Dear friends,
A new report from UN Climate Change says that the world might be on track for 2.5 °C of warming by the end of the century, a potentially catastrophic level of warming that’s far above the 1.5 °C target of the 2015 Paris Agreement. I think it is time to seriously consider a specific solution in which AI can play a meaningful role: Climate geoengineering via stratospheric aerosol injection.
Stratospheric aerosol injection involves spraying fine particles that reflect sunlight high in the atmosphere. By increasing the reflectivity (or albedo) of the planet, we can slow down the rate at which sunlight warms it, and thereby buy more time to reduce carbon emissions and develop mitigations. Harvard Professor David Keith explains the science behind this idea is in his book, A Case for Climate Engineering.
AI will be important in this effort because:
- The aerosols will likely be delivered via custom aircraft. Designing the specs for and autonomously piloting high-altitude drones falls well within AI capabilities.
- The details of the aerosols’ impact on the planet’s climate are still poorly understood. Average temperature should decrease, but will some regions cool faster? Will some continue to warm? How will this affect crops, rain acidity, wind currents, and myriad other factors? Machine learning will be critical for modeling the effects.
- In light of the likely impact of stratospheric aerosols on the climate as well as their potential for disparate impact, how can we decide which aerosols to use, where, and when in a way that’s equitable and improves the welfare of the planet as a whole? Optimization techniques akin to reinforcement learning could be useful.
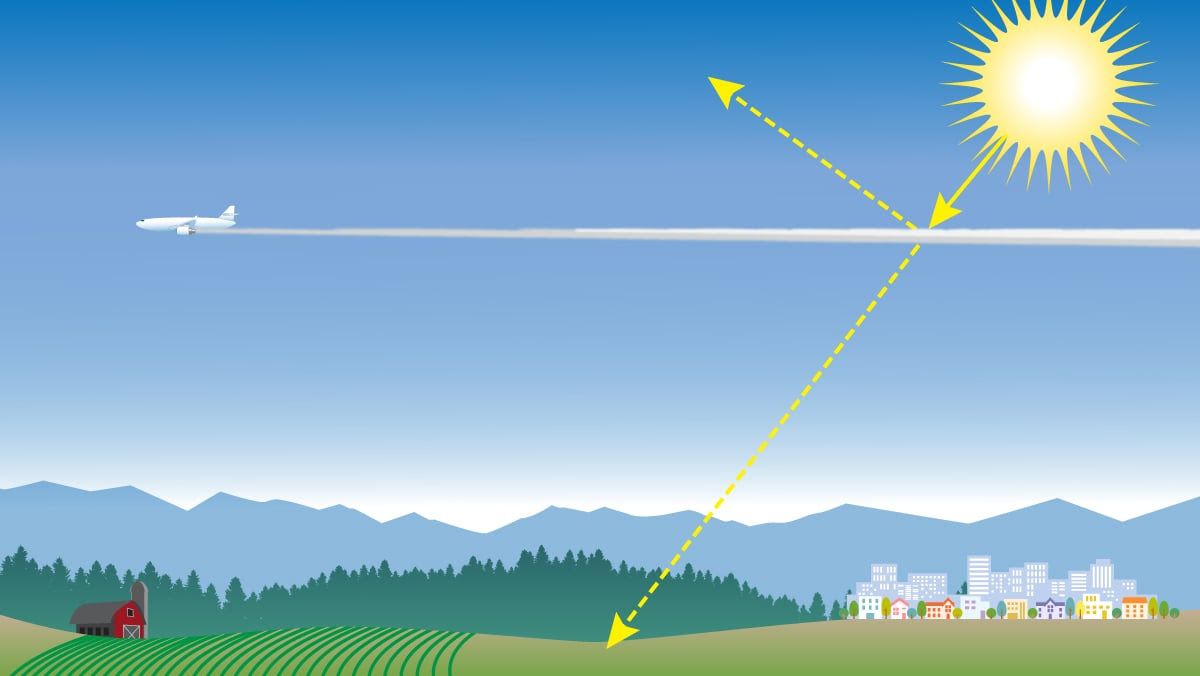
Stratospheric aerosol injection has been criticized on the following grounds:
- Moral hazard: Doing this will reduce the incentive to reduce carbon emissions. This is true, just as requiring seat belts reduces the incentive to drive safely. Nonetheless, we’re better off with seatbelts.
