Dear friends,
Last week, Silicon Valley Bank (SVB), Signature Bank, and Silvergate Bank suddenly collapsed. If it passed uneventfully from your point of view, good for you! Many companies worked nonstop through the weekend scrambling to preserve funds so they could pay their employees.
Numerous tech startups and small businesses bank at SVB, and many are among the business pioneers who are bringing AI to market. For example, when AI Fund, which I lead, works with entrepreneurs to build new companies, we used to help them set up accounts with SVB.
Last Wednesday, SVB announced a $1.8 billion loss. The next morning, rumors began circulating via text, email, and Slack about a bank run in which customers were withdrawing funds en masse. When this happens, depositors can lose money they’ve saved beyond the $250,000 limit the FDIC (a U.S. government agency) guarantees. Without access to their money, companies can’t pay employees who are counting on a paycheck to cover expenses. A permanent loss of funds would lead to numerous layoffs and company shutdowns.
While navigating the collapse of SVB, I was fortunate to be able to call on friends and allies. Several CEOs of AI Fund portfolio companies share a Slack channel and have pre-existing relationships, so none of us felt alone. We were able to share information, make introductions to new banks, and lean in to help each other. Over the weekend, the AI Fund team went to many CEOs and pledged funds from AI Fund’s management company to make sure they could cover their payrolls.
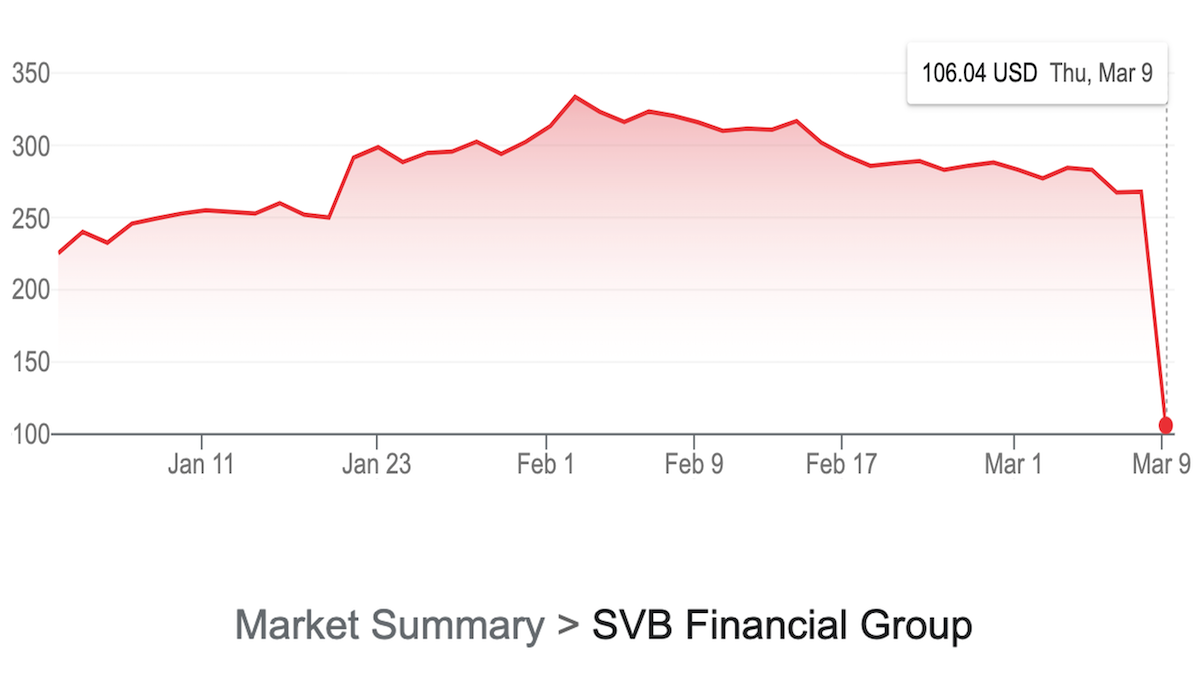
I also saw the best of the AI and tech worlds last week beyond the AI Fund ecosystem. As new information developed, executives at many companies shared it across their networks, and we worked our way through the crisis cooperatively. I’m grateful that we were able to face the storm together.
On Sunday, the U.S. government wisely announced that it would protect all depositors’ assets. This calmed the crisis and helped to head off a domino effect of further bank failures.
