Dear friends,
We here at deeplearning.ai wish you a wonderful holiday season.
As you consider your New Year’s resolutions and set goals for 2020, consider not just what you want to do, but what you want to learn:
- What courses do you want to take this year?
- What books do you want to read?
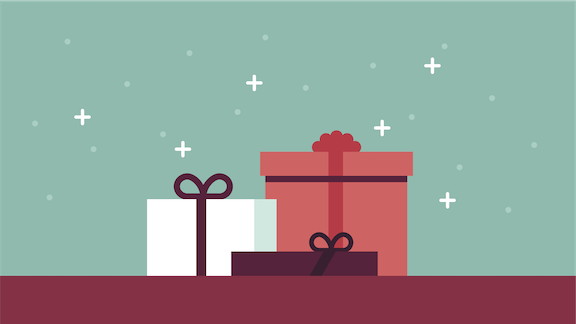
- How many papers do you want to read?
- What meetups or conferences do you want to attend?
I find that people who write down their learning goals are more likely to accomplish them. I do so regularly myself.
Making a list will help set you up for a productive new year. But for now, I hope you are able to rest, reflect with gratitude on things that happened in 2019, and spend time with loved ones.
Keep learning!
Andrew
Farewell to a Landmark Year
2019 will be remembered as a time when AI shifted from fantasy to reality in the public’s perception. Twelve months ago, much of the world equated the technology with the Hollywood dreams of The Terminator, Westworld, and Her. Today, many people understand AI as a tangible force in the world, and they’re having a serious conversation about its impact on society, economics, politics, and the international balance of power. In this issue of The Batch, we revisit the year’s biggest stories in AI.
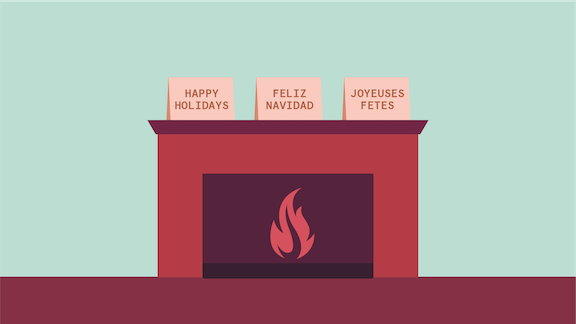
Language Models Get Literate
Earlier language models powered by Word2Vec and GloVe embeddings yielded confused chatbots, grammar tools with middle-school reading comprehension, and not-half-bad translations. The latest generation is so good, some people consider it dangerous.
What happened: A new breed of language models wrote news that readers rated as credible as the New York Times and contributed to an article in the New Yorker. Happily, these models didn’t fulfill fears that they would unleash a dark tide of disinformation.
Driving the story: In 2019, researchers made a leap in natural language performance. The new models become generally proficient by pretraining on a huge, unlabeled dataset. Then they master a given task or subject matter via fine-tuning on a specialized corpus.
- While earlier models like ULMFiT (by Jeremy Howard and Sebastian Ruder) and ELMo (from the Allen Institute for AI and University of Washington) demonstrated pretraining’s potential, Google’s BERT was the method’s first breakout success. Released in late 2018, BERT scored so high on the GLUE reading comprehension benchmark that, for the first time, the test’s organizers compared the model’s performance with human baseline scores. In June, a Microsoft derivative called MT-DNN beat the human scores.
- In mid-February, OpenAI announced GPT-2, a pretrained model it deemed too dangerous to release due to its ability to churn out convincing computer-generated prose. Trained on 40GB of Reddit comments, it didn’t fuel a fake-news apocalypse, but it did contribute to a novel, avant-garde song lyrics, and Game of Thrones fan fiction. The organization finally published the full-blown model in November.
- In between, a parade of models from Baidu, Carnegie Mellon and Google Brain, Facebook, and elsewhere topped the NLP benchmarks in turn. Many were based on the transformer architecture and took advantage of BERT-style bi-directional coding.
Behind the news: In July 2018 — months before BERT came out — DeepMind researcher Sebastian Ruder anticipated pretraining’s impact on natural language processing. Further, he predicted that breakthroughs in NLP would revolutionize AI as a whole. He based his argument on the energizing effect of pretrained vision models circa 2012. Many in the field trace the deep learning explosion to this moment.
Where things stand: Despite the year’s innovations, language models still have room to grow: Even GPT-2’s 1.5 trillion parameters often spit out gobbledygook. As for whether the latest models are capable of disrupting democracy with potent disinformation: U.S. election season is coming up fast.