- Unforeseen risks: How can we attempt something as risky as modifying the planet? What if it goes wrong? But we already have modified the planet, and it already has gone wrong. Let’s do it intentionally this time, with careful science that enables us to take baby steps that are safe.
At the current 1.1 °C of warming, the world is already experiencing increased climate-related crises. My heart goes out to the millions whose lives have been disrupted by wildfires, flooding, hurricanes, and typhoons. Just weeks ago, a forest fire came within miles of my house, and area residents were told to be ready to evacuate, a first for me. (Fortunately, the fire has since been largely contained.) It terrifies me that on the planet’s current path, the past summer’s climate — the worst I’ve experienced — might be better than what my children and I will experience for the rest of our lives.
Next week at the UN’s annual COP27 climate summit held in Egypt, government leaders will meet to discuss new agreements aimed at reducing atmospheric carbon emissions. While I hope that this meeting summons the global will to do what’s needed, I would rather count on engineers and scientists, not just politicians, to address the problem. Perhaps some of us in AI can make a critical difference.
Stay cool,
Andrew
News
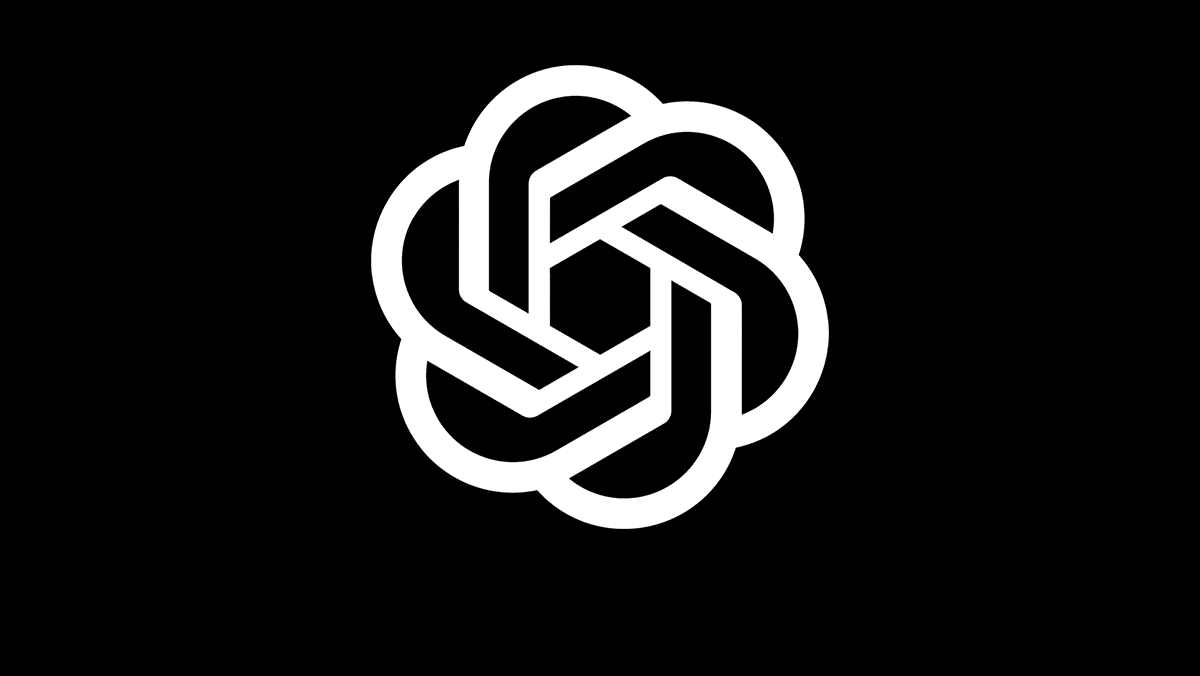
Generating Investment
The generative gold rush is on.
What’s new: Venture capitalists are betting hundreds of millions of dollars on startups that use AI to generate images, text, and more, Wired reported.
What’s happening: A handful of generative-AI startups have newly received nine-figure investments. They’re among over 140 nascent companies that aim to capitalize on applications in copywriting, coding, gaming, graphic design, and medicine, according to a growing list maintained by Stanford student David Song.
- Stability AI, the London-based company behind the open-source text-to-image generator Stable Diffusion, raised over $100 million in a seed round that valued the firm at $1 billion. It plans to use the funds to develop infrastructure for DreamStudio, a commercial version of its text-to-image model, and triple the size of its workforce, which currently numbers around 100.