Candidly, I was stressed from Thursday through the weekend about the fate of numerous people and companies. And I know that this is not the end of the challenges. Here’s what life has been like for an AI innovator in recent years (h/t @ChrisJBakke):
- 2020: Let’s see you handle a pandemic!
- 2021: Deep learning has diminishing returns.
- 2022: Generative AI is here! Time for massive FOMO.
- 2023: Your bank shut down.
I expect life to be equally dynamic in the future as well — hopefully with more ups than downs. But the fact that many people in AI have a network of trusted friends will enable us to react quickly and work together to benefit everyone.
Keep learning!
Andrew
News
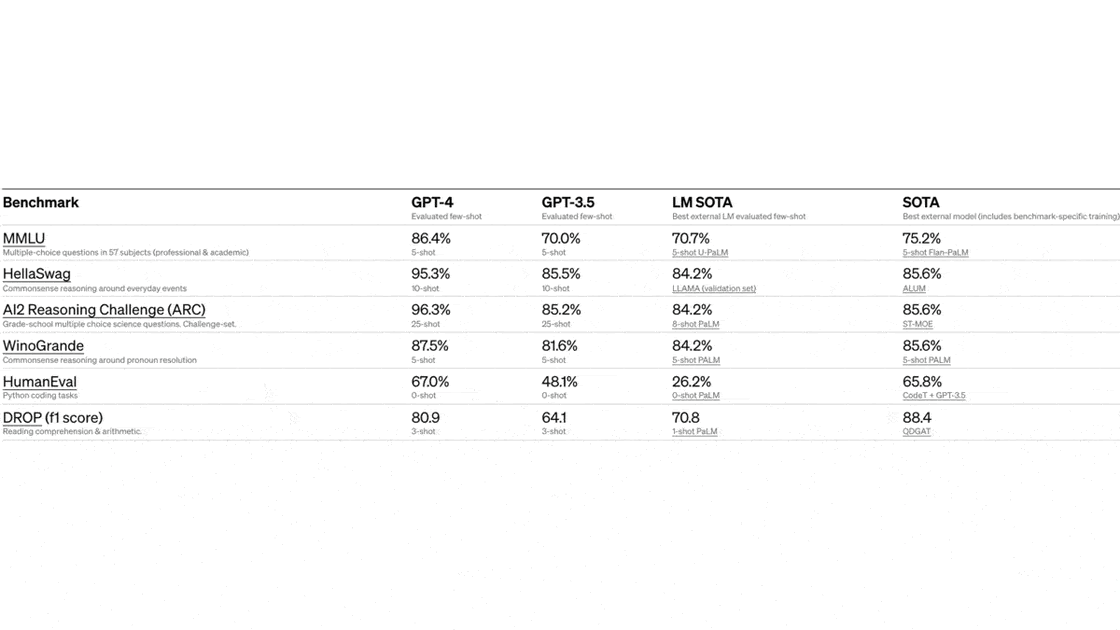
GPT-4 Has Landed
Get ready for the next wave of language-model mania.
What’s new: OpenAI introduced the latest in its GPT series of large language models to widespread excitement. The company showed statistics and examples designed to demonstrate that the new model outstrips its predecessors in its language comprehension as well as its ability to adopt a desired style and tone and stay within bounds imposed by its designers. OpenAI co-founder Greg Brockman showed off some of its capabilities in a livestream that accompanied the launch.
How to get access: Text input/output is available via ChatGPT Plus, which costs $20 monthly, with image input to come. An API is forthcoming, and you can join the waitlist here.
How it works: OpenAI didn’t share many details, citing concerns about safety and competition. Like earlier GPT models, GPT-4 is based on the transformer architecture and trained to predict the next token on a mix of public and private datasets. It was fine-tuned using reinforcement learning from human feedback and engineered prompts.
- OpenAI is keeping mum about the precise architecture (including size), datasets, training procedure, and processing requirements.
- GPT-4 processes 32,000 tokens at a time internally, Brockman said — an order of magnitude more than estimates of ChatGPT’s token count — which enables it to work with longer texts than previous large language models.
- The model accepts image inputs including pages of text, photos, diagrams, and screenshots. (This capability isn’t yet publicly available because the company is still working to speed it up, Brockman said.) In one example, GPT-4 explained the humor in a photo of an iPhone whose sleek Lightning port had been adapted to accommodate a hulking VGA connector.