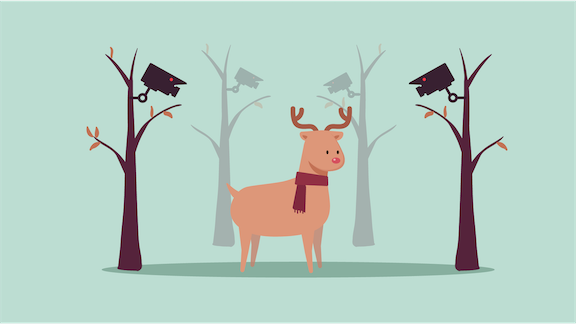
Face Recognition Meets Resistance
An international wave of anti-surveillance sentiment pushed back against the proliferation of face recognition systems.
What happened: Activist and watchdog groups in the U.S. and Europe, alarmed by the technology’s potential to infringe on civil liberties, spurred legislation restricting its use. Their efforts built momentum toward national bans on public and private uses of the technology.
Driving the story: Several U.S. cities passed anti-face recognition laws as the federal government mulled the issues. The European Union is working on its own restrictions.
- In May, San Francisco became the first U.S. city to ban face recognition by police and other government officials, followed by the Boston, MA suburb of Somerville. In the coming months, San Francisco’s neighbors Oakland and Berkeley passed similar laws. These laws were spearheaded by the American Civil Liberties Union, which aims to build momentum for national legislation.
- In Washington, members of the U.S. Congress grilled the Department of Homeland Security over the agency’s plan to use the technology at airports and the border. Legislators in both the Senate and House of Representatives have introduced at least a dozen bills — many with bipartisan support — seeking to restrict uses of face recognition to suppress liberties, deny housing, and generate profit, among other things.
- European watchdogs pushed to classify face images as biometric data subject to existing privacy regulations. The European Commission is considering legislation targeting “indiscriminate use” of face recognition by private organizations and public agencies. Nonetheless, France in October readied a national identification program based on the technology.
- China’s use of face recognition prompted opposition in the U.S., where federal trade authorities banned exports of U.S. technology to several Chinese companies.
Behind the news: In 2016, the U.S. National Telecommunications and Information Administration published face-recognition guidelines asking companies to be transparent, practice good data management, and allow the public some control over sharing of face data with third parties. Although major vendors of the technology are members of the NTIA, it’s not clear whether they follow these guidelines.
Where things stand: In June, Amazon Web Service CEO’s Andy Jassy told Recode, “I wish [Congress would] hurry up. . . . . Otherwise, you’ll have 50 different laws in 50 different states.” He may as well have spoken for the tech industry as a whole: Without legal limits, companies are left guessing how far they can push the technology before they violate public trust — risking blowback if they step over the line.
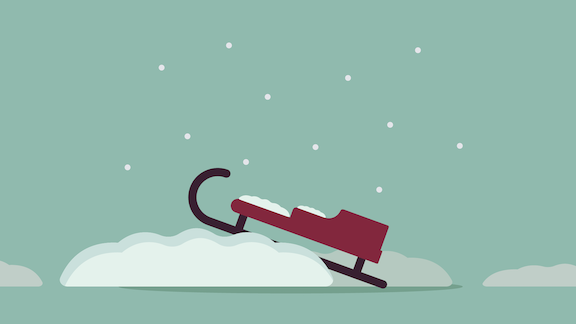
Driverless Cars Stall
Makers of self-driving cars predicted a quick race to the finish line, but their vehicles are far from the homestretch.
What happened: A few years ago, some car companies promised road-ready autonomous vehicles as early as 2017. At a Wall Street Journal conference in January, though, Waymo CEO John Krafcik disclosed his belief that autonomous vehicles would probably never be able to drive in all conditions. His comment set the tone for a year of automotive retrenchment.
Driving the story: A confluence of difficulties prompted several car companies to tap the breaks.
- Urban driving presents hazards so diverse, and dangerous edge cases so rare, that engineers have yet to figure out how to build models that overcome them. Vehicles that traverse predictable routes, such as automated buses and long haul freight trucks, likely will be first to deployment.
- The high cost and limited availability of sensors — particularly lidar — have forced companies to manufacture their own or scale back the number they use on each car. Fewer sensors mean less data for training and perception.
- GM Cruise and Tesla postponed their autonomous taxi deadlines to 2020. The U.S. city of Phoenix gave Waymo and Lyft permission to run autonomous taxis in 2018, but the service is available only to a limited area and a small number of users. In November, Waymo shuttered its Austin self-driving research facility.