- Jasper, which caters to the content-creation market, raised a $125 million Series A round. It offers a Chrome browser extension based on OpenAI’s GPT-3 language model that generates copywriting suggestions ranging from a single word to an entire article. The company boasts over 70,000 paying users.
- Microsoft is poised to inject further capital into OpenAI having invested $1 billion in 2019. Google reportedly is considering a $200 million investment into natural language processing startup Co:here.
Behind the news: Established companies, too, are looking for ways to capitalize on AI’s emerging generative capabilities.
- Microsoft is adding DALL·E 2 to its invitation-only Azure OpenAI service, which also includes GPT-3. It’s also integrating the image generator into Designer, an app that automates graphic design for social media and other uses.
- Shutterstock, which distributes stock images, will allow users to generate custom images using DALL·E 2. The company also plans to compensate creators whose work was used to train their service.
- Getty Images, which competes with Shutterstock, is adding AI-powered image editing tools from Bria, an Israeli startup. In September, it banned images that are wholly AI-generated.
Yes, but: Incumbents and class-action lawyers are lodging complaints over who owns what goes into — and what comes out of — models that generate creative works.
- The Recording Industry Association of America recently requested that U.S. regulators add several generative AI web apps for remastering, remixing, or editing music to a watchlist for intellectual property violations.
- Lawyers are preparing a class-action lawsuit against GitHub and Microsoft claiming that CoPilot, a model available on Microsoft’s Azure cloud service that generates computer code, was trained on open-source code without proper attribution.
Why it matters: Despite ongoing chatter about AI winter, it’s springtime for generative AI. Founders, investors, and trade organizations alike believe that this emerging technology has the potential to create huge value.
We’re thinking: Generative AI holds the spotlight, given the mass appeal of models that paint beautiful pictures in response to simple text prompts, but AI continues to advance in many areas that hold significant, unfulfilled commercial promise.
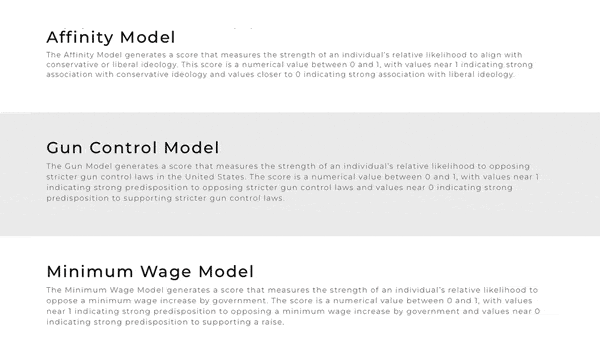
Pushing Voters’ Buttons
As the United States (along with several other countries) gears up for general elections, AI is helping campaigns attract voters with increasing sophistication.
What’s new: Strategists for both major U.S. political parties are using machine learning to predict voters’ opinions on divisive issues and using the results to craft their messages, The New York Times reported.
How it works: Consulting firms typically combine publicly available data (which might include voters’ names, ages, addresses, ethnicities, and political party affiliations) with commercially available personal data (such as net worths, household sizes, home values, donation histories, and interests). Then they survey representative voters and build models that match demographic characteristics with opinions on wedge issues such as climate change and Covid-19 restrictions.
- HaystaqDNA, scores 200 million voters on over 120 politically charged positions. The company helped U.S. president Barack Obama during his successful 2008 campaign.
- i360 scores individuals on their likelihood to support specific laws such as gun control, increasing the minimum wage, and outlawing abortion.
Behind the news: AI plays an increasing role in political campaigns worldwide.
- Both major candidates in South Korea’s presidential election earlier this year used AI-generated avatars designed to connect with voters.
- In 2020, a party leader in an Indian state election deepfaked videos of himself delivering the same message in a variety of local languages.
Yes, but: Previous efforts to predict voter opinions based on personal data have been fraught with controversy. In the mid-2010s, for instance, political advertising startup Cambridge Analytica mined data illegally from Facebook users.
Why it matters: The embrace of machine learning models by political campaigns sharpens questions about how to maintain a functional democracy in the digital age. Machine learning enables candidates to present themselves with a different face depending on the voter’s likely preferences. Can a voter who’s inundated with individually targeted messages gain a clear view of a candidate’s positions or record?
We’re thinking: Modeling of individual preferences via machine learning can be a powerful mechanism for persuasion, and it’s ripe for abuses that would manipulate people into voting based on lies and distortions. We support strict transparency requirements when political campaigns use it.