- A new type of input called a system message instructs the model on the style, tone, and verbosity to use in subsequent interactions. For example, a system message can condition the model to respond in the style of Socrates, encouraging users to arrive at their own answers through critical thinking.
- The company offers a new framework, OpenAI Evals, for creating and running benchmarks. It invites everyone to help test the model.
How it performs: GPT-4 aced a variety of AI benchmarks as well as simulated versions of tests designed for humans.
- GPT-4 outperformed the state of the art on MMLU multiple-choice question answering, HellaSwag common sense reasoning, AI2 grade-school multiple-choice science question answering, WinoGrande common-sense reasoning, HumanEval Python coding, and DROP reading comprehension and arithmetic.
- It exceeded GPT-3.5, Chinchilla, and PaLM English-language performance in 24 languages from Afrikaans to Welsh.
The model met or exceeded the state of the art in several vision benchmarks in TextVQA reading text in images, ChartQA, AI2 Diagram, DocVQA, Infographic VQA, and TVQA. - GPT-4 achieved between 80 and 100 percent on simulated human tests including the Uniform Bar Exam, LSAT, SAT, and advanced placement tests in biology, psychology, microeconomics, and statistics.
- GPT-4 jumps its guardrails when asked about disallowed topics like how to obtain dangerous substances roughly 1 percent of the time, while GPT-3.5 does so around 5 percent of the time. Similarly, GPT-4 misbehaves when asked about sensitive topics such as self-harm around 23 percent of the time, while GPT-3.5 does so around 42 percent of the time.
Where it works: Several companies are already using GPT-4.
- OpenAI itself has been using the model for content moderation, sales, customer support, and coding.
- The updated Microsoft Bing search, which launched last month, is based on GPT-4.
- Stripe uses GPT-4 to scan and write summaries of business websites.
- Paid subscribers to Duolingo can learn languages by conversing with GPT-4.
Yes, but: OpenAI doesn’t mince words about the new model’s potential to wreak havoc: “While less capable than humans in many real-world scenarios . . . GPT-4's capabilities and limitations create significant and novel safety challenges.” While the model outperformed its predecessors in internal adversarial evaluations of factual correctness, like other large language models, it still invents facts, makes reasoning errors, generates biased output, and couches incorrect statements in confident language. In addition, it lacks knowledge of events that transpired after September 2021, when its training corpus was finalized. OpenAI details the safety issues here.
Why it matters: As language models become more capable, they become more useful. It’s notable that OpenAI believes this model is ready to commercialize from the get-go: This is the first time it has introduced a new model alongside product launches that take advantage of it. Â
We’re thinking: Stable Diffusion, Phenaki, MusicLM, GPT-4: This is truly a golden time in AI!
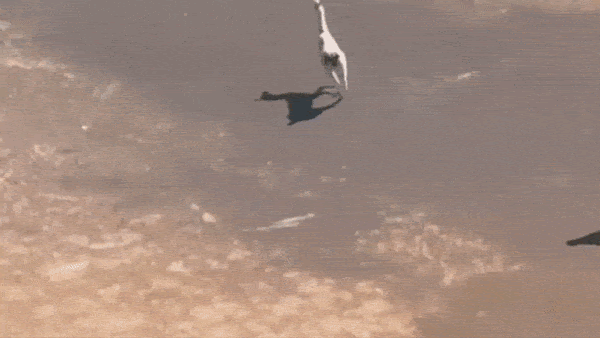
Runaway LLaMA
Meta’s effort to make a large language model available to researchers ended with its escape into the wild.
What’s new: Soon after Meta started accepting applications for developer access to LLaMA, a family of trained large language models, a user on the social network 4chan posted a downloadable BitTorrent link to the entire package, The Verge reported.
How it works: LLaMA includes transformer-based models with 7 billion, 13 billion, 33 billion, and 65 billion parameters. The models were trained on Common Crawl, GitHub, Wikipedia, Project Gutenberg, ArXiv, and Stack Exchange. Tested on 20 zero- and few-shot tasks, LLaMA outperformed GPT-3 on all tasks, Chinchilla on all but one, and PaLM on all but two.
Escape: On March 24, Meta had offered LLaMA to researchers at institutions, government agencies, and nongovernmental organizations who requested access and agreed to a noncommercial license. A week later, 4chan leaked it.