Behind the news: Cities in China are experimenting with a different approach. Rather than training autonomous vehicles to navigate existing urban settings, they’re retrofitting cities to facilitate the technology. Features include roadside sensors that pass along navigational cues, like lane changes and speed limits.
Where things stand: Traditional automakers are focusing on assisted driving features like Ford’s Driver Assist and Mercedes’ Parking Assist. Meanwhile, Waymo continues to work on fully autonomous vehicles, and smaller companies such as May Mobility and Voyage are deploying full autonomy in limited scenarios that they aim to expand over time. In parallel, companies such as TuSimple, Embark, and Starsky are concentrating on fully autonomous interstate trucking.
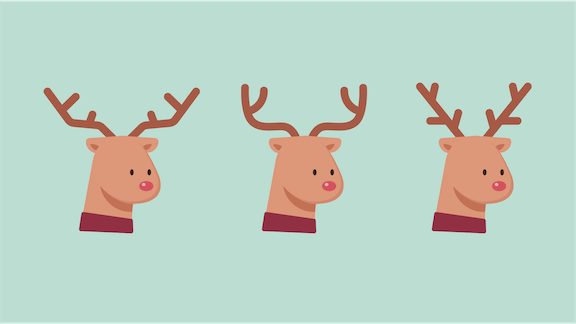
Deepfakes Go Mainstream
Society awakened to the delight, threat, and sheer weirdness of realistic images and other media dreamed up by computers.
What happened: So-called deepfakes became both more convincing and easier to make, stoking a surge of fascination and anxiety that shows every sign of intensifying in the coming year.
Driving the story: Two years ago, the majority of deepfakes were pixelated and difficult to make. Now they’re slicker than ever and improving at a quick clip.
- Late 2018 brought stand-out models like BigGAN, which creates images of the classes found in ImageNet, and StyleGAN, which generates variations such as poses, hairstyles, and clothing. In early 2019, researchers also developed a network that makes realistic talking-head models from a single photo, raising the question of whether people actually said the things you watched them say.
- The technology found positive uses such as making English football star David Beckham appear to deliver an anti-malaria message in nine languages. Chinese tech giant Momo released Zao, an app that maps users’ faces onto characters in scenes from popular movies.
- Yet deepfakes also showed their dark side. Scammers bilked a UK energy company of hundreds of thousands of dollars using fake audio of the CEO’s voice. The technology was implicated in political scandals in Malaysia and Gabon.
- A report by Deeptrace Labs, which sells deepfake detection software, found that 96 percent of deepfake videos online were non-consensual porn — mostly faces of female celebrities rendered on computer-generated naked bodies.
The reaction: Facebook, beset by a fake video of CEO Mark Zuckerberg appearing to gloat at his power over the social network’s members, announced a $10 million contest to automate deepfake detection. Meanwhile, China enacted restrictions on spreading falsified media. In the U.S., the state of California passed a similar law, while the House of Representatives considers national anti-deepfake legislation.
Where things stand: Detecting and controlling deepfakes is shaping up to be a high-tech game of cat and mouse. Although today’s fakes bear telling features, they’ll be indistinguishable from real images within a year, according to USC computer science professor Hao Li.
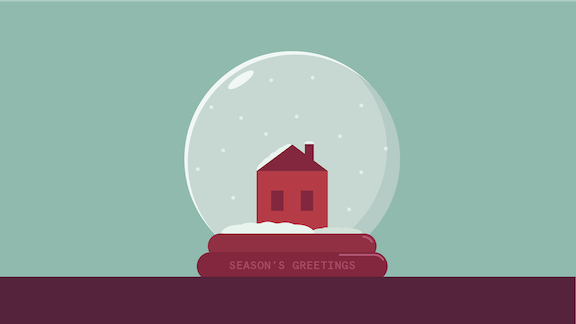
Simulation Substitutes for Data
The future of machine learning may depend less on amassing ground-truth data than simulating the environment in which a model will operate.
What happened: Deep learning works like magic with enough high-quality data. When examples are scarce, though, researchers are using simulation to fill the gap.
Driving the story: In 2019, models trained in simulated environments accomplished feats more complex and varied than previous work in that area. In reinforcement learning, DeepMind’s AlphaStar achieved Grandmaster status in the complex strategy game StarCraft II — able to beat 99.8 percent of human players — through tens of thousands of virtual years competing in a virtual league. OpenAI Five similarly trained a team of five neural nets to best world champions of Dota 2. But those models learned in a virtual world to act in a virtual world. Other researchers transferred skills learned in simulations to the real world.