A MESSAGE FROM DEEPLEARNING.AI
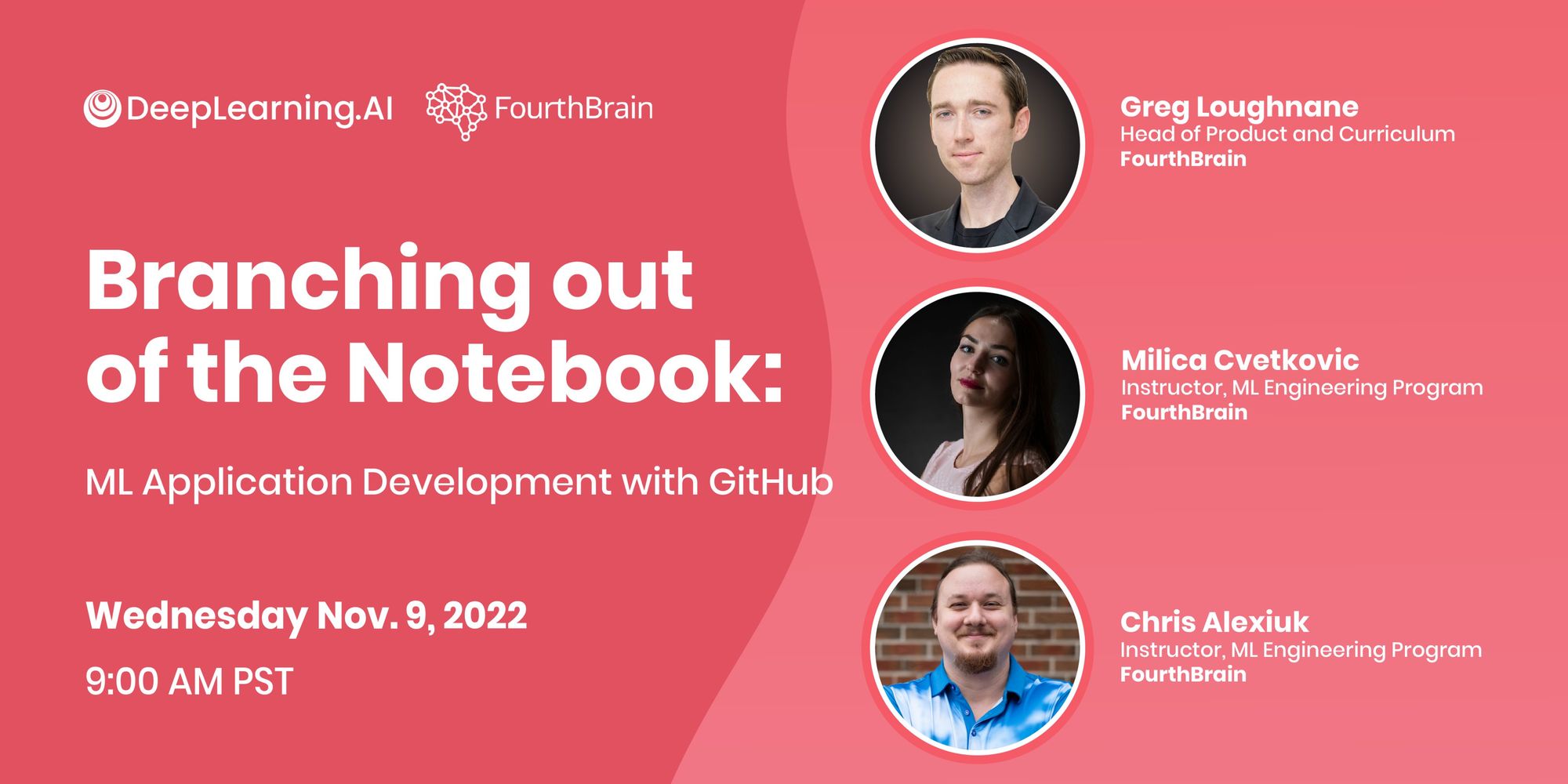
Ready to deploy your own diffusion model? Learn how to create machine learning applications using existing code in a free, hands-on workshop. Join us for “Branching out of the Notebook: ML Application Development with GitHub” on Wednesday, November 9, 2022! Register here
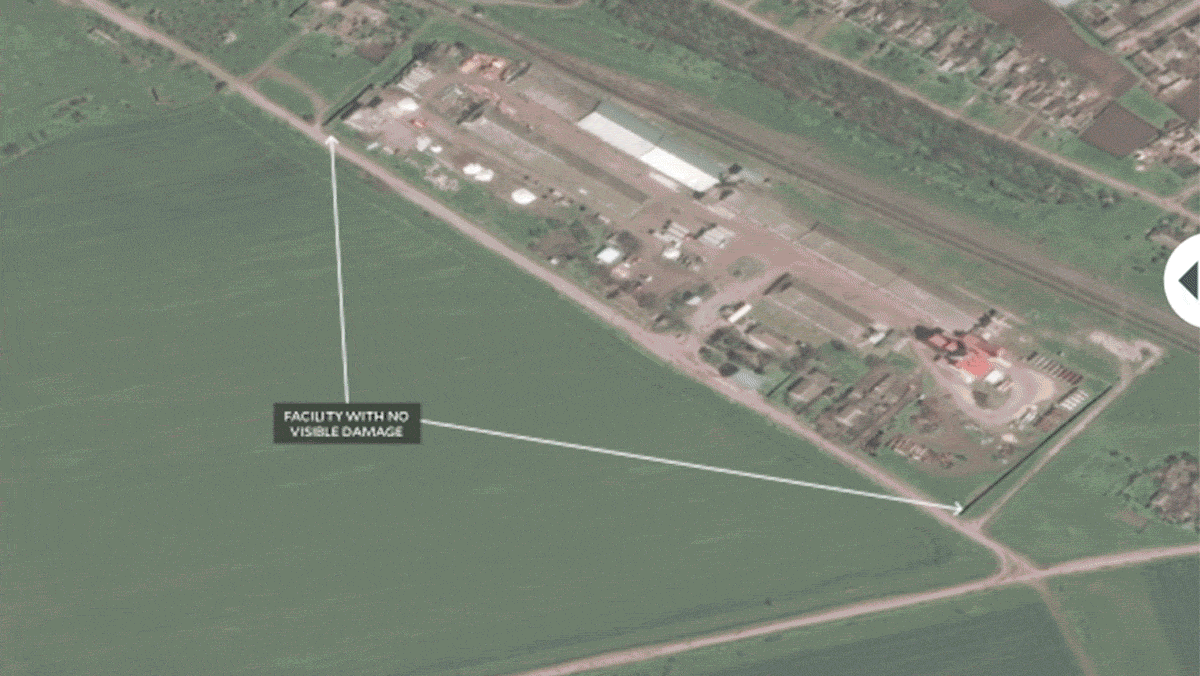
Ukraine’s Lost Harvest Quantified
Neural networks are helping humanitarian observers measure the extent of war damage to Ukraine’s grain crop.
What’s new: Analysts from the Yale School of Public Health and Oak Ridge National Laboratory built a computer vision model that detects grain-storage facilities in aerial photos. Its output helped them identify facilities damaged by the Russian invasion.
How it works: The authors started with a database of grain silos last updated in 2019. They used machine learning to find facilities missing from that survey or built since then.
- The authors used a YOLOv5 object detector/classifier that Yale researchers previously had trained to identify crop silos in images from Google Earth. They fine-tuned the model to identify other types of facilities — grain elevators, warehouses, and the like — in labeled images from commercial satellites.
- In tests, the model achieved 83.6 percent precision and 73.9 percent recall.
- They fed the model 1,787 satellite images of areas in Ukraine that were affected by the conflict, dated after February 24 (the start of the current Russian invasion). The model identified 19 previously uncatalogued crop facilities.
- Having located the grain facilities, the authors evaluated damage manually.
Results: Among 344 facilities, they found that 75 had suffered damage. They estimate that the destruction has compromised 3.07 million tons of grain storage capacity, nearly 15 percent of Ukraine’s total.
Why it matters: Before the war, Ukraine was the world’s fifth-largest wheat exporter. By disrupting this activity, the Russian invasion has contributed to a spike in global food prices, which observers warn may lead to famine. Understanding the scope of the damage to Ukraine’s grain supply could help leaders estimate shortfalls and plan responses.
Behind the news: Machine learning has been applied to a variety of information in the war between Russia and Ukraine. It has been used to verify the identities of prisoners of war, noncombatants fleeing conflict zones, and soldiers accused of committing war crimes. It has also been used to debunk propaganda, monitor the flow of displaced persons, and locate potentially damaged buildings obscured by smoke and clouds.