- Users promptly hosted the model on sites including GitHub and Hugging Face. Meta filed takedown requests.
- Users adapted it to widely available hardware. One ran the 65 billion-parameter model on a single Nvidia A100. Computer scientist Simon Willison implemented the 13 billion-parameter version on a MacBook Pro M2 with 64 gigabytes of RAM.
- Alfredo Ortega, a software engineer and user of 4chan, which is infamous for hosting objectionable content, implemented the 13 billion-parameter LLaMA as a Discord chatbot. Users have prompted the program (nicknamed BasedGPT) to output hate speech. Ortega noted that his implementation was a legitimate download.
Behind the news: Efforts to release similar models are ongoing even as the AI community continues to debate the potential risks and rewards. Those who favor limited access cite safety concerns believe that institutions are best positioned to study models and learn to control them. Proponents of open access argue that free enquiry offers the best route to innovation and social benefit.
Why it matters: LLaMA gives experimenters, small developers, and members of the general public unprecedented access to cutting-edge AI. Such access likely will enable valuable scientific, practical, and commercial experimentation. While the risk of harm via automated generation of effective spam, scams, propaganda, disinformation, and other undesirable outputs is real, open source projects like BLOOM and GPT-NeoX-20B have led to significantly more benefit than harm — so far.
We’re thinking: Making models like LLaMA widely available is important for further research. Ironically, bad actors will use the leaked LLaMA, while conscientious researchers will respect Meta’s copyright and abide by the rules. For instance, Stanford researchers announced Alpaca, a LLaMA variant that’s fine-tuned to follow instructions. However, the Stanford team is holding back the trained weights while it discusses the matter with Meta. Considering the potential benefits and harms of restricted release versus openness, openness creates more benefits all around.
A MESSAGE FROM FOURTHBRAIN
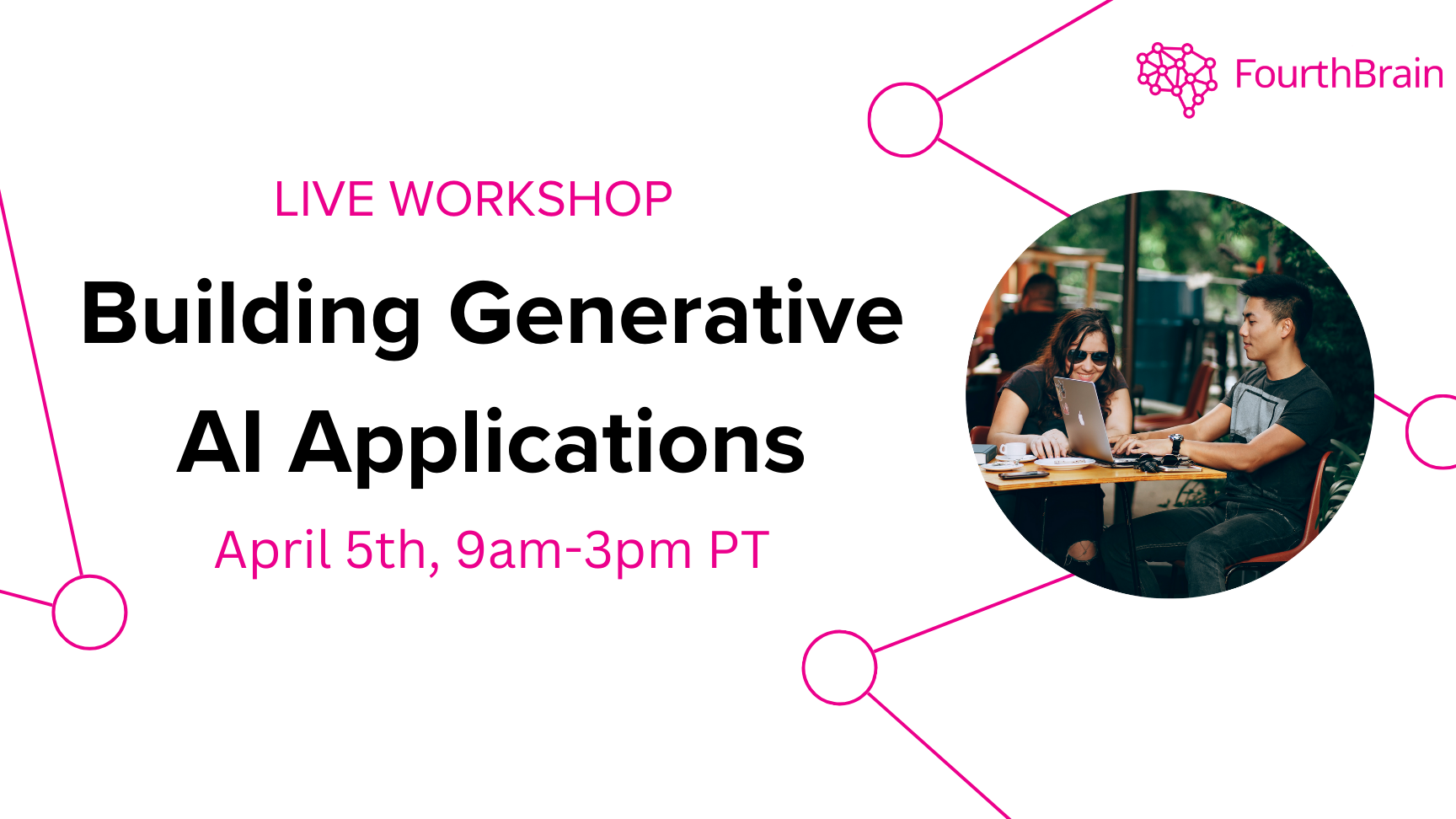
Learn how to build and deploy an end-to-end application using open source generative AI tools at a one-day workshop with FourthBrain. Join us on April 5, 2023, from 9 a.m. to 3 p.m. Pacific Time! Team registrations available! Register now
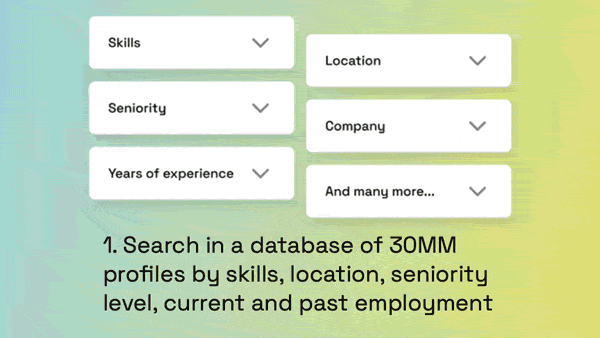
Inferring Talent
