech executives, and culture warriors. (The New York Times)
Startup Character.AI reached unicorn status upon $150 million funding
The 16-month-old chatbot maker is valued at $1 billion. It was founded by previous developers of Google's LaMDA. (The New York Times)
AI startups make strides in hospitals and drug companies despite accuracy concerns
Several healthcare startups are using generative AI in different medical applications but remain cautious about its use for diagnosing patients or directly providing medical care. (The Wall Street Journal)
Microsoft launched a service that uses ChatGPT to help spot security breaches
Security Copilot is a security field notebook that integrates system data and network monitoring from security tools to help IT teams assess potential security threats faster. The service is powered by OpenAI’s GPT-4 language model. (Wired)
Publishers brace for a battle with tech giants over generative AI tools
Media companies are concerned over ownership of data used to train generative AI systems and lack of compensation for such use of proprietary content by Microsoft and Google’s chatbots. (The Wall Street Journal)