We’re thinking: War is terrible. We’re glad that AI can help document the damage caused by invading forces, and we hope that such documentation will lead to payment of appropriate reparations.
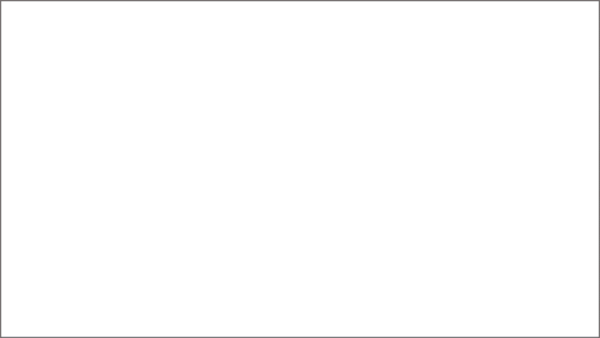
Massively Multilingual Translation
Recent work showed that models for multilingual machine translation can increase the number of languages they translate by scraping the web for pairs of equivalent sentences in different languages. A new study radically expanded the language repertoire through training on untranslated web text.
What’s new: Ankur Bapna, Isaac Caswell, and colleagues at Google collected a dataset of untranslated text that spans over 1,000 languages. Combining it with existing multilingual examples, they trained a model to translate many languages that are underrepresented in typical machine translation corpora.
Key insight: Neural networks typically learn to translate text from multilingual sentence pairs, known as parallel data. Generally this requires examples numbering in the millions, which aren’t available for the vast majority of language pairs. However, neural networks can also learn from untranslated text, also known as monolingual data, by training them to fill in a missing word in a sentence. Combined training on parallel and monolingual data — carefully filtered — can enable a model to translate among languages that aren’t represented in parallel data.
How it works: The authors scraped web text, classified the languages in it, and combined what was left with existing monolingual data. Separately, they used an established corpus of parallel data. Then they trained a transformer on the monolingual and parallel datasets.
- The authors trained a CLD3 vanilla neural network on an existing monolingual dataset to classify languages.
- The CLD3 classified 1,745 languages in the scraped text. The authors removed the languages that proved most difficult to classify. They combined the remainder with existing data to produce a monolingual corpus of 1,140 languages.
- They eliminated languages that the CLD3 had frequently confused with a different language. They removed sentences that the CLD3 (or a more computationally expensive language classifier) had failed to classify either correctly or as a related dialect. They also discarded sentences in which fewer than 20 percent of the words were among the language’s 800 most frequently used terms. Then they discarded languages for which the available text included fewer than 25,000 sentences. Finally, a team of native speakers designed criteria to remove sentences of closely related languages.
- They trained a transformer to fill in missing parts of sentences in the monolingual data. Simultaneously, they trained it to translate examples in an existing parallel dataset that comprised 25 billion sentence pairs in 102 languages. This enabled the transformer to render a rough English translation from any language in the corpora.
- Continuing to train the model on both monolingual and parallel data, the authors added parallel data formed by pairing monolingual text with translations generated by the model. In learning to translate (noisy) model-translated text into ground-truth text, the model learned to handle faulty grammar and usage. It also learned to translate from clean to noisy text. This forced it to translate among various languages more consistently and helped to avoid drastic, possibly damaging model updates.
Results: The authors compared their 1,000-language model with a version trained on 200 languages. Given a test set that comprised 38 languages, the 1000-language model performed better on most of them (including those for which plenty of training data was available), which suggests that greater language diversity was beneficial. When translating all languages into English, the 1000-language model outperformed the 200-language version by 2.5 CHRF points, a measure of overlap among groups of characters between generated and ground-truth translations. Translating from English to other languages, the 1,000-language version outperformed its 200-language counterpart by an average of 5.3 CHRF points.
Why it matters: Previous research cautioned against using monolingual data to expand a translator’s language repertoire. It was thought that training in languages that were less well-represented in the dataset would diminish performance on better-represented ones. Yet this model, trained largely on monolingual data, performed well across a variety of languages. The authors hypothesize that, once a model learns a critical number of languages, additional languages are helpful because they’re likely to share similarities with those the model already knows about.
We’re thinking: The authors went out of their way to filter out less-useful training data. Their results show that scraping the web indiscriminately only gets you so far. Rigorous curation can make a big difference.