What do your GitHub projects reveal about your professional prospects? A new model aims to help recruiters find out.
What’s new: Prog.ai analyzes GitHub repositories to help employers find engineers skilled in particular areas, TechCrunch reported. The beta-test version is available by invitation only, but recruiters can join a waitlist for forthcoming free, professional, and enterprise service tiers.
How it works: The company fine-tuned OpenAI’s GPT-3 on GitHub projects, LinkedIn resumes, and StackOverflow articles to evaluate prospective recruits.
- The model copies millions of GitHub repositories and branches. It analyzes each commit and inspects code snippets, file paths, and subjects.
- It examines the code and evaluates pull requests, rejections, and so on to infer the participants’ roles, noting core architects, frontend and backend developers, UI/UX developers, QA and test engineers, and technical writers.
- The system matches participants’ GitHub profiles with their LinkedIn pages to align their projects and employment histories.
- Recruiters can search according to characteristics like area of expertise, years of experience, programming languages, and skills. They can reach out to prospects via an integrated contact manager.
- Prog.ai says it complies with European data privacy laws. Developers can opt out of being contacted by recruiters, edit their profiles, or delete their profiles.
Behind the news: Machine learning is already involved in hiring at many companies. 63 percent of employers and 99 percent of Fortune 500 corporations in the U.S., UK, and Germany used automated systems to screen resumes and cover letters, according to a 2021 study by Accenture and Harvard Business School. However, some hiring systems have been shown to exhibit bias. A forthcoming European Union law aims to regulate certain types of algorithms, including those that control hiring.
Why it matters: Spotting the right talent for a particular position is hard, and getting harder as technical skills proliferate worldwide. If AI can do it efficiently, it may help fill open positions more effectively and distribute opportunities more evenly among the global pool of applicants.
We’re thinking: While building a portfolio of projects that reflect your skills and interests can help you get an interview, winning the job often comes down to soft skills like interviewing. To learn more, download our free ebook, How to Build Your Career in AI.