- OpenAI’s Dactyl robot hand spent the simulated equivalent of 13,000 years in virtual reality developing the dexterity required to manipulate a Rubik’s Cube puzzle. Then it applied those skills to a physical cube. It was able to solve the puzzle in 60 percent of tries when unscrambling the colored sides required 15 or fewer twists of the cube. Its success rate dropped to 20 percent when solving the puzzle required more moves.
- Researchers at CalTech trained a recurrent neural network to differentiate overlapping and simultaneous earthquakes by simulating seismic waves rippling across California and Japan and using the simulations as training data.
- Amazon’s Aurora self-driving vehicle unit runs hundreds of simulations in parallel to train its models to navigate urban environments. The company is training Alexa’s conversational faculties, delivery drones, robots for its fulfillment centers in a similar way.
Where things stand: Simulation environments like Facebook’s AI Habitat, Google’s Behavior Suite for Reinforcement Learning and OpenAI’s Gym offer resources for mastering tasks like optimizing textile production lines, filling in blank spots in 3D imagery, and detecting objects in noisy environments. On the horizon, models could explore molecular simulations to learn how to design drugs with desired outcomes.
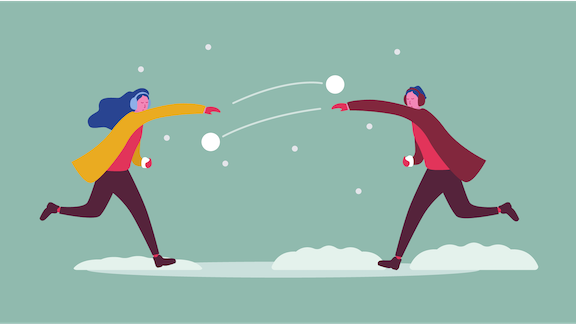
A Smoldering Conflict Flares
A year-long Twitter feud breathed fresh life into a decades-old argument over AI’s direction.
What happened: Gary Marcus, a New York University professor, author, entrepreneur, and standard bearer of logic-based AI, waged a tireless Twitter campaign to knock deep learning off its pedestal and promote other AI approaches.
Driving the story: Marcus’ incessant tweets reignited an old dispute between so-called symbolists, who insist that rule-based algorithms are crucial to cognition, and connectionists, who believe that wiring enough neurons together with the right loss function is the best available path to machine intelligence. Marcus needled AI practitioners to reacquaint itself with the symbolist approach lest connectionism’s limitations precipitate a collapse in funding, or AI winter. The argument prompted sobering assessments of AI’s future and culminated in a live debate on December 23 between Marcus and deep learning pioneer and Université de Montréal professor Yoshua Bengio. The conversation was remarkably civil, and both participants acknowledged the need for collaboration between partisans on both sides.
- Marcus kicked off his offensive in December 2018 by challenging deep learning proponents over what he termed their “imperialist” attitude. He went on to goad Facebook’s Yann LeCun, a deep learning pioneer, to choose a side: Did he place his faith in pure deep learning, or was there a place for good old-fashioned AI?
- OpenAI made headlines in October with a hybrid model. Its five-fingered robot hand solved the Rubik’s Cube puzzle through a combination of deep reinforcement learning and Kociemba’s algorithm. While Marcus pointed out that Kociemba, not deep learning, computed the solution, others asserted that the robot could have learned this skill with further training.
- Microsoft stepped into the breach in December with what it calls neurosymbolic AI, a set of model architectures intended to bridge the gap between neural and symbolic representations.
- As the year drew to a close, the NeurIPS conference highlighted soul searching in the AI community. “All of the models that we have learned how to train are about passing a test or winning a game with a score, [but] so many things that intelligences do aren’t covered by that rubric at all,” Google researcher Blaise Agüera y Arcas stated in a keynote.
Behind the news: Animosity between the symbolists and connectionists dates back more than a half-century. Perceptions, a 1969 broadside against early neural networks, helped trigger the first AI winter. The second, nearly two decades later, came about partly because symbolic AI relied on LISP computers that became obsolete with the advent of personal computers. Neural nets began to gain ground in the 1990s and achieved dominance amid the last decade’s explosion of computing power and data.
Where things stand: We look forward to exciting times ahead as connectionists and symbolists put their heads together, or until one faction wipes out the other.