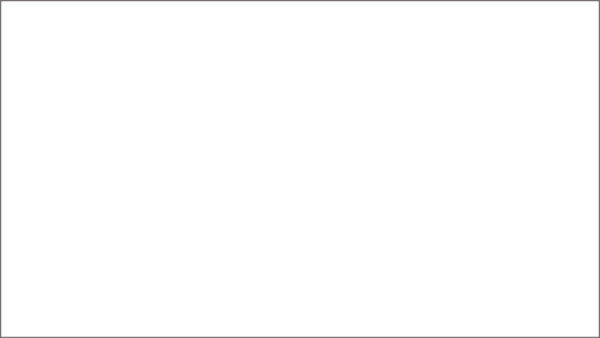
Vision and Language Tightly Bound
Recent multimodal models process both text and images as sequences of tokens, but they learn to represent these distinct data types using separate loss functions. Recent work unifies the loss function as well.
What’s new: Wenhui Wang, Hangbo Bao, Li Dong, and colleagues at Microsoft introduced BEiT-V3, a transformer pretrained on a large amount of image, text, and paired image-text data. The model set a new state of the art in several vision-language tasks. This work updates the earlier BEiT and BEiT v2.
Key insight: MoME transformer (which the authors call Multiway) processes image, text, and text-image pairs using different fully connected layers for different data types, but the same self-attention layers for all. The authors who proposed that architecture trained it using a different task and loss function for text and image data. However, pretraining it on a single task and loss function for all data types — specifically, generating masked portions of the data — enables the shared self-attention layers to learn common patterns across data types, creating similar embeddings for similar images and texts.
How it works: BEiT-V3 is a 1.9 billion parameter MoME transformer.
- The authors pretrained the model to regenerate randomly masked input tokens in the 15 million images in ImageNet-21k, 160 gigabytes of internet text, and roughly 38 million image-text pairs (a combination of datasets) including COCO.
- They fine-tuned it for five vision-language tasks, such as identifying an object in an image based on a description (NLVR2), and four vision tasks such as ImageNet classification and COCO object detection and segmentation.
Results: BEiT-V3 outperformed baseline models across all nine tasks. On ImageNet, it achieved top-1 accuracy of 89.6 percent, beating the previous state of the art, 89 percent, achieved by FD-CLIP. On NLVR2, its accuracy was 92.6 percent accuracy, while the next-best model, CoCa, achieved 87 percent.
Why it matters: Sometimes great performance lies in a combination of tried-and-true techniques. BEiT-3 takes advantage of (a) the MoME architecture, (b) masked pretraining (which has achieved excellent fine-tuned performance on text, images, and text-image pairs), and (c) a large quantity of data (which has been shown to yield high performance).
We’re thinking: If earlier vision-language models are obsolete, so BEiT!
Data Points
Bank of America forecasts AI will have a $15.7 trillion impact by 2030
The banking giant foresees a bright future for AI, as key trends  evolve rapidly. (Business Insider)
A Colombian judge used ChatGPT in court ruling
The chatbot helped the judge interpret the law in a dispute between the guardian of an autistic child and a health insurance company. (The Guardian)
Generative AI is boosting business productivity apps
Startups and big tech companies alike are riding a wave of AI-powered productivity services like writing emails, designing presentations, and crafting marketing messages. (VentureBeat)
European Union faces challenges to regulate AI in the ChatGPT era
The chatbot poses challenges for the EU’s draft regulation on AI, the Artificial Intelligence Act. While the law covers isolated applications, ChatGPT can be used for a wide variety of applications, some of the law encourages and some of which the law restricts. (Politico)
DuckDuckGo launched the AI feature DuckAssist
The search engine now includes a model that generates summaries of Wikipedia articles for certain queries. (The Verge)
Google goes all-in on AI to catch up with ChatGPT
Google plans to integrate AI into all of its products and services, in response to the threat of ChatGPT and other AI-powered chatbots. (Bloomberg)
U.S. Chamber of Commerce urges AI regulation
The organization said that AI technology needs to be regulated due to its influence in matters like the global economy and national security. (Reuters)
Baidu’s AI chatbot is facing challenges before launch
Ernie Bot, Baidu’s bid to compete with other AI-powered chatbots, is set for release on March 16, but employees say it has numerous issues. (The Wall Street Journal)